Notes
Article history
The research reported in this issue of the journal was funded by the PHR programme as project number 12/211/82. The contractual start date was in September 2014. The final report began editorial review in April 2018 and was accepted for publication in October 2018. The authors have been wholly responsible for all data collection, analysis and interpretation, and for writing up their work. The PHR editors and production house have tried to ensure the accuracy of the authors’ report and would like to thank the reviewers for their constructive comments on the final report document. However, they do not accept liability for damages or losses arising from material published in this report.
Declared competing interests of authors
Frank Kee, Emma McIntosh and David French are members of the Public Health Research (PHR) programme Funding Board. Frank Kee is also a member of the PHR Prioritisation Group. David French reports personal fees from the Medical Research Council and from the British Psychological Society, grants from the National Institute for Health Research (NIHR), grants from the Medical Research Council, Greater Sport charity (Manchester, UK), the European Commission, Prevent Breast Cancer (Manchester, UK), the Natural Environment Research Council, the Multiple Sclerosis Society and Cancer Research UK, outside the submitted work. Jean Adams reports that her partner is the Director of the NIHR’s PHR programme, which funded this research.
Permissions
Copyright statement
© Queen’s Printer and Controller of HMSO 2019. This work was produced by Hunter et al. under the terms of a commissioning contract issued by the Secretary of State for Health and Social Care. This issue may be freely reproduced for the purposes of private research and study and extracts (or indeed, the full report) may be included in professional journals provided that suitable acknowledgement is made and the reproduction is not associated with any form of advertising. Applications for commercial reproduction should be addressed to: NIHR Journals Library, National Institute for Health Research, Evaluation, Trials and Studies Coordinating Centre, Alpha House, University of Southampton Science Park, Southampton SO16 7NS, UK.
2019 Queen’s Printer and Controller of HMSO
Chapter 1 Introduction
Rationale for current study
Previously, the authors completed a pilot study of the feasibility of conducting a randomised controlled trial (RCT) investigating the use of financial incentives for physical activity in the workplace,1 which assessed the recruitment process and retention of office-based employees to a trial, tested outcome measures and data collection processes and assessed the feasibility of programme implementation in a public sector organisation. In the pilot study, a high uptake rate (63% of those invited took part) indicated that our recruitment strategy was successful and that the intervention was acceptable to the target population. Our data showed that > 50% of participants recruited at baseline were categorised as having low physical activity levels and that the intervention was acceptable to a wide range of individuals, including those currently physically inactive, with the potential for significant reach in the population. Furthermore, this strategy was successful in recruiting a representative sample in terms of sex and age from office-based public sector organisations. High retention rates at 6 months (85% of participants) showed that our electronic method of data collection was acceptable and that the outcome measures were feasible. Although there was no significant difference in physical activity levels [ascertained by the Global Physical Activity Questionnaire (GPAQ)] between the intervention and control groups in the pilot, the study was not powered to demonstrate an effect size of Cohen’s d ≈ 0.21 (suggested by the literature)2–4 that initially informed our trial design. Finally, > 90% (n = 331) of participants were satisfied with taking part in the scheme, with 89% (n = 322) of participants stating that the physical activity loyalty (PAL) card was ‘very helpful’ in encouraging them to undertake more physical activity.
Existing research
Physical activity levels
Physical inactivity is estimated to cause 6–10% of deaths worldwide from non-communicable diseases, such as coronary heart disease, type 2 diabetes mellitus and certain cancers. 5 On a global scale, estimates have highlighted that physical inactivity costs 53.8B (INT$, 2013) worldwide in 2013. 6 The impact of increasingly inactive lifestyles is thought to cost the NHS > £1B annually. 7 Within the UK, adults are recommended to undertake at least 150 minutes per week of moderate to vigorous physical activity (e.g. cycling, brisk walking or running). 8 The current guidelines also include recommendations to reduce sedentary behaviour across the day by taking regular breaks at work and incorporating active travel. 9 Despite government guidelines highlighting the health benefits of regular physical activity,7 > 80% of adults in the UK9 and > 60% of adults in Northern Ireland10,11 are still not meeting current recommended levels.
Within the most recent National Institute for Health and Care Excellence (NICE) guidelines,12 employers are encouraged to promote physical activity in the work place by identifying workplace ‘champions’ or by implementing workplace walking and cycling programmes. As a high percentage of the adult population worldwide is in employment and most of the waking day is spent at work, workplace-based interventions are practical settings for physical activity promotion and have the potential to significantly contribute to habitual physical activity and other beneficial health behaviours. With increasing numbers of inactive office-based occupations, improvements in physical activity levels may also contribute positively to well-being, mental health and productivity. 13 It has been suggested that there is a strong business case for investment in the health and well-being of the workforce. 14 In a report published by the Department for Work and Pensions,15 it was estimated that for every £1 invested in workplace health and well-being there is a potential return of > £4 as a consequence of improvements in absenteeism and productivity, which are thought to cost employers in the region of £30B annually. 13
Workplace-based physical activity interventions
Current evidence to support the effectiveness of workplace-based physical activity interventions is mixed,16 with reviews2–4 calling for more robust research on workplace-based interventions and well-designed RCTs. Previous meta-analyses of workplace-based physical activity interventions have shown small, positive short-term effects3,4 on levels of walking but little long-term effectiveness was evident. 12 Thus there is a recognised need to develop workplace-based interventions purposefully designed to encourage physical activity behaviour change maintenance.
More recently, a systematic review and meta-analysis of 26 intervention studies published in 201617 identified three main intervention strategies to reduce sedentary time and increase physical activity within the workplace: (1) educational or behavioural change programmes (e.g. goal-setting, motivational interviewing), (2) environmental changes (e.g. sit–stand workstations) and (3) multicomponent interventions (e.g. environmental changes coupled with behaviour change techniques). The review found that workplace-based interventions, especially multicomponent interventions (implementing both an educational and an environmental aspect to the intervention), effectively reduced workplace sitting time. However, most of these studies demonstrated behaviour change in the short term, with the longest follow-up from the included studies recorded at 12 months.
Financial incentives
Financial incentives have been proposed as a method of promoting healthy lifestyles by the UK government,18–20 but evidence in this field is relatively limited to date and considered controversial by some. 21,22 Financial incentives have been shown to be effective in the short term in smoking cessation studies23 and have also proved effective in encouraging discrete health behaviours, such as attendance at vaccination programmes. 20
In a systematic review published in 201524 that examined the effectiveness of financial incentives in changing health-related behaviours in general, it was reported that behaviour change was maintained until 18 months post baseline and 3 months after incentive removal. Effectiveness measured at > 6–12 months post baseline was moderated by the participants’ socioeconomic circumstances. In a recent trial,25 rebates were offered to participants when they purchased fruit and vegetables and this led to improvements in Healthy Eating Index-2010 scores. 26 The follow-up in this trial was conducted at 4–6 months and at 9–11 months after implementation, so only relatively short-term effects have been demonstrated.
Giles et al. 27 reviewed the effectiveness of financial incentives for behaviour change (17 reports of 16 studies) in a range of health behaviours, including smoking cessation, vaccinations/screening and physical activity, and found some evidence that effect sizes decreased as post-intervention follow-up period and incentive value increased.
Relatively little research has been conducted to date with regard to the provision of financial incentives in the promotion of physical activity. A recent systematic review’s results,28 based on three studies only, suggested short-term effectiveness, with the authors, unsurprisingly, calling for more research in this area. A recent trial conducted by Shin et al. 29 sought to assess the effectiveness of combining an activity tracker with financial incentives in a group of male students; the receipt of financial rewards was contingent on participants achieving their daily physical activity goals (process incentive) and achieving their target weight (outcome incentive). The trial concluded that the addition of a financial incentive was effective in increasing physical activity levels. Christian et al. 30 also sought to explore the feasibility of using financial incentives to increase physical activity levels in a teenage population (13.3 ± 0.43 years). The intervention involved provision of £25 of vouchers (five vouchers in increments of £5) per month for 6 months. The vouchers were provided to single participants. The authors found that not only did the vouchers encourage an increase in physical activity and significant improvements in the boys’ fitness, but they also reduced levels of sedentary behaviour in both sexes and encouraged friends to socialise.
Incentives have been shown to improve participant engagement and, as the aforementioned studies demonstrated, this can lead to increased levels of physical activity. However, as Mantzari et al. 24 reported, financial incentives can increase attainment of the target levels of behaviour change from the start until the end of the incentives’ offer, but subsequently there is a monotonic trend and a weakening effect over time. With such mixed findings and of which few provide longer-term follow-up data, we cannot yet be certain that increased levels of physical activity are maintained in the longer term, the behaviour change is maintained once the incentive is removed and new habits are formed. 24,31,32
Cost-effectiveness of incentive-based interventions
To date, studies have explored the cost-effectiveness of incentives across a range of health behaviours. The cost-effectiveness of financial incentives for smoking cessation in pregnancy is encouraging. Boyd et al. 33 conducted a smoking cessation trial in which women (n = 612) were randomised to usual cessation support with or without financial incentive vouchers up to the value of £400. The findings suggested that the financial incentive for smoking cessation in this population was highly cost-effective, with an incremental cost per quality-adjusted life-year (QALY) of £482.
Paul-Ebhohimhen and Avenell34 reviewed the literature on the use of financial incentives in the treatment of overweight and obesity and found that few studies had conducted cost-effectiveness analyses. The lack of evidence on cost-effectiveness poses a distinct limitation on financial incentives’ utility for policy-making.
Cost-effectiveness of incentive-based interventions for physical activity
Although the evidence for the cost-effectiveness of incentive-based interventions is limited, studies on interventions that focus on the promotion of physical activity, particularly within the workplace, are even more sparse. 35 However, encouragingly, a cost-effectiveness analysis performed in our pilot trial showed that the PAL scheme was potentially cost-effective from both a health-care and an employer’s perspective. 1
In summary, financial incentives alone may not be sufficient to promote maintained physical activity behaviour change, but may do so when embedded within an evidence-based, theoretically driven intervention. 36 Furthermore, financial incentive interventions designed using behavioural economic concepts have been shown to be effective for changing behaviour. 37 There is also limited evidence for the cost-effectiveness of interventions promoting physical activity in the workplace36,38 and for the cost-effectiveness of financial incentive-based interventions. 31 Therefore, to address such gaps in the evidence base and following the successful completion of a pilot study,1 we aimed to conduct a cluster RCT of a complex intervention (the PAL scheme), incorporating financial incentives to encourage physical activity and maintained behaviour change.
The physical activity loyalty scheme
The PAL scheme is a multicomponent intervention based on concepts similar to those that underpin a high-street loyalty card aimed at encouraging repeated behaviour (i.e. loyalty). 39 Components include the provision of points and financial incentives contingent on the targeted behaviour being achieved (in this case, physical activity) and the provision of feedback on the targeted behaviour, as well as prompting and messaging to encourage the targeted behaviour through a tailored website. Participants can log into their account on the study website and receive real-time feedback on aspects of their physical activity, including minutes of activity, that are recorded remotely by radio frequency identification (RFID)-enabled sensors strategically placed in the neighbouring environment. Minutes are converted to points (10 points for 1 minute of activity recorded) and collected points are redeemed for rewards (i.e. retail vouchers) sponsored by, and redeemable at, local businesses. In line with the recommendations of 2012 NICE guidance,40 the study aimed to gather new evidence on effective and cost-effective workplace physical activity interventions. Although recent studies show some evidence of effect,2–4 there are three problems that the current work addresses: (1) very few studies have used objective measures of physical activity, (2) relatively little is known about the use of financial incentives for workplace physical activity and free-living activity, and (3) even less is known about their cost-effectiveness. 39 Furthermore, the PAL study addresses key knowledge gaps outlined by NICE,40 including how individual interventions interact with environmental factors in encouraging people to walk, how to make walking habitual and which factors influence longer-term behaviour change. Previous studies have used significant cash payments (up to US$750)23 that are not sustainable for the long term. Furthermore, to elicit a maintained behaviour change, the intervention should incorporate a phasing strategy to ‘shift’ the focus from extrinsic to intrinsic motivation. 39
The PAL scheme has the potential for considerable reach at little cost and the trial has the potential to inform sustainable business models using a points-based loyalty platform (i.e. local businesses ‘sponsor’ the incentive with retail vouchers in return for increased footfall to their business). This model is aligned to precepts of the Department of Health and Social Care’s public health responsibility deal. 41 Our previous formative work showed that we can recruit and retain office-based employees to this intervention and that the intervention has the potential to positively influence their physical activity levels.
Aims and objectives
The cluster RCT had the following objectives to:
-
investigate the effectiveness of the intervention to increase employee’s physical activity levels
-
investigate if any change in physical activity behaviour is maintained over time
-
conduct cost-effectiveness analyses, cost–utility analysis (CUA) and cost–benefit analysis (CBA) of the intervention
-
investigate how the intervention has an impact on other health behaviours and outcomes
-
investigate wider work-related effects, including sickness absenteeism and work presenteeism
-
investigate the mediators of (1) uptake and use of the loyalty card, (2) initiation and (3) maintenance of behaviour change
-
conduct a parallel qualitative study to further identify those who benefited from the intervention, how and why it worked for them and explore mediators of behaviour change
-
conduct a discrete choice experiment (DCE) to investigate the possible effective levels of incentives for such interventions
-
conduct a behavioural economic field experiment on intertemporal and risk preferences to investigate the relationship between physical activity behaviour change, discounting and financial incentives.
Chapter 2 Methodology
Introduction
The multicentre cluster RCT aimed to evaluate the effectiveness and cost-effectiveness of the PAL scheme to maintain physical activity behaviour change. The trial incorporated nested behavioural economic experiments and a process evaluation, and the intervention was tested in an earlier feasibility study. 1
The trial was designed to target public sector employees in predominantly office-based jobs and had two groups: an intervention group and a waiting list control group. Clusters of participants were randomly allocated to either the intervention or the control group. Clusters were defined as the smallest organisational unit (e.g. specific buildings participating in the trial).
This chapter begins by setting out the methodology for the trial in relation to sampling, outcomes and measures, data collection and analyses plans. The methodological approach undertaken for both the process evaluation and the health economic evaluation is also described. In parallel to the main health economic evaluation, Chapter 6 describes behavioural economic experiments (involving contingent valuation and DCE modelling) conducted to shed light on participants’ willingness to accept (WTA) financial incentives to improve physical activity and its relation to time preference. This chapter concludes by identifying the changes to the original published protocol.
Cluster randomised controlled trial
Study population
Recruitment of workplaces
The study targeted public sector employees involved in predominantly office-based occupations whose workplace was in Belfast or Lisburn city centres, Northern Ireland. People in predominantly office-based jobs spend a large proportion of their day physically inactive and public sector organisations have been reported to have higher sickness absence rates than private sector workplaces. 42,43 Public sector organisations were purposively sampled from those within a 2-km radius of the city centre or from those that could offer physical activity opportunities within a 2-km radius of their location and had a minimum of 100 employees in predominantly office-based occupations. Meetings were held with senior management of these organisations to explain the purpose of the study and the practicalities involved if the study was to be implemented within the organisation. Workplaces were recruited between September 2014 and August 2015 and participants were recruited between January 2015 and October 2015.
Recruitment of participants
Recruitment methods included e-mail invitations to employees and posters placed around each workplace advertising the study. E-mails and posters included the website address of the study and a web link was added to the organisations’ intranet sites (previously tested in our pilot study). 1 Potential participants were able to access further information (including the participant information sheet) and register their interest to participate on the study website. Potential participants were asked to complete a screening questionnaire via the study website or by telephone to confirm their eligibility, based on the following inclusion criteria: based at recruited worksite for at least 4 hours per day (within core hours of 08.00–18.00) on at least 3 days per week, current contract anticipated to last for the duration of the study (i.e. to exclude temporary workers), access to internet at work, able to give informed consent, able to communicate in English and no self-reported recent history of myocardial infarction or stroke or physical limitations that would limit the participant’s ability to participate in physical activity (assessed using the Physical Activity Readiness Questionnaire). All participants who met the eligibility criteria and consented to participate were contacted by a member of the research team by telephone or e-mail to complete the baseline assessment. Following this, clusters of participants were randomised to the intervention or control group using computer-generated random numbers. Clusters were defined as the smallest organisational unit (e.g. a department or office/floor) within each participating workplace.
Sample
During recruitment, a revised power calculation was performed (with the approval of NIHR) assuming a less demanding effect size than in our original protocol. This was proposed in the light of more recent literature published44 and with consideration of our actual baseline data on the mean and variance of cluster size and an intracorrelation coefficient of 0.029.
In our original protocol, the sample size calculation for the trial was determined using an anticipated effect size of Cohen’s d = 0.21 that was based on a previous meta-analysis of workplace-based physical activity interventions. However, none of the studies that was included in this meta-analysis was an incentive-based intervention for physical activity behaviour change. More recent literature has been published27,44 that includes a meta-analysis showing a mean effect size of approximately 1600 steps (d = 0.40). Additionally, the TRial of Economic Incentives to Promote Physical Activity (TRIPPA) study44 that examined the influence of financial incentives on the effectiveness of a wireless-upload pedometer to encourage weekly physical activity goals was powered to detect a difference of a minimum of 30 minutes of moderate to vigorous physical activity per week between groups and was reflective of a considerably higher effect size than assumed in our original calculation.
Therefore, assuming that our original estimate was too conservative, and after consultation with the project team, Trial Steering Committee and NIHR Public Health Research board, the power calculation was updated as follows: for an effect size of 0.40, a study of 330 participants per group (or 660 participants in total) would have 90% power at the 5% significance level. Assuming a 15% dropout, the study would therefore need to randomise 776 participants. See Deviations of the evaluation from the original protocol for further details regarding deviations of the evaluation from the original protocol.
Randomisation, concealment and blinding
Clusters were the smallest work groups or units (e.g. a large open-plan office) within each participating organisation. A random allocation sequence was drawn up by the trial statistician and group allocation was stratified to ensure a similar number of clusters in both the intervention group and the control group. Research staff were blinded to group allocation until after data collection was completed. The outcome of the randomisation was communicated to participants by e-mail after baseline measurements were complete.
Intervention group
The PAL scheme is a complex multicomponent intervention based on concepts similar to those that underpin a high-street loyalty card aimed at encouraging repeated behaviour (i.e. loyalty) and is designed to incorporate a range of behaviour change techniques. Components include the provision of ‘points’ and rewards (financial incentives) contingent on meeting targeted physical activity behaviour goals (extrinsic motivation, goal-setting). Participants were encouraged to undertake 150 minutes per week of physical activity, which is in line with current guidelines. The PAL scheme integrated a novel physical activity remote tracking system with web-based monitoring and evidence-based behaviour change tools (i.e. self-monitoring, goal-setting). We carefully considered the design of this complex intervention in line with Medical Research Council’s guidelines. 45 We have followed advice from the MRC framework in our work, including theoretical work, development of the logic model, mapping of the intervention onto determinants and mapping of measures onto hypothesised mechanisms, pilot/feasibility study including qualitative work.
The 6-month intervention involved placing sensors (WiFi beacons) in the vicinity of participating workplaces at specific locations to encourage physical activity within a 2-km radius of participants’ worksites (i.e. including the provision of prompts/cues to facilitate habit formation). The WiFi beacons were placed at locations along footpaths, in local parks, leisure centres, shopping malls, bus stops and train stations. Maps of various walking routes and details about physical activity opportunities tailored to the workplace (and instructions on how to perform behaviour) were provided on the study website. Participant’s activity was logged when they passed within an approximate 25-m radius of the WiFi sensors with their PAL key fob when undertaking physical activity (e.g. walking). This logged the place, date and time of the bout of physical activity. Participants could log onto their account on the study website and receive real-time feedback on the number of minutes of physical activity logged by the tracking system. Minutes were converted to ‘points’ (10 ‘points’ for 1 minute of activity recorded), and collected ‘points’ were redeemable for rewards (i.e. downloadable retail vouchers) sponsored by, and redeemable at, local businesses. To reduce the risk of ‘gaming’, a daily ‘points’ cap was implemented and the transit times between sensors checked for anomalous values. Bonus rewards and ‘Double Points Days’ were offered when participants met specific weekly physical activity targets.
To tailor the intervention, a purposive sample of employees (both men and women across a range of ages) participated in three focus groups (maximum 10 participants per group) prior to the intervention to explore aspects such as the availability of, and preferences for, opportunities for physical activity in proximity to the workplace. Sensor locations were determined from the feedback received in the focus groups regarding popular walking routes. Employees’ opinions on the website interface and the rewards redemption function, for example, were also considered and this permitted the final tailoring of its functionality. To determine incentive levels prior to the intervention, stated preferences of the participants from the DCEs were used to assess their mean WTA, willingness to pay (WTP) and the trade-offs they would make for the attributes of the incentive programme. This information helped determine the level of the rewards available for earned ‘points’. In addition to the financial incentive element, the intervention had several other components designed to enhance the effectiveness of the incentives. These components were delivered via the study website and designed to have multiple effects: (1) to increase use of the study website, (2) as effective behaviour change techniques (BCTs) in their own right and (3) as techniques designed to aid the transition from more extrinsically motivated behaviour to more intrinsically motivated habitual behaviour. The techniques included the provision of regular tailored motivational e-mails, tailored feedback, information on walking routes in the vicinity of the participating workplaces and links to other resources, such as physical activity advice and healthy eating guidelines. They also included self-regulation techniques of goal-setting, self-monitoring and prompts to behaviour.
Underpinning theoretical framework
The financial reward component of the intervention was based on principles of learning theory46 by providing an immediate reward (extrinsic motivation) for behaviours that offer health gains in the future. The financial reward component of the intervention also contained elements based on other approaches, such as goal-setting, prompts, self-monitoring and habit formation that fit within a self-regulation control theory framework, motivational messages (persuasion) and social support (vicarious experience), all of which should increase self-efficacy according to social cognitive theory. 47 Social cognitive theory also holds that satisfaction with the consequences of behaviour change can act as a reinforcing mechanism, in addition to the reinforcement of financial rewards. 47 Therefore, the financial incentive component was embedded in a complex intervention containing evidence-informed BCTs. Figure 1 presents the logic model underpinning the development of the intervention.
FIGURE 1.
Logic model of the PAL scheme. Reproduced with permission from Hunter et al. 39,48 This article is distributed under the terms of the Creative Commons Attribution 4.0 International License (http://creativecommons.org/licenses/by/4.0/), which permits unrestricted use, distribution, and reproduction in any medium, provided you give appropriate credit to the original author(s) and the source, provide a link to the Creative Commons license, and indicate if changes were made. The Creative Commons Public Domain Dedication waiver (http://creativecommons.org/publicdomain/zero/1.0/) applies to the data made available in this article, unless otherwise stated. CVD, cardiovascular disease.

Control group
Participants assigned to the waiting list control group (n = 388) were offered the opportunity to participate in the intervention after the 12-month follow-up period. Participants in this group completed outcome measures at the same time points as the intervention group. The waiting list control group received the intervention following the 12-month data collection period.
Outcomes and measures
Outcome measures are grouped into primary and secondary outcomes as displayed in Table 1. In summary, the primary outcome was physical activity behaviour change (mean number of steps per day) measured using a sealed pedometer. Participants were asked to wear the pedometer for 7 consecutive days on the waistband (dominant hip). They were asked to complete a wear time diary providing details on the dates and times that they removed and wore the monitor. Participants were advised to remove the monitor when showering, bathing or undertaking any water-based activities. Pedometer data were considered valid if the participant provided ≥ 250 steps per day for ≥ 3 days at each data collection period. 70 Pedometers were sealed at all times to blind participants to the output and prevent reactivity, which is standard practice. This device was used as a measurement tool only (i.e. the pedometer was not part of the intervention) and a standardised measurement protocol was used for the intervention and control groups. We followed a standard protocol that has been well validated71–75 and successfully employed as a measurement tool in numerous intervention studies.
Outcomes | Measures |
---|---|
Primary outcomes | |
Physical activity | |
Mean number of steps per day, objectively measured by a sealed pedometer (to blind participants to the output) worn on the waistband (Yamax Digiwalker CW-701; Yamax, Tasley, UK) |
Participants were asked to wear the pedometer for 7 consecutive days and to complete a wear time diary. Pedometer data were considered valid if the participant provided ≥ 250 steps per day for ≥ 3 days at each data collection period Participants were also asked to complete the GPAQ to elucidate the context of the physical activity undertaken. 49 These measures were collected at baseline, at 6 months (immediately post intervention) and at 12 months (6 months post intervention). This schedule allowed the trial researchers to account for the seasonality of physical activity behaviours |
Secondary outcomes | |
Health and well-being | |
General health, mental health, quality of life and mental well-being |
The following validated self-report measures were completed at baseline and at 6 months via Qualtrics SF-850 items from the SF-8 questionnaire can be derived to give an indication of both physical and mental health EQ-5D-5L51 is a measure of quality of life and is used to derive the health state utility measure based on five dimensions of mobility, self-care, usual activities, pain/discomfort and anxiety/depression (on a scale of 0–100), and the weighted health index WEMWBS is derived from 14 statements (with higher scores indicating better mental health). 52,53 The WEMWBS comprises 14 positively worded statements, where scores are summed with higher scores indicating greater mental well-being |
Work-related impacts | |
Absenteeism and presenteeism |
The WHO’s HPQ:54 work absenteeism was measured by asking participants to state the number of days of sick leave they took in the past 6 months (collected at baseline and at 6 months) Specific questions from the WHO’s HPQ54 were used to measure work presenteeism. This validated method comprises three questions with answers provided on an 11-point Likert scale and asks participants to rate their job performance levels |
Mediators and moderators | |
Proposed mediators and moderators of physical activity behaviour change |
Common core theoretical constructs of physical activity behaviour change, including outcome expectancy,55 social norms,16 self-efficacy,56 financial motivation,57 planning,58 identified regulation,59,60 integrated regulation,59,60 intrinsic motivation59,60 and intention61 were collected at baseline and at 4 weeks to assess initiation of behaviour change Perceptions of workplace environment (measured at baseline only):62 these included perceptions of workplace environment (i.e. attractiveness, safety, accessibility, availability). Perceptions of workplace attractiveness were assessed as the sum of four items (e.g. ‘in my workplace environment it is pleasant to walk’, score range 1–5). Perceptions of workplace safety were assessed as the sum of four items (e.g. ‘in my workplace environment the roads are dangerous for cyclists’, score range 1–5). Perceptions of workplace accessibility were assessed as the sum of three items (e.g. ‘in my workplace environment there is convenient public transport’, score range 1–5). Perceptions of workplace availability were assessed as the sum of three items (e.g. ‘in my workplace environment there is a park within walking distance’, score range 1–5) Objective measures of the workplace environment using GIS data, including walkability,63 street connectivity and access to physical activity opportunities (e.g. shops, parks, leisure facilities, train/bus stations), were measured at baseline. 64 The walkability index was computed using four constructs, namely: Individual-level cognitive constructs measured to assess the maintenance of physical activity behaviour change were collected at baseline and at 6 months and included planning,58 self-determined motivation (i.e. identified regulation, integrated regulation and intrinsic motivation),59,60 habit,17 recovery and maintenance self-efficacy,20 and outcome satisfaction55,65 Measures of social norms16 and workplace norms16 were also collected at baseline and at 6 months Other measures included web engagement, confidence in using the internet and loyalty card use Potential moderators were collected at baseline. These included perceptions of workplace environment (i.e. attractiveness, safety, accessibility, availability),62 age, sex, highest educational level, income, marital status, BMI, SF-8 Mental and Physical Component Scores,50 EQ-5D Health State and Weighted Health Index,51 WEMWBS,52,53 WTA [assessed by asking the participants two open-ended questions requiring the respondents to give a minimum amount in GBP necessary for compliance with an additional 30 minutes (WTA30) or 60 minutes (WTA60) of outdoor physical activity] and time discount rates [Q1: Imagine that you are offered either £1000 today or £1005 tomorrow. Which one would you choose? Q2: Imagine that you are offered either £1000 today or £1050 tomorrow. Which one would you choose? Coded 1 (low discount rate) if answer to Q1 is ‘£1005 tomorrow’ and answer to Q2 is ‘£1050 tomorrow’, coded 2 (moderate discount rate) if answer to Q1 is ‘£1000 today’ and the answer to Q2 is ‘£1050 tomorrow’, and coded 3 (high discount rate) if answer to Q1 and Q2 is ‘£1000 today’] |
Compensatory behaviours | |
Smoking, alcohol consumption and diet |
Short FFQ was collected at baseline and at 6 months66,67 Self-reported smoking and alcohol consumption behaviours were collected at baseline and at 6 months |
Health economic evaluation | |
Changes in HRQoL (as expressed using QALYs using EQ-5D data) were measured from the participant’s perspective. The EQ-5D is a validated measure and has been used extensively for cost-effectiveness analyses Utilisation of health-care resources was captured using a specially devised online health and social care resource use data collection form These measures were completed at baseline and at 6 months. Intervention costs were obtained using a modified template,68 explicitly discriminating between intervention and research costs. These include website development, software (e.g. licence fee), hardware (e.g. sensors, loyalty cards) and intervention running costs (e.g. maintenance of sensors), the costs of negotiating on incentives from local businesses and the delivery of vouchers |
|
Process evaluation | |
Informed by the logic model developed from the feasibility study and guided by the evaluation planning framework for public health interventions and research.69 The process evaluation employed a triangulated design using both quantitative and qualitative data |
Secondary outcomes included measures of health and well-being, work-related impacts, proposed mediators of behaviour change, compensatory behaviours, a range of variables for use in the economic evaluation and a range of variables for use in the process evaluation.
All outcome measures were analysed and, when required, collected by a postdoctoral research fellow blinded to group allocation. Self-reported outcome measures were collected at baseline and at 6 months (unless otherwise stated) via online questionnaires distributed by e-mail and automatically collated via Qualtrics.
Data collection
Statistical analysis (primary and secondary outcomes)
Primary and secondary outcomes at 6 and 12 months (when applicable) were compared between the intervention and control groups using analyses of covariance (ANCOVAs) adjusting for baseline values, randomisation stratum (i.e. large ≥ 50 employees, medium 20–49 employees, small < 20 employees or schools/colleges) and season (1 = December 2015–April 2016, 2 = July–August 2016) with standard errors (SEs) corrected for clustering. Owing to large seasonal effects, results are presented before and after adjusting for season.
As specified in our published protocol,39 the relationship between group assignment and the 6-month mean number of steps per day (measured using pedometers) was examined for moderating effects with ANCOVAs, adjusting for baseline pedometer number of steps per day, randomisation stratum and season, with SEs and p-values corrected for clustering. These analyses were then repeated to examine the relationship between group assignment and 12–month mean number of steps per day for moderating effects.
The moderators and moderator-by-group interactions were included as predictor variables with mean-centring for continuous moderators and the creation of dummy variables for dichotomous moderators. Moderators examined included baseline sociodemographic variables [i.e. age, sex, body mass index (BMI), income, education level, marital status] health measures [i.e. Short Form Questionnaire-8 items (SF-8) mental and physical component scores, EuroQol-5 Dimensions (EQ-5D) health state and weighted health index and the Warwick–Edinburgh Mental Wellbeing Scale (WEMWBS)], perceptions of the workplace environment (i.e. attractiveness, safety, accessibility, availability), WTA and time discount rates.
The level of significance was a p-value of < 0.05 for all analyses. Analyses were carried out using Stata®, version 13 (StataCorp LP, College Station, TX, USA).
Sensitivity analysis
For a preliminary assessment of possible non-response bias, analysis of the primary outcome (i.e. 6-month pedometer steps per day, 12-month pedometer steps per day) was repeated after imputation of missing data using multiple imputation by chained equations (MICE) with 20 imputations. The MICE procedure operates on the assumption that data are missing at random. 76 Baseline variables were inspected for potential predictors of missingness of the 6-month outcomes and predictors of the outcome itself. Baseline ‘physical activity self-efficacy’,56 ‘intrinsic motivation’,59 ‘habit’,17 ‘recovery self-efficacy’20 and ‘maintenance self-efficacy’20 scores in addition to marital status (0 = married/cohabiting, 1 = other) significantly predicted missingness of the 6-month outcome. Baseline ‘physical activity self-efficacy’,56 ‘intentions’,61 ‘habit’,17 ‘outcome expectations’,55 ‘identified regulation’,59,60 ‘integrated regulation’,59,60 ‘intrinsic motivation’,59,60 ‘financial motivation’,57 ’outcome satisfaction’,55,65 ‘planning’,58 ‘social norms’,16 group assignment (0 = control group, 1 = intervention group), BMI, income (0 = ≤ £20,000, 1 = > £20,000) and season (1 = December 2015–April 2016, 2 = July–August 2016) significantly predicted the 6-month and 12-month outcome (i.e. mean number of pedometer steps per day). These variables were used in the imputation model to make predictions about the values of missing data. We then repeated the primary outcome analyses using imputed values and pedometer steps per day at 6 and 12 months were compared between the intervention and control groups using ANCOVAs adjusting for baseline values, randomisation stratum and season with SEs corrected for clustering. Owing to large seasonal effects, results are presented before and after adjusting for season. These analyses were carried out using the ‘mi estimate’ command in Stata. Rubin’s rules were used to obtain the final estimates from the estimates derived in the 20 imputed data sets. 77
Ethics and consent
Ethics approval was sought from and granted by the Office of Research Ethics Committees Northern Ireland (ORECNI) prior to the start of the study (reference number 14/NI/0090). Fully informed consent was obtained from all participants prior to their inclusion in the study. Participants were asked to confirm that they had read and understood the information sheet prior to agreeing to participate. Participants were also given an opportunity to ask any questions and ensure that these were answered satisfactorily prior to completing the consent form. Research governance approval was granted by the South Eastern Health and Social Care Trust and Belfast Health and Social Care Trust.
Qualitative process evaluation
Methods
Participants’ experiences of being involved in the PAL scheme, including barriers to and facilitators of engagement with the intervention, were explored using focus groups (lasting approximately 1 hour) at 6 months (n = 9 focus groups) and 12 months (n = 6 focus groups). Purposive sampling was undertaken to ensure a representative sample of participants within each focus group (a maximum of 10 participants per group). A schedule of open-ended questions was employed to elicit information about reactions to the intervention, barriers to physical activity and suggestions for a future roll-out of the intervention if proven effective. At 12 months, additional focus groups were also undertaken with 17 of the same individuals who participated in the 6-month focus groups (n = 6 focus groups). On this occasion, the open-ended questions focused on physical activity behaviour change maintenance. Semistructured interviews were also undertaken with senior managers of six participating employers (n = 7 managers) and participating retailers (n = 4 retailers) to explore their perceptions of being involved in the study. Discussions were facilitated by AG, Doctor of Philosophy (PhD) (female), and analysis of the data was undertaken by LP, PhD (male), both of whom have prior focus group and qualitative methodology experience. The researchers did not have a relationship established with participants prior to study commencement.
Qualitative analysis
Focus groups and semistructured interviews were audiorecorded and transcribed verbatim. Transcripts from focus groups and semistructured interviews with retailers and managers were analysed using thematic analysis. 78 The thematic analysis followed six key steps, including (1) familiarisation with the data, (2) systematic coding, (3) grouping of codes to form potential themes, (4) reviewing themes and (5) formally naming and (6) defining themes. 78 All transcripts were independently coded by two members of the research team (AG and LP). Meaningful quotes from participants were extracted to highlight typical responses.
Health economics
Methods
Resource use
Resource use data were collected from trial participants via an online questionnaire at baseline and 6 months. Service use included visits to a general practice (GP), a nurse, a physiotherapist and accident and emergency (A&E) attendance, outpatient appointments, inpatient stays, medications use and other services. Unit costs in the financial year 2015–16 published by the Personal Social Service Research Unit (PSSRU)79 and NHS Reference Costs80 (when information was not available from PSSRU) were attached to each item of resource use.
Table 2 shows the unit costs applied to each resource use item. Costs of service use between the groups were compared using four methods: (1) complete case only, not adjusting for covariates; (2) complete case only, adjusting for covariates; (3) using imputed data sets, not adjusting for covariates; and (4) using imputed data sets and adjusting for covariates. Standard errors and 95% confidence intervals (CIs) for estimates for all models were adjusted for cluster effects.
Resource use item (unit used in the source) | Unit cost (£) | Source |
---|---|---|
NHS resource use | ||
GP (per contact) | 36.00 | Unit Costs of Health and Social Care 2016,79 p. 145. Per-patient contact lasting 9.22 minutes, with qualifications |
Practice nurse (per hour) | 43.00 (11.11 per contact) |
Unit Costs of Health and Social Care 2016,79 p. 143. Nursing average cost per hour, with qualifications Duration of contact per patient is 15.5 minutes (Unit Costs of Health and Social Care 2015,81 p. 174, based on the 2006/7 UK general practice survey57) |
NHS physiotherapist (per contact) | 49.00 | NHS Reference Costs 2015–16.80 AHP, physiotherapist, adult, one to one (A08A1). National average unit cost |
A&E attendance (per attendance) | 185.00 | NHS Reference Costs 2015–16.80 Average of ‘see and treat and convey’ (by ambulance, £236), ‘see and treat or refer’ (emergency care only, £181) and A&E attendance by own transport (£138) (p. 18). Table 8: costs by currency for ambulance services between 2013–14 and 2015–16 (p. 18). Table 2: unit costs by point of delivery, 2013–14 to 2015–16 (p. 10)82 |
Outpatient appointment (per attendance) | 117.00 | NHS Reference Costs 2015–16,80 p. 10. Table 2: unit costs by point of delivery, 2013–14 to 2015–16. Unit cost per outpatient attendance |
Inpatient stays (per night) | 373.00 | NHS Reference Costs 2015–16, main schedule.80 Average of costs per elective and non-elective inpatient excess bed-days across all currency codes. Elective inpatient excess bed-days average across all currency codes at £395. Non-elective inpatient excess bed-days average across all currency codes at £351 |
Intervention cost
The cost of implementing the PAL scheme was collected from the study management team and equipment development team. Only the participants allocated to the intervention group were assumed to incur the cost of the intervention and any research-related costs were stripped from the intervention cost. This ensured the relevance of the cost estimates if the programme was to be ‘rolled out’ to larger numbers of participants. Equipment costs were annualised to the 6-month trial period by spreading the costs over their anticipated lifespan.
