Notes
Article history
The research reported in this issue of the journal was funded by the PHR programme as award number NIHR128533. The contractual start date was in February 2020. The draft manuscript began editorial review in October 2022 and was accepted for publication in July 2023. The authors have been wholly responsible for all data collection, analysis and interpretation, and for writing up their work. The PHR editors and production house have tried to ensure the accuracy of the authors’ manuscript and would like to thank the reviewers for their constructive comments on the draft document. However, they do not accept liability for damages or losses arising from material published in this article.
Permissions
Copyright statement
Copyright © 2024 Moore et al. This work was produced by Moore et al. under the terms of a commissioning contract issued by the Secretary of State for Health and Social Care. This is an Open Access publication distributed under the terms of the Creative Commons Attribution CC BY 4.0 licence, which permits unrestricted use, distribution, reproduction and adaption in any medium and for any purpose provided that it is properly attributed. See: https://creativecommons.org/licenses/by/4.0/. For attribution the title, original author(s), the publication source – NIHR Journals Library, and the DOI of the publication must be cited.
2024 Moore et al.
Chapter 1 Introduction
Impact and aetiology (causation) of dental caries
As the most common disease affecting humanity,1 dental caries is a major public health problem with significant costs for both the individual and society. 2 Untreated caries (commonly known as tooth decay) can cause pain, sleepless nights, sepsis, overuse of antibiotics, embarrassment and the loss of productive workdays. As the disease claims progressively more tooth tissue throughout life, its effects are cumulative and can lead to complete tooth loss – one of the leading causes of years lost to disability. 1 Treatment can provoke severe anxiety for some and be an uncomfortable experience for many others. 3 It is also very costly; the NHS in England spends around £3.4 billion per year on dental services, and patients contribute a further £653 million as ‘out of pocket’ copayments. 4 A significant proportion of this spend will be related to the treatment and repair of tooth decay, with more than 9 in 10 adults affected by their mid-30s. 5 As with many chronic diseases, social inequalities exist, and the incidence and severity of decay are strongly associated with low income and deprivation. 6
Dental caries is a dynamic process whereby the fermentation of free sugars by intraoral bacteria creates an acidic environment, leading to dissolution of the hard, inorganic mineral component of dentine and enamel. This dissolution process is called ‘de-mineralisation’. Free sugars include ‘monosaccharides and disaccharides added to foods and beverages by the manufacturer, cook or consumer, and sugars naturally and beverages by the manufacturer, cook or consumer, and sugars naturally present in honey, syrups, fruit juices and fruit juice concentrates’. 7 A dose–response relationship between free sugars and dental caries has been observed at all levels of intake above zero,8 but as a pragmatic goal, the World Health Organization recommends that free sugars should make up < 5% of total daily energy intake. 9 For an adult, this is around six teaspoons of sugar (25 g). Recent estimations of sugar intake in the UK range from 9.4% of energy for adults aged 65 years and over to 12.3% for children aged 11–18 years. 10 In-between times of sugar consumption, when the oral environment returns to a neutral pH, the minerals that were dissolved from the tooth surface can be redeposited from the saliva; this is known as ‘re-mineralisation’. 11–13
The delicate balance of de-mineralisation and remineralisation in the mouth depends mostly on the dietary and oral hygiene behaviours of the individual. If behaviours favour de-mineralisation, the loss of minerals will progress until the tooth surface breaks down and a cavity develops. If remineralisation is favoured, early lesions can be repaired, and even larger cavities can become inactive. Key behaviours include reducing the frequency and amount of sugar consumed, tooth brushing to remove acidogenic bacteria in plaque and the use of fluoridated oral care products, which promote remineralisation. Health behaviours such as these are influenced by the position an individual holds in the socioeconomic hierarchy, which itself is influenced by social and economic policies and culture. 14 Obvious examples of how an individual’s social and economic position may impact caries progression are through material restrictions on their ability to buy healthy food and oral hygiene equipment, but there are many other factors which may be restricted, such as free time, locus of control, self-esteem and coping ability. 14,15 Risk factors for