Absenteeism and presenteeism
The effect of physical activity on absolute absenteeism was used to generate a proxy financial impact on employers. The World Health Organization (WHO) Health and Work Performance Questionnaire (HPQ)54 was used to capture the number of hours absent from work, this questionnaire was completed by participants at baseline and 6 months. Impacts on employee absenteeism were measured using question four of the questionnaire [‘About how many hours altogether did you work in the past 4 weeks (28 days)?’] and question two (‘How many hours does your employer expect you to work in a typical 7-day week?’), using the formula:
Individual hourly salary values in the financial year 2016 were attached to the number of hours absent from work. The hourly salaries were obtained from the 2016 NHS pay scales, with the lowest grade at band 1 (£7.8 per hour, equivalent to £15,251 annually), mid-grade at band 8A (£22.86 per hour, equivalent to £44,703 annually) and highest grade at band 9 (£50.85 per hour, equivalent to £99,437) taken separately to reflect the range of potential cost savings for employees at various salary grades. 83
Utility and quality-adjusted life-years
Effectiveness in the CUA was expressed as QALYs. QALYs were estimated using the utility index values generated from the EuroQol-5 Dimensions, five-level version (EQ-5D-5L) questionnaire collected from participants at baseline and the 6-month follow-up. EQ-5D is a commonly used standardised generic preference-based quality-of-life measure addressing five domains: mobility, self-care, usual activities, pain/discomfort and anxiety/depression. 84 The five-level version allows the participants to select the level of problems from five levels: no problems, slight problems, moderate problems, severe problems and extreme problems/unable to do. The five-level version was chosen, rather than the three-level version, for its potentially improved sensitivity and decreased ceiling effect (i.e. there is a high proportion, typically > 15%, of respondents reporting the best possible health who are, therefore, unable to record any improvement in health status). 85,86 This makes EQ-5D-5L the preferred measure, especially in this population. The employees are drawn from the general population without any specified conditions and, therefore, it is common to see ‘no problem’ being answered for all dimensions, which is what creates a ceiling effect. EQ-5D-5L responses from the participants were converted to utility scores by mapping the five-level descriptive system data on the three-level valuation set using the mapping algorithm developed by van Hout et al. ,87 as recommended by the NICE guideline and its position statement published in August 2013. 88 The area under the curve method was then used to estimate the QALY score over a 12-month period, following the trapezium rule assuming a linear change in utility between each assessment time point. 89
Missing data and multiple imputation
Data were missing if participants did not complete the online follow-up assessment or returned an incomplete response. Multiple imputation was conducted for utility values and total costs at aggregate level (compared with individual dimension and resource use item) to circumvent convergence issues with the imputation model when it contained many variables. To maximise the use of the completed resource use data, missingness of any single item on the resource use questionnaire was assumed to indicate that no resource was used for that item during the assessment period. This assumption has been used in a previous NIHR Health Technology Assessment (HTA) report. 90 The aggregate-level missing data were imputed using MICE. When the cost data had a heavily zero-inflated right-skewed distribution, on account of this being a generally healthy population, the predictive mean matching method on log-transformed costs was employed as recommended. 91 A constant (£1 in this case) is added to the raw cost data to avoid problems when log-transforming zero values. After imputation, the cost data were transformed back to the original scale for estimation. 91 Intervention and control groups were imputed separately. In addition to cost (on log-scale) and utility at baseline and 6 months, the imputation model also contained several baseline characteristics that either were shown to be statistically significantly related to cost and utility or were primary variables in the analysis, in line with the primary analysis of effectiveness. The baseline characteristics were mean number of steps per day, age, sex, SF-8 physical and mental scores, cluster, randomisation stratum (categorical variables coded as large, schools/colleges, medium or small) and season (categorical variables indicating 6-month follow-up data collection time, December 2015–April 2016 or July–August 2016 as periods for data collection). Fifty imputed data sets were generated and then used to estimate the difference in QALYs and costs, and the incremental cost-effectiveness ratio (ICER).
Statistical analysis
Cost–utility analysis
In the CUA, differences in cost and QALYs between the two groups were estimated using generalised linear models (GLM), which take into account the typically skewed nature of cost and QALY data. When histogram evidence identified cost data as zero-inflated and right-skewed, a gamma distribution with a log-link was recommended. When QALY data were in a distribution with a left-skewed tail bounded by 0.5 QALYs (maximum QALYs accrued for a 6-month period), decrements of QALYs were predicted in the GLM regression with a gamma and log-link. The method of predicting decrements of QALYs was applied in a previous NIHR HTA report,92 with decrements calculated as the difference between the maximum QALYs that could possibly be accrued within the time horizon of the analysis and the actual QALYs gained. This ensures that the QALY variable is right-skewed and left-bounded by 0, fitting into a gamma distribution. Covariates in the GLM model were selected based on statistical significance and using regressions to predict QALYs and costs with complete cases. As recommended in the literature,93 the chosen covariates included in the model were the same as in the imputation model, as mentioned in Missing data and multiple imputation. Baseline utility scores and baseline costs were also included to adjust for any imbalance between groups. 94
Mean costs and QALYs for each group were estimated using the method of recycled predictions to adjust for covariates as well as to the use of log-link in the GLM. The ICER was estimated from the difference in costs and QALYs from the GLM regression. A 1000-iteration bootstrapping procedure was conducted to investigate the uncertainty surrounding the ICER estimate and the probability that the intervention was cost-effective under a wide range of hypothetical thresholds (£0–100,000). Standard errors for differences in costs and QALYs were estimated through the bootstrap, adjusting for cluster effects. These results were visualised in the cost-effectiveness plane and cost-effectiveness acceptability curves (CEACs).
Sensitivity analyses
A number of sensitivity analyses were undertaken to assess the robustness of the base-case results of the CUA to alternative assumptions. These sensitivity analyses are summarised in Table 3.
Scenario | Element | Position in base-case analysis | Variation for the sensitivity analysis |
---|---|---|---|
1 | Intervention: voucher cost | Voucher cost £21,000 | Three business models are tested:
|
2 | Least expensive scenario | Voucher cost £21,000
|
|
3 | Missing data | Missing data are assumed to be missing at random and a mixed strategy of imputation was conducted | Complete-case analysis: missing data assumed to be missing completely at random |
The cost of the intervention could easily vary with the development of appropriate technology, hence differing deals with local manufacturers and retailers are explored in the sensitivity analysis. Costs for retail vouchers can be reduced when identifying different deals with the retailers and, thus, assumptions were made varying the cost of the vouchers. Three business models were tested by the trial group.
Deal 1
An external company (i.e. Club Marketing Ltd) was used to negotiate deals with retailers. The negotiation fee was estimated to be £100 per retailer. There were 23 retailers in total, so the total voucher cost was estimated to be reduced down to £2300. This led to a much smaller intervention cost of £14.77 per participant over the 6-month period.
Deal 2
A ‘shops for all’ voucher was paid at a £10 rate for every 400 points. The total number of points earned by the participants was 425,201, leading to a total voucher cost of £10,630.25. The resulting intervention cost was £32.99 per participant over the 6-month period.
Deal 3
The third option was a ‘sustainable business model’ that was employed in Dallat et al. ’s study. 95 A marketing consultant was hired to act as an intermediary with the local business sector and was able to negotiate the provision of these vouchers ‘in kind’ from local retailers. The cost was calculated using the hourly salary and the number of hours the consultant worked to negotiate the deals. Dallat et al. ’s95 study reported a cost of £4077 for hiring the consultant and this estimate was doubled (£8154) as the PAL scheme doubled the number of business partners. Again, this option led to a smaller intervention cost than the base-case analysis, which cost £27.58 per participant.
Costs of sensors and key fobs could also be reduced by assuming an extended lifespan with upgraded design facilitating or enabling battery replacement; thereby, costs with upgraded devices were used in the sensitivity analysis. In addition, a complete-case analysis was conducted assuming that data were missing completely at random to assess the impact that imputation strategies had on the incremental costs and QALYs.
Cost–benefit analysis: a net cost model
In addition to the CUA, a CBA was conducted from the employer’s perspective. This enabled the broader impact of the intervention beyond the health-related quality of life to be considered by applying values for differences in absenteeism and presenteeism rates. The CBA employed a ‘net cost model’ to obtain a comprehensive estimate of intervention costs from an employer’s perspective by subtracting the avoided cost of absenteeism from the direct intervention cost incurred from employers. 96 This provides an estimate of the potential economic benefit of the PAL scheme from the employer’s perspective. The uncertainty of the potential economic benefit of the PAL scheme was estimated using bootstrapping methods (1000 iterations).
Behavioural economics
Methods
Systematic reviews suggest that financial incentive interventions have become increasingly popular in promoting health behaviour change. 97 Although the idea of using financial incentives to encourage healthier behaviours seems acceptable to the public in the UK context,98 the levels of incentives utilised in previous studies (i.e. the monetary amount thought necessary to trigger behaviour change) were not based on prior research and, consequently, led to uncertainty about the effectiveness and cost-effectiveness of financial incentives as an intervention tool. To investigate the optimal levels of financial incentives required to encourage changes in physical activity, all participants in our study were sent an online questionnaire at baseline to assess the average level of incentives that participants would be WTA to increase their physical activity overall as well as for increasing different types of physical activity. The values obtained in the preliminary contingent valuation analysis were later used to fix the level of the rewards available for earned ‘points’.
Although in its infancy, behavioural economics is helping our understanding of the relationship between behaviour change, time discounting and incentives, and is providing new insights that have sparked the interest of public health researchers and policy-makers. 99 The principle behind financial incentives is to capitalise on the notion of time preference (i.e. the tendency for an individual to place greater value on present benefits relative to future benefits). Time preference is typically elicited through a binary question that asks an individual to choose between an immediate, smaller reward and a delayed, larger reward. Individuals who choose the smaller, immediate reward are said to have a high time preference and, thus, it is hypothesised that they are likely to act on the present immediate benefits of a behaviour (unhealthy) at the expense of the perceived future benefits (of healthy behaviours). Systematic reviews have shown extensively that time preference plays an important role in shaping some unhealthy behaviours (e.g. smoking and obesity). 100,101
Two other key concepts possibly related to behaviour change are risk preference and loss aversion. The former refers to the participants’ willingness to take risks whereas the latter refers to the tendency of participants to prefer avoidance of losses to winning equivalent gains. A recent advance in the methodology of eliciting time preferences is the joint elicitation of risk and time preferences. Andersen et al. 102 have shown that when discount rates and risk preferences are elicited and analysed together the discount rates are significantly reduced and are more accurately defined. Major behavioural economic theories have suggested that people are more sensitive to losses than to gains. For instance, prospect theory has demonstrated that the utility foregone from a unit monetary loss is twice the utility gain from a unit monetary gain. 103 How loss aversion is related to unhealthy behaviours is rarely investigated. As a result, all participants remaining in our study were invited at the 6-month follow-up to participate in an incentive-compatible behavioural economic field experiment that was scheduled at their workplaces to elicit their personal behavioural economic traits (i.e. time discounting, risk preference and loss aversion).
Apart from the baseline contingent valuation survey that was necessary to inform plausible levels of incentives, the subsequent economic experiments were conducted on smaller subsamples of the population during follow-up (to keep the participant burden and costs to a minimum). This was planned as ancillary to the main trial analysis, with the primary purpose of shedding light on the possible behavioural economic moderators supplementary to the main mediation–moderation analysis conducted for the trial as a whole.
Contingent valuation
Double-bounded dichotomous choice (DBDC),104 which is a contingent valuation method frequently used in the valuation of non-market goods, was used at baseline to measure participants’ WTA financial incentives for increasing their physical activity. Through an online questionnaire, participants were asked to answer two dichotomous hypothetical questions. In the first question, participants were asked ‘Would you be willing to increase your total amount of physical activity by 30 minutes per week if you were paid £0.50 per week?’. A ‘no’ or ‘don’t know’ answer to the first question indicated that the participant considered the £0.50 financial incentive too low. The follow-up question then applied a similar format but with a doubled bid level (i.e. £1.00). Likewise, a ‘yes’ response to the first question indicated that the £0.50 incentive sufficed so that the proposed amount in the second question was halved to £0.25. We considered three treatments using a between-subject design. First, to investigate whether or not the minimum monetary incentives required by participants varied across the range of proposed physical activity increments. We considered two separate scenarios, an increase of 30 or 60 minutes of general physical activity per week. Second, to avoid order effects, participants were randomised into two groups; one group was first presented with the 30-minute physical activity scenario and then the 60-minute physical activity scenario, and the other group was presented first with the 60-minute physical activity scenario and then the 30-minute physical activity scenario. Third, each participant was presented with a starting bid level randomly chosen from four levels (i.e. £0.10, £0.50, £1.50 and £5.00) for the 30-minute physical activity scenario and a starting bid level randomly chosen (from £0.15, £1.00, £3.00 and £10.00) for the 60-minute physical activity scenario. Because what was considered a reasonable bid set was unknown, we provisionally suggested some initial bid sets that were pretested among a pilot group of 98 participants. An updated questionnaire was then sent to the rest of the study participants. Participants who failed to complete the questionnaire were excluded, leading to a sample size of 663 participants in the final analysis.
Discrete choice modelling
Double-bounded dichotomous choice provides no information about participants’ WTA financial incentives for increasing different types of physical activity (i.e. walking or cycling to and from places, or moderate-intensity and vigorous-intensity recreational physical activity). Alternatively, a DCE approach can be applied to provide a more direct route to the valuation of different characteristics or attributes of physical activity and of marginal changes in these characteristics rather than general physical activity as a whole. The levels for the four attributes are: walking or cycling to and from places (0, 30, 60 and 90 minutes per week), moderate-intensity recreational physical activity (0, 30, 60 and 90 minutes per week), vigorous-intensity recreational physical activity (0, 30 and 60 minutes per week) and monetary rewards (£0.50, £1.00, £3.00, £5.00 and £10.00 per week). Each choice set had two alternative physical activity scenarios with different combinations of attributes and levels and one opt-out option. Combining all attributes and levels results in 240 combinations (4 × 4 × 3 × 5 = 240), making it infeasible to present participants with all possible choice sets. Instead, 24 choice sets were generated following a Bayesian-efficient design based on the minimisation of the D-error criterion to reduce participant fatigue while being sufficient to estimate the main effects of attributes. 105 This was accomplished by using the N-Gene software (version 1.1.2; Choice Metrics Pty Ltd, Sydney, NSW, Australia). We then divided the 24 choice sets into four blocks of six choice sets and each participant received one randomised block of questions. A web-based DCE questionnaire was disseminated to participants at baseline. All participants who completed the baseline physical activity measurement by wearing a pedometer for 7 consecutive days were categorised as active (≥ 10,000 steps per day), moderate-active (≥ 5000 and < 10,000 steps per day) and inactive (< 5000 steps per day). The participants who did not complete the DCE questionnaire were excluded from the analysis, leading to a final sample size of 673 included in the analysis.
Behavioural economics experiments
Behavioural economics concepts are useful for understanding the psychological roots of healthy and unhealthy behaviours. We focus on three behavioural economics concepts: time preference (i.e. the extent to which future benefits are discounted), risk preference (i.e. the willingness to take risks with greater or lesser positive outcomes) and loss aversion (i.e. the inclination to avoid losses). These behavioural economic experiments were proposed as an adjunct to the main study analysis to yield further insights into possible moderators and mediators of intervention effects. As such, they point to avenues that might be fruitful for further public health research.
Measurement of time preference
To elicit the time preference of participants, an economic experiment methodology first employed by Coller and Williams106 and further developed by Andersen et al. 102 was utilised. This methodology presents participants with multiple price lists that offer a choice between two amounts paid for real: option A and option B. Option A pays a smaller amount of money after a delay (e.g. £250 in a week), whereas option B offers a larger amount of money after a further delay (e.g. £300 in 25 weeks). The larger payment represents the sooner amount plus the interest from ‘saving’ the option A payment within the experiment for the duration of the delay. Each multiple price list consisted of 10 choices between option A and option B, the option A amount and the delay in receiving the option B amount remained constant and the interest rate increased as one progressed down through the sequential choices. Two ‘teatments’ were included in this design in order to assess the structural form of the time-discounting behaviour of participants. A front-end delay treatment for which option A is either offered immediately or after a short delay (time horizon treatment) allows a test for quasi-hyperbolic discounting. 107 The basic advantage of quasi-hyperbolic discounting over the typically modelled single parameter functional forms (i.e. Mazur’s hyperbolic discounting or exponential discounting) is that it is more flexible and incorporates two parameters, each of which represents the two elements of time preference: (1) a conventional discount rate that refers to the extent to which future rewards are discounted and (2) present bias that means that the relative discount rate between two proximal delays is higher than the relative discount rate between two distal dates. There were five front-end delays (i.e. 1 day, 3 days, 1 week, 2 weeks and 4 weeks) and three time horizons (i.e. 4 weeks, 12 weeks and 24 weeks), giving it a 5 x 3 design. Only one front-end delay and two time horizons were randomly drawn, leading to four choice tasks for each participant.
Measurement of risk preference
Participants were presented with a single multiple price list that consisted of ten decisions between two lotteries: option A or option B. Each option consists of a chance to receive a large or a small amount of money. Option A is a ‘safe’ option that pays a certain and small amount (e.g. £60), whereas option B is the ‘risky’ option and involves the risk to win a substantially higher amount (e.g. £95) but also has the risk of getting a much lower amount (e.g. £50). Each of the participants was presented with two such choice tasks.
Measurement of loss aversion
The multiple price list format, consisting of 10 decisions between two lotteries (option A or option B), was also used to measure loss aversion. Option A pays nothing (e.g. £0), whereas option B gives the participant the chance to win more (e.g. £22) but also has the chance of losing money (e.g. £11). Each of the participants was presented with two such choice tasks. The maximum possible loss from the task was £20; this was no more than the £20 show-up fee that participants received so that they would always get non-negative payments.
Payment mechanism
Participants received two forms of payment. First, all participants were credited with £20 as a thank you for participating in the substudy, where this £20 then functioned as an endowment to use in the loss aversion tasks. Second, real, rather than hypothetical, money was at stake in the behavioural economic experiments to ensure that participants made considerations that were incentivised and focused on the choice tasks at hand. Participants had a 10% chance (implemented by rolling a 10-sided die) of receiving payment based on the random choice of one of their decisions in both the discounting task and the risk preference task. In the loss aversion tasks, participants had a chance to gain more money (or lose money) on top of the £20 that had been credited to them earlier. The balance after the loss aversion task was then paid in cash at the end of the session.
Participants
All participants remaining in the study at the 6-month follow-up received an e-mail invitation (including a copy of the participant information sheet) detailing the expected time needed to complete the tasks. A total of 21 sessions were organised during lunchtime in meeting rooms at the participating organisations. A total of 153 participants from the intervention group and 54 participants from the control group took part in this substudy.
Statistical analysis
The statistical package Stata was used to carry out all analyses. For the contingent valuation, a conditional log-likelihood function for the set of DBDC responses was constructed and a maximum-likelihood estimation was used to predict the mean WTA. In the DCE analysis, the responses to a choice set were taken as a specific observation. A generalised multinomial logit model108 was used that assumed a specification that nests both scale heterogeneity and parameter heterogeneity. The participants who chose the opt-out options for all choice sets, indicating that they did not believe in the proposed hypothetical system of rewards, were excluded from the analysis. Interaction terms were used to explore whether or not preferences for attributes varied by the participants’ physical inactivity status at baseline. Next, we estimated a double-hurdle model109 that assumed that the decision to use the physical activity monitoring system and how long to use it for were independent decisions so that the determinants of the two decisions are allowed to differ. The estimation was accomplished by using the Stata module ‘craggit’. Missing data were excluded from the analysis. Furthermore, another double-hurdle model was estimated to investigate the determinants for the decision to increase physical activity and the amount by which physical activity had increased at the 6-month follow-up compared with the baseline (i.e. the mean number of steps per day at the 6-month follow-up was larger than the number of baseline mean steps per day), respectively. For the analysis of the behavioural economic experiments, we simultaneously elicited present bias, the discount rate, risk preference and loss aversion in an integrated framework using a hierarchical Bayesian methodology. 110 More specifically, a higher discount rate implies a higher level of impatience. And a present-bias parameter of significantly smaller than one implies a decreasing impatience over time (i.e. a participant has long-term goals that could benefit them in the future but no present actions will be taken to achieve these goals because of self-control problems). The risk aversion and loss aversion parameters take a value bigger than 0 and a higher value implies a higher degree of risk aversion and loss aversion, respectively. The estimated behavioural economic parameters were later entered into a double-hurdle model to investigate their influences on the decision to increase physical activity and the amount by which physical activity had increased at the 12-month follow-up compared with the 6-month follow-up, respectively.
Mediation analyses
Methods
Constructs hypothesised to mediate the initiation of physical activity behaviour were collected at baseline and at 4 weeks and included outcome expectations,55 physical activity self-efficacy,56 intention,61 planning,58 financial motivation,57 self-determined motivation (i.e. identified regulation, integrated regulation and intrinsic motivation)59,60 and social norms. 16
Constructs hypothesised to mediate the maintenance of physical activity behaviour were collected at baseline and at 6 months and included planning,58 self-determined motivation (i.e. identified regulation, integrated regulation and intrinsic motivation),59,60 habit,17 recovery and maintenance self-efficacy,20 outcome satisfaction,55,65 social norms16 and workplace norms. 16
Engagement and non-use attrition analyses
In addition to the mediation analysis, factors associated with participant engagement and non-use attrition were explored for participants in the intervention group. ‘Engagement’ refers to the level of exposure to an intervention and the amount of skills practice involved (i.e. completing activities or exercises to acquire knowledge or learn behaviour relevant to the target outcome). 111 A participant’s level of engagement determines the extent to which they receive the intended intervention and research on engagement is useful for identifying which intervention components are associated with health outcomes. 112,113 Non-use attrition refers to the phenomenon of participants ceasing intervention use, which is particularly observed in the literature to occur in web-based interventions. 114 It is important to understand participants’ non-usage patterns and the contributing factors, and to make recommendations for retaining all participants in future intervention studies because it is difficult to measure intervention effect if participants have not been exposed to the intervention.
Three markers of overall intervention engagement (i.e. daily physical activity captured via the PAL scheme physical activity monitoring system, use of the PAL website and reward redemption) were tracked throughout the 6-month intervention period and the following variables were derived:
-
The percentage of intervention days during which participants walked for at least 10 minutes115 captured via the PAL scheme physical activity monitoring system over the 6-month intervention period.
-
The percentage of intervention weeks during which participants logged onto the PAL scheme website at least once over the 6-month intervention period. 116
-
The percentage of earned points redeemed over the 6-month intervention period. Aside from earning points by recording activity via the physical activity monitoring system, this indicator captured whether or not participants were interested in redeeming their earned points for financial rewards to incentivise their physical activity behaviour.
Engagement with the different aspects of the PAL scheme website was assessed by the frequency of ‘hits’ on each intervention component for every 10 days the participant accessed the website and by the total number of intervention components accessed on the website at least once (0–6 intervention components). The six intervention components that participants could access on the website were as follows:
-
Monitoring and feedback – data and visual representation (i.e. graphics) of the participant’s physical activity over the intervention period for self-monitoring purposes (i.e. self-monitoring and feedback, goal-setting).
-
Rewards – a platform for participants to view their earned and bonus points, information on available rewards and points redemption (i.e. immediate reward contingent on behaviour change).
-
Maps – maps of sensor locations and example walking routes for the planning of physical activity (i.e. information on when/where to perform physical activity, action planning).
-
Health information (physical activity behaviour only) – physical activity facts and information, health benefits, safety tips and tips for a physically active lifestyle (i.e. provision of information about the health benefits of physical activity).
-
Health information (other behaviours) – information related to healthy eating, smoking, alcohol consumption and stress reduction (i.e. provision of information about health benefits of other health behaviours).
-
Discussion forums –a platform for participants to contact researchers and other participants to ask questions, make enquiries, raise concerns, respond to comments and provide social support.
Non-use attrition (assessed separately in relation to the use of the physical activity monitoring system to record activity and website use) was considered to occur if a participant had at least a 2-week lapse from use. 117,118 Non-use attrition for recording activity via the physical activity monitoring system was measured as the number of days until the first 2-week lapse from recording activity. Website non-use attrition was measured as the number of days until the first 2-week lapse from logging onto the website.
Statistical analysis
All questionnaire items were scaled so that lower values indicated lower levels of the mediator/outcome. The mediation analyses are exploratory and should be interpreted with caution because of issues associated with multiple testing and because the study was powered for the primary physical activity outcome and not to detect change in mediators. The level of significance was a p-value of < 0.05 for all analyses. Analyses were carried out using Stata.
Initiation and maintenance of physical activity behaviour
Mediators of the initiation of physical activity behaviour (assessed at 4 weeks; i.e. outcome expectancies, physical activity self-efficacy, intention, planning, financial motivation, identified regulation, integrated regulation, intrinsic motivation and social norms) and mediators of the maintenance of physical activity behaviour (assessed at 6 months; i.e. planning, identified regulation, integrated regulation, intrinsic motivation, habit, recovery and maintenance self-efficacy, outcome satisfaction, social norms and work norms) were compared between intervention and control groups using random-effects regressions adjusting for baseline values of the mediator, randomisation stratum, season and baseline pedometer steps per day with SEs and p-values adjusted for cluster effects. The visual inspection of outcome distributions showed that some outcomes did not conform well to the normal distribution. Therefore, as a sensitivity analysis, these analyses were repeated using ordered-logistic regressions with mediators categorised arbitrarily into three or four categories.
Single mediator models
Single mediator models were built to explore the possible mechanisms of behaviour change in the PAL scheme. Single mediator models were run for all mediators of initiation and maintenance individually based on the structural equation modelling (SEM) based product-of-coefficients approach. 119 In each model, the independent variable was group assignment, the mediating variable was the follow-up (i.e. 4 weeks or 6 months) score of the mediator and the dependent variable was the 6-month physical activity outcome. All analyses were adjusted for randomisation stratum, season, baseline values of the mediator and baseline physical activity, with SEs and p-values corrected for clustering. The significance of indirect effects was determined by 95% CIs estimated using the bias-corrected bootstrap (with 10,000 iterations) procedure recommended by MacKinnon et al. 120 and MacKinnon. 121 The maximum-likelihood method of estimation was used. Model fit was assessed with reference to the coefficient of determination and the standardised root mean square residual (SRMR), adjusting SEs and p-values for clustering in Stata. A cut-off value of close to 0.08 for SRMR was required to consider the model a relatively good fit to the data. 122
Single moderated mediation models
For mediators showing significant indirect effects in single mediator models and moderators showing significant interaction effects, single moderated mediation models were run using the moderated product-of-coefficients approach. Research suggests that mediators and moderators should be investigated as they operate together, both to provide a fuller understanding of mechanisms of behaviour change in intervention studies and to promote the advancement of theory. 123,124 Moderation of the a-path was tested by adding the moderator and moderator-by-group interaction as independent variables in the relevant single mediator models. A significant coefficient for the interaction term indicated that moderation of the a-path was present (representing an ‘intervention challenge’). 124 Moderation of the b-path was tested by adding the moderator and moderator-by-mediator interaction as independent variables in relevant single mediator models. A significant coefficient for the interaction term indicated that moderation of the b-path was present (representing a ‘theoretical challenge’). 124
Engagement and non-use attrition
For the intervention group only, random-effects generalised least squares (GLS) regressions were run with 6-month physical activity (i.e. mean number of steps per day) or 6-month mediators as the dependent variables, and engagement variables (i.e. the percentage of intervention days during which participants undertook at least 10 minutes of physical activity captured using the PAL scheme physical activity monitoring system, the percentage of intervention weeks that participants logged onto the PAL scheme website, the percentage of earned points redeemed, the frequency of ‘hits’ on each of the six intervention components that could be accessed on the website for every 10 days the participant accessed the website, the total number of website sections accessed at least once and the total number of minutes spent on the website) as the independent variables. For models that included 6-month physical activity as the dependent variable, strata, season and baseline mean number of steps per day were included as covariates with SEs and p-values adjusted for clustering. For models that included 6-month mediators as the dependent variable, strata, season, baseline mean number of steps per day and baseline values of the mediator were included as covariates, with SEs and p-values adjusted for clustering. This determined whether levels of 6-month physical activity or any of the 6-month mediators were significantly explained by levels of engagement in various aspects of the intervention when controlling for baseline levels of physical activity and mediators, group assignment, randomisation stratum, season and cluster. Because changes in the mediators of initiation (measured at baseline and 4 weeks) were not related to changes in the mean number of steps per day at 6 months (see Chapter 7, Single mediator models), they were not subjected to testing as part of the engagement analysis. Engagement variables showing a significant relationship with 6-month physical activity in univariable analyses (p < 0.05) were included in a multivariable model to determine their combined effects on 6-month physical activity.
Estimated median lifetime use (i.e. time after which 50% of participants stopped use) was calculated separately for use of the physical activity monitoring system and use of the PAL scheme website. Baseline measures of sociodemographic variables, psychosocial variables, environmental variables and physical activity were investigated as predictors of non-use attrition of the physical activity monitoring system to record daily activity and non-use attrition of the PAL website using Cox proportional hazards regression analyses. In the first analysis, the time variable was the number of days until the first 2-week lapse from using the physical activity monitoring system to record daily activity and the event variable was coded 1 if non-use attrition occurred or 0 if non-use attrition did not occur. In the second analysis, the time variable was the number of days until the first 2-week lapse from logging onto the website and the event variable was coded 1 if non-use attrition occurred or 0 if non-use attrition did not occur. Univariate analyses were conducted on all predictor variables and those with a p-value of < 0.25125 were included in a multivariable model with backwards elimination of the predictor with the highest p-value until all included predictors had a p-value of < 0.05. All analyses included SEs and p-values corrected for clustering and Efron’s procedure was used for handling ties as it is advocated over Breslow’s method and can be implemented with models adjusting SEs and p-values for clustering. 126
Deviations of the evaluation from the original protocol
Deviations and rationale
On 31 August 2015, the research team corresponded with the NIHR Public Health Research secretariat to indicate that the initial recruitment target was not met, explaining a variety of reasons why the original target proved to be problematic. The significant restructuring of a number of the public sector organisations that were to host the intervention had a severe impact on participant recruitment and the study team undertook various actions to mitigate the shortfall (e.g. by extending the number of sites and taking on advice from patient and public involvement members on our Trial Steering Committee regarding alternative recruitment methods within the host organisations).
Because recruitment numbers were lower than anticipated from our initial baseline data collection phase in Lisburn (from the restructured local authority and health-care trust), the intervention phase started later than scheduled, in May 2014. It was decided that recruitment would continue across other agreed sites in Belfast [Queen’s University Belfast (offering a pool of approximately 3000 staff) and the Stormont Civil Service Estate (offering a pool of approximately 3000 staff)] to attempt to meet the recruitment target and that the intervention would be implemented on a rolling basis thereafter. Therefore, randomisation and the intervention phases were implemented in July and August 2015 in the Queen’s University Belfast campus and Stormont Civil Service Estate, respectively.
Recruitment continued to be slower than anticipated, even with the addition of our new sites at Queen’s University Belfast and the Stormont Civil Service Estate. This was a result of unforeseen circumstances (austerity-related restructuring of local authorities and restructuring within the civil service) and, therefore, we sought approval to recruit from two final sites within the Belfast Health and Social Care Trust (Royal Victoria Hospital and Belfast City Hospital). Subsequently, we undertook an intense recruitment period in early September 2015. Participant recruitment was completed in January 2016 following the addition of a new worksite (n = 853 participants in total).
Professor Chris Patterson (study statistician) made a revised power calculation that took account of more recent literature on effect size estimates and the baseline data on cluster size, cluster variation and intraclass correlation coefficient. As reported from the previous Trial Steering Committees, the revised sample size requirement demonstrated a minimal impact on power (see Chapter 2, Sample, for further details).
Furthermore, to maximise trial retention, all participants received a £10 gift card at the 6- and 12-month stage for the full completion of study outcomes (funded from internal sources). The trial was originally designed to include the follow-up of participants up to 18 months post intervention. However, with unavoidable delays in initial recruitment attendant on the restructuring of the local authorities and in the light of the findings at the 6-month follow-up, it was agreed with NIHR that follow-up should stop at 12 months rather than at 18 months as was originally planned.
Stakeholder engagement
Stakeholder engagement in this evaluation has been a key element throughout this study. The purpose of such engagement has been to:
-
inform aspects of the study design
-
raise awareness of the research in the workplace and its potential benefits
-
be actively involved in the interpretation of the trial findings and the process evaluation
-
help identify the practical significance of the findings from the trial and the implications for further delivery of the PAL scheme or similar schemes
-
help plan a dissemination strategy.
Such engagement has taken four forms: project team meetings, stakeholder members of the Trial Steering Commitee, dissemination events and process evaluation.
Project team meetings
From the outset of the trial, the research team has attended and fully engaged in the project team meetings with staff from the health trusts and organisations participating in the trial. This forum has maintained a schedule of twice-yearly meetings throughout the lifespan of the trial. The aim has been to help influence the research at an early stage of development to raise awareness of it and support the organisations’ involvement in the process.
Stakeholder members of the Trial Steering Committee
Alongside the project team meetings, key stakeholder (comprising staff from the health trusts and participating organisations) also contributed directly to the overall study evaluation as members of the Trial Steering Committee. Critically, this included contributing to the emerging interpretation of the findings and the development of the dissemination strategy.
Dissemination events
Alongside the dissemination of the key findings of this study at academic conferences, a number of non-academic dissemination activities were planned. Such activities have included the distribution of two annual study ezines to all host organisations, study participants and to the UK Clinical Research Collaboration Centre of Excellence (Northern Ireland) partners. Dr Aisling Gough (key co-ordinating postdoctoral research fellow for the study) attended a Northern Ireland Public Health Research Network workshop to update the network on the PAL scheme (February 2017). The offer of a health and well-being workshop and a PAL study debriefing was extended to the ‘champions’ or key contacts at each of the participating worksites. The research team also received interest from a number of organisations that expressed an interest in adopting the study’s bespoke web platform for promoting physical activity in the workplace, and the study team are currently in talks with the Health and Safety Executive of the South Eastern Health and Social Care Trust and Sport Northern Ireland regarding this. Talks have also been held with the Queen’s University Belfast Research and Enterprise team regarding the licensing of the web platform for such purposes. Furthermore, plans have been made to archive the results of dissemination activities on the British Science Association’s Collective Memory database (see http://collectivememory.britishscienceassociation.org/).
Process evaluation
The aim of the process evaluation was to examine (1) what the participants’ exposure was to the intervention; (2) the extent to which the intervention was implemented across the participating organisations; (3) how, for whom and under what circumstances the intervention could bring about behaviour change; (4) how, for whom and under what circumstances the intervention might maintain behaviour change; and (5) whether or not there were any unintended consequences of the intervention.
As described earlier in this chapter, the process evaluation involved in-depth engagement with all of the key stakeholders to ascertain their experiences of and perspectives on the programme. The qualitative insights gained from the participants and also from the employers are demonstrated in Chapter 4, Senior managers of participating employees.
Chapter 3 Results from the trial
Recruitment
A total of 1209 employees expressed an interest in participating in the study and were assessed for eligibility. Reasons for exclusion included the following:
-
the employee was not based at the recruited worksite for at least 4 hours per day on at least 3 days per week (n = 93)
-
the employee’s contract did not last the duration of the study (n = 1)
-
the employee no longer wished to participate pre randomisation (n = 107)
-
the employee did not provide baseline data (n = 150)
-
the researchers were unable to contact the employee (n = 5).
In total, 853 individuals were randomised to either the intervention group (n = 457) or the control group (n = 396). The study flow diagram is presented in Figure 2.
FIGURE 2.
The CONSORT flow diagram. Reproduced with permission from Hunter et al. 48 This article is distributed under the terms of the Creative Commons Attribution 4.0 International License (http://creativecommons.org/licenses/by/4.0/), which permits unrestricted use, distribution, and reproduction in any medium, provided you give appropriate credit to the original author(s) and the source, provide a link to the Creative Commons license, and indicate if changes were made. The Creative Commons Public Domain Dedication waiver (http://creativecommons.org/publicdomain/zero/1.0/) applies to the data made available in this article, unless otherwise stated.
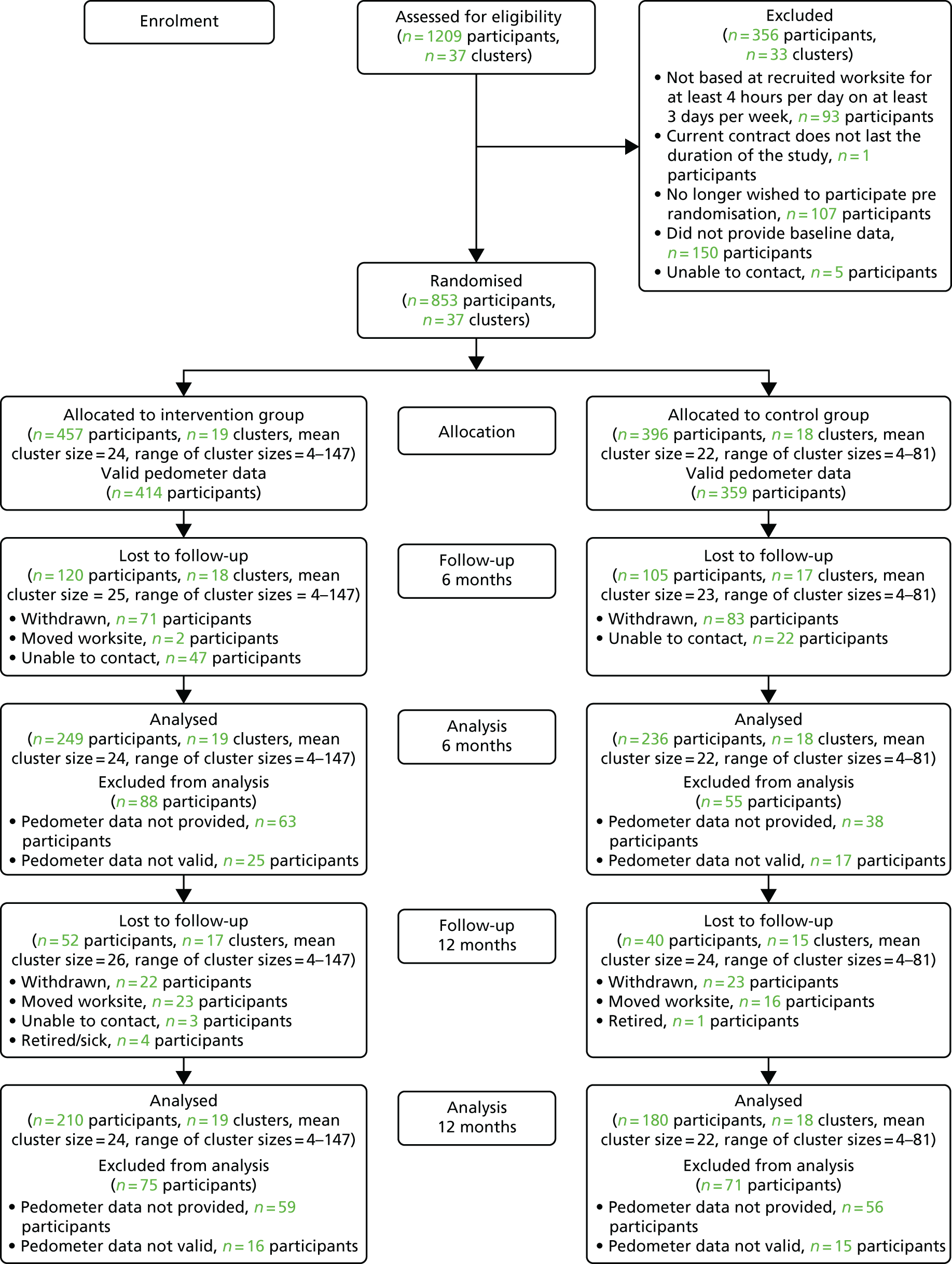
Withdrawals
Participants noted as withdrawals were those who formally, via e-mail, by telephone or in person, expressed their wish to no longer take part in the trial. At 6 months post baseline, 154 participants (n = 71 participants from the intervention group) had withdrawn from the study. At 12 months post baseline, a further 45 participants had withdrawn (n = 22 participants from the intervention group). The stated reasons for withdrawals included lack of time and/or interest.
Lost to follow-up
Participants noted as ‘lost to follow-up’ were those who were unable to be contacted as a result of moving worksite location or those who did not reply to e-mail/telephone contact by the research team. At 6 months post baseline, 71 participants were lost to follow-up (n = 49 participants from the intervention group). Across the two groups, 69 participants were unable to be contacted and two participants had moved location. At 12 months post baseline, a further 47 participants (n = 30 participants from the intervention group) were lost to follow-up, of whom five had retired or were off sick, 39 had moved location and a further three were unable to be contacted by the research team.
Harms and adverse effects
There were no harms or adverse effects reported by any participant throughout the conduct of the trial.
Sample characteristics
A total of 853 participants from 37 clusters were recruited and randomised into two groups (intervention group, n = 457, control group, n = 396). Table 4 shows baseline characteristics of the clusters and participants stratified by group. The mean age of participants was 43.6 years [standard deviation (SD) 9.6 years] and 71% of the participants were female. At baseline, the mean number of steps per day were 7826 steps (SD 3425), measured over 7 consecutive days. According to the self-report GPAQ, 29% of participants were categorised as having ‘low’ levels of physical activity.
Characteristics | Group | |
---|---|---|
Intervention | Control | |
Characteristics of clusters | N = 19 | N = 18 |
Number of participants, mean (range) | 24 (4 to 147) | 22 (4 to 81) |
Randomisation stratum, clusters (n, % of participants) | ||
Small (< 20 employees) | 11 (114, 25%) | 11 (105, 27%) |
Medium (20–49 employees) | 5 (167, 37%) | 4 (123, 31%) |
Large (≥ 50 employees) | 1 (147, 32%) | 2 (144, 36%) |
Schools | 2 (29, 6%) | 1 (24, 6%) |
Characteristics of participants | N = 457 | N = 396 |
Age (years), n (%) | 44.0 (9.3) | 43.0 (10.0) |
Female, n (%) | 329 (72) | 278 (70) |
BMI (kg/m2), n (%) | 27.2 (5.6) | 26.6 (5.3) |
Income > £20,000, n (%) | 341 (75) | 291 (73) |
Education: some higher level, n (%) | 295 (65) | 270 (68) |
Marital status: married/cohabiting; n (%) | 313 (68) | 274 (69) |
Objective physical activity: pedometer steps (steps per day) | 7977 (3602) | 7650 (3204) |
Objective: physical activity category, n (%) | ||
High (> 7500 steps per day) | 204 (45) | 167 (42) |
Moderate (> 2500 to ≤ 7500 steps per day) | 199 (44) | 184 (46) |
Low (< 2500 steps per day) | 11 (2) | 8 (2) |
GPAQ: minutes of work physical activity (minutes per week) | 42 (138) | 58 (151) |
GPAQ: minutes of MVPA (minutes per week) | 296 (342) | 344 (333) |
GPAQ: physical activity category, n (%) | ||
High | 70 (15) | 76 (19) |
Moderate | 140 (31) | 130 (33) |
Low | 141 (31) | 104 (26) |
SF-8: mental component score | 48.0 (8.9) | 47.7 (9.3) |
SF-8: physical component score | 52.5 (6.6) | 52.7 (7.0) |
EQ-5D: health state | 82.4 (13.8) | 83.8 (14.3) |
EQ-5D: weighted health index | 0.89 (0.11) | 0.89 (0.12) |
WEMWBS: mental well-being scale | 50.2 (8.2) | 50.3 (8.9) |
Physical activity self-efficacy scale | 2.91 (0.97) | 2.92 (0.94) |
HPQ: 4-week absolute absenteeism | 5.04 (41.3) | 3.48 (50.0) |
HPQ: absolute presenteeism | 80.3 (13.6) | 81.0 (13.4) |
HPQ: combined relative absenteeism and absolute presenteeism | 8.84 (12.57) | 8.56 (7.32) |
Findings at the end of the intervention (6 months post baseline)
Primary outcomes
At 6 months post baseline, the mean number of steps per day were 6990 (SD 3078) steps for the intervention group and 7576 (SD 3345) steps for the control group. There was a significant difference between the intervention and control groups in the mean number of steps per day (b = –336 steps, 95% CI –612 to –60 steps; p = 0.02) after adjustment for baseline values, randomisation stratum and season, and correction for cluster effects (Table 5). Possible reasons for this result are explored in Sensitivity analysis.
Outcome | Group | ANCOVAb | ANCOVAc | |||||
---|---|---|---|---|---|---|---|---|
Interventiona | Controla | |||||||
N | Mean (SD) | N | Mean (SD) | b (95% CI) | p-value | b (95% CI) | p-value | |
Primary outcome | ||||||||
Objective physical activity: pedometer steps (steps per day) | 249 | 6990 (3078) | 236 | 7576 (3345) | –519 (–931 to –107) | 0.01 | –336 (–612 to –60) | 0.02 |
Secondary outcomes | ||||||||
GPAQ: minutes of work physical activity (minutes per week) | 253 | 16.32 (57.55) | 235 | 43.72 (129.33) | –33.67 (–65.32 to –2.02) | 0.04 | –33.34 (–65.44 to –1.24) | 0.04 |
GPAQ: minutes of MVPA (minutes per week) | 231 | 291.84 (255.76) | 221 | 350.14 (329.41) | –2.75 (–63.36 to 57.85) | 0.93 | 4.12 (–47.07 to 55.31) | 0.88 |
SF-8: mental component score | 269 | 48.43 (8.90) | 244 | 47.12 (9.44) | 1.07 (–0.27 to 2.42) | 0.12 | 1.17 (–0.23 to 2.56) | 0.10 |
SF-8: physical component score | 269 | 50.87 (8.37) | 244 | 50.99 (7.64) | 0.43 (–1.26 to 2.12) | 0.62 | 0.64 (–0.79 to 2.08) | 0.38 |
EQ-5D: health state | 262 | 77.95 (16.16) | 239 | 78.26 (16.08) | 0.67 (–2.35 to 3.70) | 0.66 | 0.70 (–2.45 to 3.86) | 0.66 |
EQ-5D: weighted health index | 262 | 0.83 (0.14) | 239 | 0.84 (0.15) | 0.005 (–0.02 to 0.03) | 0.71 | 0.01 (–0.01 to 0.04) | 0.37 |
WEMWBS: mental well-being scale | 266 | 50.34 (8.38) | 243 | 49.42 (8.24) | 1.15 (0.18 to 2.12) | 0.02 | 1.34 (0.48 to 2.20) | < 0.01 |
HPQ: 4-week absolute absenteeism | 247 | 4.04 (66.11) | 227 | 7.01 (51.40) | –2.25 (–12.49 to 8.00) | 0.67 | –2.59 (–12.79 to 7.62) | 0.62 |
HPQ: absolute presenteeism | 261 | 78.62 (14.58) | 236 | 78.47 (14.21) | 0.82 (–1.79 to 3.44) | 0.54 | 1.48 (–0.43 to 3.38) | 0.13 |
HPQ: combined relative absenteeism and absolute presenteeism | 246 | 9.52 (24.63) | 226 | 7.91 (4.94) | –0.03 (–0.91 to 0.85) | 0.95 | –0.02 (–0.92 to 0.87) | 0.96 |
At 6 months post baseline, there was a significant difference between the intervention group compared with the control group for minutes per week of self-reported work-related physical activity (b = –33.3 steps, 95% CI –65.44 to –1.24 steps; p = 0.04). There was no significant difference between the groups for minutes per week of self-reported moderate- to vigorous-intensity physical activity (b = 4.12 steps, 95% CI –47.07 to 55.31 steps; p = 0.88).
Sensitivity analyses
At 6 months post baseline, the mean number of steps per day in the imputed data set were 6914 steps (SD 3142 steps, n = 457 participants) for the intervention group and 7408 steps (SD 3253 steps, n = 369 participants) for the control group. There was a significant difference in the number of steps in the intervention group compared with the control group (b = –526 steps, 95% CI –948 to –104 steps; p = 0.02) after adjustment for baseline values, randomisation stratum and season, and correction for cluster effects (Table 6).
Outcome | Group | ANCOVAb | ANCOVAc | |||||
---|---|---|---|---|---|---|---|---|
Interventiona | Controla | |||||||
N | Mean (SD) | N | Mean (SD) | b (95% CI) | p-value | b (95% CI) | p-value | |
6-month objective physical activity: pedometer steps (number of steps per day) | 457 | 6914 (3142) | 369 | 7408 (3253) | –574 (–1109 to –41) | 0.04 | –526 (–948 to –104) | 0.02 |
12-month objective physical activity: pedometer steps (number of steps per day) | 457 | 7522 (3489) | 369 | 7739 (3340) | –543 (–1207 to 120) | 0.11 | –558 (–1203 to 87) | 0.09 |
Because the intervention had marginally but significantly lowered ‘steps per day’ (in the intervention group), we abductively explored possible reasons for this. In one recruitment site there were some periodic technical malfunctions affecting the sensors in the environment (which recorded participants’ physical activity). These malfunctions were routinely detected by the network and the sensor was replaced usually within 24–48 hours. However, some intervention group participants may have been temporarily demotivated when they knew that their activity was not being monitored for these short periods. Therefore, the main analysis was repeated excluding the affected clusters (n = 11). The magnitude of the estimated difference in the mean number of steps per day between the intervention and control groups was reduced and the difference was non-significant (b = –172 steps, 95% CI –472 to 128 steps; p = 0.26) after adjustment for baseline values, randomisation stratum and season, and correction for cluster effects.
Exploratory subgroup analyses
Moderation analyses in Table 7 showed that perceptions of the availability of physical activity opportunities in the workplace environment moderated the relationship between group assignment and 6-month mean number of steps per day, as indicated by a significant coefficient for the interaction term (b = –280 steps; 95% CI –538 to –23 steps; p = 0.03). This indicated that the negative effect of assignment to the intervention group on 6-month mean number of steps per day was greater at higher levels of workplace environment availability. Therefore, there was a greater decrease in the mean number of steps per day (between the baseline and the 6-month follow-up) for the intervention group participants than control group participants when perceptions of the availability of physical activity opportunities in the workplace environment were higher. It should be noted that, owing to issues with multiple testing, this result should be interpreted with caution. The results of all other moderation analyses were non-significant.
Moderator | Moderator-by-group interaction | |||||
---|---|---|---|---|---|---|
6-month mean number of steps per day | 12-month mean number of steps per day | |||||
N | b (SE) | p-value | N | b (SE) | p-value | |
Age (years) | 454 | 10.7 (21.0) | 0.61 | 366 | –10.5 (31.8) | 0.74 |
Sex (male)a | 456 | –310.0 (330.6) | 0.35 | 368 | 709.1 (684.7) | 0.30 |
BMI (kg/m2) | 442 | 56.4 (39.1) | 0.15 | 358 | 61.3 (46.5) | 0.19 |
Income (> £20,000)a | 449 | –92.7 (591.8) | 0.88 | 363 | –25.7 (748.5) | 0.97 |
Education (higher level)a | 449 | 374.1 (384.7) | 0.33 | 363 | 272.7 (534.2) | 0.61 |
Marital status (married/cohabiting)a | 448 | –181.6 (454.4) | 0.69 | 362 | –183.0 (758.2) | 0.81 |
SF-8 | ||||||
Mental component score | 449 | 6.48 (29.0) | 0.82 | 363 | –1.61 (44.3) | 0.97 |
Physical component score | 449 | 9.12 (26.5) | 0.73 | 363 | –49.9 (38.5) | 0.20 |
EQ-5D | ||||||
Health state | 426 | –5.17 (14.7) | 0.72 | 347 | 9.95 (22.0) | 0.65 |
Weighted health index | 427 | 1444 (2045) | 0.48 | 347 | –1352 (2829) | 0.63 |
WEMWBS: mental well-being scale | 449 | –7.88 (32.0) | 0.81 | 363 | 30.3 (42.6) | 0.48 |
Workplace environment | ||||||
Attractiveness | 448 | –73.8 (51.7) | 0.15 | 363 | –50.5 (115.9) | 0.66 |
Safety | 448 | –76.3 (85.6) | 0.37 | 363 | 294.2 (122.2) | 0.02 |
Accessibility | 448 | –173.1 (102.0) | 0.09 | 363 | 57.8 (176.5) | 0.74 |
Availability | 448 | –280.3 (131.3) | 0.03 | 363 | –49.3 (194.1) | 0.80 |
WTA30 | 405 | –2.91 (39.7) | 0.94 | 329 | 25.0 (52.5) | 0.63 |
WTA60 | 401 | –5.48 (47.8) | 0.91 | 325 | –7.44 (57.4) | 0.90 |
Time discount 2 (2 vs. 1)a | 419 | 162.7 (384.0) | 0.67 | 340 | 889.9 (670.2) | 0.18 |
Time discount 3 (3 vs. 1)a | 419 | –630.0 (385.1) | 0.10 | 340 | –1216 (515.5) | 0.02 |
Findings from 6-month follow-up (12 months post baseline)
Primary outcomes at 6-month follow-up (12 months post baseline)
At 12 months, the mean number of steps per day were 7790 (SD 3462) steps for the intervention group and 8203 (SD 3,401) steps for the control group. There was a non-significant difference between the intervention and control groups in the number of steps per day (b = –570 steps, 95% CI –1267 to 127 steps; p = 0.11) after adjustment for baseline values, randomisation stratum and season, and correction for cluster effects (Table 8).
Outcome | Group | ANCOVAb | ANCOVAc | |||||
---|---|---|---|---|---|---|---|---|
Interventiona | Controla | |||||||
N | Mean (SD) | N | Mean (SD) | b (95% CI) | p-value | b (95% CI) | p-value | |
Primary outcome | ||||||||
Objective physical activity: pedometer steps (number of steps per day) | 210 | 7790 (3462) | 180 | 8203 (3401) | –561 (–1243 to 120) | 0.11 | –570 (–1267 to 127) | 0.11 |
Secondary outcomes | ||||||||
GPAQ: minutes of work physical activity (minutes per week) | 207 | 43.2 (146.6) | 182 | 44.7 (121.7) | 1.77 (–22.3 to 25.8) | 0.89 | 7.00 (–12.6 to 26.6) | 0.48 |
GPAQ: minutes of MVPA (minutes per week) | 188 | 387.4 (385.9) | 169 | 354.4 (314.7) | 75.2 (–8.29 to 158.7) | 0.08 | 77.0 (–7.94 to 162.0) | 0.08 |
At 12 months, there were no significant differences between the intervention and control groups for minutes per week of self-reported work-related physical activity (b = 7.00, 95% CI –12.55 to 26.56 minutes; p = 0.48) and for minutes per week of self-reported moderate- to vigorous-intensity physical activity (b = 77.04, 95% CI –7.94 to 162.0 minutes; p = 0.08) (see Table 8).
Sensitivity analyses
At 12 months, mean number of steps per day in the imputed data set were 7522 steps (SD 3489, in n = 457 participants) for the intervention group and 7739 steps (SD 3340, in n = 369 participants) for the control group. There was no significant difference between the intervention group and the control group (b = –558, 95% CI –1203 to 87; p = 0.09) after adjustment for baseline values, randomisation stratum and season, and correction for cluster effects (see Table 6).
Exploratory subgroup analyses
Moderation analyses (see Table 7) showed that perceptions of the safety of the workplace environment moderated the relationship between group assignment and the 12-month mean number of steps per day, as indicated by a significant coefficient for the interaction term (b = 294.2 steps, 95% CI 55 to 534 steps; p = 0.02). This indicated that the negative effect of assignment to the intervention group on the 12-month mean number of steps per day was greater at lower levels of workplace environment safety. Therefore, there was a greater decrease in the mean number of steps per day (between the baseline and the 12-month follow-up) for intervention group participants compared with control group participants when perceptions of the safety of the workplace environment were lower. Moderation analyses also showed that the ‘Time discount 3’ variable (high discount rate vs. low discount rate) moderated the relationship between group assignment and the 12-month mean number of steps per day, as indicated by a significant coefficient for the interaction term (b = –1216, 95% CI –2226 to –205; p = 0.02). This indicated that the negative effect of assignment to the intervention group on the 12-month mean number of steps per day was greater when discount rates were higher. Therefore, there was a greater decrease in the mean number of steps per day (between the baseline and the 12-month follow-up) for intervention group participants than with control group participants when participants’ discount rates were higher (i.e. participants prefer an immediate reward with a lower monetary value rather than having to wait to get a reward with a slightly higher monetary value tomorrow). Owing to issues with multiple testing, these results should be interpreted with caution. The results of all other moderation analyses were non-significant.
Secondary outcomes
There was a significant difference between groups for the WEMWBS score (b = 1.34, 95% CI 0.48 to 2.20; p < 0.01), with well-being scores higher in the intervention group participants than in the control group participants. There were no significant differences between groups for SF-8 mental (b = 1.17, 95% CI –0.23 to 2.56; p = 0.10) and physical (b = 0.64, 95% CI –0.79 to 2.08; p = 0.38) component scores. There were no significant differences between groups for the EQ-5D-5L health state (b = 0.70, 95% CI –2.45 to 3.86; p = 0.66) and weighted health index scores (b = 0.01, 95% CI –0.01 to 0.04; p = 0.37). There were no significant differences between groups in 4-week absolute work absenteeism (b = –2.59, 95% CI –12.8 to 7.62; p = 0.62), absolute work presenteeism (b = 1.48, 95% CI –0.43 to 3.38; p = 0.13) and combined relative work absenteeism and absolute work presenteeism (b = –0.02, 95% CI –0.92 to 0.87; p = 0.96) (see Table 5).
Chapter 4 Results from the process evaluation
Introduction
The intervention produced a small but significant decline from baseline to the 6-month follow-up in the mean number of steps per day (objectively measured) in the intervention group compared with the control group. The results highlight a need to explore and understand the processes underlying these findings.
Implementation
Participation and reach
A total of 9031 participants were invited to participate across four main sites (i.e. Lisburn, Stormont Estate, Queen’s University Belfast and Belfast City Hospital), as shown in Figure 2. In total, 853 participants were recruited and randomised. The demographic characteristics of the study population are shown in Table 4.
Intervention fidelity and ‘dose’
Standardised training manuals were provided to research staff to guide the intervention delivery and study conduct (including collection of outcome data and all e-mail communication with participants). Participants had access to an online discussion forum where queries or issues in relation to the day-to-day running of the intervention were voiced. Commonly reported issues, consistent across study sites, included issues with the sensors not accurately recording time spent doing physical activity across the work day, discrepancies between times recorded for other participants undertaking the same activity, the location of the sensors and the rewards available to participants.
On average, 1000 minutes (SD 987.08) of physical activity was recorded per participant via the PAL scheme physical activity monitoring system across the intervention period (n = 422 participants). Physical activity was captured by the PAL monitoring system on 43.41 (SD 33.21) days over the 6-month intervention, equating to physical activity being recorded on approximately one-third (32.71%, SD 25.05 days) of intervention days. With regard to the intervention ‘dose’, participants walked for at least 10 minutes on 32.80 (SD 28.87) days across the intervention period (Table 9).
Engagement | Mean (SD) |
---|---|
Physical activity monitoring system (N = 422) | |
Number of days participants recorded physical activity for at least 10 minutes (week 4)a | 5.99 (5.09) |
Number of days participants recorded physical activity for at least 10 minutes (month 6)a | 32.80 (28.87) |
Percentage of days participants recorded physical activity for at least 10 minutes (week 4)a | 29.95 (28.87) |
Percentage of days participants recorded physical activity for at least 10 minutes (month 6)a | 24.71 (21.76) |
Study website (N = 418) | |
Total minutes | 1170.68 (2048.27) |
Percentage of days participants logged on to the website (month 6) | 17.39 (20.06) |
Percentage of weeks participants logged on to the website (month 6) | 37.75 (32.45) |
Frequency of hits | |
Feedback and monitoringb | 13.7 (3.54) |
Rewardsb | 5.7 (4.47) |
Mapsb | 3.4 (3.97) |
Health information (physical activity)b | 0.5 (1.72) |
Health information (other)b | 1.2 (3.17) |
Discussion forumb | 1.9 (4.23) |
Study informationb | 4.0 (5.23) |
Percentage of total website time spent on | |
Feedback and monitoring | 44.66 (31.16) |
Rewards | 17.45 (23.78) |
Maps | 15.32 (24.07) |
Health information (physical activity) | 1.36 (7.34) |
Health information (other) | 3.04 (10.18) |
Discussion forum | 3.14 (10.21) |
Study information | 10.25 (18.58) |
Participants in the intervention group self-reported spending 2.31 (SD 2.12) hours per day on the internet. There was no significant difference in reported internet use (hours per day) between the intervention and control groups. Participants reported high confidence in using the internet at baseline (intervention group 8.67, SD 1.74), ranked from 1–10 on the Likert scale.
Across the intervention period, on average, participants earned 932.37 (SD 825.33) points by tracking their physical activity using the physical activity monitoring system. In addition, participants earned, on average, an additional 75.21 (SD 106.30) points from the scheme’s double-point days. Participants redeemed 39.33% (SD 42.51%) of points accumulated throughout the intervention for vouchers.
Responsiveness
The aim of the thematic analyses was to elicit the main themes and subthemes that were considered to be important factors for engagement/disengagement with the intervention and the maintenance of physical activity. The themes pertaining to the patients’ overall perceptions of the intervention were very positive, including those relating to mental health and well-being, social aspects and physical fitness and health. In terms of the key behaviour change and intervention components, themes included the issue of rewards by means of a financial incentive (and also disincentives linked to ‘voucher type’) and technology as a means of tracking progress, support and feedback (and also negative aspects relating to technological issues with sensors and key fobs (see General perceptions of the intervention for further details).
Participants
In total, nine focus groups (FGs) were conducted at the end of the intervention period across the study sites: Lisburn (n = 3 FGs; n = 21 participants), Stormont Estate (n = 2 FGs; n = 12 participants), Queen’s University Belfast (n = 2 FGs; n = 16 participants) and Belfast City Hospital (n = 2 FGs; n = 16 participants). Sixty six participants took part in the FG discussions [n = 52, 78% female; mean age 43.8 (SD 9.0) years]. At 12 months, 17 participants who had taken part in the 6-month FGs also took part in the 12-month FG discussions [n = 9, 53% female; mean age 46.1 (SD 9.3) years].
The following coding was applied to quotes to distinguish between the different FGs and semistructured interviews undertaken: FG denotes the focus group sessions (1–9) at 6 months (e.g. FG1, FG2); FG12 denotes the focus group session (12.1–12.6) at 12 months (e.g. FG12.1, FG12.2); MR denotes male respondent; FR denotes female respondent; R denotes retailer; SM denotes senior manager.
General perceptions of the intervention
The overall feedback from participants about the PAL scheme was positive. Many participants cited how the scheme had helped them engage in more physical activity and develop habits that could be maintained once the intervention had ended:
It’s been a very positive thing to get people out walking and to encourage them to walk more, with all the wee perks and to do a bit extra every day.
FG3, FR
So it’s been a brilliant success to get me walking and it’s now part of my daily routine.
FG8, FR
Type of physical activity
The type of physical activity on which the intervention was based was also viewed as positive by the participants, with many citing how convenient walking and/or jogging were compared with other activities:
. . . it doesn’t suit everybody to go to a class, to be there at a certain time . . . that’s the good thing about walking and running, that you can do it at any time it suits . . .
FG3, FR
It wasn’t hard, that’s what I quite liked about it. You could do as much or as little as you wanted, and that was quite nice.
FQ9, FR
Format of the physical activity loyalty scheme
Many participants felt that the format of the scheme, targeted at changing physical activity across the working day, was restrictive. Participants highlighted that the timing of the intervention did not enable them to account for how active they had been across the whole day:
What I found with it was that I was really doing the most of the stuff. I was a bit aggrieved because I was doing a lot in the morning which wasn’t recorded on the fob, you didn’t record those things.
FG6, FR
Some participants also found the intervention limiting because it did not take account of other types of physical activity:
I think it’s very restrictive in just having walking or running, because we do have very good staff membership for the gym, which a lot of the staff do avail of but you didn’t get any recognition for it . . .
FG3, FR
Increasing physical activity and health benefits
A number of key themes emerged when participants discussed their reasons for signing up to the PAL scheme. Increasing physical activity across the work day was cited by many participants as their main reason for signing up:
I felt I needed more exercise, I knew that it was beneficial, I thought it was a good idea and would help me do more.
FG1, FR
Mine was to get up from my desk and get out to walk more, that was my reason, more self-motivation . . .
FG7, FR
In addition to being more active, the associated health benefits of this behaviour change were also noted by participants, with many commenting that they signed up to lose weight or improve their health:
For me, it was health and fitness and good habit forming, plus you could see it was just better for you to get away from the desk, mentally.
FG6, FR
Some participants felt that they were already active during the working day and that the scheme was worthwhile to gain something for their efforts:
I did walk a lot anyway, at lunchtime and before work and stuff and I sort of thought I might as well get something for it. I was doing it anyway . . .
FG9, FR
Whereas other participants noted that helping out with research was a factor that encouraged them to sign up:
I do a lot of cycling and running and record it. I just figured that there’s a bit of data that maybe someone else could use, they may as well have my data.
FG1, MR
The convenience of an intervention being delivered within the workplace was also an influencing factor in encouraging people to take part:
I think generally if you can incentivise anything that’s within work hours, I know personally for me, with working with a young family, if I can get that activity within those hours from 8 to 6 then it will really work for me . . .
FG8, FR
Key barriers to and facilitators of the physical activity loyalty scheme
Key barriers to and facilitators of the PAL scheme and of being active throughout the working day were identified through the FG discussions. Work demands, time and the weather were cited by participants as barriers to being more active at work and participating in the PAL scheme. The structure of the working day meant that it was not always possible for participants to be active during lunchtime:
I find, especially in this place, you don’t always get the chance to get out at lunchtime anyway. Once I started, if I didn’t get out at lunchtime I would be more inclined to make sure that I did get out in the evening for a walk.
FG6, MR
In addition, increased workload had an impact on the time participants had available to be active:
But as term got busier and we got into September it just got more and more difficult to find the time to fit it all in.
FG9, FR
Participants highlighted how the weather was also a barrier, deterring them from taking part in the scheme on occasions:
We walked in a group, so I think if it rained the girls got their hair wet, so that sort of stopped them, really. If it rained we didn’t walk basically.
FG6, MR
With regard to facilitating increasing physical activity through the PAL scheme across the working day, a number of key themes emerged. Access to nice areas for physical activity was noted as a facilitator:
We’re very fortunate in this area, we’ve this lovely green park and you can walk out there and walk back.
FG6, FR
Support within the workplace from colleagues was another facilitator of physical activity, with participants commenting on the importance of sharing experiences of being involved in the scheme:
Even if you didn’t want to walk in a group, just chatting about it ‘How much did you do . . .?’ I think that works for a lot of people.
FG8, FR
In addition to support from colleagues, the attitude of employers was noted as another important influence:
I think that this council is very good as an employer, as they do put on the likes of the classes at lunchtime, you have access to the gym and everything.
FG3, FR
General benefits of the physical activity loyalty scheme
A number of themes emerged from the FG discussions on the benefits participants received from their participation in the PAL scheme. Benefits of the intervention included increased levels of physical activity, health benefits, social benefits and increased productivity. Participants highlighted how the scheme had instigated changes in their usual routine, leading to increased physical activity across the day:
If I got the train in I would actually make my route longer to make sure that I passed a few points in the way in and on the way home, rather than just straight here.
FG4, MR
A number of health benefits were listed by participants, including the impact that being more active had on their physical well-being:
I wanted to lose weight. I’ve lost 2 stone since I started, but I’ve done lots of other activity. But it spurred me on and gave me the motivation, and actually I built up my fitness so that I could do some of the other activities.
FG9, FR
In addition to the positive impact that the intervention had on physical health, participants also noted the benefits in relation to their mental health and well-being:
It’s quite nice too, because even the mindfulness idea, as you’re walking along on your own you’re more mindful of what you’re doing, and you’re into health issues there too.
FG8, FR
There were mixed responses from FG participants with regard to how physical activity behaviour change was maintained post intervention. A number of participants noted how the PAL scheme had changed their routine from doing no physical activity across the working day to now being more active:
I would walk more now; I wouldn’t have thought of doing it at all.
FG4, FR
Incorporating physical activity into habits and daily life
In addition, being involved in the intervention encouraged participants to make small changes to their daily habits to facilitate physical activity within the working day:
. . . I do at least as much now, even after it’s finished, as I did when I was doing it, and in fact I probably do more now and I’m probably much more conscious of what I do because of even trying to think up how I was going to make those points in a day. I keep walking, I still walk up and down from the school every day, and I do it at least five times a week, if I can, to build up 150 minutes.
FG9, FR
Other participants commented on how being involved in the intervention had made them think about their physical activity and that they were still making efforts to increase their activity as a result of being involved in the PAL scheme:
I haven’t continued as much but I am really determined to, because I am aware of the fact when I’m not walking and that your health is not quite as good, so I have made more of a conscious effort recently to try and get back into it, especially now that the days are a little bit longer and it’s a bit brighter outside. I kept up other exercise, other than walking as well, quite consistently, even more so than I would have done previously.
FG9, FR
For some, the extra physical activity that they were doing during the PAL scheme stopped once the concept of the points and rewards was removed:
I did it when the vouchers were there but now I wouldn’t . . . I would go outside but I wouldn’t actually make an effort now. So I would never dream of going for a walk or anything during work.
FG7, FR
Social aspects of the physical activity loyalty scheme
The participants commented on the social aspects of being involved in the PAL scheme, with many citing the intervention as a good way of socialising with colleagues:
There was a social element to it too, like someone would say ‘oh, do you want to go outside and get some points?’
FG6, FR
Participating in the intervention group identified a number of benefits specific to the workplace. Within the FG discussions, participants noted that the intervention provided them with the opportunity to have a proper break during the work day:
Yeah, usually you have your lunch here, sometimes at your desk, so you aren’t getting away from it, so it’s good to get outside and get fresh air and you’re more awake in the afternoons then because you have been out.
FG3, FR
Furthermore, participants felt that the opportunity to be active during the day had a positive effect on their productivity:
I probably worked harder because I’d normally be sleeping after lunch.
FG4, MR
As well as increasing levels of physical activity across the work day, participants highlighted an additional effect being involved in the PAL scheme had on other types of physical activity they were involved in:
It triggered off for me to go and join a club, a rowing club, because you weren’t really aware that you weren’t doing as much and you were sitting quite a bit at the desk, and you thought ‘right OK’ . . .
FG7, FR
In addition, the PAL scheme increased awareness of other improvements individuals could be making to their overall health and well-being:
Like others, it stimulated me to look at other parts of my lifestyle and try to amend them, to make small changes as well.
FG8, MR
Perceptions of intervention components and behaviour change techniques
The key themes in relation to participants’ thoughts on the different intervention components of the PAL scheme for behaviour change are summarised in Table 10. These include the use of incentives, sensors, maps, feedback, fobs, e-mail and social support.
Intervention components | Quotation |
---|---|
Sensors | I do quite a bit of walking and I was just outside of the area and I kind of felt I was changing my walk just so I could walk past the sensors, whereas it would be handy if it wasn’t quite so dependent on the sensorsFG2, MRYeah, they were definitely occasions, I think everybody experienced it at some stage. It hadn’t recorded parts of your journey and the sensors hadn’t been working. There was other things as well. If you went so far and you didn’t come across a second sensor, it was only calculating your journey from . . . between two sensors rather than the total time of the journeyFG5, MRYou sort of had to plan, which sometimes I did plan, I thought then . . . I know you were doing it for your research but I thought this is just me planning my route round this way to do it, as opposed to this would be my normal walkFG7, FR |
Maps | I thought the wee maps were good. I looked at them, because you could think ‘oh, I could go out now but I have only half an hour.’ So you could have a look and see where you could walk . . .FG7, FRI found they weren’t that easy to read . . .FG8, FR |
Feedback | It was good to go in and see how far you had walked, how long you had been out walkingFG5, FR. . . but, for me, whenever I was in the habit of it, it was seeing the circle be green, if I got to Friday and there was a chunk left, I would think ‘oh, I’d better get out and try to make it to the full amount’FG6, FR |
Fob | But there was a number of times I kept forgetting the key fob. I had my keys on it and then they keys were on the desk and you would walk out without it, so if the sensors were the clip on belt varietyFG8, FRI quite like the idea of a fob that you maybe wore, because I don’t carry my phone around . . . something connected to the phone, that could in some ways be more cumbersome, or something where the two would be synchronised . . .FG2, FR |
E-mail prompts | The weekly e-mails helped as well, just to spur you on a Monday morning, just to remind you that you were involved and you had to participateFG9, FRI got so many e-mails, I got annoyed with them, the ones that were ‘You should be out walking’FG6, MR |
Support | . . . I found they were always really quick at getting back to me, even if I rang or I e-mailed, it was really goodFG2, MR. . . answered the e-mails really quickly and were really responsive and that helped keep the motivation going as well, so we do appreciate what you didFG9, FR |
With regard to the sensors, participants reported some issues that affected the day-to-day running of the intervention, including issues with the accuracy, placement and restrictiveness of the sensors (see Table 10). The participants had mixed opinions on a number of website components, including the maps and e-mail prompts. The feedback element of the website was a positive feature for many, with participants highlighting that the visual feedback often prompted them to be more active.
A number of themes emerged in relation to rewards (retail vouchers) offered to participants in exchange for points they had accumulated during their participation in the PAL scheme. Some participants felt that the vouchers did not provide a sufficient reward for the effort and activity undertaken:
You’ll get incentives joining gyms anyway, so there wasn’t really any incentive in the incentives, really, unless it was a free cup of tea. Walking ten miles for a cup of tea. It needed to be more; the incentives just weren’t enough for me.
FG5, FR
Meanwhile, other participants felt that the vouchers were limited:
The vouchers were an issue, when you go to get them there wasn’t the ones you wanted, such as Argento. Just there was only so many available.
FG3, FR
Participants noted that the issues around the format and availability of the vouchers had an impact on their interest in other elements of the intervention:
And I found then that I lost interest because the points weren’t really going anywhere. And then whatever points I had, the incentive wasn’t good enough for the points I had.
FG5, FR
Focus groups at 12 months post baseline
The main aim of the FGs conducted at 12 months was to explore behaviour change maintenance. On the whole, participants referred to their maintained, or at least their attempt to maintain, physical activity levels and a desire to keep up with the levels that they had achieved during the 6-month intervention:
I know I started well but I think I wasn’t consistent the whole way through because of other factors that were happening at work at the time, but I tried to maintain a level, I was trying to sort of hit the target a week.. after the scheme ended I did try and keep it going.
FG12.4, MR
The detailed walking routes provided during the scheme were also still utilised after the intervention period ended:
. . . so, I would do that route more or less exactly everyday whenever I do go out.
FG12.4, MR
. . . and in the mornings I’m still doing a lot of the same routes.
FG12.3, FR
For participants, the notion of building physical activity into their day was a key aspect to maintaining levels of physical activity:
Yeah, and I’ll tell you another thing, while even waiting on the train on the platform I’ll be walking up and down the platform, rather than just stand there, I’ll walk.
FG12.1, FR
Another key aspect was the notion of making conscious efforts to move more:
Well now, if I’m cooking, I would tend to do that step, from one side to the other or back and forth, so as I’m not just standing still.
FG12.1, FR
This was also noted with regard to the idea of looking for opportunities to be active beyond the 6-month intervention period:
It’s something I can work into my lifestyle, and even when you don’t have it you’re sort of thinking well is there an opportunity to perhaps go for a walk at lunchtime to just keep that activity going.
FG12.4, MR
Participants commented on looking for additional opportunities in their day to be active:
. . . I can adapt my pattern and I spotted two other opportunities, the beginning of the working day and the end of the working day, where I can do something that fits in.
FG12.4, MR
Habit, be that continuing with the established walking routes or continuing with their lunchtime walk or step goals, alongside seeking out opportunities to be active were common threads throughout the FGs for maintained physical activity behaviour change after the scheme had ended.
Senior managers of participating employees
Semistructured interviews were also undertaken with senior managers of six participating employers (n = 7 managers) and four participating retailers. Feedback about the intervention was positive from employers, with themes emerging from the interviews about employee benefits, both by means of getting active (a focus on physical and mental health) and relating to workplace productivity. Senior managers’ perceptions of the intervention were aligned with participants’ perceptions, regarding time as a key barrier to physical activity in the workplace.
Senior managers’ overall perceptions of the physical activity loyalty scheme
Employers’ overall thoughts on the PAL scheme were positive, with managers highlighting the benefits of getting their employees to be more active:
We know that walking is better than being sedentary and it reduces lots of different diseases and things. So in principle it’s very good.
SM2
It’s the health benefits . . . that people are more active. You know yourself, it reduces risks of lots of different types of diseases . . .
SM2
. . . it’s the mental health and people are enjoying the brighter evenings and the sunshine.
SM4
The wider benefits of the intervention were also noted for the employer in relation to productivity:
A lot of them [employees] commented recently on feeling more productive in the afternoon when they’ve had a chance to get some fresh air and a chance to stretch their legs. We’ve seen a great stretch in productivity.
SM4
In addition, senior managers commented on how the intervention aligned with other initiatives within the workplace:
I think it’s very valuable to add some evidence to the research that’s already been done, and obviously it fits very nicely with us in terms of workplace health . . .
SM3
In addition, employers commented on the benefits of the intervention in terms of making contacts with other businesses and organisations:
. . . suppose in terms of the business side of it too, in terms of the partnerships with the business side, in terms of incentives, that’s of value too.
SM6
Senior managers highlighted a number of barriers to promoting physical activity within the workplace. Time and resources were commonly cited as barriers to being active within the working day:
Time. I think that’s the reality, is we are all increasingly . . . work pressure is probably the most significant barrier, certainly in my opinion and from my own personal experience . . .
SM1
Employers noted the need for a change of attitudes at management level to facilitate such schemes:
. . . question comes from their line managers: ‘Where are the staff going to get the time for this?’ And our job is really to convince them of the bigger picture . . .
SM1
Retailers
Semistructured interviews were undertaken with four participating retailers. A number of key themes emerged with regard to why retailers became involved with the PAL scheme, including benefits for their business and the broader benefits of the scheme for participants. Of the participating retailers interviewed, all cited the potential benefits being involved in the PAL scheme could have for their business.
Potential of the physical activity loyalty scheme to improve business
The PAL scheme had the potential to increase use and sales within participating businesses:
. . . somebody that hadn’t been into the shop before, they’ve got a voucher so they might be encouraged to come in, they might like what they see when they come in . . .
R1
It also provided businesses with an opportunity to promote their services and entice participants into their businesses over other similar businesses surrounding the workplace:
The opportunity to go down Botanic Avenue and go into those cafes and restaurants is always there. So this was an opportunity for me to promote the cafes and restaurants on the site.
R1
Potential benefits of the physical activity loyalty scheme for participants
Participating retailers also acknowledged the benefits such a scheme could have on the health and well-being of participants, and cited this as another factor behind their willingness to participate in the scheme:
. . . doing more exercise is kind of what everybody needs to be doing but people don’t do it, and encouraging them in some way, I think is definitely a good idea.
R2
Incentives and rewards
A number of themes emerged in relation to the incentive-based element of the scheme, including (1) the concept of incentive-based physical activity, (2) the type of reward and (3) the value of the rewards. All participating retailers felt that incentivising participants to increase their physical activity was a good idea, from both a behaviour change perspective and also for the added benefit that it could have for businesses:
. . . if I knew there was something at the end of it that you’re going to or you’re working towards getting money off or working towards a different voucher then it makes it seem more worthwhile.
R2
We like it from the idea of the retail point of view because they don’t have to pay anything and they’re getting free promotion, which everybody should appreciate . . .
R3
The type of reward offered varied across the participating businesses, with different reward structures being perceived to have a different influence from the retailers’ points of view. Some retailers offered a cash value voucher as their reward, with a view that participants would spend more when cashing in their voucher:
So a £10 voucher, there’s a fair chance if they come in they will upscale in terms of what they’re going to buy. So you’ll hopefully get it in the long-term.
R4
There was consensus among retailers that the rewards offered to participants were not ‘overly generous’.
The retailers highlighted a number of issues that may influence upscaling the present intervention or taking part in similar schemes in the future. All retailers spoke about the sustainability of the incentive-based approach and highlighted that they would take part in future schemes if they were getting a good return from a business perspective:
. . . future for me going, is down the voucher route. So yeah, it’s sustainable providing people come and use it.
R4
Retailers also cited requests from other organisations and schemes as a potential barrier, with most retailers constantly being asked to provide rewards and sponsorship:
But the down side is we as a shop again are always asked for vouchers and sponsorship and you have to pick what you can go for.
R4
Questions regarding the impact and financial gains from participating in the scheme were cited as another key theme in relation to future participation. All retailers highlighted the need for feedback from participants and the need to track how the rewards were used, which was not monitored within the present study by the researchers or the businesses themselves. Retailers noted that they would make efforts to track voucher use and spending in future. In addition, retailers highlighted that tracking use could also provide them with an opportunity to gain feedback from new customers:
. . . I would just like some feedback from the participants of the scheme in terms of their experience . . . on a purely customer-focused level, did they have a positive experience when they used the cafes . . .
R1
Chapter 5 Results from the health economics analysis
Overview
The cost-effectiveness analysis (CEA) of the PAL scheme is presented in this chapter. Overall, the intervention induced a small (but significant) decline in the mean number of steps per day by the intervention group compared with the control group. Therefore, the economic evaluation took the form of a within-trial CUA and CBA, comparing the intervention group with the waiting list control group. In line with NICE methods for the development of NICE public health guidance, the CUA adopted a public sector perspective. 127 Costs included intervention costs (apportioned per participant) and health-care resource use. Health outcomes were expressed in terms of QALYs accrued over the 6-month trial period in the CUA. Results are presented using an ICER estimated by dividing the adjusted difference in mean costs between groups by the adjusted difference in mean QALYs between groups. ICER estimates were compared with a £20,000–30,000 per QALY threshold applied by NICE. 127 The CBA was undertaken from an employer’s perspective by employing a ‘net-cost model’96 by incorporating not only the intervention cost but also the avoided costs of absenteeism and productivity loss owing to sick days. It is a technique recognised in the NICE guideline for economic evaluations for public health interventions to allow the broader benefit of the intervention to be considered in decision-making. 86 All analyses were undertaken according to the principle of intention to treat and in Stata®, version 12.0 (StataCorp, College Station, TX, USA).
Intervention costs
The intervention costs are detailed in Table 11. Intervention costs included the retail vouchers, sensors and key fobs, electrical engineering staff and travel for maintaining the sensors, and a computer scientist for monitoring and managing the data from the sensors and maintaining the website. The cost for the placement of the sensors on the lampposts was not included as it was refundable by local government. The vouchers cost £21,000 in total. These voucher costs could be greatly reduced if there was more meaningful engagement with the local authority/city management groups to facilitate better deals with the retailers. Therefore, the costs of vouchers were varied in sensitivity analysis. The lifespan of the sensors was estimated to be 2 years because the screw bosses were made of plastic, which limits the number of battery replacements. With upgraded screw bosses, the lifespan can be prolonged to over 5 years. The battery used in the key fobs cannot be replaced so the lifespan for the current key fobs in the trial is the life of the battery (i.e. 9 months). This short lifespan will be extended to 5 years with a battery replaceable design. Therefore, in sensitivity analyses, the costs for sensors with upgraded screw bosses and battery-replaceable key fobs are considered to explore the influence of upgraded technology on the cost-effectiveness of the intervention. The cost per participant for each disaggregable item is shown in Figure 3, from which the retail voucher and sensor together accounted for > 90% of the total cost.
Cost items | Unit cost (£) | Quantity | Total cost for 6 months (£) |
---|---|---|---|
Retail vouchers (Club Marketing Ltd) | N/A | N/A | 21,000.00 |
Equipment | |||
Keyrings | 0.35 | 503 [457 participants × (1 + 10%)]a | 117.37b |
Numbering of keyrings | 0.04 | 503 [457 participants × (1 + 10%)]a | 16.91 |
Sensor cost | 151.55 | 70 | 2792.16c |
Staff time and travel | |||
Sensor maintenance (electrical engineer) | 13.33/hour | 26 hours (1 hour per week × 26 weeks) | 346.58 |
Sensor maintenance (travel) | 0.40/mile | 338 miles (13 miles per week × 26 weeks) | 135.20 |
Website maintenance (computer scientist) | 13.33/hour | 78 hours (3 hours per week × 26 weeks) | 1039.74 |
Total (6 months) | 25,447.96 | ||
Cost per participant in the intervention group (N = 457) | 55.68 |
FIGURE 3.
Cost of the PAL scheme over 6 months (per participant).

Missing data
The number and percentage of missing data for each collected NHS resource use item and EQ-5D-5L questions at baseline and at the 6-month assessment point are shown in Table 12 for intervention and control groups, respectively. Baseline for the resource use refers to the 6-month period before randomisation completed by participants retrospectively at baseline. All of the missing data for the EQ-5D-5L responses were caused by whole questionnaires missing, which can be seen from the uniform proportion of missing data for the five dimensions. A similar proportion of missing data was observed across the randomised groups and for both cost and EQ-5D-5L data. Approximately 15% of data were missing at baseline and 40% of data were missing at the 6-month follow-up.
Data items collected from the trial | Intervention (N = 457) | Control (N = 396) | ||
---|---|---|---|---|
Baseline, n (%) | 6 months, n (%) | Baseline, n (%) | 6 months, n (%) | |
NHS resource use | ||||
GP | 73 (15.97) | 196 (42.89) | 63 (15.91) | 161 (40.66) |
Nurse | 73 (15.97) | 206 (45.08) | 62 (15.66) | 165 (41.67) |
Physiotherapist | 73 (15.97) | 206 (45.08) | 63 (15.91) | 164 (41.41) |
A&E | 73 (15.97) | 207 (45.30) | 63 (15.91) | 166 (41.92) |
Outpatient | 73 (15.97) | 205 (44.86) | 63 (15.91) | 166 (41.92) |
Nights in hospital | 73 (15.97) | 207 (45.30) | 62 (15.66) | 170 (42.93) |
EQ-5D-5L dimensions | ||||
Mobility | 67 (14.66) | 195 (42.67) | 57 (14.39) | 157 (39.65) |
Self-care | 67 (14.66) | 195 (42.67) | 57 (14.39) | 157 (39.65) |
Usual activities | 67 (14.66) | 195 (42.67) | 57 (14.39) | 157 (39.65) |
Pain and discomfort | 67 (14.66) | 195 (42.67) | 57 (14.39) | 157 (39.65) |
Anxiety and depression | 67 (14.66) | 195 (42.67) | 57 (14.39) | 157 (39.65) |
Resource use and costs
Table 13 reports the use of each resource item (mean number of visits, SD, median, minimum and maximum) accrued over the 6-month primary follow-up period in the intervention and the control group. Overall, there were no statistically significant differences in the use of each resource item between the groups.
Resource use (number of visits) | Group | ||||||||||
---|---|---|---|---|---|---|---|---|---|---|---|
Intervention (N = 457) | Control (N = 396) | p-value | |||||||||
n | Mean | SD | Median | Min.–max. | n | Mean | SD | Median | Min.–max. | ||
GP | 261 | 1.21 | 1.36 | 1 | 0–7 | 235 | 1.24 | 1.53 | 1 | 0–10 | 0.78 |
Nurse | 251 | 0.71 | 2.08 | 0 | 0–20 | 231 | 0.54 | 1.25 | 0 | 0–10 | 0.28 |
Physiotherapist | 251 | 0.51 | 1.49 | 0 | 0–8 | 232 | 0.76 | 2.77 | 0 | 0–23 | 0.20 |
A&E | 250 | 0.10 | 0.38 | 0 | 0–3 | 230 | 0.07 | 0.29 | 0 | 0–2 | 0.48 |
Outpatient | 252 | 0.43 | 0.99 | 0 | 0–8 | 230 | 0.57 | 1.28 | 0 | 0–8 | 0.17 |
Inpatient | 251 | 0.04 | 0.28 | 0 | 0–3 | 229 | 0.04 | 0.22 | 0 | 0–2 | 0.98 |
Nights in hospital | 250 | 0.16 | 1.86 | 0 | 0–28 | 226 | 0.13 | 0.86 | 0 | 0–7 | 0.86 |
Table 14 shows other services used by the participants in each group. Other services and medications are not included in the total resource use owing to the similar amount of service use between groups, their randomness (i.e. they are most likely unrelated to the intervention) and the difficulty in matching unit cost with the free-text inconsistent reporting.
Group | |||
---|---|---|---|
Intervention (N = 457) | Control (N = 396) | ||
Services | Frequency (times) | Services | Frequency (times) |
Services used in both groups | |||
Dentist | 77 | Dentist | 86 |
Counselling | 22 | Counselling | 11 |
CBT | 1 | CBT | 6 |
Chiropractor | 1 | Chiropractor | 2 |
Diabetic clinic | 1 | Diabetic clinic | 2 |
Breast screening | 1 | Breast screening | 1 |
Day inpatient | 3 | Day inpatient | 1 |
Optician | 7 | Optician | 1 |
Orthopaedic referral | 1 | Orthopaedic surgery | 1 |
Private physiotherapist | 1 | Private physiotherapist | 1 |
Other services | |||
MRI scanning | 4 | Pain clinic | 5 |
Haematology | 3 | Podiatry | 4 |
Kinesiology | 3 | Asthma clinic | 1 |
Blood donation | 2 | Cardiac rehabilitation | 1 |
Mammogram | 2 | Hearing test | 1 |
Sports massage | 2 | Knee consultant | 1 |
Bone scan | 1 | Operation on broken wrist | 1 |
Dermatology | 1 | Private orthopaedic surgeon | 1 |
Dietitian at hospital | 1 | Surgeon | 1 |
Flu jab | 1 | Treatment | 1 |
Medical day-case unit | 1 | GP/nurse assigned to workplace | 1 |
Therapist | 1 | ||
Radiography | 1 | ||
Total number of services | 138 | 130 |
Table 15 compares the cost of service use between the groups with four regression approaches, as specified in Chapter 2, Health economics, Methods. There is no statistically significant difference in resource use between the groups. Despite this, the decrease of resource use in the intervention group compared with the control group was larger (approximately £60) with the gamma distribution adjusting for covariates than the OLS (approximately £15) unadjusted model, in both complete case and multiply imputed data.
Statistical methods | Mean | SEa | 95% CIa | p-value |
---|---|---|---|---|
Complete case:b unadjusted | ||||
Intervention | 200.00 | 49.65 | 99.31 to 300.69 | 0.829 |
Control | 212.41 | 28.21 | 155.21 to 269.62 | |
Complete case:b adjustedc | ||||
Intervention | 193.25 | 32.42 | 129.71 to 256.80 | 0.326 |
Control | 249.89 | 44.92 | 161.84 to 337.94 | |
MI:d unadjusted | ||||
Intervention | 200.50 | 41.39 | 114.99 to 286.01 | 0.755 |
Control | 216.47 | 26.72 | 161.27 to 271.67 | |
MI:d adjustedc | ||||
Intervention | 190.14 | 31.21 | 128.67 to 251.60 | 0.235 |
Control | 247.70 | 39.49 | 170.16 to 325.24 |
Health-related quality of life
The EQ-5D-5L health utility values and visual analogue scale (VAS) for each group at baseline and 6 months are shown in Table 16 (complete case) and Table 17 (after imputation). The completeness at the 6-month follow-up was 57% (262/457) for the intervention group and 52.3% (239/457) for the control group. This was lower than the rate of completeness at baseline, which was 85.3% (390/457) and 74.2% (339/457) for the intervention and control group, respectively. A total of 45.0% (328/729) of participants recorded a state of ‘full health’ at baseline and 29.1% (146/501) of participants recorded the state of ‘full health’ at 6 months. The utility values for both groups declined over the 6 months from approximately 0.89 to 0.83. There was no statistically significant difference between groups at both baseline and 6 months.
EQ-5D-5L | n | Mean | SD | Min., max. | p-valuea |
---|---|---|---|---|---|
Baseline index score | |||||
Intervention | 390 | 0.887 | 0.113 | 0.316, 1 | 0.736 |
Control | 339 | 0.890 | 0.120 | 0.320, 1 | |
6-month index score | |||||
Intervention | 262 | 0.828 | 0.143 | 0.310, 1 | 0.650 |
Control | 239 | 0.835 | 0.150 | 0.238, 1 | |
Difference between baseline and 6-month index score | |||||
Intervention | 242 | –0.053 | 0.125 | –0.586, 0.259 | 0.401 |
Control | 219 | –0.064 | 0.117 | –0.453, 0.346 | |
Baseline VAS | |||||
Intervention | 389 | 82.42 | 13.83 | 38, 100 | 0.317 |
Control | 339 | 83.76 | 14.27 | 0, 100 | |
6-month VAS | |||||
Intervention | 262 | 77.95 | 16.16 | 19, 100 | 0.827 |
Control | 239 | 78.26 | 16.08 | 19, 100 |
Cost–utility base-case analysis
The cost-effectiveness results for the intervention are presented in Table 18. Overall, the intervention was approximately £25 more costly, but had no effect on QALYs. The average cost per participant was £253.5 (95% CI £188.4 to £318.6) in the intervention group and £227.64 (95% CI £170.9 to £284.4) in the control group. Mean QALYs accrued over the 6-month trial period were 0.4157 (95% CI 0.4077 to 0.4238) for the intervention group and 0.4158 (95% CI 0.4057 to 0.4260) for the control group, leading to a 0.0000891 (95% CI –0.008 to 0.008) lower QALY gain in the intervention group than in the control group.
Treatment group | Cost (£)a,b | QALYb | ||||
---|---|---|---|---|---|---|
Mean | SE | 95% CI | Mean | SE | 95% CI | |
Intervention (N = 457) | 253.49 | 33.06 | 188.41 to 318.57 | 0.4157 | 0.0041 | 0.4077 to 0.4238 |
Control (N = 396) | 227.64 | 28.90 | 170.86 to 284.43 | 0.4158 | 0.0052 | 0.4057 to 0.4260 |
Difference (95% CI) | 25.85 | 28.44 | –29.89 to 81.60 | –0.0000891 | 0.004 | –0.008 to 0.008 |
ICER | –£290,178 per QALY | |||||
95% CI for ICER (from bootstrap) | –£480,011.80 to –£100,336.10 per QALY |
The cost-effectiveness plane for the base-case analysis is shown in Figure 4. The dyads come from the 1000 bootstrap iterations. The x-axis represents the bootstrapped incremental QALYs between the randomisation groups and the y-axis represents the incremental costs. The bootstrapped pairs of cost difference and QALY difference were across the four quadrants, indicating greater uncertainty around the estimate of ICER. The bootstrapped pairs were almost evenly (47% vs. 53%) distributed across the y-axis in the first and second quadrant, caused by the close to zero mean incremental QALY. The existence of dyads in the third and fourth quadrants indicates that there was a small possibility (i.e. 13.4%) that the intervention is cost saving. The proportion of the simulated pairs distributed in each quadrant is presented in Table 19.
FIGURE 4.
Cost-effectiveness plane representing 1000 bootstrapped cost difference and QALY difference pairs.

Quadrant | Description | Probability (%) |
---|---|---|
1st, north-east | Intervention is more costly and more effective | 38.6 |
2nd, north-west | Intervention is more costly and less effective | 48.0 |
3rd, south-west | Intervention is less costly and less effective | 5.0 |
4th, south-east | Intervention is less costly and more effective | 8.4 |
The CEAC for the base-case analysis is shown in Figure 5. The probability that the intervention was cost-effective at the £30,000 threshold was 34.6%. This corresponds to the proportion of dyads on the right side of the sand colour line in the first, third and fourth quadrant of the cost-effectiveness plane in Figure 4.
FIGURE 5.
Cost-effectiveness acceptability curve.
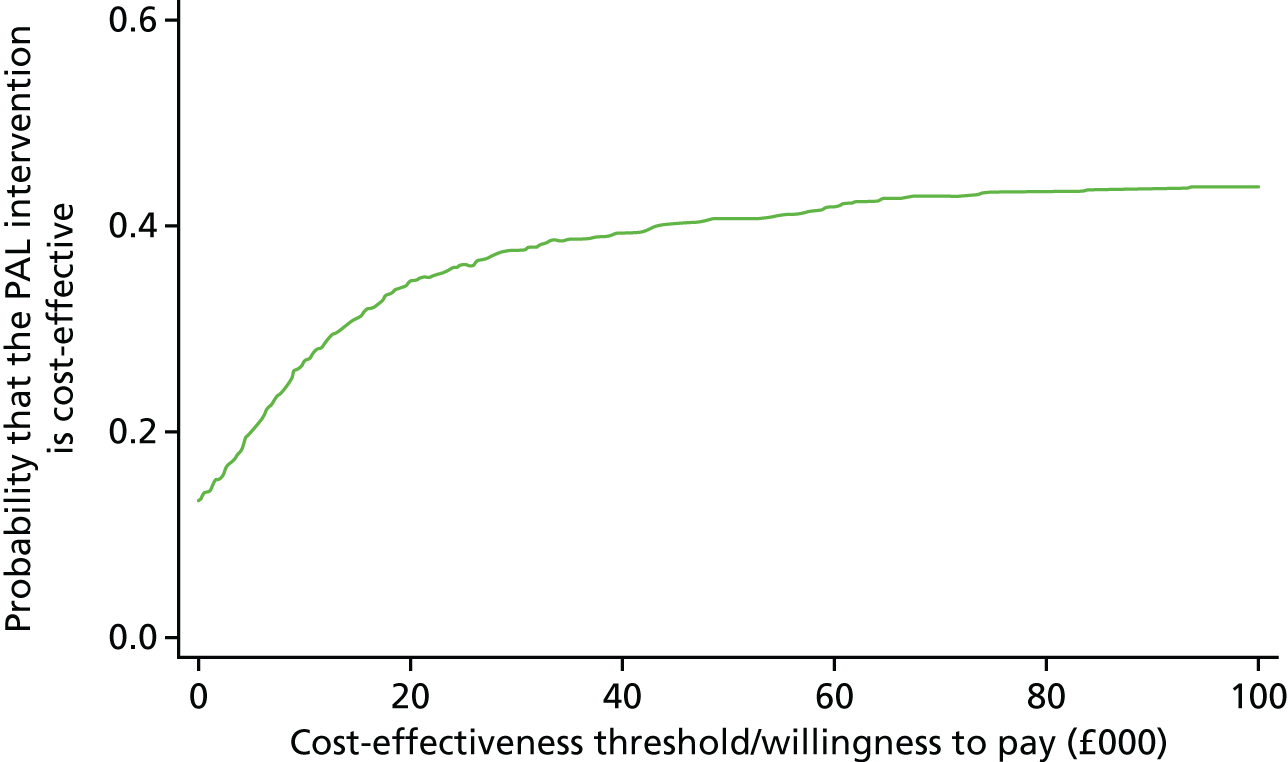
Sensitivity analysis
Results for the sensitivity analysis are presented in Table 20.
Analysis | Incremental cost (intervention – control) (95% CI) (£) | Incremental QALYs (intervention – control) (95% CI) | ICER (£ per QALYs gained) | Probability of being cost-effective at the threshold of £20,000 per QALY gained (%) |
---|---|---|---|---|
Base-case analysis | ||||
Base case (6 months) | 25.85 (–29.89 to 81.60) | –0.0000891 (–0.008 to 0.008) | –290 (173.9) | 34.6 |
Voucher business mode | ||||
Club Marketing Ltd | –32.71 (–89.84 to 24.43) | –0.0000891 (–0.008 to 0.008) | 367 (102) | 60.8 |
£10 ‘shops for all’ voucher | –5.34 (–61.21 to 50.53) | –0.0000891 (–0.008 to 0.008) | 599 (13.87) | 48 |
Marketing consultant | –13.19 (–69.27 to 42.89) | –0.0000891 (–0.008 to 0.008) | 148 (040.2) | 52.1 |
Least expensive scenario | ||||
Upgraded devices, club marketing | –42.61 (–100.41 to 15.19) | –0.0000891 (–0.008 to 0.008) | 47 (828.1) | 69.3 |
Missing data | ||||
Complete-case analysis (n = 427), gamma family, log-link | 36.24 (–60.52 to 133.00) | –0.00047 (–0.013 to 0.012) | –76 (978.1) | 35.7 |
Cost–benefit analysis employing net-cost model
It was reported earlier in Table 5 that the absolute absenteeism hours over a 4-week period for the intervention and control groups was 4.04 (66.11) and 7.01 (51.40), respectively (p = 0.62). The difference was estimated to be 2.59 hours over a 4-week period after adjusting for stratum, season and cluster (p = 0.62). This equates to 15.54 hours pro rata for a 6-month time period. After attaching the hourly salary values, the avoided cost of absenteeism and the net cost of intervention for each representative salary grade is shown in Table 21. This estimated cost saving for the employers ranged from £66 to £735 depending on the wage rate employed.
Pay grade | Avoided cost of absenteeism (£) | Net cost of the intervention (intervention cost minus the avoided cost of absenteeism) (£) |
---|---|---|
Lowest (NHS band 1, £7.8 per hour) | 121.21 | –65.53 |
Middle (NHS band 8A, £22.86 per hour) | 355.24 | –299.56 |
Highest (NHS band 9, £50.85 per hour) | 790.21 | –734.53 |
Given the difference in absolute absenteeism was not statistically significant, Figure 6 presents the uncertainty of the potential economic benefit from the employer’s perspective for each representative salary grade. At current intervention cost (= £55.68), the probability that the PAL intervention is cost saving from employer’s perspective is 64% for the high-salary group, 62% for the middle-salary group, and 57% for the low-salary group.
FIGURE 6.
Uncertainty surrounding the potential economic benefit from the employer’s perspective: net cost model result.
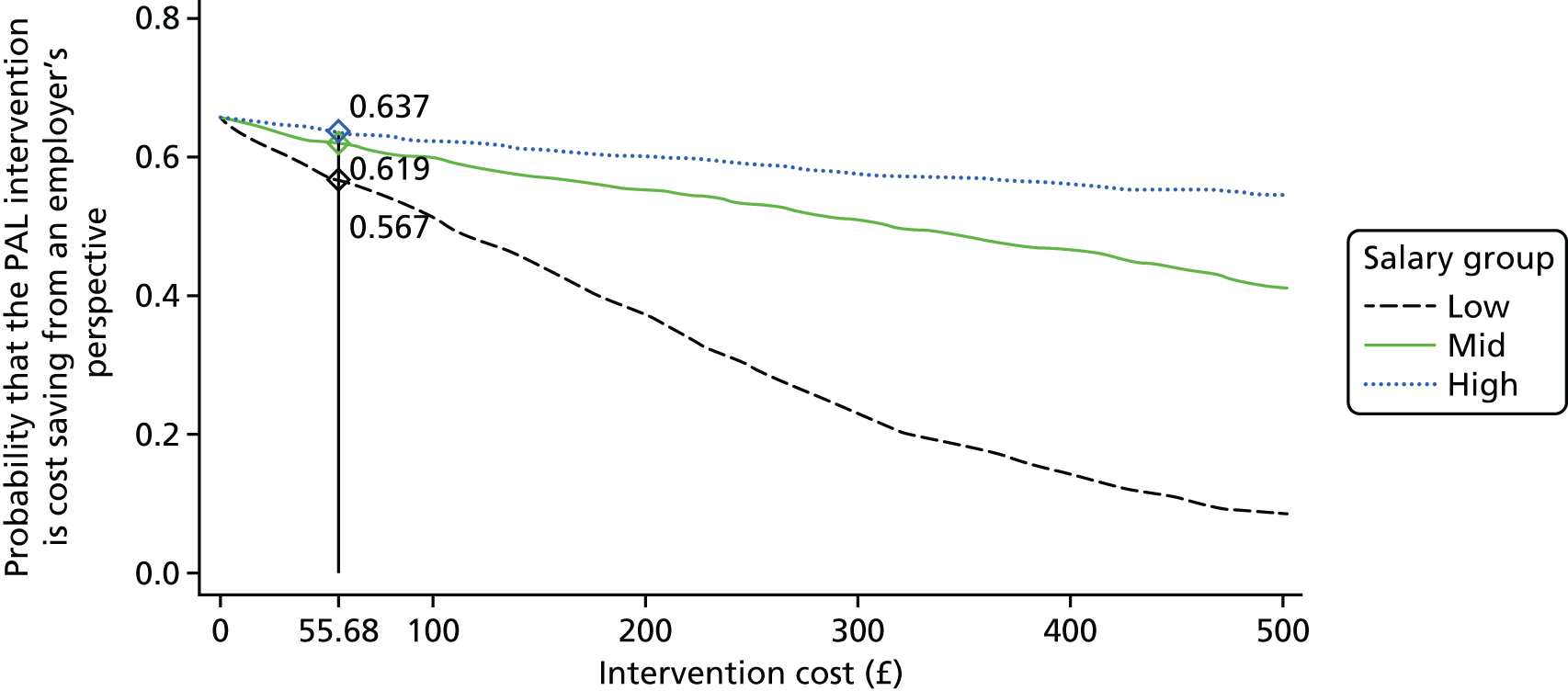
Chapter 6 Results from the behavioural economics analysis
Contingent valuation was used to elicit the minimum financial incentives required by participants to increase general physical activity (PA). Next, discrete choice modelling was utilised to examine the monetary value required for increasing different types of PA (i.e. walking or cycling to and from places, and moderate-intensity and vigorous-intensity recreational PA).
Two double-hurdle models were then estimated, with the first examining which individual sociodemographic characteristics were associated with the decision to increase PA at 6-month follow-up compared with baseline, and analysis of the extent to which PA increased; the second investigated the influence of behavioural economic traits and other variables on the decision to increase PA at 12-month follow-up (compared with 6 months) and the amount by which PA increased.
Contingent valuation
On average, participants’ WTA financial incentives was £1.38 (95% CI £1.16 to £1.61) for increasing general PA for 30 minutes and was £2.80 per week (95% CI £2.32 to £3.27) for 60 minutes per week, respectively.
Discrete choice modelling
In general, the average amounts required by participants (WTA) for increasing walking or cycling to and from places, moderate-intensity and vigorous-intensity recreational PA were £2.88 per hour (95% CI £2.33 to £3.43), £1.02 per hour (95% CI £0.68 to £1.37), and £3.29 per hour (95% CI £2.72 to £3.86), respectively. In particular, the minimum monetary incentives necessary for increasing PA differed significantly according to whether a participant was inactive or active at baseline. Participants from the inactive group at baseline required a mean of £5.45 per hour (95% CI £4.39 to £6.51) to walk or cycle (e.g. active travel behaviour), in contrast with their counterparts from the moderate-active group (£3.31 per hour, 95% CI £2.32 to £4.29) and active group (£3.19 per hour, 95% CI £1.69 to £4.68). Similarly, inactive participants required £3.24 (95% CI £2.30 to £4.17) and £4.79 (95% CI £3.25 to £6.33) to undertake an additional 60 minutes of moderate and vigorous PA, respectively. These values were significantly higher than those required for the moderate-active group (£0.92, 95% CI £0.15 to £1.68, and £3.51, 95% CI £2.24 to £4.78, respectively) and active group (£1.82, 95% CI £0.73 to £2.90, and £1.84, 95% CI –£0.04 to £3.72, respectively).
Double-hurdle models
The estimated double-hurdle model (n = 372 from the intervention group) in Table 22 shows that none of the sociodemographic variables (e.g. age, sex, education, and marital status, etc.) were significantly associated with any use of the PA monitoring system. However, compared with active participants (baseline pedometer steps more than 10,000 steps per week), moderately active [coefficients (SE); p-value] [–50.453 (SE 25.144); p < 0.05] participants had significantly earlier non-use attrition. Being in the inactive group [–55.126 (SE 32.055); p < 0.10] was only marginally related to non-use attrition. Additionally, older participants [2.783 (SE 1.261); p < 0.05] had a longer duration of usage before attrition than younger participants and those who were married/co-habituating [–49.524 (SE 24.748); p < 0.05] had significantly shorter duration to non-use attrition than single participants.
Determinants | The decision to use PA monitoring system | The duration to non-use attrition | ||
---|---|---|---|---|
Coefficient (SE) | p-value | Coefficient (SE) | p-value | |
Age (years) | –0.005 (0.008) | 0.496 | 2.783 (1.261)* | 0.027 |
Sex (reference group: male) | ||||
Female | 0.035 (0.161) | 0.828 | –32.794 (25.434) | 0.197 |
Income (reference group: ≤ £20,000 per year) | ||||
£20,001 to £39,961 | –0.040 (0.209) | 0.849 | –3.064 (29.773) | 0.918 |
> £39,961 | –0.243 (0.231) | 0.294 | –46.686 (36.591) | 0.202 |
Education attainment (reference group: no higher education) | ||||
Higher education | 0.134 (0.150) | 0.368 | 3.473 (22.568) | 0.878 |
Marital status (reference group: unmarried/not co-habiting) | ||||
Married/co-habiting | 0.054 (0.167) | 0.745 | –49.524 (24.748)* | 0.045 |
Physical activity [reference group: physically active group (baseline physical activity steps ≥ 10,000/day)] | ||||
Moderately active (≥ 5000 and < 10,000 steps per day) | –0.267 (0.168) | 0.113 | –50.453 (25.144)* | 0.045 |
Inactive (< 5000 steps per day) | –0.186 (0.214) | 0.385 | –55.126 (32.055) | 0.085 |
Strata: [reference group: small (< 20)] | ||||
Strata: school | –0.480 (0.322) | 0.137 | –39.580 (67.343) | 0.557 |
Strata: medium (20–50) | –0.039 (0.172) | 0.818 | 52.423 (27.672) | 0.058 |
Strata: large (50+) | 0.028 (0.192) | 0.883 | 30.591 (31.134) | 0.326 |
n | 372 | |||
Log-likelihood | –1575.10 |
The second Cragg’s double-hurdle model in Table 23 shows the influence of sociodemographic characteristics on the decision to increase PA at 6-month follow-up compared with baseline and the amount by which PA increased. Those participants who were moderately active [coefficients (SE); p-value] [0.519 (SE 0.159); p < 0.001] and inactive [1.067 (SE 0.197); p < 0.001] at baseline were more likely to increase their PA at the 6-month follow-up, compared with the participants who had already been active at baseline. Moreover, participants from the organisations with > 50 participants [0.400 (SE 0.164); p < 0.05] were more likely to increase PA than participants in those organisations with a smaller number (< 20) of participants (Table 23).
Determinants | The decision to increase PA at 6-month follow-up compared with baseline | The amount of PA increased | ||
---|---|---|---|---|
Coefficient (SE) | p-value | Coefficient (SE) | p-value | |
Age (years) | –0.004 (0.007) | 0.527 | –0.014 (0.010) | 0.188 |
Sex (reference group: male) | ||||
Female | 0.193 (0.141) | 0.172 | –0.136 (0.228) | 0.553 |
Income (reference group: ≤ £20,000 per year) | ||||
£20,001 to £39,961 | 0.386 (0.179)* | 0.031 | –0.611 (0.300)* | 0.041 |
> £39,961 | 0.417 (0.196)* | 0.034 | –0.629 (0.327) | 0.055 |
Education attainment (reference group: no higher education) | ||||
Higher education | 0.164 (0.133) | 0.215 | 0.590 (0.204)** | 0.004 |
Marital status (reference group: unmarried/not co-habiting) | ||||
Married/co-habiting | 0.195 (0.156) | 0.212 | –0.072 (0.251) | 0.774 |
Physical activity [reference group: physically active group (baseline physical activity steps ≥ 10,000/day)] | ||||
Moderately active (≥ 5000 and < 10,000 steps per day) | 0.519 (0.159)*** | 0.001 | 0.131 (0.283) | 0.644 |
Inactive (< 5000 steps per day) | 1.067 (0.197)*** | 0.000 | 0.296 (0.312) | 0.343 |
Strata: [reference group: small (< 20)] | ||||
Strata: school | 0.287 (0.287) | 0.317 | –0.334 (0.423) | 0.429 |
Strata: medium (20–50) | 0.031 (0.156) | 0.843 | –0.166 (0.244) | 0.497 |
Strata: large (50+) | 0.400 (0.164)* | 0.015 | –0.269 (0.244) | 0.270 |
Intervention group | –0.163 (0.126) | 0.196 | 0.079 (0.189) | 0.674 |
n | 467 | |||
Log-likelihood | –548.74 |
Next, participants from the medium [0.386 (SE 0.179); p < 0.05] and high income [0.417 (SE 0.196); p < 0.05] groups had a significantly higher likelihood of increasing PA at 6 months, in comparison with the participants from the low-income group. Finally, the variables that were significantly related to the amount by which PA increased (among those that for whom increases were observed at 6 months) were higher education [0.590 (SE 0.204); p < 0.01] and medium income [–0.611 (SE 0.300); p < 0.05], suggesting that participants with university education improved more than those without higher education and that participants with medium income improved less than did low-income participants.
Behavioural economic experiments
Present bias, discount rate, risk aversion and loss aversion among the 207 individuals (153 from the intervention group and 54 from the control group) who participated in the behavioural economic experiments were estimated to be 0.995 (95% CI 0.989 to 1.000), 0.18 (95% CI 0.14 to 0.23), 0.50 (95% CI 0.37 to 0.63) and 1.32 (95% CI 0.26 to 4.27), respectively. The estimated discount rate was comparable to the result (0.18) reported in a similar study conducted in Northern Ireland128 but was much higher than the discount rate estimates elicited in similar experimental settings among a general Danish population (0.10). 129 Meanwhile, the present-bias parameter was estimated to be 0.995 and is significantly smaller than 1, indicating that our sample was relatively present-bias, in contrast with the general Danish population, which did not manifest present-bias. Moreover, risk preference was estimated to be 0.50, indicating that our sample was more willing to take risks than the general Danish population (0.65). Finally, the loss aversion estimates were similar to the figure (1.35) reported in West Africa130 and were lower than in Vietnam (2.63). 131
Table 24 shows the results of Cragg’s double-hurdle model that account for the effects of behavioural economic characteristics on the decision to increase PA at the 12-month follow-up compared with the 6-month follow-up, and the amount by which PA increased. None of the four behavioural economic characteristics was associated with the actual increment of PA at the 12-month follow-up compared with the 6-month follow-up. Neither did present-bias nor discount rate play a role in either of the hurdle models. When it comes to the amount of PA increased, however, participants who were more loss-averse [coefficient 1.189 (SE 0.523); p < 0.001] improved their PA significantly more than the less loss-averse participants. Obviously, we caveat these interpretations with due regard to the self-selection of participants into this subsample and cannot make broader inferences concerning the entire set of trial participants at baseline.
Determinants | The decision to increase PA at the 12 month follow-up compared with the 6-month follow-up | The amount of PA increased | ||
---|---|---|---|---|
Coefficient (SE) | p-value | Coefficient (SE) | p-value | |
Age (years) | 0.026 (0.013) | 0.055 | 0.011 (0.014) | 0.436 |
Sex (reference group: male) | ||||
Female | –0.209 (0.253) | 0.409 | 0.268 (0.243) | 0.269 |
Income (reference group: ≤ £20,000 per year) | ||||
£20,001 to £39,961 | –0.258 (0.398) | 0.518 | 0.280 (0.362) | 0.439 |
> £39,961 | –0.407 (0.434) | 0.348 | 0.182 (0.409) | 0.656 |
Education attainment (reference group: no higher education) | ||||
Higher education | –0.073 (0.244) | 0.764 | –0.090 (0.234) | 0.701 |
Marital status (reference group: unmarried/not co-habiting) | ||||
Married/co-habiting | 0.051 (0.278) | 0.854 | 0.750 (0.289)** | 0.009 |
Physical activity [reference group: physically active group (baseline physical activity steps ≥ 10,000/day)] | ||||
Moderately active (≥ 5000 and < 10,000 steps per day) | 0.091 (0.259) | 0.724 | –0.404 (0.243) | 0.097 |
Inactive (< 5000 steps per day) | –0.256 (0.333) | 0.441 | –0.570 (0.331) | 0.085 |
Strata: [reference group: small (< 20)] | ||||
Strata: medium (20–50) | –0.026 (0.261) | 0.920 | 0.496 (0.266) | 0.062 |
Strata: large (50+) | 0.097 (0.293) | 0.742 | –0.083 (0.283) | 0.770 |
Intervention group | –0.522 (0.260) | 0.045 | –0.016 (0.245) | 0.948 |
Behavioural economics concepts | ||||
Present-bias | –31.078 (32.336) | 0.337 | –26.021 (29.126) | 0.372 |
Discounting rate | 0.023 (1.059) | 0.983 | 0.510 (1.067) | 0.633 |
Risk preference | –0.827 (0.692) | 0.232 | 1.131 (0.593) | 0.056 |
Loss aversion | 0.506 (0.573) | 0.378 | 1.189 (0.523)*** | 0.000 |
n | 149 | |||
Log-likelihood | –205.66 |
Table 25 illustrates the associations between PA at 6- and 12-month follow-ups and their possible behavioural economic determinants, respectively. A higher discount rate [–0.662 (0.329); p < 0.05] was associated with a lower PA at the 6-month follow-up. This is in line with previous time preference studies suggesting that people with higher discount rates were less likely to resist the temptation for an immediate gratification which might harm their future health. 101,102 However, discount rate was not associated with the PA level observed at the 12-month follow-up [coefficient –0.073 (SE 0.315); p > 0.10]. The remaining behavioural economic parameters did not play a role in determining PA measured either at the 6- or the 12-month time points.
Determinants | PA at 6-month follow-up | PA at 12 month follow-up | ||
---|---|---|---|---|
Coefficient (SE) | p-value | Coefficient (SE) | p-value | |
Age (years) | 0.004 (0.004) | 0.303 | 0.010 (0.004)* | 0.014 |
Sex (reference group: male) | ||||
Female | –0.038 (0.074) | 0.605 | –0.067 (0.073) | 0.360 |
Income (reference group: ≤ £20,000 per year) | ||||
£20,001 to £39,961 | 0.267 (0.111)* | 0.017 | 0.287 (0.116)* | 0.015 |
> £39,961 | 0.137 (0.124) | 0.270 | 0.169 (0.128) | 0.191 |
Education attainment (reference group: no higher education) | ||||
Higher education | 0.123 (0.074) | 0.095 | 0.105 (0.072) | 0.147 |
Marital status (reference group: unmarried/not co-habiting) | ||||
Married/co-habiting | 0.069 (0.083) | 0.404 | 0.058 (0.082) | 0.481 |
Physical activity [reference group: physically active group (baseline physical activity steps ≥ 10,000/day)] | ||||
Moderately active (≥ 5000 and < 10,000 steps per day) | –0.557 (0.080)*** | 0.000 | –0.465 (0.076)*** | 0.000 |
Inactive (< 5000 steps per day) | –0.792 (0.098)*** | 0.000 | –0.754 (0.099)*** | 0.000 |
Strata: [reference group: small (< 20)] | ||||
Strata: medium (20–50) | –0.054 (0.078) | 0.492 | –0.001 (0.077) | 0.987 |
Strata: large (50+) | 0.093 (0.090) | 0.304 | 0.007 (0.088) | 0.939 |
Intervention group | –0.160 (0.077)* | 0.040 | –0.217 (0.075)** | 0.005 |
Behavioural economics concepts | ||||
Present-bias | –7.287 (9.178) | 0.428 | –12.063 (9.074) | 0.186 |
Discounting rate | –0.662 (0.329)* | 0.046 | –0.073 (0.315) | 0.817 |
Risk preference | –0.108 (0.210) | 0.608 | 0.134 (0.197) | 0.500 |
Loss aversion | –0.293 (0.166) | 0.080 | –0.012 (0.167) | 0.943 |
n | 174 | 157 | ||
R 2 | 0.42 | 0.42 |
Chapter 7 Results from the mediation analyses
The results of the main outcome evaluation show that there was a small but significant decline in the mean number of steps per day at 6 months relative to baseline for the intervention group compared with the control group, and there were no significant differences at 12 months. Several authors have claimed that investigation of causal mechanisms is more valuable when interventions are not successful as information about which components (if any) were successful, and which were not, can inform the design of future studies. 132,133 Therefore, mediation analyses were conducted to explore potential reasons for this result and to determine whether or not there were any intervention components with beneficial effects for PA.
Means and SDs for all putative mediator variable scores are presented in Table 26. Descriptive statistics are presented according to time point (i.e. baseline, 4 weeks or 6 months) and group.
Variables (possible range) | Time point | |||||||||||
---|---|---|---|---|---|---|---|---|---|---|---|---|
Baseline | 4 weeks | 6 months | ||||||||||
Intervention | Control | Intervention | Control | Intervention | Control | |||||||
n | Mean (SD) | n | Mean (SD) | n | Mean (SD) | n | Mean (SD) | n | Mean (SD) | n | Mean (SD) | |
PA self-efficacy (1–5) | 439 | 2.91 (0.97) | 376 | 2.92 (0.94) | 344 | 2.83 (0.89) | 319 | 2.80 (0.92) | ||||
Intentions (1–7) | 435 | 5.38 (1.68) | 375 | 5.37 (1.75) | 343 | 5.42 (1.58) | 321 | 5.09 (1.77) | ||||
Outcome expectations (1–5) | 418 | 3.37 (0.62) | 354 | 3.36 (0.64) | 318 | 3.25 (0.67) | 292 | 3.27 (0.60) | ||||
Financial motivation (1–7) | 439 | 1.71 (1.16) | 376 | 1.79 (1.28) | 345 | 2.12 (1.36) | 320 | 2.06 (1.42) | ||||
Planning (1–4) | 414 | 2.37 (0.69) | 363 | 2.45 (0.69) | 344 | 2.29 (0.70) | 319 | 2.32 (0.75) | 255 | 2.35 (0.74) | 235 | 2.32 (0.71) |
Social norms (1–7) | 414 | 3.87 (1.20) | 357 | 4.04 (1.14) | 346 | 3.93 (1.16) | 317 | 3.78 (1.29) | 253 | 3.90 (1.13) | 235 | 3.90 (1.12) |
Identified regulation (1–5) | 438 | 3.81 (0.87) | 375 | 3.92 (0.83) | 346 | 3.99 (0.78) | 319 | 3.89 (0.83) | 262 | 3.93 (0.82) | 239 | 3.91 (0.87) |
Integrated regulation (1–5) | 439 | 3.12 (1.13) | 373 | 3.27 (1.11) | 344 | 3.37 (1.08) | 319 | 3.24 (1.10) | 258 | 3.41 (1.10) | 238 | 3.31 (1.12) |
Intrinsic motivation (1–5) | 438 | 3.52 (0.99) | 376 | 3.63 (0.97) | 346 | 3.70 (0.88) | 320 | 3.58 (0.94) | 259 | 3.70 (0.91) | 239 | 3.63 (0.97) |
Habit (1–5) | 437 | 2.89 (1.32) | 375 | 3.08 (1.24) | 256 | 3.18 (1.40) | 235 | 2.87 (1.45) | ||||
Workplace norms (1–5) | 439 | 3.20 (0.82) | 377 | 3.20 (0.85) | 260 | 3.19 (0.76) | 237 | 3.14 (0.83) | ||||
Recovery self-efficacy (1–4) | 438 | 2.36 (0.82) | 375 | 2.34 (0.79) | 261 | 2.41 (0.73) | 238 | 2.41 (0.70) | ||||
Maintenance self-efficacy (1–4) | 438 | 2.79 (0.86) | 376 | 2.77 (0.89) | 262 | 2.69 (0.83) | 237 | 2.69 (0.75) | ||||
Outcome satisfaction (1–5) | 404 | 3.85 (0.68) | 352 | 3.87 (0.65) | 257 | 3.87 (0.62) | 233 | 3.80 (0.69) | ||||
WE: attractiveness (4–20) | 438 | 10.83 (2.71) | 377 | 10.37 (3.14) | ||||||||
WE: safety (4–20) | 438 | 10.63 (2.47) | 377 | 10.39 (2.68) | ||||||||
WE: accessibility (3–15) | 439 | 9.27 (2.14) | 377 | 9.14 (2.07) | ||||||||
WE: availability (3–15) | 439 | 10.36 (2.00) | 376 | 10.27 (2.11) | ||||||||
WE (14–70) | 437 | 38.10 (6.22) | 376 | 37.19 (7.07) | ||||||||
WTA30 | 352 | 3.26 (4.70) | 315 | 2.72 (4.03) | ||||||||
WTA60 | 345 | 5.37 (5.99) | 312 | 4.22 (4.67) |
Mediator outcomes
Table 27 shows the results of random-effects regressions for individual mediators, at 4 weeks and 6 months and these are briefly described below. These results should be interpreted with caution as no adjustment has been made for multiple testing, meaning that type I errors may occur.
Mediator | Mediators | |||||
---|---|---|---|---|---|---|
4 week | 6 month | |||||
n | b (SE) | p-value | n | b (SE) | p-value | |
PA self-efficacy | 597 | 0.09 (0.08) | 0.31 | |||
Intentions | 595 | 0.29 (0.13) | 0.02 | |||
Outcome expectations | 528 | –0.03 (0.06) | 0.58 | |||
Financial motivation | 600 | 0.11 (0.15) | 0.46 | |||
Planning | 575 | 0.02 (0.05) | 0.75 | 436 | 0.08 (0.06) | 0.18 |
Social norms | 576 | 0.23 (0.08) | < 0.01 | 434 | 0.10 (0.12) | 0.42 |
Identified regulation | 598 | 0.14 (0.06) | 0.01 | 459 | 0.11 (0.05) | 0.02 |
Integrated regulation | 595 | 0.23 (0.07) | < 0.01 | 454 | 0.26 (0.08) | < 0.01 |
Intrinsic motivation | 599 | 0.18 (0.06) | < 0.01 | 456 | 0.17 (0.06) | < 0.01 |
Habit | 448 | 0.48 (0.12) | < 0.01 | |||
Workplace norms | 456 | 0.13 (0.07) | 0.06 | |||
Recovery self-efficacy | 457 | 0.02 (0.06) | 0.80 | |||
Maintenance self-efficacy | 459 | –0.02 (0.09) | 0.80 | |||
Outcome satisfaction | 427 | 0.03 (0.05) | 0.56 |
Initiation of physical activity
At 4 weeks post baseline, there were significant differences between the intervention and control groups for intentions (b = 0.29, SE = 0.13; p = 0.02), social norms (b = 0.23, SE = 0.08; p< 0.01), identified regulation (b = 0.14, SE = 0.06; p = 0.01), integrated regulation (b = 0.23, SE = 0.07; p < 0.01), and intrinsic motivation (b = 0.18, SE = 0.06; p < 0.01). There were non-significant between-group differences for PA self-efficacy (p = 0.31), outcome expectations (p = 0.58), financial motivation (p = 0.46) and planning (p = 0.75) (see Table 27). Thus, the intervention group participants showed increases above the control group participants in intentions, social norms, identified regulation, integrated regulation and intrinsic motivation between baseline and 4 weeks, approximately equivalent to 0.29, 0.23, 0.14, 0.23 and 0.18 points, respectively, on their original scale. Sensitivity analyses conducted using logistic regressions showed that the significance level changed for some mediators. Specifically, logistic regressions indicated that there was a significant between-group difference for financial motivation [exp(b) = 0.37, SE = 0.15; p = 0.02] and no significant between-group difference for social norms (p = 0.11).
Maintenance of physical activity
At 6 months, there were significant differences between the intervention and control groups for identified regulation (b = 0.11, SE = 0.05; p = 0.02), integrated regulation (b = 0.26, SE = 0.08; p < 0.01), intrinsic motivation (b = 0.17, SE = 0.06; p < 0.01), and habit (b = 0.48, SE = 0.12; p < 0.01). There were non-significant between-group differences for planning (p = 0.18), social norms (p = 0.42), workplace norms (p = 0.06), recovery self-efficacy (p = 0.80), maintenance self-efficacy (p = 0.80), and outcome satisfaction (p = 0.56) (see Table 27). Thus, the intervention group participants showed increases above the control group participants in identified regulation, integrated regulation, intrinsic motivation and habit between baseline and 6 months, approximately equivalent to 0.11, 0.26, 0.17 and 0.48 points, respectively, on their original scale. Sensitivity analyses conducted using logistic regressions showed that the significance level changed for some mediators. Specifically, logistic regressions indicated that there was a significant between-group difference for workplace norms [exp(b) = 0.37, SE = 0.18; p < 0.05].
Single mediator models
Table 28 shows the results of single mediator models based on the product-of-coefficients approach with CIs for the indirect effect formed using the bias-corrected bootstrap, and adjustment for randomisation strata, season, baseline values of the mediator and baseline pedometer steps per day, with SEs and p-values corrected for clustering. An explanatory diagram for single mediator models is presented in Figure 7.
Mediator | 6-month mean number of steps per day | |||||
---|---|---|---|---|---|---|
4-week mediators | 6-month mediators | |||||
n | Indirect effect (SE) | 95% CI | n | Indirect effect (SE) | 95% CI | |
PA self-efficacy | 417 | 32.74 (28.20) | –2.87 to 116.0 | |||
Intentions | 415 | –11.19 (30.18) | –89.87 to 38.52 | |||
Outcome expectations | 363 | 1.84 (14.41) | –16.63 to 49.68 | |||
Financial motivation | 420 | 2.55 (17.92) | –27.61 to 51.77 | |||
Planning | 403 | –3.06 (17.50) | –60.43 to 19.87 | 382 | 50.64 (42.34) | –17.31 to 153.3 |
Social norms | 405 | –33.26 (32.69) | –131.2 to 8.09 | 382 | 22.02 (32.71) | –23.41 to 115.8 |
Identified regulation | 417 | 13.09 (35.48) | –50.45 to 98.09 | 403 | 35.11 (36.90) | –32.39 to 116.9 |
Integrated regulation | 416 | 22.91 (38.42) | –46.88 to 111.4 | 399 | 94.70 (46.27) | 18.69 to 204.4 |
Intrinsic motivation | 418 | 5.99 (34.79) | –62.39 to 81.47 | 400 | 59.00 (36.81) | 3.09 to 154.5 |
Habit | 394 | 198.7 (70.73) | 84.32 to 369.9 | |||
Workplace norms | 400 | –36.10 (32.58) | –129.6 to 5.61 | |||
Recovery self-efficacy | 402 | –2.47 (17.11) | –55.60 to 20.98 | |||
Maintenance self-efficacy | 403 | –3.41 (19.55) | –57.08 to 28.95 | |||
Outcome satisfaction | 376 | 28.04 (29.82) | –11.61 to 112.7 |
FIGURE 7.
Explanatory diagram for single mediator models showing an indirect effect of group assignment on the 6-month mean number of steps per day through a 4-week/6-month mediator.

Initiation of physical activity behaviour
There were no significant indirect effects in any of the 4-week single mediator models (left side of Table 28). SRMR values were close to zero for all models, indicating a good fit,122 and CD values ranged from 0.56 to 0.76.
Maintenance of physical activity behaviour
The right side of Table 28 shows that there were significant, positive indirect effects in single mediator models including the following mediators: integrated regulation (b = 94.70, 95% CI 18.69 to 204.4), intrinsic motivation (b = 59.00, 95% CI 3.09 to 154.5) and habit (b = 198.7, 95% CI 84.32 to 369.9). SRMR values were close to zero for all models, indicating a good fit,122 and CD values ranged from 0.62 to 0.76. Therefore, although the total intervention effect on pedometer steps per day at 6 months was negative, this was partially mitigated by increases in integrated regulation, intrinsic motivation and habit (baseline–6 months), which were positively related to 6 month steps per day, as a result of being assigned to the intervention group. Assignment to the intervention group resulted in increased intrinsic motivation, integrated regulation and habit; as such, participants who experienced an increase of these constructs (baseline–6 months) also experienced less of a decline in PA. Therefore, an increase in such constructs lessened the decline in PA.
Single moderated-mediation models
Single mediator models including 6-month scores of integrated regulation, intrinsic motivation, and habit (with 6-month mean number of steps per day as the dependent variable) were tested for moderation of the a-path and b-path respectively by perceptions of workplace environment availability. Significant a-path moderation was found for the single mediator model with integrated regulation as the mediator (b = –0.10, SE = 0.05; p < 0.05), and the single mediator model with habit as the mediator (b = –0.17, SE = 0.05; p < 0.01). There was a non-significant moderator-by-group interaction coefficient (i.e. a-path moderation) for the single mediator model with intrinsic motivation as the mediator (p = 0.19). There was a non-significant moderator-by-mediator interaction coefficient (b-path moderation) for the single mediator models with integrated regulation (p = 0.36), intrinsic motivation (p = 0.06) and habit (p = 0.44) as the mediator. SRMR values ranged from 0.00 to 0.05, indicating a good fit,122 and CD values ranged from 0.66 to 0.77. Thus the apparent mitigation of the intervention effect, by virtue of higher levels of intrinsic motivation and habit was dependent on participant’s perception of workplace environment availability for PA. It is unclear if those with higher levels of such constructs benefited more with regard to PA because they had more PA opportunities near their workplace or if this was as a result of their perception of such opportunities. This is representative of an ‘intervention challenge’, meaning future intervention studies should give consideration to how to increase levels of integrated regulation and habit for participants with higher perceptions of the availability of PA opportunities in the workplace environment.
Engagement and non-use attrition
Table 29 shows descriptive statistics for 6-month engagement and non-use attrition. Engagement variables that were significant predictors of 6-month mean number of steps per day in univariable analyses were included in a multivariable model, which showed that frequency of hits on the monitoring and feedback component of the website across the 6-month intervention period (b = 50.2, SE = 24.5; p = 0.04). The percentage of earned points redeemed across the 6-month intervention period (b = 9.1, SE = 3.3; p < 0.01) were positively related to the 6-month mean number of steps per day, whereas frequency of hits on the discussion forum component of the website across the 6-month intervention period (b = –69.3, SE = 26.6; p < 0.01) was negatively related to the 6-month mean number of steps per day (Table 30). None of the other variables was significant predictors of the 6-month mean number of steps per day in univariable analyses.
Variables | Six months | |
---|---|---|
n | Mean (SD) | |
Engagement | ||
% intervention days participants walked for at least 10 minutes captured via the PA monitoring systema | 422 | 24.7 (21.8) |
% intervention weeks participants logged onto the websiteb | 418 | 37.8 (32.5) |
% earned points redeemedc | 422 | 39.3 (42.5) |
Frequency: monitoring and feedbackd | 418 | 13.7 (3.5) |
Frequency: rewardsd | 418 | 5.7 (4.5) |
Frequency: mapsd | 418 | 3.4 (4.0) |
Frequency: health information (PA)d | 418 | 0.5 (1.7) |
Frequency: health information (other)d | 418 | 1.2 (3.2) |
Frequency: discussion forumsd | 418 | 1.9 (4.2) |
Total no. sections (website)e | 418 | 3.9 (1.5) |
Total minutes (recording daily activity via PA monitoring system) | 422 | 1000 (987) |
Total minutes (PAL website) | 418 | 1171 (2048) |
Non-use attrition | ||
Days to non-use attrition (recording daily activity via PA monitoring system)f | 422 | 53.7 (61.2) |
Days to non-use attrition (PAL website)g | 418 | 31.7 (43.4) |
Number of participants with non-use attrition for recording daily activity via PA monitoring system (%) | 375 (89%) | |
Number of participants with PAL website non-use attrition (%) | 403 (96%) |
Engagement variables | Model | |||||
---|---|---|---|---|---|---|
Univariable | Multivariable | |||||
n | b (SE) | p-value | n | b (SE) | p-value | |
Engagement indicators | ||||||
% intervention days participants walked for at least 10 minutes captured via the PA monitoring systema | 231 | 4.21 (8.54) | 0.62 | |||
% intervention weeks participants logged onto the websiteb | 234 | 4.39 (6.01) | 0.47 | |||
% earned points redeemedc | 231 | 8.26 (4.07) | 0.04 | 230 | 9.1 (3.3) | < 0.01 |
Website sections | ||||||
Monitoring and feedbackd | 234 | 66 (18) | < 0.01 | 230 | 50.2 (24.5) | 0.04 |
Rewardsd | 234 | 14 (36) | 0.70 | |||
Mapsd | 234 | –47 (44) | 0.28 | |||
Health information: PAd | 234 | 35 (160) | 0.83 | |||
Health information: otherd | 234 | 26 (66) | 0.70 | |||
Discussion forumsd | 234 | –77 (27) | < 0.01 | 230 | –69.3 (26.6) | < 0.01 |
Number of sectionse | 234 | –32 (117) | 0.78 |
The percentage of days (Table 31) that participants were recorded walking for at least 10 minutes captured via the PA monitoring system was a significant predictor of integrated regulation (b = 0.007, SE = 0.002; p < 0.01), intrinsic motivation (b = 0.003, SE = 0.001; p = 0.04), habit (b = 0.007, SE = 0.003; p = 0.01) and outcome satisfaction (b = 0.005, SE = 0.02; p = 0.01). The percentage of weeks that participants logged onto the website at least once was a significant predictor of integrated regulation (b = 0.004, SE 0.002; p = 0.02), intrinsic motivation (b = 0.002, SE 0.0010; p = 0.04), habit (b = 0.004, SE 0.002; p = 0.03) and outcome satisfaction (b = 0.004, SE 0.001; p = 0.01). The percentage of earned points redeemed was a significant predictor of 6-month social norms (b = 0.003, SE 0.001; p = 0.01).
Outcome | % intervention days participants walked for at least 10 minutes captured via the PA monitoring systema | % intervention weeks participants logged onto the websiteb | % earned points redeemedc | ||||||
---|---|---|---|---|---|---|---|---|---|
n | b (SE) | p-value | n | b (SE) | p-value | n | b (SE) | p-value | |
Pedometer steps per day | 231 | 4.21 (8.54) | 0.62 | 234 | 4.39 (6.01) | 0.47 | 231 | 8.26 (4.07) | 0.04 |
Planning | 228 | 0.003 (0.002) | 0.18 | 229 | 0.003 (0.002) | > 0.05 | 228 | 0.0008 (0.001) | 0.56 |
Social norms | 230 | 0.004 (0.003) | 0.19 | 230 | 0.002 (0.003) | 0.36 | 230 | 0.003 (0.001) | 0.01 |
Identified regulation | 241 | 0.002 (0.002) | 0.33 | 243 | 0.002 (0.0008) | 0.06 | 241 | 0.0001 (0.0007) | 0.87 |
Integrated regulation | 238 | 0.007 (0.002) | < 0.01 | 240 | 0.004 (0.002) | 0.02 | 238 | –0.0002 (0.0009) | 0.82 |
Intrinsic motivation | 238 | 0.003 (0.001) | 0.04 | 240 | 0.002 (0.0010) | 0.04 | 238 | 0.0004 (0.001) | 0.72 |
Habit | 233 | 0.007 (0.003) | 0.01 | 235 | 0.004 (0.002) | 0.03 | 233 | –0.00004 (0.002) | 0.98 |
Workplace norms | 239 | –0.0004 (0.002) | 0.83 | 241 | 0.001 (0.002) | 0.55 | 239 | 0.0007 (0.0009) | 0.45 |
Recovery self-efficacy | 241 | 0.004 (0.003) | 0.15 | 243 | 0.002 (0.001) | 0.18 | 241 | 0.0002 (0.0009) | 0.81 |
Maintenance self-efficacy | 242 | 0.002 (0.003) | 0.55 | 244 | 0.002 (0.001) | 0.27 | 242 | –0.0005 (0.0005) | 0.36 |
Outcome satisfaction | 225 | 0.005 (0.002) | 0.01 | 225 | 0.004 (0.001) | 0.01 | 225 | 0.001 (0.0007) | 0.11 |
Frequency of hits (Table 32) on the monitoring and feedback component of the website was a significant predictor of 6-month planning (b = –0.04, SE 0.01; p < 0.01), identified regulation (b = 0.02, SE 0.01; p = 0.04) and integrated regulation (b = 0.03, SE 0.01; p = 0.02). Frequency of hits on PA health information was a significant predictor of integrated regulation (b = 0.11, SE 0.05; p = 0.03). Frequency of hits on discussion forums was a significant predictor of 6-month social norms (b = –0.03, SE 0.01; p = 0.03) and integrated regulation (b = –0.02, SE 0.01; p = 0.03). Number of sections accessed on the website at least once was a significant predictor of identified regulation (b = 0.07, SE 0.02; p < 0.01) and integrated regulation (b = 0.09, SE 0.03; p < 0.01).
Outcome | n | Monitoring and feedbacka | Rewardsa | Mapsa | Health information: PAa | Health information: Othera | Discussion forumsa | Number of sectionsb | |||||||
---|---|---|---|---|---|---|---|---|---|---|---|---|---|---|---|
b (SE) | p-value | b (SE) | p-value | b (SE) | p-value | b (SE) | p-value | b (SE) | p-value | b (SE) | p-value | b (SE) | p-value | ||
Planning | 229 | –0.04 (0.01) | < 0.01 | –0.005 (0.01) | 0.59 | –0.01 (0.01) | 0.55 | –0.05 (0.05) | 0.36 | –0.05 (0.03) | 0.06 | 0.002 (0.01) | 0.80 | 0.03 (0.04) | 0.43 |
Social norms | 230 | 0.01 (0.02) | 0.61 | –0.02 (0.02) | 0.31 | –0.03 (0.02) | 0.09 | –0.05 (0.06) | 0.46 | 0.01 (0.03) | 0.68 | –0.03 (0.01) | 0.03 | 0.04 (0.04) | 0.29 |
Identified regulation | 243 | 0.02 (0.01) | 0.04 | –0.01 (0.01) | 0.61 | 0.005 (0.01) | 0.52 | 0.06 (0.04) | 0.08 | 0.04 (0.04) | 0.33 | 0.01 (0.005) | 0.15 | 0.07 (0.02) | < 0.01 |
Integrated regulation | 240 | 0.03 (0.01) | 0.02 | 0.0005 (0.01) | 0.97 | –0.02 (0.01) | 0.10 | 0.11 (0.05) | 0.03 | 0.06 (0.06) | 0.27 | –0.02 (0.01) | 0.03 | 0.09 (0.03) | < 0.01 |
Intrinsic motivation | 240 | 0.01 (0.01) | 0.29 | –0.01 (0.01) | 0.67 | 0.002 (0.01) | 0.88 | 0.06 (0.05) | 0.23 | 0.05 (0.05) | 0.34 | –0.004 (0.01) | 0.61 | 0.04 (0.03) | 0.12 |
Habit | 235 | –0.0004 (0.02) | 0.99 | –0.01 (0.02) | 0.79 | 0.01 (0.02) | 0.50 | 0.06 (0.06) | 0.36 | –0.06 (0.07) | 0.40 | –0.02 (0.01) | 0.07 | 0.01 (0.04) | 0.73 |
Workplace norms | 241 | 0.005 (0.01) | 0.70 | –0.001 (0.01) | 0.96 | –0.001 (0.01) | 0.95 | 0.06 (0.04) | 0.18 | 0.01 (0.04) | 0.71 | 0.003 (0.01) | 0.72 | 0.03 (0.03) | 0.28 |
Recovery self-efficacy | 243 | –0.01 (0.01) | 0.46 | –0.01 (0.01) | 0.68 | –0.01 (0.01) | 0.11 | 0.05 (0.04) | 0.24 | 0.03 (0.03) | 0.30 | 0.002 (0.01) | 0.80 | 0.03 (0.02) | 0.10 |
Maintenance self-efficacy | 244 | –0.01 (0.01) | 0.53 | –0.01 (0.01) | 0.40 | –0.02 (0.02) | 0.31 | –0.03 (0.07) | 0.65 | –0.02 (0.04) | 0.59 | –0.01 (0.01) | 0.06 | 0.003 (0.04) | 0.94 |
Outcome satisfaction | 225 | –0.01 (0.01) | 0.38 | 0.01 (0.01) | 0.57 | –0.01 (0.01) | 0.44 | –0.04 (0.04) | 0.31 | –0.02 (0.02) | 0.35 | 0.01 (0.01) | 0.11 | 0.05 (0.04) | 0.16 |
The estimated median lifetime usage (i.e. after which 50% of participants stopped use) was 25.5 days for use of the PA monitoring system to record daily activity and 12.5 days for use of the website. Non-use attrition of the PA monitoring system to record daily activity occurred for 89% of participants (n = 375), and website non-use attrition occurred for 96% of participants (n = 403). The results of univariable and multivariable Cox regression analyses examining baseline measures of sociodemographic variables, psychosocial variables, environmental variables and PA as predictors of non-use attrition are presented separately for use of the PA monitoring system to record daily activity and use of the website. The multivariable analysis for use of the PA monitoring system to record daily activity showed that having higher levels of identified regulation at baseline (hazard ratio 0.88, 95% CI 0.81 to 0.97), having higher levels of recovery self-efficacy at baseline (hazard ratio 0.88, 95% CI 0.80 to 0.98), or having a lower perception of the safety of the workplace environment for PA at baseline (hazard ratio 1.06, 95% CI 1.02 to 1.11) reduced the risk of attrition. The multivariable analysis for website use showed that being older (hazard ratio 0.99, 95% CI 0.98 to 1.00), unmarried/not co-habiting (hazard ratio 0.79, 95% CI 0.63 to 1.00), having higher values on the EuroQol (EQ-5D) weighted health index (hazard ratio 0.32, 95% CI 0.12 to 0.86), having higher levels of financial motivation at baseline (hazard ratio 0.93, 95% CI 0.87 to 0.99), having a lower perception of the attractiveness of the workplace environment for PA at baseline (hazard ratio 1.06, 95% CI 1.01 to 1.11), or having a higher perception of the availability of PA opportunities in the workplace environment at baseline (hazard ratio 0.94, 95% CI 0.90 to 0.98) reduced the risk of attrition.
Chapter 8 Discussion and conclusions
Introduction
This chapter first summarises key findings and gives an overview of how the PAL scheme delivery and fidelity may have had an impact on the findings and their generalisability, and then offers a more detailed examination of how the pre-planned moderator and mediator analyses might help us interpret the study.
Key findings
Results from the study demonstrate that assignment to the intervention group resulted in a small but significant decline in the primary outcome (i.e. mean number of steps per day at 6 months) relative to baseline compared with the waiting list control group. This result was observed despite the fact that pedometer steps per day were lower for participants in the waiting list control group compared with the intervention group at baseline. Self-reported minutes per week of workplace PA (measured using the GPAQ) also declined among intervention compared with Control participants, but there was no significant change for total moderate- to vigorous-intensity physical activity (MVPA).
The overall results of this study (i.e. that a workplace scheme incorporating an offer of small financial incentives had a negative impact on PA at 6 months) are in contrast to the findings of earlier systematic reviews. Previous workplace PA interventions have shown promise135–137 but though the literature suggests that financial incentives can promote short-term (i.e. at least to 6 months) increases in PA28,97,138,139 and other healthy behaviours,24,27,139,140 it seems that increases are rarely maintained, or dissipate, after this point. 24,27,28,97,138,140
This was the case, for example, in the TRIPPA study. 44 This study compared the use of activity trackers (i.e. self-monitoring) with or without financial incentives to increase PA in office employees. It found that provision of personal or charitable cash incentives, in addition to an activity tracker, significantly increased PA compared with a control group but not compared with an activity tracker (only) group at 6 months. At 12 months, although activity trackers and charitable donations fostered further PA increases, PA in the personal cash incentive group was not significantly different from that in the control group, and had actually decreased compared with the activity tracker (only) group. Albeit not in line with our own pilot work,1 some of the literature highlights a concern that programmes that offer rewards are attractive only to individuals who are already active, which would place bounds on the potential effectiveness of our own intervention targeted at inactive employees. As rewards-based programmes are unlikely to be a good use of resources if dominated by active employees, a recent national study of full-time employees in Singapore used stated-preference techniques to understand who is more likely to participate in rewards-based PA programmes, quantifying uptake by both sufficiently active and inactive employees. However, the authors found that there was high demand for PA rewards programmes for both active and inactive employees. 141
At 12 months, those in the intervention group still had a lower mean number of steps per day total than those in the control group, but the difference was not significant. The focus groups conducted at 12 months further highlighted that at least some of those who maintained the PA levels that they achieved during the intervention had been previously active before the study commenced. The idea that the PAL scheme would prompt participants to look for opportunities to embed PA as a habit within a typical working day was, to a certain extent, aligned with the findings of our mediation analyses, whereby the intervention group participants showed increases (above control group participants) in identified regulation, integrated regulation, intrinsic motivation and habit between baseline and 6 months. These changes were propelled through to the 12-month follow-up. Although the overall findings around the primary outcome (mean number of steps per day) were negative, and null for self-reported MVPA, the results are more complex and from a public health perspective harder to interpret given the encouraging signals with regard to improved well-being and reduced absenteeism. Although many public health programme evaluations struggle with issues around implementation and ‘dose’ (both of which may have had a bearing on our findings), Tannahill and Kelly142 have discussed some of the complexities that evaluators face when interpreting workplace-based interventions. By their account, workplaces are part of a complex system and contextual factors within such a complex system will have a significant bearing on generalisability.
Moreover, recent work by Nooijen et al. 143 has suggested that those who move into a sedentary occupation, may compensate by exercising more outside the workplace. It might therefore be reasonable to think that some of those participants who became active during the early phase of the PAL scheme subsequently took up other forms of PA (gym membership, sports and evening walks, suggested by some in our qualitative findings) outside working hours, and then later, in compensation, placed less emphasis on workplace-based PA. Although this was not explicitly gauged in our study, as Nooijen et al. 143 concluded, ‘mutual influences on PA of different contexts should be considered.’143 Moreover, a novelty or ‘observation’ effect may have affected the control group more than the intervention group, in that although the intervention group were more continuously aware of their activity, wearing a pedometer may have had a greater impact on the control group who only wore it when steps were being recorded at 6 and 12 months. 144,145
Programme delivery
It is notable that the PAL scheme received generally positive comments from participants, employers and retailers. However, a number of key issues emerged from the qualitative process evaluation.
There was a consensus among participants and senior managers that the employer has a role to play in ensuring that their employees have access to opportunities and support for being more active within the work environment. During the study, a range of programmes were concurrently delivered within the trial workplaces, over which we had no discretion, including health promotion talks and exercise classes, as well as other ongoing initiatives, such as cycle to work schemes. Both the control and intervention groups would have had variable exposure to these parallel programmes. However, only six employers outlined having policies in place in relation to staff health and well-being. Horodyska et al. 146 have compiled a checklist for developing practice and reporting research on workplace interventions and policies and a key aspect for success relates to integration and institutionalisation of well-being programs. Although many of the participating organisations in the study had ongoing workplace health promotion schemes, there were some which did not and the PAL scheme was seen as a significant opportunity for PA. Our qualitative work also highlighted how participants would have liked the PAL scheme to continue, stating that it would have had organisational support.
Given the many barriers to being active during the working day reported by our participants, it is not clear how effective existing schemes and policies within the workplace are, or how they are being implemented. This evaluative work highlighted the need for a ‘top-down’ approach, for employees to feel they were supported by management in being more active within the workplace.
A number of issues were identified in relation to the day-to-day running of the scheme. There were occasional technical issues with the sensors and fobs that had a knock on effect on points accumulated. In addition, participants felt the scheme was restrictive in terms of the location of the sensors and the daily time limits surrounding the scheme.
A strong theme to emerge from the focus group discussions related to the feedback on PA that the intervention provided to participants, with many noting that their main motivation was to complete their personal daily PA goals. However, many participants felt the rewards element of the scheme needed to be improved, with a greater choice of vouchers (of higher value) needed if participants were to feel the effort was worthwhile. Furthermore, < 40% of participants redeemed their points for vouchers which may suggest that the vouchers were not a suitable incentive for a proportion of participants. However, the types of vouchers available were based on those which were most popular in our pilot trial (same target population) and verified in pre-intervention focus groups with the target population.
Limitations
With sparse literature on PA workplace-based behaviour change interventions, the PAL scheme is one of the few studies which has used a cluster RCT design and has assessed the longer-term effects of the intervention on objectively measured PA up to 12 months post baseline.
Nevertheless, we faced some important limitations. As recruitment rates were lower than anticipated, there was a delay in commencing the intervention as well as a need for additional recruitment sites. The overall levels of retention were lower than anticipated but not too different from some other recent work-place based studies. For example van Dongen et al. 147 managed to record outcome data in 56% of trial participants at 12 months (n = 232) while Puig-Ribera et al. 148 managed to obtain outcome data on 67% of their subjects at 19 weeks (n = 178). The loss to follow-up and attrition in our study were, in part, a side effect of current austerity related re-structuring of the local authorities and within the civil service which was ongoing throughout the trial. This resulted in upheaval or uncertainty about job location and security for some participants.
There were clearly some technical issues affecting intervention fidelity. Some of the sensors developed battery problems which affected the recording and accuracy of the PA behaviour captured, and required replacement. Recruitment of our intended sample size is also a limitation of this work. Similarly, previously our pilot work did not have a true-control group, nor did it use the same technological design for the PA monitoring system or the same sensors. 1 Thus, while pilot work was informative and provided promise, such methodological differences and the possible chance finding due to the underpowered nature of a pilot study may partially account for discrepancies in expectation and reality.
Generalisability
The study design, a cluster RCT, was robust and following the amendment of the target sample size, should have been sufficiently powered (90% power at the 5% significance level) for an effect size of d = 40. It is difficult to directly compare the profile of our study participants with those in other studies but the mean number of steps per day in our control group at baseline is not dissimilar to values reported for other office workers. 149 Clearly the variation in step counts among such staff, even in UK settings, will be driven by a complex mixture of social, environmental and organisational factors.
Interpretation and implications of the health economics findings
The results showed that the intervention was not likely to be cost-effective for employees working in office settings, from the NHS and PSSRU’s perspectives over the 6-month time horizon. The intervention group consumed fewer health-care resources than the control group, but the difference (£57.56 per person for the 6-month trial period, see Table 15, ‘MI: adjusted’ model) was not statistically significant after adjusting for covariates. This indicates the large variations between individuals’ health-care resource use and uncertainty surrounding this variable. There were no statistically significant differences in the utility values between the intervention group and control group at both baseline and 6-month follow-up, leading to no difference in QALY gained between the groups. Despite the close to zero mean QALY gained, the probability that the intervention might be cost-effective was 34%, indicating uncertainty in the estimation. This uncertainty was visualised in the cost-effectiveness plane, which showed the bootstrapped pairs covered all of the four quadrants.
Uncertainty in the ICER estimates results from both the uncertainty around the incremental cost and, primarily, the incremental QALY. There is a small chance (13.4%) that the intervention is a cost-saving strategy from the public sector perspective. This is because of the combination effect of small intervention costs and the possible reduction in resource use by the intervention. The CEAC shows that the probability that the intervention might be cost-effective is almost constant with the increase of the willingness-to-pay threshold, suggesting that the uncertainty is mainly surrounding the QALY gained. The ICER had a very large magnitude with a negative sign, which can be attributed to the close to zero negative mean QALY gained in the intervention group compared with the control group. This also leads to a wide CI for the estimate of ICER as a result of the relatively large variation of the QALY gained compared with its mean estimate.
Sensitivity analysis results
The sensitivity analysis showed that when an alternative business model was used or the cost of sensors was reduced, the incremental cost of the intervention became negative. The probability of the intervention being cost-effective increased to around 60%, but this may not be considered as a dominant scenario given the ‘benefit’ of the intervention on utility was still negative and unclear.
A potential ceiling effect of EQ-5D-5L was observed in this population, with > 40% and > 30% of participants responding ‘full health’ at baseline and 6 months, respectively. This may limit the sensitivity of this instrument for assessing the potential benefit of interventions such as this in public health settings, as there is little room for utility values to get higher in a generally healthy population.
Cost–benefit analysis/net cost model results
Adopting an ‘employee’ perspective, there was a net cost saving for the employers due to reduced absenteeism in the intervention group. This net cost saving ranged from £66 to £735 depending on the wage rate employed. By exploring the uncertainty surrounding this net cost saving estimate, we determined that the probability that the intervention is cost saving at current intervention cost (£55.68) is highest (= 64%) in the high-salary group and lowest (= 57%) in the low-salary group. If the intervention cost reduces in the future, with the aforementioned alternative business model or upgraded technology for the sensors, the probability to be cost saving will increase and be capped at 65.4% (the intercept of the three lines with y-axis in Figure 6). This capped value is owing to the great uncertainty (p = 0.62) surrounding the benefit in terms of the difference in absolute absenteeism rates between the groups.
A number of issues arising from the study merit further research from a health economic perspective. Although we used utilities directly elicited from participants using the EQ-5D, we observed small positive benefits in terms of well-being scores in the intervention group, which were at odds with the negative impact on the primary outcome. Studies with longer-term follow-up could usefully explore further how to aggregate or reconcile the various health and productivity effects of PA programmes in workplace settings, especially because, in the longer term, they may have an impact on the individual and the employer in different ways. To date, there have been few attempts to model the impact of workplace wellness programmes that are based on financial incentives. Basu and Kiernan150 constructed a novel modelling framework that identified how to optimise the marginal return on investment from workplace programmes that were based on incentivizing behaviour change (including those targeting PA). By integrating ‘demand curves’ that captured individual differences in response to any given incentive level with employee demographic and risk factor data (from the USA) they demonstrated that an employer (with ≈3000 employees) would see optimal return on investment by offering incentives of US$367 per year (i.e. somewhat larger than in the PAL scheme) but that this return on investment (ROI) was very sensitive to self-selection (if already active participants enrolled). Even with the optimally designed programmes, the actual degree of behaviour change varied widely, with most participants still undertaking low to moderate levels of PA despite the incentive, and > 70% of participants not engaging in PA on a weekly basis (in other words, they would be intermittent participants). Thus, it is right that future research in this area take a broad perspective and attempts to integrate individual and employer perspectives.
Interpretation and implications of the behavioural economics analyses
It was shown that participants were generally prepared, prior to the intervention, to receive financial incentives to increase their PA, which accords with the findings of Giles et al. ,27 who also observed that the use of financial incentives was acceptable to the public. Furthermore, we found that high-value incentives were preferred over low-value incentives, which is consistent with findings reported in Farooqui et al. 151 based on a sample of 802 Singaporean adults. However, the opposite trend was reported when UK respondents were asked to decide on the provision of financial incentives from public funds. 27 Next, the stated incentive levels in our study (£2.80 per hour) are small but are comparable to the levels used (although not empirically justified) in some other PA promotion schemes (US$4.27 per hour152) and in a weight loss programme (US$3.75/week). 153 Although our trial reported negative effects on PA, having utilised DCE results to determine the level of the rewards, we suggest that it is still useful to incorporate the stated preference method into the design of similar schemes, as argued by Tinelli et al. 154 to better understand respondents’ preferences for incentives and to increase their chances of demonstrable effectiveness and cost-effectiveness.
Physically inactive participants, especially those who were highly inactive (daily steps < 5000) at baseline, were more susceptible to early non-use attrition. Moreover, physically inactive participants increased PA less at the 12-month follow-up than the physically active participants. Because of these trends, physically inactive participants still had lower PA at both the 6- and the 12-month follow-up than those who had already been active at baseline. This phenomenon can be (partly) explained by our analysis indicating that inactive participants would require substantially higher financial incentives to increase their PA. It may also be the case that they had relatively higher discount rates, which were significantly and negatively associated with PA. Future PA interventions should target especially the highly inactive population who were revealed to be the most difficult to be incentivised for behavioural change.
Our sample manifested relatively high discount rates, impling that incentivisation of our sample was rather difficult and our sample was present-biased implying that participants with high discount rates are more likely to pursue immediate gratification rather than execute their salutary intentions for their future selves. As these behavioural economic experiments were undertaken among participants who remained in the study at the 6-month follow-up, incentivisation might have been even more difficult among the drop-outs because of inter alia even higher levels of discount rates. Next, although discount rates were not associated with behavioural change (i.e. the actual increment of PA at the 12-month follow-up compared with the 6-month follow-up), higher discount rates did result in significantly lower PA at the 6-month follow-up. Consequently, reducing a participant’s discount rate might potentially promote the participant’s PA. Recent studies suggest that discount rates can be altered or modulated by therapeutic cognitive, behavioural, or structural environmental manipulation. 155
Higher loss aversion was associated with a larger PA increase at the 12-month follow-up than at the 6-month follow-up. This reconciles with the idea of ‘deposit contracts’ asking participants to deposit forfeitable money which can only be redeemed if a certain behavioural change target is met. It was already reported that smoking cessation programs were more effective in deposit-based programs than reward-based programmes. 156 As a result, utilising the concept of loss aversion could be a useful part of interventions to promote PA.
Interpretation and implications of the mediation and moderation analyses
The main results of the trial were negative at 6 months and null for the primary outcome at 12 months and in order to explore potential reasons for these results, we carried out a range of mediation and moderation analyses as specified in our protocol.
Out of 19 tests of intervention effects on mediators (i.e. tests of the significance of the relationship between group assignment and the mediator), nine were significant (n = 9/19; 47%). Of 19 formal tests for indirect effects (i.e. the multiplication of the coefficient of the path relating group assignment to the mediator and the coefficient of the path relating the mediator to PA), three were significant (n = 3/19; 16%). However, it should be noted that p-values have not been adjusted for multiple testing. This finding suggests that although the intervention was effective in changing some mediating constructs targeted by the intervention, mediator changes were generally not translated to changes in PA behaviour. For constructs showing significant mediating effects on PA behaviour, indirect effects were in the opposite direction to the overall intervention effect on PA. Specifically, although the total effect of assignment to the intervention group (vs. control group) on PA behaviour at 6 months was negative, indirect effects through significant mediators were in the positive direction. Therefore, the intervention’s negative effect on PA at 6 months may have been mitigated by modest PA increases achieved through increases in some of our measured mediators. However, it is apparent that the results of these tests of the behaviour change theories on which the intervention was designed were not sufficient to explain the overall change in PA behaviour at 6 months.
In summary, none of the putative mediators of initiation (measured at baseline and 4 weeks) significantly mediated the relationship between group assignment and PA behaviour change at 6 months. However, there were significant increases for intervention group participants compared with control group participants in intentions, social norms, identified regulation, integrated regulation and intrinsic motivation between baseline and 4 weeks. Therefore, it is apparent that although assignment to the intervention group led to increases in some putative mediators of initiation, these increases were not related to PA behaviour change at 6 months. Two potential reasons are as follows: (1) changes in these mediators of initiation do not induce change in positive PA behaviour and (2) changes in mediators of initiation are not carried through to PA behaviour change at 6 months (i.e. perhaps PA behaviour change caused by changes in these mediators occurred at an earlier time point).
Several of the mediators of maintenance (measured at baseline and 6 months) significantly mediated the relationship between group assignment and PA behaviour change at 6 months (i.e. integrated regulation, intrinsic motivation and habit) in the positive direction (i.e. there were increases in these constructs between baseline and 6 months for intervention participants compared with control participants and changes in these mediators were positively related to changes in PA behaviour). There were also significant increases for intervention group participants compared with control group participants in identified regulation, integrated regulation, intrinsic motivation and habit between baseline and 6 months. This was notwithstanding the overall negative intervention effect, suggesting that participants who were able to increase their levels of these constructs (e.g. by focusing on the scheme’s self-regulation, social or environmental aspects rather than the financial incentives or scheme limitations) experienced less of a decline in PA. There is support in the theoretical and empirical literature to suggest that integrated regulation, intrinsic motivation and habit are important mediators of PA change and for maintenance of behaviour change. 157–164 However, results of the mediation analyses suggest that the behavioural changes observed in the present study were not achieved by means of the mechanisms posited by the behaviour change theories on which the intervention was designed. Therefore, these behaviour change theories were not sufficient to explain the overall change in PA behaviour. Finally, the fact that changes in mediators of initiation were not translated to changes in PA behaviour while changes in some mediators of maintenance were related to changes in PA behaviour implies that participants were in the behavioural maintenance phase at 6 months. This is in line with the definition of behaviour change maintenance adopted in a recent systematic review investigating maintenance in PA interventions targeting young and middle-aged adults. 165
These considerations highlight what has been emphasised by Threlfall et al. ,166 that interpretation of intervention effects (whether positive, negative or null) is rarely a-theoretic. In the sections that follow, we offer some theoretical contextualisation of these moderation and mediation findings.
Perception of the availability of physical activity opportunities in the workplace environment
Perception of availability of PA opportunities in the workplace environment was a significant moderator of the relationship between group assignment and PA change between baseline and 6 months. Specifically, higher levels of perceived availability of PA in the workplace environment were associated with a greater decrease in PA between baseline and 6 months for an intervention group participant than for a control participant. Perceptions of workplace environment safety was a significant moderator of the relationship between group assignment and PA change between baseline and 12 months, with lower levels of perceived safety associated with a greater decrease in PA between baseline and 12 months for an intervention group participant compared with a control group participant. Previous studies have also supported the idea that perceptions of the environment are important moderators of PA behaviour change. 167,168 However, the direction of the moderating effect of perceptions of availability of PA in the workplace environment on PA at 6 months may appear counterintuitive (i.e. the intervention’s negative effect on PA was stronger when perceptions of availability were high). Perhaps participants who perceived that there were plentiful opportunities for PA around their workplace felt less reliant on the intervention to identify opportunities for PA or were less willing to confine their PA within the limited geographical range of the study’s sensors. This result merits exploration in future research.
Participant discount rate
Participant discount rate was a significant moderator of the relationship between group assignment and PA change between baseline and 12 months, with higher (vs. lower) discount rates associated with a greater decrease in PA between baseline and 12 months for an intervention group participant compared with a control participant. Thus, when participants were more impatient (i.e. preferred an immediate reward with a slightly lower monetary value to a reward with a short delay and a slightly higher monetary value) there was a greater decrease in PA for intervention group participants compared with control participants. This pattern of results may make intuitive sense for the PA outcomes at 12 months (if, for a subgroup at least, increases in intrinsic motivation were not sufficient to compensate over the longer follow-up period when the extrinsic rewards had been removed after the 6-month intervention) but cannot explain the decline in PA at 6 months (the primary outcome).
Financial incentive and motivation
Self-determination theory (SDT) posits that increasing intrinsic motivation (i.e. based on an individual’s own values) leads to more sustainable behaviour change than can be achieved by inducing extrinsic motivation. 169 The finding of increases in intrinsic forms of motivation (i.e. identified regulation, integrated regulation and intrinsic motivation) for intervention group participants compared with control group participants at 4 weeks and 6 months, and no intervention effect for financial (extrinsic) motivation at 4 weeks, has important implications for some of the main contentious issues highlighted in the literature on the use of financial incentives for achieving behaviour change. First, extrinsic (e.g. financial) rewards are not thought to produce long-term behavioural changes because withdrawal of the reward causes the behaviour to be extinguished. 170 Second, the use of financial incentives has been thought to have a ‘crowding out’ effect on intrinsic motivation for behaviours that are already internalised. 169 For example, the qualitative data from our study showed that some participants, who were already physically active, reported that they joined the scheme to receive rewards for behaviour that they already engaged in. Based on this argument, we may have expected to observe decreases in intrinsic motivation for intervention group participants versus control group participants. Conversely, the review conducted by Promberger and Marteau169 suggested that there was no current evidence to support the notion that extrinsic rewards or incentives undermine any intrinsic motivation in relation to health-related behaviours. In line with the findings of that review, our results suggest that using financial incentives in a behaviour change intervention does not necessarily diminish intrinsic motivation and can actually increase it if rewards are delivered as part of a complex behaviour change intervention with multiple components. 32
The consensus is that financial incentives may be useful in acting as a ‘kick-start’ to PA but are less useful for promoting long-term behaviour change. The qualitative work in our study would attest to this, with some participants commenting on the positive aspects of getting people out walking, and to do a little extra every day. Under Learning Theory, previous behaviour is predictive of subsequent behaviour. 46,171 This may be explained by the persistence of factors (e.g. thoughts or rewards) that cause the behaviour to be repeated, or by the formation and strengthening of habits (i.e. learned behavioural responses to certain stimuli and repetition in similar contexts). 162 Thorndike’s Law of Exercise states that the strength of the connection between a behavioural response and a given situation is directly related to the number of times the connection has occurred previously and to the potency and duration of those connections. 172 By the use of contingent reinforcement it was hypothesised that repetition of the behaviour-reward cycle would lead to workplace PA becoming a ‘learned’ behaviour. According to SDT, external regulation occurs when the individual engages in the behaviour to gain reward (e.g. financial incentives) or to avoid punishment by others. 173 However, the reward offered must be deemed worthwhile by them personally. For example, previous studies have found that monetary value138 or type (e.g. individual versus group based)174 of the reward can impact its effectiveness for behaviour change. There was no overall effect of group assignment on changes in financial motivation for participants in this study and focus group interviews indicated that, for some participants at least, vouchers failed to live up to expectations (e.g. insufficient monetary value, lacking in variety, undesirable goods or services, inconvenient expiration dates, or that desirable vouchers ‘ran out’). Therefore, the incentive may not have been attractive enough to entice participants to start (and by repetition, to ‘learn’) PA behaviour in the first place. This is despite the fact that these aspects of the study were designed using ‘best practice’ of pre-intervention development focus groups with participants to discuss all important features of the intervention (e.g. types of rewards, appropriate level of rewards, and placement of sensors).
Intrinsic motivation (integrated regulation and intrinsic motivation) and physical activity
The results of mediation analyses suggested that intrinsic forms of motivation (i.e. integrated regulation and intrinsic motivation) were important mediators of the relationship between group assignment and PA behaviour change at 6 months. SDT, for example, suggests that individuals experience greater vitality, self-motivation and well-being when the basic psychological needs for autonomy, competence and relatedness to others are fulfilled within the social context. 175 Focus groups highlighted that participants wanted more options with respect to rewards, and how and when they engaged in PA. Perhaps participants did not perceive a high degree of autonomy support (i.e. opportunities for choice with minimal use of pressure or demands)176 in the scheme to fully allow internalisation of PA behaviour.
Habit formation and physical activity
Mediation analyses indicated that the strongest indirect effects of group assignment on 6-month PA occurred through habit formation. The emphasis placed on the intervention’s self-regulation techniques supports the observation that people develop habits after a period of successful self-regulation. The process of habit formation incorporates an exchange between automatic and self-regulatory processes within the context of a range of external and internal stimuli. 164 Habits are formed when the behaviour becomes regular, automatic and ingrained in the self-concept. 17,159 Prompts in the social (e.g. e-mail reminders sent by the study team and having work colleagues participating in the intervention) and physical (e.g. seeing sensors surrounding the workplace) environments could have aided habit formation. Moreover, focus groups at 12 months post baseline sought to establish if participants were still engaging with PA and, if so, for what reason. The underlying rationale was to establish if behaviour change maintenance had occurred. Many of those who participated in the focus group discussion at 12 months commented about how they had continued to incorporate PA into their working day, from utilising the walking routes provided by the PAL scheme, to walking to meetings in short proximity rather than driving and generally looking for new ways to fit PA into their day. This is also in line with several habit theories,177–179 which hypothesise that environmental cues associated with prior and new behaviours determine whether or not the new behaviour is maintained. Moderation analyses also indicated that participants’ perceptions of the environment had an impact on the effectiveness of the intervention in line with theory that suggests that supportive social and physical environments facilitate behaviour change maintenance by lowering the opportunity cost of the behaviour. 164 When participants utilised the scheme’s self-regulatory, social and environmental aspects to increase their PA habits, this seemed to mitigate the overall negative impact on PA.
Discount rates and intervention effectiveness
Moderation analyses also indicated that participants’ derived discount rates had an impact on the ‘effectiveness’ of the intervention, with higher discount rates (i.e. higher levels of impatience) associated with greater decreases in the primary outcome for intervention group participants. This is line with the findings of the study’s additional behavioural economics analyses (see Key findings) and also with the qualitative work from which we noted how vexed some participants were on not achieving enough points to redeem a certain voucher, or that a priority voucher had been time expired (and withdrawn from offer) before they had collected enough points and so they subsequently ‘gave up’. Although we cannot infer causality, these associations suggest that when designing financial incentive based interventions, future studies should consider using information on participants’ discount functions to tailor incentive structures (e.g. timing, contingency and value) in order to achieve behavioural initiation and maintenance. 180 Other intervention strategies (e.g. goal-setting,181 provision of health information182) could also be usefully targeted depending on information available on individual participants’ time preferences.
The concept of adaptive versus static goals has also been explored in recent PA literature, whereby Zhou et al. 183 employed automated machine learning-based personalised daily step goals delivered via a mobile phone app. Their study sought to differentiate PA behaviours in those who received personalised daily step goals compared with a control group who had a constant step goal of 10,000 steps. Although the PAL scheme incorporated personalisation via the website where users could track and monitor progress, a more advanced method for deriving adaptive goals might be beneficial for PA. Adams et al. ,184 for example, also found that dynamic adaptive goal-setting may outperform static approaches for goal-setting for PA (steps), with slower decreases in PA steps from baseline to intervention end. The use of adaptive goals may be particularly useful in a workplace setting. Where some employees may be able to incorporate PA into their commute to work, others – as we identified from the qualitative work – may find the workplace to be their only opportunity to be physically active. Recent research on goals does not generally account for the potential time discounting confound. ‘Nearer’ outcomes are more motivating, and are proposed to explain differences in motivation over time in human behaviour as well. 185 One interpretation is that more temporally distant rewards are less motivating specifically because delayed rewards are discounted (and potentially also riskier). In this view, goal-gradient behaviour (exerting more effort for sooner rewards, depending on how far away you are from your goal) is another source of evidence for time discounting. Urminsky et al. 186 reported discount rates that were lower when separately accounting for goal gradient effects. With this in mind, the effects of adaptive goals, attendant on differing feedback schedules, could potentially be more or less motivating in people with different discount rates and may cater for patterns of behaviour resulting from changes in day-to-day circumstances. This could usefully be the subject of future research.
Physical activity self–efficacy
Although constructs such as PA self-efficacy are frequently shown to be important mediators or predictors of PA behaviour187–192 PA self-efficacy and phase-specific self-efficacy (i.e. maintenance and recovery self-efficacy) were not shown to be significant mediators in the present analysis. There is emerging literature disputing the causal role of self-efficacy in behaviour change193 and several systematic reviews show that the supporting evidence is lukewarm at best. Rhodes and Pfaeffli194 found limited evidence for self-efficacy and outcome expectations, but stronger support for self-regulatory processes and social support as mediators of behaviour change (focusing on initiation) in PA intervention studies targeting adult non-clinical populations. Notably, although self-regulation constructs have not been examined as putative mediators in this study, the qualitative analysis revealed that participants appreciated the self-regulation (e.g. self-monitoring, planning, goal-setting) and social aspects of the PAL scheme. Another review conducted by Teixeira et al. 158 revealed that PA self-efficacy was related to short-term PA outcomes (i.e. at < 12 months) in 10 out of 15 tests (67%), while outcome expectations were not related to PA outcomes in one test. Importantly, these results did not rely on formal mediation tests, highlighting the need for robust mediation analysis in PA intervention studies.
Engagement and non-use attrition
The results of GLS regressions showed that engagement in specific components of the intervention (i.e. the frequency of using the website self-monitoring and feedback component, the percentage of earned points redeemed, and the frequency of using website discussion forums) was significantly related to PA at 6 months. These findings suggest that when participants focused more on the intervention’s positive aspects (e.g. self-monitoring), this mitigated the intervention’s overall negative impact on PA behaviour. Previous research also shows that self-regulation techniques and self-monitoring are useful strategies for changing PA behaviour. 2,38,194–198 Redeeming a higher proportion of earned points for rewards was also associated with slightly higher PA levels at 6 months. Therefore, it appears that when participants found the financial incentives desirable and redeemed their accumulated points, this also mitigated the overall negative impact of the scheme on PA behaviour. Previous studies have also shown that in order for financial incentives to be successful in inducing behaviour change, the reward on offer must be deemed to be valuable to the individual participant. For example, monetary value138 or type (e.g. individual vs. group based)174 of reward can have an impact on effectiveness for behaviour change, and this was alluded to in feedback from focus groups. Examination of the relationship between engagement in different aspects of the intervention and PA at 6 months showed that a higher frequency of accessing the discussion forum component of the website was associated with a significant decline in PA at 6 months. Discussion forums were included on the PAL scheme website as a means of providing social support for behaviour change. For example, it was expected that participants would use these forums to contact researchers and interact with other participants to support behaviour change. However, this component of the scheme was primarily used by participants as a vehicle to report technical issues and queries, rather than as a means of engaging with other users in a support network. Therefore this finding may indicate that participant frustration with some of the intervention’s perceived limitations contributed to the overall negative impact on PA. One previous study that also found negative intervention effects on PA behaviour concluded that reduced support for the intervention over time was a contributing factor. 199
Intervention fidelity
The literature highlights the importance of intervention fidelity and of ensuring that variations from the original design can be assessed since non-significant findings may be attributable to intervention delivery rather than intervention design. 200 Carroll et al. ’s theoretical framework provides the means of measuring intervention fidelity and understanding its role in the process of intervention implementation. 201 For example, the authors highlight that several potential moderators of the fidelity with which an intervention is implemented include intervention complexity, use of facilitation strategies, quality of intervention delivery and participant responsiveness. Future intervention studies could potentially improve intervention outcomes by improving intervention fidelity with reference to such theoretical frameworks. Finally, systematic reviews also show that the effectiveness of web-based interventions may be enhanced by including various modes of intervention delivery (e.g. text messages, e-mail),201,202 which the PAL scheme did (e.g. through the use of e-mail prompts). However, we have been unable to include data on whether or not participants received and read these e-mails in our engagement analysis. Future work may seek to delve into further analyses around the delivery, open and click rate of e-mails relating to an intervention.
The GLS regressions also showed that use of particular intervention components was associated with increases in some mediators between baseline and 6 months for Intervention Group participants. A higher frequency of using the PAL scheme PA monitoring system (i.e. behavioural practice) and a higher frequency of accessing the study website was associated with higher levels of integrated regulation, intrinsic motivation and habit between baseline and 6 months (i.e. the constructs for which mediation analyses showed significant positive indirect effects). This suggests that a higher level of overall engagement with the intervention (as opposed to engagement with specific intervention components) was related to higher levels of these constructs for intervention group participants. Accessing a higher number of intervention components on the website at least once was associated with increases in identified regulation and integrated regulation, although there was no association with PA levels. Previous work has also identified that overall intervention engagement, and also greater use of intervention components was associated with increases in the primary outcome. 202,203
Further evidence for the importance of the intervention’s self-regulation component was provided by the results of the study’s engagement analysis (i.e. a higher frequency of accessing the website’s feedback and monitoring component was also associated with increases in integrated regulation and intrinsic motivation) which broadly support the findings of the mediation and qualitative analyses. Previous systematic reviews of web-based interventions also highlight web-based self-monitoring as a potentially effective technique. 203 In comparison, engagement with the financial incentive component of the intervention (i.e. redeeming a higher proportion of earned incentives for rewards or a higher frequency of accessing the website’s rewards component) was not found to be related to levels of identified regulation, integrated regulation and intrinsic motivation. Thus, we have further supporting evidence to conclude that the use of financial rewards does not necessarily ‘crowd out’ or diminish intrinsic motivation when delivered as part of a complex multicomponent behaviour change intervention.
Scheme non-use attrition
High levels of non-use attrition in the present study may, to some, reflect dissatisfaction with the intervention’s perceived limitations contributing to the decline in PA outcomes at follow-up. It should also be noted that the definition of non-use attrition that we employed (i.e. occurring at the time of the first 2-week lapse from intervention use) in the present study may be viewed as somewhat arbitrary. Although several studies of web-based PA interventions have adopted this definition, it may be less applicable to the analysis of non-use attrition in workplace interventions for which a 2-week lapse from intervention use may occur for many different reasons (e.g. if a participant is on annual leave, out of the office on work-related travel, or is absent from work for ≥ 2 weeks through illness). Time to non-use attrition for use of the PA monitoring system and use of the website was shown to be significantly predicted by some of the baseline sociodemographic, psychosocial and environmental variables (e.g. age, marital status, health status, financial motivation, identified regulation, recovery self-efficacy and perceptions of the workplace environment) in multivariable Cox regression models. These results are consistent with findings in previous intervention studies, demonstrating that older participants (vs. younger),117,204–208 unmarried participants (vs. married/co-habiting)209 and participants with higher reported health status at baseline (vs. lower health status)210 have decreased risk for non-usage. Individuals with higher levels of financial motivation may have been encouraged to continue participation in the intervention over time in order to continue benefiting from financial rewards. Existing theory suggests that identified regulation and recovery self-efficacy are constructs which are important for long-term behavioural maintenance. 175,176,210–214 For example, it makes sense that individuals with higher recovery self-efficacy should experience a longer period of consistent intervention use before encountering their first 2-week break as they are quicker to recover from a lapse. Notably, the results of our behavioural economics analysis (see Chapter 6, Results from the behavioural economic analysis) indicated that individuals who were more present-biased (i.e. who had less self-control) were also at higher risk for stopping intervention use. Furthermore, some subgroups of participants who were found to be less present-biased in the behavioural economics analysis were also found to be at reduced risk for non-use attrition in survival analyses (e.g. older participants, single participants). This may point to possible interactions between age or marital status and levels of present-bias.
Mediation and moderation analyses: implications for future research
Future PA intervention studies should make use of self-regulation techniques (e.g. self-monitoring, goal-setting), with social and environmental prompts. This may promote habit formation as the new PA behaviour, originally carried out consciously, becomes more automatic. This should also allow for the internalisation of the behaviour, enabling it to be more sustainable long-term. Monitoring of behavioural outcomes is an additional self-regulation technique which should be explored, as it can potentially encourage participants to focus on their satisfaction with more internal outcomes of behaviour change (and, therefore, to maintain it long-term). As Bouton aptly summarised, ‘successful learning of a new behaviour does not permanently replace an earlier one’. 215 Moreover, behaviour change context is also key to maintained change and as such, new behaviours (in the case of the current work, increased PA) may be easily disrupted. Although the PAL scheme utilised cues with regard to e-mails and environmental cues with the placement of sensors, more specific work on the types of contextual cues to aid with behaviour change should be explored.
To promote the internalisation and maintenance of PA behaviour change, future intervention studies should support all of the basic psychological needs posited as important in SDT (i.e. competence, autonomy, relatedness to others). 175,176,212 Studies should also examine how the social and physical environment can influence participants’ experience of PA interventions, and behaviour change (e.g. enjoyment, autonomy). Finally, behaviour change theories should be developed which accurately capture the process of PA behaviour change for specific individuals. This is reliant on well-designed intervention studies investigating causal mechanisms by means of mediation and moderation analyses, and exploring how these constructs operate together.
Engagement and non-use attrition: implications for future research
Participant engagement and non-use attrition are challenges faced in public health intervention research. Therefore, future intervention studies should measure levels of engagement and non-usage with a view to making recommendations for retaining groups of participants who are at the highest risk for non-usage and lack of intervention engagement. This is particularly important for studies of web-based interventions which are known to be particularly susceptible to non-usage and lack of participant engagement. 114 Better guidelines on how to measure intervention engagement are needed. Future intervention studies should also consider how to ensure that those with high risk for non-use attrition keep engaging in the intervention. This is important to ensure participants receive the full potential benefits of the intervention.
Our engagement analysis indicated that when participants found the financial incentive attractive and redeemed their points for rewards, there was a positive impact on PA. This suggests that offering financial incentives can potentially encourage participants to increase their PA behaviour. However, future research is warranted investigating the ability of financial incentives to initiate PA and facilitate long-term behaviour change. There is little evidence on optimal monetary values, varieties (e.g. retail vouchers, more generic gift cards, charity donations, group rewards) or timing of incentives, for achieving health behaviour change. Interventionists should consider how to retain participants who are initially less financially motivated in studies whose main component is the offer of financial rewards. Moreover, where trials of complex interventions such as the PAL scheme result in a negative primary finding, future work needs to determine which element of the intervention ‘does not work’ or whether there is a ‘failure’ that signifies suboptimal fidelity, dose, implementation or of the underlying programme logic model. For studies of PA interventions requiring behavioural practice in the outdoor environment (as was the case for the current study), participants’ perceptions of the environment with respect to PA are important influencing factors that should be mitigated to optimise engagement and minimise non-usage.
Overall limitations and strengths
There is a long-standing belief that positive results are favoured by scientific journals and that this may contribute to ‘publication bias’. On the other hand, some journals claim now to select articles for publication based on their contribution to the literature and welcome null results that challenge conventional wisdom or prior expectations. 216 The results from our trial certainly challenged prior expectations. However, it is notoriously hard to disprove any hypothesis, and so negative studies must have the precision and strength of design to be reasonably persuasive. The revised sample size and power calculations for our trial were approved by NIHR, and the effects size on which they were premised was within the category of ‘moderate’ effect size observed in past PA interventions. 217 Even though attrition and loss to follow-up in our study was higher than predicted (but comparable to some other published workplace PA trials, such as those of van Dongen et al. 147 and Puig-Ribera et al. 148), we might have concluded that accepting the null hypothesis at 12 months was justified. However, the primary outcome was specified at 6 months and the non-significant difference in the mean number of steps per day at 12 months (≈500 steps) was, arguably, still of a magnitude which could be of public health importance. We would probably have claimed as much had the direction of the intervention effect been positive. If the significant negative effect at 6 months is indicative of a true negative effect of this intervention in this setting, one might still ask whether the result is generalisable. Is this recruited sample of public sector office workers in Northern Ireland representative of office workers elsewhere in the UK? We have no reason to conclude that it is not,2–4,37 but are mindful of the modelling undertaken by Basu and Kiernan,150 which demonstrated that two key factors impacting the success of workplace-based financial incentives for behaviour change are (1) who participates and (2) the levels of incentives. Thus, we need to examine carefully the strengths and limitations of our mediation and moderation analyses to gain a better understanding of our findings.
The authors acknowledge the well-known self-report biases associated with the use of questionnaires. Where possible, well validated questionnaires were used. There are some limitations regarding the use of a pedometer for measuring our primary outcome. These include the inability to consider wear time in the processing of the data. The use of < 250 steps per day as an indicator of non-wear could have removed participants who were inactive, or included participants who wore the monitor for a short period and erroneously classified them as inactive. The use of a pedometer meant we were not able to detect when in the day the PA was accumulated. Using a research grade accelerometer could have addressed some of these limitations. Obviously careful consideration was given to our choice of primary outcome and the appropriate measurement instrument. This inevitably involved a trade-off between reliability, efficiency and the cost of PA measurement in a sample of this size (n = 1390 was our initial sample size). First, we wanted a measure of total PA, which is captured by the pedometer, not just MVPA. However, the estimated cost for using accelerometers would be £400,000 (Actigraph GT3X ≈£250 each) compared with £75,796 for the pedometers. After careful consideration, the study team agreed that pedometers provided an objective, valid and reliable measure of PA at a reasonable cost for this sample size.
We were able to specifically capture workplace PA using the remote sensing monitoring system (sensors and key fobs) and the GPAQ, which incorporates a workplace PA domain. However, we used a well-validated pedometer, followed a standardised measurement protocol (including sealing the pedometer to prevent reactivity), and we supplemented the data with the GPAQ and daily PA monitoring using the remote sensing system. A broader question remains whether a maximum of seven days of physical activity measurement at baseline, 6 months and 12 months is sufficient to adequately document behaviour change. However, at present this is the most commonly used approach. 158
The mediation analyses controlled for baseline values and improves on previous studies, which have usually relied on cross-sectional data. We followed recommended practice and used bias-corrected bootstrap CIs in assessing the significance of the indirect effects. 120,121 Studying the relationship between intervention engagement, PA outcomes and mediators is a useful adjunct to other aspects of the trial’s process evaluation that can help us gain additional insights and perspective on the overall results. Previous studies have investigated whether engagement (or adherence) is predictive of behavioural outcomes204,218–220 without consideration of how they may relate to the mechanisms of behaviour change. A strength of our analysis is the examination of several distinct indicators of engagement with different components of the intervention. The wealth of our qualitative data supporting and complementing the quantitative mediator analysis is a key asset that helps us look at ‘pictures as a whole’ to find the missing pieces of the health improvement intervention ‘jigsaw’ described by Tannahill and Kelly. 142 Qualitative analysis is to a certain extent, subjective and reliant on the researchers’ interpretation of the data. 221 However, all transcripts in our trial were independently coded by two members of the research team (one member was directly involved in facilitating the focus group discussions).
Our study was not powered to detect changes in mediating variables and the power to detect changes in some mediators may have been low. Mediators were based on self-report questionnaire items and may have been subject to measurement error. Although all mediator measures were based on previously used validated measurement instruments, the internal consistency of some mediators was low. Inspection of residual plots revealed some analyses may have violated normal-distribution assumptions, and sensitivity analyses using logistic regressions showed there were changes in the significance of results for some mediators. Although previous research on engagement in intervention studies has compared engagement between an intervention group and a comparison group,118 we were unable to include comparable engagement (e.g. recording of daily activity and web usage) data from our control group because of the nature of the waiting list control. Thus we are cautious not to overinterpret any comparison between the mediators of engagement and those disclosed by our main effects analysis. Furthermore, although measures of the frequency of hits on different sections of the website may indicate the participant’s level of interest in a specific intervention component, they do not capture how well the participant processed the information.
Another potential limitation is that only baseline variables were investigated as predictors of non-use attrition. Possibly, other factors such as changing levels of satisfaction with the intervention, or of PA behaviour itself, may have influenced attrition rates. However, our goals were broadly similar to previous studies of predictors of non-use attrition,207 the aim being to better describe the groups who continue engaging in an intervention at enrolment. Our definition of non-use attrition (i.e. occurring at the time of the first 2-week lapse from intervention use) may have contributed to the high levels of non-use attrition observed because of some participants potentially taking a 2-week period of annual leave or other absence from work. In general, defining non-use attrition in workplace interventions is difficult as any period of non-use may not be indicative of an intentional lapse from using the intervention but may indicate temporary absence from the workplace. Finally, it should be remembered that mediation analysis, and analyses pertaining to intervention engagement and non-use attrition, are exploratory. Because of low power and multiple testing, they need to be interpreted with caution.
There are two key strengths to our behavioural economics analysis. First, two stated preference methods (i.e. contingent valuation and DCE) were used to investigate the optimal levels of financial incentives required to encourage changes in PA, whereas previous studies on financial incentives have eschewed such empirical a priori groundwork into the appropriate levels of financial incentives. Our approach also resonates with the argument that a better understanding of participant preferences through DCEs is useful for developing or more effectively delivering interventions. 154 Next, in these adjunct behavioural economic studies, we have used an objective measure of PA (i.e. pedometer measurement) to avoid the biases and inaccuracies of self-reported measures. In addition, using real incentives in the form of cash payments in the economic experiments is widely interpreted as helping to ensure that participants respond carefully and truthfully in the experiments, by comparison with hypothetical elicitations where the participant burden is not related to real rewards. In addition, discount rates, present-bias, risk preferences and loss aversion were jointly estimated using a tried and tested procedure to eliminate the bias that afflicts analyses that attempt their separate estimations. 102
However, the behavioural economics analysis also has several limitations. First, because of the nature of incentivised and experimentally controlled economic experiments, they take approximately 1 hour to complete. Consequently, the experiments were not conducted during the baseline assessment to keep the participant burden to a minimum. However, the participants who had withdrawn from the study before the 6-month follow-up measurement (i.e. when the experiments were conducted) were not invited to participate in the experiments and this was specified in our ethics approval. This may have resulted in a biased sample154 if the participants lost to follow-up had systematically higher or lower discount rates. Next, the principle behind the time preference theory suggests that individuals who have higher discount rates and who are more present-biased are more difficult to be incentivised so that they should be provided with greater financial incentives to encourage them to commence behavioural change. This hypothesis was not tested in our study, but it opens new avenues for future research.
Table 33 provides an overview of the possible competing explanations for the results of the trial (negative primary outcome) that have been mentioned throughout the report. Broadly, these cover contextual factors, intervention design and methodological issues.
Competing explanation | Source of evidence | Strength of evidence | Author commentary |
---|---|---|---|
Contextual factors | |||
Significant organisational restructuring |
Senior management interviews Participant focus groups |
+ supportive evidence | During our recruitment phase, a number of participating organisations undertook significant restructuring because of the then current economic austerity, resulting in uncertainty regarding job security and job location; a time when employee health and well-being was at its most vulnerable. The impact of this was evident in our qualitative data, which highlighted how motivation can be more usefully seen as a property of systems (incorporating technologies, organisation and action) rather than just of individuals |
Seasonal effect | All analyses adjusted for season | – evidence against | Our analyses included an adjustment for seasonal effects that would suggest that the 6-month difference in steps per day between the intervention and control groups not caused by a seasonal effect |
Intervention design | |||
Suboptimal choice of incentives |
Participant focus groups 39% of points reimbursed for vouchers |
+ supportive evidence | The types of vouchers available were based on those which were most popular in our pilot trial (same target population) and verified in pre-intervention focus groups with the target population |
Suboptimal level of incentives |
Participant focus groups 39% of points reimbursed for vouchers |
+ supportive evidence | The level of incentive (i.e. points value) was determined by a pre-intervention Contingent Valuation survey with all participants. The determined levels were then verified in pre-intervention focus groups with the target population |
Incorrectly specified logic model | Mediation analyses | + supportive evidence |
Our results showed increased internal forms of motivation (i.e. identified regulation, integrated regulation and intrinsic motivation) for the intervention group compared with the control group at 4 weeks and 6 months Our results demonstrated that there was no intervention effect for financial (extrinsic) motivation at 4 weeks Although the intervention group showed increases in some hypothesised mediators of initiation, these increases were not related to PA behaviour at 6 months. However, hypothesised mediators of maintenance were related to PA behaviour at 6 months As reported above, our results run counter to predictions of self-determination theory suggesting that there is a role for financial incentives and self-regulation interventions. However, the amount of variance explained by our measured mediators of behaviour change was low. Therefore, future studies would need to examine other potential mediators of behaviour change to shed further light on these associations |
Technical issues causing frustration in intervention group | Participant focus groups | + supportive evidence |
There were occasional technical issues with the sensors and fobs, which had a knock on effect on points accumulated There were clearly some technical issues affecting intervention fidelity. Some of the sensors developed battery problems which affected the recording and accuracy of the PA behaviour captured, and required replacement |
Intervention too short to support behaviour change maintenance | Author hypothesis | – evidence against | Our intervention was 6 months in duration which is in line with other PA interventions attempting to elicit and support PA maintenance (a period consonant with the mean in a recent review) |
Boredom with intervention |
Participant focus groups Usage data from the PA monitoring system showed that the minutes of activity recorded on the system declined over the 6-month intervention period (participants logging at least 10 minutes of activity via the PA monitoring system on 25% of all possible intervention days) |
+ supportive evidence | New rewards, walking routes, and double points days were regularly introduced in an attempt to keep the format fresh and appealing but this did not improve workplace PA |
Study participants in the intervention group could have felt patronised or infantilised by certain components of the intervention which may have been considered ‘control mechanisms’ by some. For example, steps being counted and recorded online, regular e-mail prompts | Reviewer hypothesis | – evidence against |
These specific intervention components are not ‘control mechanisms’ but are evidence-based behaviour change techniques that were embedded within the intervention to help support PA behaviour change initiation and maintenance. The rationale for each intervention component is detailed in Chapter 2, Intervention group, and the logic model (see Figure 1) Furthermore, there was also no evidence from our focus group discussions to suggest that the participants felt ‘patronised’ or ‘infantalised’. Rather our focus group findings suggested that participants valued the opportunity to self-monitor their PA behaviour and receive regular feedback (both evidence-based behaviour change techniques). They also found the maps very helpful in letting them know where the sensors were placed. However, it is possible that those who attended a focus group were more likely to be positive which is a common criticism of qualitative research |
Methodological considerations | |||
Selection bias | Reviewer hypothesis | – evidence against | Our eligibility criteria was very broad; participants were randomly allocated using suitable methods; cluster randomisation limited contamination between groups; the groups were balanced at baseline for key demographic characteristics; demographic characteristics of the recruited sample are similar to the demographics of public sector employees in Northern Ireland |
Physical activity measurement tool | Reviewer hypothesis | – evidence against | A well validated, objective measure of physical activity was employed following a validated, standardised protocol |
Inadequate sample size | Author hypothesis | + supportive evidence | The revised sample size and power calculations for our trial were approved by the study funder, and the effect size on which they were premised was within the category of ‘moderate’ effect sizes observed in past physical activity interventions |
Hawthorne effect in control group | Reviewer hypothesis | – evidence against | At 6 months, the intervention group decreased the mean number of steps per day by 947 steps, and the control group by 398 steps from baseline. At 12 months, the intervention group maintained a reduction in steps from baseline (decrease of 552 steps), with the control group returning to baseline levels (increase of 98 steps from baseline) |
Confounding | Author hypothesis | – evidence against | All analyses were adjusted for baseline values, randomisation stratum (size of participating organisation) and season with standard errors corrected for clustering. The analysis was by intention to treat |
Research recommendations
Several recommendations for intervention design, trial design and future research have been made throughout the report. Table 34 provides a comprehensive summary of these recommendations. Briefly, key methodological recommendations include accounting for negative findings and conflicting results between primary and secondary outcomes, the need to account for context, understanding causal mechanisms, and engagement and non-use attrition. Intervention design recommendations include the use of financial incentives and self-regulation techniques, the design of financial incentives-based interventions, the role of SDT and the need to integrate individual and employer perspectives.
Consideration | Recommendation |
---|---|
Methodological | |
Negative findings | Determine which element of the intervention ‘did not work’ or whether there is a ‘failure’ that signifies suboptimal fidelity, dose, implementation or of the underlying programme logic model |
Conflicting findings from primary and secondary outcomes | Need to develop methods on how best to balance positive and negative results when primary and secondary outcomes are discordant |
Taking account of context | Future interventions must take account of context in their intervention and evaluation framework |
Understanding causal mechanisms | Need for a framework based on consensus about how mediation should be measured and tested in trials of complex interventions. Such a framework should include the use of formal mediation tests, the embedding of evidence-based techniques for changing hypothesised mediators and the need to investigate constructs with particular relevance for initiation and maintenance of behaviour change |
Future research should examine mediators of adverse effects so we can better understand unintended consequences and negative findings | |
Behaviour change theories should be developed which accurately capture the process of PA behaviour change for specific individuals. This is reliant on well-designed intervention studies investigating causal mechanisms by means of mediation and moderation analyses, and exploring how these constructs operate together | |
Engagement and non-use attrition | Need to measure levels of engagement and non-usage to understand participants’ non-usage patterns and the contributing factors with a view to making recommendations for retaining groups of participants who are at the highest risk for non-usage and lack of intervention engagement |
Need to consider how to ensure that those with high risk for non-use attrition keep engaging in the intervention | |
Need better guidelines on how to measure intervention engagement | |
Intervention design | |
Use of financial incentives | Our findings suggest that using financial incentives within a complex behaviour change intervention with multiple components collectively does not necessarily diminish and may facilitate intrinsic motivation |
Our findings suggest that the provision of financial incentives does not necessarily increase financial (extrinsic) motivation | |
Our findings run counter to predictions of self-determination theory suggesting that there is a role for financial incentives and self-regulation interventions | |
Design of financial incentive component | The use of time preference theory should be considered when designing financial incentive-based interventions |
More research is needed on optimal monetary values, types (e.g. retail vouchers, generic gift cards, charity donations, group rewards) and timing of incentives, for achieving health behaviour change | |
Use of self-regulation techniques | Our findings suggest that future PA interventions should make use of self-regulation techniques (e.g. self-monitoring, goal-setting), with social and environmental prompts. This may promote habit formation and should also allow for the internalisation of the behaviour, enabling it to be more sustainable long term |
SDT | To promote the internalisation and maintenance of PA behaviour change, future intervention studies should support all of the basic psychological needs posited as important in SDT (i.e. competence, autonomy, relatedness to others) |
Studies should also examine how the social and physical environment can influence participants’ experience of PA interventions, and behaviour change (e.g. enjoyment, autonomy) | |
Integration of individual and employer perspectives | Given the many barriers to being active during the working day reported by our participants, it is not clear how effective existing schemes and policies within the workplace are, or how they are being implemented. This evaluative work highlighted the need for a ‘top-down’ approach, for employees to feel they were supported by management in being more active within the workplace |
Conclusion
In summary, the PAL scheme intervention was not more effective than the waiting list control. The reduced health-care costs, reduced absenteeism and improved mental well-being in the intervention group are somewhat noteworthy, and results suggest that the intervention could be cost beneficial for employers. Finally, we believe that the results of this trial pose several scientific and real-world implementation challenges that are too infrequently exposed in public health intervention trials.
Acknowledgements
The study team would like to acknowledge the involvement of several members from the Institute of Electronics, Communications and Information Technology at Queen’s University Belfast, for their role in developing the physical activity monitoring system and study website. They include Professor William Scanlon, Dr Adrian McKernan, Dr William Scully, Mr Ryan McConville and Mr Philip McShane. Club Marketing Ltd and Lisburn City Centre Management were instrumental in their role liaising with retail partners. We acknowledge support from project partners, the South Eastern Health and Social Care Trust and Lisburn and Castlereagh City Council. The study team also wish to acknowledge the involvement of Dr Jennifer Badham (Queen’s University Belfast) for her assistance with cleaning the main trial data.
The authors thank the study participants, many of whom contributed to multiple parts of the study in multiple years, and for guidance and support from members of the independent study steering committee.
Contributions of authors
Ruth F Hunter (Reader in Physical Activity and Public Health, Centre for Public Health, Queen’s University Belfast) was joint Principal Investigator on the project, led the design and execution of the study, oversaw all subsequent study analyses and publications and led the preparation of the final report.
Aisling Gough (Postdoctoral Research Fellow, Centre for Public Health, Queen’s University Belfast) was the project manager and lead qualitative researcher for the 6- and 12-month follow-up time points, led the qualitative analyses and publications and contributed to others, co-ordinated the preparation of the final report.
Jennifer M Murray (Postdoctoral Research Fellow, Centre for Public Health, Queen’s University Belfast) was a Doctor of Philosophy student working on the project, assisted with recruitment, data collection and data analyses, led the mediation analyses and publications and contributed to others, led the preparation of the mediation analyses of the final report.
Jianjun Tang (Postdoctoral Research Fellow, Centre for Public Health, Queen’s University Belfast) led the behavioural economic aspect of the project including recruitment, data collection and data analyses, assisted with recruitment and data collection of the main trial, led the behavioural economic analyses and publications and contributed to others, led the preparation of the behavioural economic analyses of the final report. He is now a Lecturer at the School of Agricultural Economics and Rural Development, Renmin University of China, Beijing, China.
Sarah F Brennan (previous Postdoctoral Research Fellow, Centre for Public Health, Queen’s University Belfast) was the project manager for the first 2 years of the project and led recruitment and data collection up until the 6-month follow-up time point, contributed to the final report.
Oliver J Chrzanowski-Smith (previous Research Assistant, Centre for Public Health, Queen’s University Belfast) was a Research Assistant for the first 2 years of the project and assisted with recruitment and data collection up until the 6-month follow-up time point, contributed to the final report. He is now a PhD student at the University of Bath.
Angela Carlin (previous Research Assistant, Centre for Public Health, Queen’s University Belfast) was a Research Assistant for the final year of the project and assisted with data collection of the quantitative and qualitative data at the 12-month follow-up time point, contributed to the final report. She is now a Lecturer at Ulster University.
Chris Patterson (Professor of Medical Statistics, Centre for Public Health, Queen’s University Belfast), co-investigator, led and oversaw the conduct and interpretation of the quantitative data for the primary and secondary analyses and mediation analyses, contributed to the final report.
Alberto Longo (Professor in Environmental Economics, School of Biological Sciences, Queen’s University Belfast), co-investigator, led and oversaw the conduct and interpretation of the behavioural economic data, contributed to the final report.
George Hutchinson (Professor of Environmental Economics, School of Biological Sciences, Queen’s University Belfast), co-investigator, led and oversaw the conduct and interpretation of the behavioural economic data, contributed to the final report.
Lindsay Prior (Professor Emeritus of Sociology, Centre for Public Health, Queen’s University Belfast), co-investigator, led and oversaw the conduct and interpretation of the qualitative data, contributed to the final report.
Mark A Tully (Professor, Institute of Mental Health Sciences, Ulster University), co-investigator, advised on intervention design, physical activity measurement and analyses, contributed to the final report.
David P French (Professor of Health Psychology, School of Psychological Sciences, University of Manchester), co-investigator, oversaw the theoretical aspect of the intervention, advised on the measurement of mediators of behaviour change, was significantly involved in the interpretation of the mediation analyses, contributed to the final report.
Jean Adams (Senior Research Fellow, CEDAR, University of Cambridge), co-investigator, advised on the development of the incentive element of the intervention, contributed to the final report.
Emma McIntosh (Professor of Health Economics, Health Economics and Health Technology Assessment, University of Glasgow), co-investigator, led and oversaw the conduct and interpretation of the health economic data, contributed to the final report.
Yiqiao Xin (Research Assistant, Health Economics and Health Technology Assessment, University of Glasgow), led the health economic analyses, contributed to the final report.
Frank Kee (Professor of Public Health Medicine, Centre for Public Health, Queen’s University Belfast), joint Principal Investigator, led the design and execution of the study, oversaw all subsequent study analyses and publications and led the preparation of the final report.
Publications
Hunter RF, Brennan SF, Tang J, Smith OJ, Murray J, Tully MA, et al. Effectiveness and cost-effectiveness of a physical activity loyalty scheme for behaviour change maintenance: a cluster randomised controlled trial. BMC Public Health 2016;16:618.
Gough A, Prior L, Kee F, Hunter RF. Physical activity and behaviour change: the role of distributed motivation. Critical Public Health 2018;1–13.
Hunter RF, Murray JM, Gough A, Tang J, Patterson CC, French DP, et al. Effectiveness and cost-effectiveness of a loyalty scheme for physical activity behaviour change maintenance: results from a cluster randomised controlled trial. Int J Behav Nutr Phys Act 2018;15:127.
Murray JM, French DP, Patterson CC, Kee F, Gough A, Tang J, Hunter RF. Predicting outcomes from engagement with specific components of an internet-based physical activity intervention with financial incentives: process analysis of a cluster randomized controlled trial. J Med Internet Res 2019;21:e11394.
Data-sharing statement
All data requests should be submitted to the corresponding author for consideration. Access to anonymised data may be granted following review and necessary agreements being in place.
Patient data
This work uses data provided by patients and collected by the NHS as part of their care and support. Using patient data is vital to improve health and care for everyone. There is huge potential to make better use of information from people’s patient records, to understand more about disease, develop new treatments, monitor safety, and plan NHS services. Patient data should be kept safe and secure, to protect everyone’s privacy, and it’s important that there are safeguards to make sure that it is stored and used responsibly. Everyone should be able to find out about how patient data are used #datasaveslives. You can find out more about the background to this citation here: https://understandingpatientdata.org.uk/data-citation.
Disclaimers
This report presents independent research funded by the National Institute for Health Research (NIHR). The views and opinions expressed by authors in this publication are those of the authors and do not necessarily reflect those of the NHS, the NIHR, NETSCC, the PHR programme or the Department of Health and Social Care. If there are verbatim quotations included in this publication the views and opinions expressed by the interviewees are those of the interviewees and do not necessarily reflect those of the authors, those of the NHS, the NIHR, NETSCC, the PHR programme or the Department of Health and Social Care.
References
- Hunter RF, Tully MA, Davis M, Stevenson M, Kee F. Physical activity loyalty cards for behavior change: a quasi-experimental study. Am J Prev Med 2013;45:56-63. https://doi.org/10.1016/j.amepre.2013.02.022.
- Abraham C, Graham-Rowe E. Are worksite interventions effective in increasing physical activity? A systematic review and meta-analysis. Health Psychol Rev 2009;3:108-44. https://doi.org/10.1080/17437190903151096.
- Conn VS, Hafdahl AR, Cooper PS, Brown LM, Lusk SL. Meta-analysis of workplace physical activity interventions. Am J Prev Med 2009;37:330-9. https://doi.org/10.1016/j.amepre.2009.06.008.
- Rongen A, Robroek SJ, van Lenthe FJ, Burdorf A. Workplace health promotion: a meta-analysis of effectiveness. Am J Prev Med 2013;44:406-15. https://doi.org/10.1016/j.amepre.2012.12.007.
- Lee IM, Shiroma EJ, Lobelo F, Puska P, Blair SN, Katzmarzyk PT. Lancet Physical Activity Series Working Group . Effect of physical inactivity on major non-communicable diseases worldwide: an analysis of burden of disease and life expectancy. Lancet 2012;380:219-29. https://doi.org/10.1016/S0140-6736(12)61031-9.
- Ding D, Lawson KD, Kolbe-Alexander TL, Finkelstein EA, Katzmarzyk PT, van Mechelen W, et al. Lancet Physical Activity Series 2 Executive Committee . The economic burden of physical inactivity: a global analysis of major non-communicable diseases. Lancet 2016;388:1311-24. https://doi.org/10.1016/S0140-6736(16)30383-X.
- Allender S, Foster C, Scarborough P, Rayner M. The burden of physical activity-related ill health in the UK. J Epidemiol Community Health 2007;61:344-8. https://doi.org/10.1136/jech.2006.050807.
- Department of Health and Social Care . Start Active, Stay Active. Report on Physical Activity in the UK 2011. www.gov.uk/government/publications/start-active-stay-active-a-report-on-physical-activity-from-the-four-home-countries-chief-medical-officers (accessed 28 March 2018).
- Farrell L, Hollingsworth B, Propper C, Shields MA. The Socioeconomic Gradient in Physical Inactivity in England. CMPO Working Paper Series No. 13 311 2013. www.bristol.ac.uk/media-library/sites/cmpo/migrated/documents/wp311.pdf (accessed 11 September 2018).
- Sport Northern Ireland . The Northern Ireland Sport and Physical Activity Survey 2010 (SAPAS) 2011. www.sportni.net/sportni/wp-content/uploads/2013/03/SAPASReport.pdf (accessed 28 March 2018).
- Department for Communities . Experience of Sport and Physical Activity in Northern Ireland: Findings from the Continuous Household Survey 2015 2016 2016. www.communities-ni.gov.uk/sites/default/files/publications/communities/experience-of-sport-and-physical-activity-in-northern-ireland-201516.pdf (accessed 28 March 2018).
- National Institute for Health and Care Excellence (NICE) . Physical Activity: Local Government Briefing 2012. www.nice.org.uk/advice/lgb3/chapter/Introduction (accessed 28 March 2018).
- McEachan RR, Lawton RJ, Jackson C, Conner M, Meads DM, West RM. Testing a workplace physical activity intervention: a cluster randomized controlled trial. Int J Behav Nutr Phys Act 2011;8. https://doi.org/10.1186/1479-5868-8-29.
- The Stationery Office . Working for a Healthier Tomorrow 2008. https://assets.publishing.service.gov.uk/government/uploads/system/uploads/attachment_data/file/209782/hwwb-working-for-a-healthier-tomorrow.pdf (accessed 28 March 28 2018).
- Department for Work and Pensions . Building the Case for Wellness 2008. www.gov.uk/government/publications/work-health-and-wellbeing-building-the-case-for-wellness (accessed 28 March 2018).
- Ball K, Jeffery RW, Abbott G, McNaughton SA, Crawford D. Is healthy behavior contagious: associations of social norms with physical activity and healthy eating. Int J Behav Nutr Phys Act 2010;7. https://doi.org/10.1186/1479-5868-7-86.
- Verplanken B, Orbell S. Reflections on past behavior: a self-report index of habit strength. J Appl Soc Psychol 2003;33:1313-30. https://doi.org/10.1111/j.1559-1816.2003.tb01951.x.
- O’Malley GC, Baker PR, Francis DP, Perry I, Foster C. Incentive-based interventions for increasing physical activity and fitness (protocol). Cochrane Database Syst Rev 2012;1. https://doi.org/10.1002/14651858.CD009598.
- Higgins R, Murphy B, Worcester M, Daffey A. Supporting chronic disease self-management: translating policies and principles into clinical practice. Aust J Prim Health 2012;18:80-7. https://doi.org/10.1071/PY11006.
- Scholz U, Sniehotta FF, Schwarzer R. Predicting physical exercise in cardiac rehabilitation: the role of phase-specific self-efficacy beliefs. J Sport Exerc Psychol 2005;27:135-51. https://doi.org/10.1123/jsep.27.2.135.
- Giles EL, Robalino S, Sniehotta FF, Adams J, McColl E. Acceptability of financial incentives for encouraging uptake of healthy behaviours: a critical review using systematic methods. Prev Med 2015;73:145-58. https://doi.org/10.1016/j.ypmed.2014.12.029.
- Giles EL, Sniehotta FF, McColl E, Adams J. Acceptability of financial incentives and penalties for encouraging uptake of healthy behaviours: focus groups. BMC Public Health 2015;15. https://doi.org/10.1186/s12889-015-1409-y.
- Volpp KG, Troxel AB, Pauly MV, Glick HA, Puig A, Asch DA, et al. A randomized, controlled trial of financial incentives for smoking cessation. N Engl J Med 2009;360:699-70. https://doi.org/10.1056/NEJMsa0806819.
- Mantzari E, Vogt F, Shemilt I, Wei Y, Higgins JP, Marteau TM. Personal financial incentives for changing habitual health-related behaviors: a systematic review and meta-analysis. Prev Med 2015;75:75-8. https://doi.org/10.1016/j.ypmed.2015.03.001.
- Olsho LE, Klerman JA, Wilde PE, Bartlett S. Financial incentives increase fruit and vegetable intake among Supplemental Nutrition Assistance Program participants: a randomized controlled trial of the USDA Healthy Incentives Pilot. Am J Clin Nutr 2016;104:423-35. https://doi.org/10.3945/ajcn.115.129320.
- Guenther PM, Kirkpatrick SI, Reedy J, Krebs-Smith SM, Buckman DW, Dodd KW, et al. The Healthy Eating Index-2010 is a valid and reliable measure of diet quality according to the 2010 Dietary Guidelines for Americans. J Nutr 2014;144:399-407. https://doi.org/10.3945/jn.113.183079.
- Giles EL, Robalino S, McColl E, Sniehotta FF, Adams J. The effectiveness of financial incentives for health behaviour change: systematic review and meta-analysis. PLOS ONE 2014;9. https://doi.org/10.1371/journal.pone.0090347.
- Molema CCM, Wendel-Vos GCW, Puijk L, Jensen JD, Schuit AJ, de Wit GA. A systematic review of financial incentives given in the healthcare setting; do they effectively improve physical activity levels?. BMC Sports Sci Med Rehabil 2016;8. https://doi.org/10.1186/s13102-016-0041-1.
- Shin DW, Yun JM, Shin JH, Kwon H, Min HY, Joh HK, et al. Enhancing physical activity and reducing obesity through smartcare and financial incentives: a pilot randomized trial. Obesity 2017;25:302-10. https://doi.org/10.1002/oby.21731.
- Christian D, Todd C, Hill R, Rance J, Mackintosh K, Stratton G, et al. Active children through incentive vouchers – evaluation (ACTIVE): a mixed-method feasibility study. BMC Public Health 2016;16. https://doi.org/10.1186/s12889-016-3381-6.
- Jochelson K. Paying the Patient: Improving Health Using Financial Incentives. London; 2007.
- Marteau TM, Ashcroft RE, Oliver A. Using financial incentives to achieve healthy behaviour. BMJ 2009;338. https://doi.org/10.1136/bmj.b1415.
- Boyd KA, Briggs AH, Bauld L, Sinclair L, Tappin D. Are financial incentives cost-effective to support smoking cessation during pregnancy?. Addiction 2016;111:360-70. https://doi.org/10.1111/add.13160.
- Paul-Ebhohimhen V, Avenell A. Systematic review of the use of financial incentives in treatments for obesity and overweight. Obes Rev 2008;9:355-67. https://doi.org/10.1111/j.1467-789X.2007.00409.x.
- National Institute for Health and Care Excellence (NICE) . Workplace Health Promotion: How to Encourage Employees to Be Physically Active: A Rapid Review of Economic Literature 2007. www.nice.org.uk/guidance/ph13/documents/workplace-physical-activity-economics-review2 (accessed 11 September 2018).
- Kohl HW, Craig CL, Lambert EV, Inoue S, Alkandari JR, Leetongin G, et al. Lancet Physical Activity Series Working Group . The pandemic of physical inactivity: global action for public health. Lancet 2012;380:294-305. https://doi.org/10.1016/S0140-6736(12)60898-8.
- Hutchinson AD, Wilson C. Improving nutrition and physical activity in the workplace: a meta-analysis of intervention studies. Health Promot Int 2012;27:238-49. https://doi.org/10.1093/heapro/dar035.
- Bird EL, Baker G, Mutrie N, Ogilvie D, Sahlqvist S, Powell J. Behavior change techniques used to promote walking and cycling: a systematic review. Health Psychol 2013;32:829-38. https://doi.org/10.1037/a0032078.
- Hunter RF, Brennan SF, Tang J, Smith OJ, Murray J, Tully MA, et al. Effectiveness and cost-effectiveness of a physical activity loyalty scheme for behaviour change maintenance: a cluster randomised controlled trial. BMC Public Health 2016;16. https://doi.org/10.1186/s12889-016-3244-1.
- National Institute for Health and Care Excellence (NICE) . Walking and Cycling: Local Measures to Promote Walking and Cycling As Forms of Travel or Recreation 2012. www.nice.org.uk/guidance/ph41 (accessed 28 March 2018).
- Department of Health and Social Care . Healthy Lives, Healthy People: Our Strategy for Public Health in England 2010. www.gov.uk/government/publications/healthy-lives-healthy-people-our-strategy-for-public-health-in-england (accessed 11 September 2018).
- Black C, Frost B. Health at Work: An Independent Review of Sickness Absence. Department for Work and Pensions 2011. www.gov.uk/government/publications/review-of-the-sickness-absence-system-in-great-britain (accessed 1 February 2017).
- Hamilton MT, Hamilton DG, Zderic TW. Role of low energy expenditure and sitting in obesity, metabolic syndrome, type 2 diabetes, and cardiovascular disease. Diabetes 2007;56:2655-67. https://doi.org/10.2337/db07-0882.
- Finkelstein EA, Haaland BA, Bilger M, Sahasranaman A, Sloan RA, Nang EEK, et al. Effectiveness of activity trackers with and without incentives to increase physical activity (TRIPPA): a randomised controlled trial. Lancet Diabetes Endocrinol 2016;4:983-95. https://doi.org/10.1016/S2213-8587(16)30284-4.
- Craig P, Dieppe P, Macintyre S, Michie S, Nazareth I, Petticrew M. Developing and Evaluating Complex Interventions: New Guidance 2008. https://mrc.ukri.org/documents/pdf/complex-interventions-guidance/ (accessed 15 July 2019).
- Miller N, Dollard J. Social Learning and Imitation. New Haven, CT: Yale University Press; 1941.
- Bandura A. Self efficacy: the exercise of control. New York, NY: Freeman; 1997.
- Hunter RF, Murray JM, Gough A, Tang J, Patterson CC, French DP, et al. Effectiveness and costeffectiveness of a loyalty scheme for physical activity behaviour change maintenance: results from a cluster randomised controlled trial. Int J Behav Nutr Phys Act 2018;15. https://doi.org/10.1086/s12966-018-0758-1.
- Bull FC, Maslin TS, Armstrong T. Global physical activity questionnaire (GPAQ): nine country reliability and validity study. J Phys Act Health 2009;6:790-804. https://doi.org/10.1123/jpah.6.6.790.
- Ware JE, Kosinski M, Dewey JE, Gandek B. How to Score and Interpret Single-Item Health Status Measures: A Manual for Users of the SF-8 Health Survey. Lincoln, RI: QualityMetric Incorporated; 2001.
- EuroQol Group . EuroQol: a new facility for the measurement of health-related quality of life. Health Policy (New York) 1991;16:199-208. https://doi.org/10.1016/0168-8510(90)90421-9.
- Lloyd K, Devine P. Psychometric properties of the Warwick-Edinburgh Mental Well-being Scale (WEMWBS) in Northern Ireland. J Ment Health 2012;21:257-63. https://doi.org/10.3109/09638237.2012.670883.
- Tennant R, Hiller L, Fishwick R, Platt S, Joseph S, Weich S, et al. The Warwick-Edinburgh Mental Well-being Scale (WEMWBS): development and UK validation. Health Qual Life Outcomes 2007;5. https://doi.org/10.1186/1477-7525-5-63.
- Kessler RC, Barber C, Beck A, Berglund P, Cleary PD, McKenas D, et al. The World Health Organization Health and Work Performance Questionnaire (HPQ). J Occup Environ Med 2003;45:156-74. https://doi.org/10.1097/01.jom.0000052967.43131.51.
- Finch EA, Linde JA, Jeffery RW, Rothman AJ, King CM, Levy RL. The effects of outcome expectations and satisfaction on weight loss and maintenance: correlational and experimental analyses – a randomized trial. Health Psychol 2005;24:608-16. https://doi.org/10.1037/0278-6133.24.6.608.
- Marcus BH, Selby VC, Niaura RS, Rossi JS. Self-efficacy and the stages of exercise behavior change. Res Q Exerc Sport 1992;63:60-6. https://doi.org/10.1080/02701367.1992.10607557.
- Moller AC, Buscemi J, McFadden HG, Hedeker D, Spring B. Financial motivation undermines potential enjoyment in an intensive diet and activity intervention. J Behav Med 2014;37:819-27. https://doi.org/10.1007/s10865-013-9542-5.
- Sniehotta FF, Schwarzer R, Scholz U, Schüz B. Action planning and coping planning for long-term lifestyle change: theory and assessment. Eur J Soc Psychol 2005;35:565-76. https://doi.org/10.1002/ejsp.258.
- Markland D, Tobin V. A modification to the behavioural regulation in exercise questionnaire to include an assessment of amotivation. J Sport Exerc Psychol 2004;26:191-6. https://doi.org/10.1123/jsep.26.2.191.
- Wilson PM, Rodgers WM, Loitz CC, Scime G. ‘It’s who I am … Really!’ The importance of integrated regulation in exercise contexts. J Appl Biobehav Res 2007;11:79-104. https://doi.org/10.1111/j.1751-9861.2006.tb00021.x.
- Fishbein M, Ajzen I. Belief, Attitude, Intention and Behavior: An Introduction to Theory and Research. Reading: Addison-Wesley; 1975.
- Ogilvie D, Mitchell R, Mutrie N, Petticrew M, Platt S. Perceived characteristics of the environment associated with active travel: development and testing of a new scale. Int J Behav Nutr Phys Act 2008;5. https://doi.org/10.1186/1479-5868-5-32.
- Frank LD, Sallis JF, Saelens BE, Leary L, Cain K, Conway TL, et al. The development of a walkability index: application to the Neighborhood Quality of Life Study. Br J Sports Med 2010;44:924-33. https://doi.org/10.1136/bjsm.2009.058701.
- Dalton AM, Jones AP, Panter JR, Ogilvie D. Neighbourhood, route and workplace-related environmental characteristics predict adults’ mode of travel to work. PLOS ONE 2013;8. https://doi.org/10.1371/journal.pone.0067575.
- Rothman AJ, Sheeran P, Wood W. Reflective and automatic processes in the initiation and maintenance of dietary change. Ann Behav Med 2009;38:4-17. https://doi.org/10.1007/s12160-009-9118-3.
- Harrington J, Perry I, Lutomski J, Morgan K, McGee H, Shelley E, et al. SLÁN 2007: Survey of Lifestyle, Attitudes and Nutrition in Ireland. Dietary Habits of the Irish Population. Dublin: Department of Health and Children; 2008.
- Harrington J, Perry IJ, Lutomski J, Fitzgerald AP, Shiely F, McGee H, et al. Living longer and feeling better: healthy lifestyle, self-rated health, obesity and depression in Ireland. Eur J Public Health 2010;20:91-5. https://doi.org/10.1093/eurpub/ckp102.
- Thompson S, Wordsworth S. UK Working Party on Patient Costs. An Annotated Cost Questionnaire for Completion by Patients 2001.
- Steckler A, Linnan L, Israel B. Process Evaluation for Public Health Interventions and Research. San Francisco, CA: Jossey-Bass; 2002.
- Tudor-Locke C, Burkett L, Reis JP, Ainsworth BE, Macera CA, Wilson DK. How many days of pedometer monitoring predict weekly physical activity in adults?. Prev Med 2005;40:293-98. https://doi.org/10.1016/j.ypmed.2004.06.003.
- Bassett DR, Ainsworth BE, Leggett SR, Mathien CA, Main JA, Hunter DC, et al. Accuracy of five electronic pedometers for measuring distance walked. Med Sci Sports Exerc 1996;28:1071-7. https://doi.org/10.1097/00005768-199608000-00019.
- Schneider PL, Crouter SE, Lukajic O, Bassett DR. Accuracy and reliability of 10 pedometers for measuring steps over a 400-m walk. Med Sci Sports Exerc 2003;35:1779-84. https://doi.org/10.1249/01.MSS.0000089342.96098.C4.
- Tudor-Locke C, Craig CL, Brown WJ, Clemes SA, De Cocker K, Giles-Corti B, et al. How many steps/day are enough? For adults. Int J Behav Nutr Phys Act 2011;8. https://doi.org/10.1186/1479-5868-8-79.
- Tudor-Locke C, Williams JE, Reis JP, Pluto D. Utility of pedometers for assessing physical activity: convergent validity. Sports Med 2002;32:795-808. https://doi.org/10.2165/00007256-200232120-00004.
- Corder K, Brage S, Ekelund U. Accelerometers and pedometers: methodology and clinical application. Curr Opin Clin Nutr Metab Care 2007;10:597-603. https://doi.org/10.1097/MCO.0b013e328285d883.
- Azur MJ, Stuart EA, Frangakis C, Leaf PJ. Multiple imputation by chained equations: what is it and how does it work?. Int J Methods Psychiatr Res 2011;20:40-9. https://doi.org/10.1002/mpr.329.
- Rubin DB. Multiple Imputation for Nonresponse in Surveys. John Wiley & Sons; 1987.
- Braun V, Clarke V. Using thematic analysis in psychology. Qual Res Psychol 2006;3:77-101. https://doi.org/10.1191/1478088706qp063oa.
- Curtis L, Burns A. Unit Costs of Health and Social Care 2016. Canterbury: PSSRU, University of Kent; 2016.
- NHS . Reference Costs 2015–16 2016. www.gov.uk/government/organisations/department-of-health-and-social-care (accessed 28 March 2018).
- Personal Social Service Research Unit (PSSRU) . Unit Costs of Health and Social Care 2015 2015. www.pssru.ac.uk/project-pages/unit-costs/unit-costs-2015/ (accessed 11 July 2019).
- Department of Health and Social Care . Reference Costs 2015–16 n.d. https://assets.publishing.service.gov.uk/government/uploads/system/uploads/attachment_data/file/577083/Reference_Costs_2015-16.pdf (accessed 11 July 2019).
- Royal College of Nursing . NHS Pay Scales 2016–17 n.d. www.rcn.org.uk/employment-and-pay/nhs-pay-scales-2016-17 (accessed 11 July 2019).
- Herdman M, Gudex C, Lloyd A, Janssen M, Kind P, Parkin D, et al. Development and preliminary testing of the new five-level version of EQ-5D (EQ-5D-5L). Qual Life Res 2011;20:1727-36. https://doi.org/10.1007/s11136-011-9903-x.
- Feng Y, Devlin N, Herdman M. Assessing the health of the general population in England: how do the three- and five-level versions of EQ-5D compare?. Health Qual Life Outcomes 2015;13. https://doi.org/10.1186/s12955-015-0356-8.
- Agborsangaya CB, Lahtinen M, Cooke T, Johnson JA. Comparing the EQ-5D 3L and 5L: measurement properties and association with chronic conditions and multimorbidity in the general population. Health Qual Life Outcomes 2014;12. https://doi.org/10.1186/1477-7525-12-74.
- van Hout B, Janssen MF, Feng YS, Kohlmann T, Busschbach J, Golicki D, et al. Interim scoring for the EQ-5D-5L: mapping the EQ-5D-5L to EQ-5D-3L value sets. Value Health 2012;15:708-15. https://doi.org/10.1016/j.jval.2012.02.008.
- National Institute for Health and Care Excellence (NICE) . Guide to the Methods of Technology Appraisal 2013. www.nice.org.uk/process/pmg9/chapter/the-reference-case#measuring-and-valuing-health-effects (accessed 28 March 2018).
- Brazier J, Ratcliffe J, Saloman J, Tsuchiya A. Measuring and Valuing Health Benefits for Economic Evaluation. Oxford: Oxford University Press; 2017.
- McMillan A, Bratton DJ, Faria R, Laskawiec-Szkonter M, Griffin S, Davies RJ, et al. A multicentre randomised controlled trial and economic evaluation of continuous positive airway pressure for the treatment of obstructive sleep apnoea syndrome in older people: PREDICT. Health Technol Assess 2015;19. https://doi.org/10.3310/hta19400.
- MacNeil Vroomen J, Eekhout I, Dijkgraaf MG, van Hout H, de Rooij SE, Heymans MW, et al. Multiple imputation strategies for zero-inflated cost data in economic evaluations: which method works best?. Eur J Health Econ 2016;17:939-50. https://doi.org/10.1007/s10198-015-0734-5.
- Paton NI, Stöhr W, Oddershede L, Arenas-Pinto A, Walker S, Sculpher M, et al. The Protease Inhibitor Monotherapy Versus Ongoing Triple Therapy (PIVOT) trial: a randomised controlled trial of a protease inhibitor monotherapy strategy for long-term management of human immunodeficiency virus infection. Health Technol Assess 2016;20. https://doi.org/10.3310/hta20210.
- White IR, Royston P, Wood AM. Multiple imputation using chained equations: issues and guidance for practice. Stat Med 2011;30:377-99. https://doi.org/10.1002/sim.4067.
- Manca A, Hawkins N, Sculpher MJ. Estimating mean QALYs in trial-based cost-effectiveness analysis: the importance of controlling for baseline utility. Health Econ 2005;14:487-96. https://doi.org/10.1002/hec.944.
- Dallat MA, Hunter RF, Tully MA, Cairns KJ, Kee F. A lesson in business: cost-effectiveness analysis of a novel financial incentive intervention for increasing physical activity in the workplace. BMC Public Health 2013;13. https://doi.org/10.1186/1471-2458-13-953.
- Lahiri S, Faghri PD. Cost-effectiveness of a workplace-based incentivized weight loss program. J Occup Environ Med 2012;54:371-7. https://doi.org/10.1097/JOM.0b013e318247a394.
- Barte JCM, Wendel-Vos GCW. A systematic review of financial incentives for physical activity: the effects on physical activity and related outcomes. Behav Med 2017;43:79-90. https://doi.org/10.1080/08964289.2015.1074880.
- Giles EL, Becker F, Ternent L, Sniehotta FF, McColl E, Adams J. Acceptability of financial incentives for health behaviours: a discrete choice experiment. PLOS ONE 2016;11. https://doi.org/10.1371/journal.pone.0157403.
- Bickel WK, Moody L, Higgins ST. Some current dimensions of the behavioral economics of health-related behavior change. Prev Med 2016;92:16-23. https://doi.org/10.1016/j.ypmed.2016.06.002.
- Barlow P, McKee M, Reeves A, Galea G, Stuckler D. Time-discounting and tobacco smoking: a systematic review and network analysis. Int J Epidemiol 2017;46:860-9. https://doi.org/10.1093/ije/dyw233.
- Story GW, Vlaev I, Seymour B, Darzi A, Dolan RJ. Does temporal discounting explain unhealthy behavior? A systematic review and reinforcement learning perspective. Front Behav Neurosci 2014;8. https://doi.org/10.3389/fnbeh.2014.00076.
- Andersen S, Harrison GW, Lau MI, Rutström EE. Eliciting risk and time preferences. Econometrica 2008;76:583-618. https://doi.org/10.1111/j.1468-0262.2008.00848.x.
- Kahneman D, Tversky A, MacLean LC, Ziemba WT. Handbook of the Fundamentals of Financial Decision Making: Part I. Toh Tuck Link, Singapore: World Scientific; 2013.
- Hanemann M, Loomis J, Kanninen B. Statistical efficiency of double-bounded dichotomous choice contingent valuation. Am J Agric Econ 1991;73:1255-63. https://doi.org/10.2307/1242453.
- Ferrini S, Scarpa R. Designs with a priori information for nonmarket valuation with choice experiments: a Monte Carlo study. J Environ Econ Manage 2007;53:342-63. https://doi.org/10.1016/j.jeem.2006.10.007.
- Coller M, Williams MB. Eliciting individual discount rates. Exp Econ 1999;2:107-27. https://doi.org/10.1023/A:1009986005690.
- Laibson D. Golden eggs and hyperbolic discounting. Q J Econ 1997;112:443-78. https://doi.org/10.1162/003355397555253.
- Fiebig DG, Keane MP, Louviere J, Wasi N. The generalized multinomial logit model: accounting for scale and coefficient heterogeneity. Mark Sci 2010;29:393-421. https://doi.org/10.1287/mksc.1090.0508.
- Cragg JG. Some statistical models for limited dependent variables with application to the demand for durable goods. Econometrica 1971;39:829-44. https://doi.org/10.2307/1909582.
- Ferecatu A, Onculer A. Heterogeneous risk and time preferences. J Risk Uncertain 2016;53:1-28. https://doi.org/10.1007/s11166-016-9243-x.
- Saul JE, Amato MS, Cha S, Graham AL. Engagement and attrition in internet smoking cessation interventions: insights from a cross-sectional survey of ‘one-hit-wonders’. Internet Interv 2016;5:23-9. https://doi.org/10.1016/j.invent.2016.07.001.
- Saunders RP, Evans MH, Joshi P. Developing a process-evaluation plan for assessing health promotion program implementation: a how-to guide. Health Promot Pract 2005;6:134-47. https://doi.org/10.1177/1524839904273387.
- Thomson JL, Tussing-Humphreys LM, Goodman MH, Zoellner JM. Engagement indicators predict health changes in a lifestyle intervention. Am J Health Behav 2015;39:409-20. https://doi.org/10.5993/AJHB.39.3.13.
- Eysenbach G. The law of attrition. J Med Internet Res 2005;7. https://doi.org/10.2196/jmir.7.1.e11.
- World Health Organization . Global Recommendations on Physical Activity for Health 2010.
- Kelders SM, Kok RN, Ossebaard HC, Van Gemert-Pijnen JE. Persuasive system design does matter: a systematic review of adherence to web-based interventions. J Med Internet Res 2012;14. https://doi.org/10.2196/jmir.2104.
- Guertler D, Vandelanotte C, Kirwan M, Duncan MJ. Engagement and nonusage attrition with a free physical activity promotion program: the case of 10,000 Steps Australia. J Med Internet Res 2015;17. https://doi.org/10.2196/jmir.4339.
- Kolt GS, Rosenkranz RR, Vandelanotte C, Caperchione CM, Maeder AJ, Tague R, et al. Using Web 2.0 applications to promote health-related physical activity: findings from the WALK 2.0 randomised controlled trial. Br J Sports Med 2017;51:1433-40. https://doi.org/10.1136/bjsports-2016-096890.
- Preacher KJ, Hayes AF. Asymptotic and resampling strategies for assessing and comparing indirect effects in multiple mediator models. Behav Res Methods 2008;40:879-91. https://doi.org/10.3758/BRM.40.3.879.
- Mackinnon DP, Lockwood CM, Williams J. Confidence limits for the indirect effect: distribution of the product and resampling methods. Multivariate Behav Res 2004;39. https://doi.org/10.1207/s15327906mbr3901_4.
- MacKinnon D. Introduction to Statistical Mediation Analysis. New York, NY: Taylor & Francis; 2007.
- Hooper D, Coughlan J, Mullen M. Structural equation modelling: guidelines for determining model fit. Electron J Bus Res Methods 2008;6:53-60.
- Muller D, Judd CM, Yzerbyt VY. When moderation is mediated and mediation is moderated. J Pers Soc Psychol 2005;89:852-63. https://doi.org/10.1037/0022-3514.89.6.852.
- Rothman AJ, Baldwin AS, Deaux K, Snyder M. The Oxford Handbook of Personality and Social Psychology. Oxford: Oxford University Press; 2012.
- Hosmer DW, Lemeshow S, Sturdivant RX, Hosmer DW, Lemeshow S, Sturdivant RX. Applied Logistic Regression. New York, NY: John Wiley & Sons, Inc; 2013.
- Borucka J. Methods for handling tied events in the Cox proportional hazard model. Stud Oeconomica Posnaniensia 2014;2:91-106.
- National Institute for Health and Care Excellence (NICE) . Methods for the Development of NICE Public Health Guidance (Third Edition) [PMG4]. 6 Incorporating Health Economics 2012. www.nice.org.uk/process/pmg4/chapter/incorporating-health-economics (accessed 11 September 2018).
- Tang J, Hutchinson WG, Chilton SM, Hunter R, Lau MI, Kee F. Exponential or hyperbolic? Identifying and testing the predictive power of time preference over unhealthy behaviours. Soc Sci Res Netw 2016. https://doi.org/10.2139/ssrn.2823468.
- Andersen S, Harrison GW, Lau MI, Rutström EE. Discounting behavior: a reconsideration. Eur Econ Rev 2014;71:15-33. https://doi.org/10.1016/j.euroecorev.2014.06.009.
- Liebenehm S, Waibel H. Simultaneous estimation of risk and time preferences among small-scale cattle farmers in West Africa. Am J Agric Econ 2014;96:1420-38. https://doi.org/10.1093/ajae/aau056.
- Tanaka T, Camerer CF, Nguyen Q. Risk and time preferences: linking experimental and household survey data from Vietnam. Am Econ Rev 2010;100:557-71. https://doi.org/10.1257/aer.100.1.557.
- Cheong J, Mackinnon DP, Khoo ST. Investigation of mediational processes using parallel process latent growth curve modeling. Struct Equ Modeling 2003;10. https://doi.org/10.1207/S15328007SEM1002_5.
- Whittle R, Mansell G, Jellema P, van der Windt D. Applying causal mediation methods to clinical trial data: what can we learn about why our interventions (don’t) work?. Eur J Pain 2017;21:614-22. https://doi.org/10.1002/ejp.964.
- Murray JM, French DP, Patterson CC, Kee F, Gough A, Tang J, et al. Predicting outcomes from engagement with specific components of an internet-based physical activity intervention with financial incentives: process analysis of a cluster randomized controlled trial. J Med Internet Res 2019;21. https://doi.org/10.2196/11394.
- Dugdill L, Brettle A, Hulme C, McCluskey S, Long AF. Workplace physical activity interventions: a systematic review. Int J Work Heal Manag 2013;1:20-4. https://doi.org/10.1108/17538350810865578.
- Malik SH, Blake H, Suggs LS. A systematic review of workplace health promotion interventions for increasing physical activity. Br J Health Psychol 2014;19:149-80. https://doi.org/10.1111/bjhp.12052.
- Schröer S, Haupt J, Pieper C. Evidence-based lifestyle interventions in the workplace – an overview. Occup Med 2014;64:8-12. https://doi.org/10.1093/occmed/kqt136.
- Finkelstein EA, Linnan LA, Tate DF, Birken BE. A pilot study testing the effect of different levels of financial incentives on weight loss among overweight employees. J Occup Environ Med 2007;49:981-9. https://doi.org/10.1097/JOM.0b013e31813c6dcb.
- Tanham J, Murphy MH, Breslin G. Using financial incentives to increase physical activity, weight loss and well being: a randomized control trial. Work Pap Heal Sci 2014;1:1-5.
- Cahill K, Perera R. Competitions and incentives for smoking cessation. Cochrane Database Syst Rev 2011;4. https://doi.org/10.1002/14651858.CD004307.pub4.
- Ozdemir S, Bilger M, Finkelstein EA. Stated uptake of physical activity rewards programmes among active and insufficiently active full-time employees. Appl Health Econ Health Policy 2017;15:647-56. https://doi.org/10.1007/s40258-017-0327-9.
- Tannahill A, Kelly MP. Layers of complexity in interpreting evidence on effectiveness. Public Health 2013;127:164-70. https://doi.org/10.1016/j.puhe.2012.11.011.
- Nooijen CFJ, Del Pozo-Cruz B, Nyberg G, Sanders T, Galanti MR, Forsell Y. Are changes in occupational physical activity level compensated by changes in exercise behavior?. Eur J Public Health 2018;28:940-3. https://doi.org/10.1093/eurpub/cky007.
- Godin G, Bélanger-Gravel A, Amireault S, Vohl MC, Pérusse L. The effect of mere-measurement of cognitions on physical activity behavior: a randomized controlled trial among overweight and obese individuals. Int J Behav Nutr Phys Act 2011;8. https://doi.org/10.1186/1479-5868-8-2.
- Spence JC, Burgess J, Rodgers W, Murray T. Effect of pretesting on intentions and behaviour: a pedometer and walking intervention. Psychol Health 2009;24:777-89. https://doi.org/10.1080/08870440801989938.
- Horodyska K, Luszczynska A, Hayes CB, O’Shea MP, Langøien LJ, Roos G, et al. Implementation conditions for diet and physical activity interventions and policies: an umbrella review. BMC Public Health 2015;15. https://doi.org/10.1186/s12889-015-2585-5.
- van Dongen JM, Proper KI, van Wier MF, van der Beek AJ, Bongers PM, van Mechelen W, et al. Systematic review on the financial return of worksite health promotion programmes aimed at improving nutrition and/or increasing physical activity. Obes Rev 2011;12:1031-49. https://doi.org/10.1111/j.1467-789X.2011.00925.x.
- Puig-Ribera A, Bort-Roig J, González-Suárez AM, Martínez-Lemos I, Giné-Garriga M, Fortuño J, et al. Patterns of impact resulting from a ‘sit less, move more’ web-based program in sedentary office employees. PLOS ONE 2015;10. https://doi.org/10.1371/journal.pone.0122474.
- Department of Finance and Personnel . Personnel Statistics for the 11 NI Departments 2010. https://data.gov.uk/dataset/1baa362b-715d-45e5-addc-fb0c52d81683/personnel-statistics-for-northern-ireland-departments (accessed 28 March 2018).
- Basu S, Kiernan M. A simulation modeling framework to optimize programs using financial incentives to motivate health behavior change. Med Decis Making 2016;36:48-5. https://doi.org/10.1177/0272989X15585984.
- Farooqui MA, Tan YT, Bilger M, Finkelstein EA. Effects of financial incentives on motivating physical activity among older adults: results from a discrete choice experiment. BMC Public Health 2014;14. https://doi.org/10.1186/1471-2458-14-141.
- Finkelstein EA, Brown DS, Brown DR, Buchner DM. A randomized study of financial incentives to increase physical activity among sedentary older adults. Prev Med 2008;47:182-7. https://doi.org/10.1016/j.ypmed.2008.05.002.
- Leahey TM, LaRose JG, Mitchell MS, Gilder CM, Wing RR. Small Incentives Improve Weight Loss in Women From Disadvantaged Backgrounds. Am J Prev Med 2018;54:e41-e47. https://doi.org/10.1016/j.amepre.2017.11.007.
- Tinelli M, Ryan M, Bond C. What, who and when? Incorporating a discrete choice experiment into an economic evaluation. Health Econ Rev 2016;6. https://doi.org/10.1186/s13561-016-0108-4.
- Koffarnus MN, Jarmolowicz DP, Mueller ET, Bickel WK. Changing delay discounting in the light of the competing neurobehavioral decision systems theory: a review. J Exp Anal Behav 2013;99:32-57. https://doi.org/10.1002/jeab.2.
- Halpern SD, French B, Small DS, Saulsgiver K, Harhay MO, Audrain-McGovern J, et al. Randomized trial of four financial-incentive programs for smoking cessation. N Engl J Med 2015;372:2108-17. https://doi.org/10.1056/NEJMoa1414293.
- Teixeira PJ, Carraça EV, Markland D, Silva MN, Ryan RM. Exercise, physical activity, and self-determination theory: a systematic review. Int J Behav Nutr Phys Act 2012;9. https://doi.org/10.1186/1479-5868-9-78.
- Teixeira PJ, Carraça EV, Marques MM, Rutter H, Oppert JM, De Bourdeaudhuij I, et al. Successful behavior change in obesity interventions in adults: a systematic review of self-regulation mediators. BMC Med 2015;13. https://doi.org/10.1186/s12916-015-0323-6.
- Kassavou A, Turner A, Hamborg T, French DP. Predicting maintenance of attendance at walking groups: testing constructs from three leading maintenance theories. Health Psychol 2014;33:752-6. https://doi.org/10.1037/hea0000015.
- Kaushal N, Rhodes RE. Exercise habit formation in new gym members: a longitudinal study. J Behav Med 2015;38:652-63. https://doi.org/10.1007/s10865-015-9640-7.
- Wood W, Rünger D. Psychology of habit. Annu Rev Psychol 2016;67:289-314. https://doi.org/10.1146/annurev-psych-122414-033417.
- Lally P, Gardner B. Promoting habit formation. Health Psychol Rev 2013;7:S137-58. https://doi.org/10.1080/17437199.2011.603640.
- Gardner B, de Bruijn GJ, Lally P. A systematic review and meta-analysis of applications of the Self-Report Habit Index to nutrition and physical activity behaviours. Ann Behav Med 2011;42:174-87. https://doi.org/10.1007/s12160-011-9282-0.
- Kwasnicka D, Dombrowski SU, White M, Sniehotta F. Theoretical explanations for maintenance of behaviour change: a systematic review of behaviour theories. Health Psychol Rev 2016;10:277-96. https://doi.org/10.1080/17437199.2016.1151372.
- Murray JM, Brennan SF, French DP, Patterson CC, Kee F, Hunter RF. Effectiveness of physical activity interventions in achieving behaviour change maintenance in young and middle aged adults: a systematic review and meta-analysis. Soc Sci Med 2017;192:125-33. https://doi.org/10.1016/j.socscimed.2017.09.021.
- Threlfall AG, Meah S, Fischer AJ, Cookson R, Rutter H, Kelly MP. The appraisal of public health interventions: the use of theory. J Public Health 2015;37:166-71. https://doi.org/10.1093/pubmed/fdu044.
- King AC, Toobert D, Ahn D, Resnicow K, Coday M, Riebe D, et al. Perceived environments as physical activity correlates and moderators of intervention in five studies. Am J Health Promot 2006;21:24-35. https://doi.org/10.1177/089011710602100106.
- Rhodes RE, Dickau L. Moderators of the intention–behaviour relationship in the physical activity domain: a systematic review. Br J Sports Med 2013;47:215-25. https://doi.org/10.1136/bjsports-2011-090411.
- Promberger M, Marteau TM. When do financial incentives reduce intrinsic motivation? Comparing behaviors studied in psychological and economic literatures. Health Psychol 2013;32:950-7. https://doi.org/10.1037/a0032727.
- Johnston M, Sniehotta F. Financial incentives to change patient behaviour. J Health Serv Res Policy 2010;15:131-2. https://doi.org/10.1258/jhsrp.2010.010048.
- Johnston M. What more can we learn from early learning theory? The contemporary relevance for behaviour change interventions. Br J Health Psychol 2016;21:1-10. https://doi.org/10.1111/bjhp.12165.
- Thorndike EL, Green CD. Reproduced in Classics in the History of Physchology, an Internet Resource. Toronto, ON: York University; 1911.
- Deci EL, Eghrari H, Patrick BC, Leone DR. Facilitating internalization: the self-determination theory perspective. J Pers 1994;62:119-42. https://doi.org/10.1111/j.1467-6494.1994.tb00797.x.
- Kullgren JT, Troxel AB, Loewenstein G, Asch DA, Norton LA, Wesby L, et al. Individual- versus group-based financial incentives for weight loss: a randomized, controlled trial. Ann Intern Med 2013;158:505-14. http://doi.org/10.7326/0003-4819-158-7-201304020-00002.
- Ryan RM. Self-determination theory and wellbeing. Wellbeing Dev Ctries 2009;1:1-2.
- Deci EL, Ryan RM. Intrinsic Motivation and Self-Determination in Human Behavior. Berlin: Springer Science + Business Media; 1985.
- Hofmann W, Friese M, Wiers RW. Impulsive versus reflective influences on health behavior: a theoretical framework and empirical review. Health Psychol Rev 2008;2:111-37. https://doi.org/10.1080/17437190802617668.
- Hunt SM, Martin CJ. Health-related behavioural change – a test of a new model. Psychol Health 1988;2:209-30. https://doi.org/10.1080/08870448808400352.
- Verplanken B, Aarts H. Habit, attitude, and planned behaviour: is habit an empty construct or an interesting case of goal-directed automaticity?. Eur Rev Soc Psychol 1999;10:101-34. https://doi.org/10.1080/14792779943000035.
- Lawless L, Drichoutis AC, Nayga RM. Time preferences and health behaviour: a review. Agric Food Econ 2013;1. https://doi.org/10.1186/2193-7532-1-17.
- Lim SL, Bruce AS. Can’t wait to lose weight? Characterizing temporal discounting parameters for weight-loss. Appetite 2015;85:8-13. http://doi.org/10.1016/j.appet.2014.11.001.
- Roberto CA, Kawachi I. Use of psychology and behavioral economics to promote healthy eating. Am J Prev Med 2014;47:832-7. http://doi.org/10.1016/j.amepre.2014.08.002.
- Zhou M, Fukuoka Y, Mintz Y, Goldberg K, Kaminsky P, Flowers E, et al. Evaluating machine learning-based automated personalized daily step goals delivered through a mobile phone app: randomized controlled trial. JMIR Mhealth Uhealth 2018;6. http://doi.org/10.2196/mhealth.9117.
- Adams MA, Sallis JF, Norman GJ, Hovell MF, Hekler EB, Perata E. An adaptive physical activity intervention for overweight adults: a randomized controlled trial. PLOS ONE 2013;8. http://doi.org/10.1371/journal.pone.0082901.
- Gjesme T. Goal distance in time and its effects on the relations between achievement motives and performance. J Res Pers 1974;8:161-71. https://doi.org/10.1016/0092-6566(74)90017-8.
- Urminsky O, Goswami I, Diehl K, Yoon C, Duluth MN. Advances in Consumer Research. Association for Consumer Research; 2015.
- Kaewthummanukul T, Brown KC. Determinants of employee participation in physical activity: critical review of the literature. AAOHN J 2006;54:249-61. https://doi.org/10.1177/216507990605400602.
- Rovniak LS, Anderson ES, Winett RA, Stephens RS. Social cognitive determinants of physical activity in young adults: a prospective structural equation analysis. Ann Behav Med 2002;24:149-56. https://doi.org/10.1207/S15324796ABM2402_12.
- Burke V, Beilin LJ, Cutt HE, Mansour J, Mori TA. Moderators and mediators of behaviour change in a lifestyle program for treated hypertensives: a randomized controlled trial (ADAPT). Health Educ Res 2008;23:583-91. https://doi.org/10.1093/her/cym047.
- Darker CD, French DP, Eves FF, Sniehotta FF. An intervention to promote walking amongst the general population based on an ‘extended’ theory of planned behaviour: a waiting list randomised controlled trial. Psychol Health 2010;25:71-88. https://doi.org/10.1080/08870440902893716.
- Dutton GR, Tan F, Provost BC, Sorenson JL, Allen B, Smith D. Relationship between self-efficacy and physical activity among patients with type 2 diabetes. J Behav Med 2009;32:270-7. https://doi.org/10.1007/s10865-009-9200-0.
- Sharma M, Sargent L, Stacy R. Predictors of leisure-time physical activity among African American women. Am J Health Behav 2005;29:352-9. https://doi.org/10.5993/AJHB.29.4.7.
- French DP. The role of self-efficacy in changing health-related behaviour: cause, effect or spurious association?. Br J Health Psychol 2013;18:237-43. https://doi.org/10.1111/bjhp.12038.
- Rhodes RE, Pfaeffli LA. Mediators of physical activity behaviour change among adult non-clinical populations: a review update. Int J Behav Nutr Phys Act 2010;7. https://doi.org/10.1186/1479-5868-7-37.
- Bravata DM, Smith-Spangler C, Sundaram V, Gienger AL, Lin N, Lewis R, et al. Using pedometers to increase physical activity and improve health: a systematic review. JAMA 2007;298:2296-304. https://doi.org/10.1001/jama.298.19.2296.
- Hobbs N, Godfrey A, Lara J, Errington L, Meyer TD, Rochester L, et al. Are behavioral interventions effective in increasing physical activity at 12 to 36 months in adults aged 55 to 70 years? A systematic review and meta-analysis. BMC Med 2013;11. https://doi.org/10.1186/1741-7015-11-75.
- Michie S, Abraham C, Whittington C, McAteer J, Gupta S. Effective techniques in healthy eating and physical activity interventions: a meta-regression. Health Psychol 2009;28:690-701. https://doi.org/10.1037/a0016136.
- Williams SL, French DP. What are the most effective intervention techniques for changing physical activity self-efficacy and physical activity behaviour – and are they the same?. Health Educ Res 2011;26:308-22. https://doi.org/10.1093/her/cyr005.
- Azevedo LB, Burges Watson D, Haighton C, Adams J. The effect of dance mat exergaming systems on physical activity and health-related outcomes in secondary schools: results from a natural experiment. BMC Public Health 2014;14. https://doi.org/10.1186/1471-2458-14-951.
- Horner S, Rew L, Torres R. Enhancing intervention fidelity: a means of strengthening study impact. J Spec Pediatr Nurs 2006;11:80-9. https://doi.org/10.1111/j.1744-6155.2006.00050.x.
- Carroll C, Patterson M, Wood S, Booth A, Rick J, Balain S. A conceptual framework for implementation fidelity. Implement Sci 2007;2. https://doi.org/10.1186/1748-5908-2-40.
- Webb TL, Joseph J, Yardley L, Michie S. Using the internet to promote health behavior change: a systematic review and meta-analysis of the impact of theoretical basis, use of behavior change techniques, and mode of delivery on efficacy. J Med Internet Res 2010;12. https://doi.org/10.2196/jmir.1376.
- Carolan S, Harris PR, Cavanagh K. Improving employee well-being and effectiveness: systematic review and meta-analysis of web-based psychological interventions delivered in the workplace. J Med Internet Res 2017;19. https://doi.org/10.2196/jmir.7583.
- Heesch KC, Mâsse LC, Dunn AL, Frankowski RF, Mullen PD. Does adherence to a lifestyle physical activity intervention predict changes in physical activity?. J Behav Med 2003;26:333-48.
- Glasgow RE, Nelson CC, Kearney KA, Reid R, Ritzwoller DP, Strecher VJ, et al. Reach, engagement, and retention in an internet-based weight loss program in a multi-site randomized controlled trial. J Med Internet Res 2007;9. https://doi.org/10.2196/jmir.9.2.e11.
- Verheijden MW, Jans MP, Hildebrandt VH, Hopman-Rock M. Rates and determinants of repeated participation in a web-based behavior change program for healthy body weight and healthy lifestyle. J Med Internet Res 2007;9. https://doi.org/10.2196/jmir.9.1.e1.
- Neve MJ, Collins CE, Morgan PJ. Dropout, nonusage attrition, and pretreatment predictors of nonusage attrition in a commercial web-based weight loss program. J Med Internet Res 2010;12. https://doi.org/10.2196/jmir.1640.
- Wanner M, Martin-Diener E, Bauer G, Braun-Fahrländer C, Martin BW. Comparison of trial participants and open access users of a web-based physical activity intervention regarding adherence, attrition, and repeated participation. J Med Internet Res 2010;12. https://doi.org/10.2196/jmir.1361.
- Murray E, White IR, Varagunam M, Godfrey C, Khadjesari Z, McCambridge J. Attrition revisited: adherence and retention in a web-based alcohol trial. J Med Internet Res 2013;15. https://doi.org/10.2196/jmir.2336.
- Lim S, Norman R, Clifton P, Noakes M. Weight loss and attrition in overweight and obese young women during a 36-week internet-based lifestyle intervention. J Obes Weight Loss Ther 2014;4. https://doi.org/10.4172/2165-7904.1000235.
- Jahangiry L, Shojaeizadeh D, Montazeri A, Najafi M, Mohammad K, Yaseri M. Adherence and attrition in a web-based lifestyle intervention for people with metabolic syndrome. Iran J Public Health 2014;43:1248-58.
- Deci EL, Ryan RM. Facilitating optimal motivation and psychological well-being across life’s domains. Can Psychol 2008;49:14-23. https://doi.org/10.1037/0708-5591.49.1.14.
- Schwarzer R. Modeling health behavior change: how to predict and modify the adoption and maintenance of health behaviors. Appl Psychol 2008;57:1-29. https://doi.org/10.1111/j.1464-0597.2007.00325.x.
- Schwarzer R. Self-Efficacy in the Adoption and Maintenance of Health Behaviors: Theoretical Approaches and a New Model. Washington, DC: Hemisphere Publishing Corporation; 1992.
- Bouton ME. Why behavior change is difficult to sustain. Prev Med 2014;68:29-36. https://doi.org/10.1016/j.ypmed.2014.06.010.
- Wilcox AJ. A positive approach to negative results. Epidemiology 2014;25. https://doi.org/10.1097/EDE.0000000000000022.
- Conn VS, Hafdahl AR, Mehr DR. Interventions to increase physical activity among healthy adults: meta-analysis of outcomes. Am J Public Health 2011;101:751-8. https://doi.org/10.2105/AJPH.2010.194381.
- Zbikowski SM, Hapgood J, Smucker Barnwell S, McAfee T. Phone and web-based tobacco cessation treatment: real-world utilization patterns and outcomes for 11,000 tobacco users. J Med Internet Res 2008;10. https://doi.org/10.2196/jmir.999.
- Glasgow RE, Christiansen SM, Kurz D, King DK, Woolley T, Faber AJ, et al. Engagement in a diabetes self-management website: usage patterns and generalizability of program use. J Med Internet Res 2011;13. https://doi.org/10.2196/jmir.1391.
- Davies C, Corry K, Van Itallie A, Vandelanotte C, Caperchione C, Mummery WK. Prospective associations between intervention components and website engagement in a publicly available physical activity website: the case of 10,000 Steps Australia. J Med Internet Res 2012;14. https://doi.org/10.2196/jmir.1792.
- Krueger RA, Casey MA. Focus Groups: A Practical Guide for Applied Research. Thousand Oaks, CA: Sage Publications; 2000.
Appendix 1 Qualitative process evaluation study context
The majority of participating workplaces had ongoing health improvement programmes or policies, with only one workplace reporting no such programmes or policies in place. Examples of ongoing programmes within the participating workplaces included free health checks, fitness classes, health talks by local charities or other organisations and cycle-to-work schemes. Six participating workplaces explicitly mentioned policies within their organisation relevant to health improvement, with examples including ‘Health and Wellbeing Strategy’, ‘Healthy Eating Policy’ and ‘Staff Welfare policies’. One participating organisation did not have such policies in place. The majority of participating workplaces provided free car parking for staff within walking distance to the workplace.
The majority of participants reported travelling > 1 mile to their place of work (92.9% of participants). The average distance travelled by participants was 10.70 (SD 9.92) miles. Individual perceptions of the workplace environment are shown in Table 35. There was a small but significant difference between intervention and control groups for perceived workplace attractiveness at baseline (p = 0.028).
Perceived workplace measure | Group | |||
---|---|---|---|---|
Intervention | Control | |||
n | Mean (SD) | n | Mean (SD) | |
Workplace attractiveness (4–20) | 438 | 10.17 (2.71) | 377 | 10.63 (3.14) |
Workplace safety (4–20) | 438 | 10.37 (2.47) | 377 | 10.61 (2.68) |
Workplace accessibility (3–15) | 439 | 6.73 (2.14) | 377 | 6.86 (2.08) |
Workplace availability (3–15) | 439 | 5.65 (2.00) | 376 | 5.73 (2.11) |
Workplace environment (14–70) | 437 | 32.90 (6.22) | 376 | 33.81 (7.07) |
Environmental measures, including neighbourhood walkability (for buffers of 200 m, 400 m, 800 m and 1600 m) and distance to the nearest green space, are shown in Table 36. The average distance to the nearest green space across all participating sites was 160 m. See Table 1 for descriptions of the constructs of the perceptions of workplace environment.
Workplace | Neighbourhood walkability | Distance to the nearest green space (m) | |||
---|---|---|---|---|---|
200 m | 400 m | 800 m | 1600 m | ||
Queen’s University Belfast | 4.713929 | 5.589759 | 4.525275 | 6.746569 | 83.88 |
Lisburn City Council | 0.91465 | 1.02877 | –1.4841 | –1.57334 | 25.02 |
Stormont Estate | –3.991 | –4.3998 | –6.93502 | –6.83894 | 93.24 |
Belfast City Hospital | 5.323861 | 5.174881 | 4.492633 | 7.360332 | 299.21 |
Northern Ireland Housing Executive | 0.193904 | –0.03975 | –0.01453 | –0.48188 | 260.72 |
Lisburn Jobs and Benefits Office | 0.21769 | –0.28801 | 0.182381 | –0.70459 | 215.45 |
Northern Ireland Fire and Rescue Service | –0.36682 | 0.10832 | –0.72576 | –0.74107 | 418.31 |
Northern Ireland Environment Agency | 0.32344 | 0.540713 | –0.19459 | –0.67335 | 278.65 |
Wallace High School | –2.62456 | –2.5966 | –1.27271 | –0.40642 | 55.57 |
Friends School | –4.55482 | –4.13064 | –0.18929 | –0.53366 | 36.48 |
South Eastern Regional College | 3.599555 | 1.994418 | 1.088759 | –0.77367 | 85.57 |
Lagan Valley Hospital | –3.74985 | –2.98204 | 0.526789 | –1.37984 | 67.75 |
List of abbreviations
- A&E
- accident and emergency
- ANCOVA
- analysis of covariance
- BCT
- behaviour change technique
- BMI
- body mass index
- CBA
- cost–benefit analysis
- CD
- coefficient of determination
- CEAC
- cost-effectiveness acceptability curve
- CI
- confidence interval
- CUA
- cost–utility analysis
- DBDC
- double-bound dichotomous choices
- DCE
- discrete choice experiment
- EQ-5D
- EuroQol-5 Dimensions
- EQ-5D-5L
- EuroQol-5 Dimensions, five-level version
- FG
- focus group
- GLM
- generalised linear model
- GLS
- generalised least squares
- GP
- general practice
- GPAQ
- Global Physical Activity Questionnaire
- HPQ
- Health and Work Performance Questionnaire
- HRQOL
- health-related quality of life
- HTA
- Health Technology Assessment
- ICC
- intraclass correlation coefficient
- ICER
- incremental cost-effectiveness ratio
- MICE
- multiple imputation of chained equations
- MVPA
- moderate- to vigorous-intensity physical activity
- NICE
- National Institute for Health and Care Excellence
- NIHR
- National Institute for Health Research
- OLS
- ordinary least squares regression
- ORECNI
- Office of Research Ethics Committees Northern Ireland
- PA
- physical activity
- PAL
- physical activity loyalty
- PhD
- Doctor of Philosophy
- PPI
- patient and public involvement
- PSSRU
- Personal Social Service Research Unit
- QALY
- quality-adjusted life-year
- RCT
- randomised controlled trial
- RFID
- radio frequency identification
- ROI
- return on investment
- SD
- standard deviation
- SDT
- self-determination theory
- SE
- standard error
- SEM
- structural equation modelling
- SF-8
- Short Form Questionnaire-8 items
- SRMR
- standardised root mean square residual
- TRIPPA
- TRial of Economic Incentives to Promote Physical Activity
- VAS
- visual analogue scale
- WE
- work environment
- WEMWBS
- Warwick–Edinburgh Mental Wellbeing Scale
- WHO
- World Health Organization
- WTA
- willingness to accept
- WTP
- willingness to pay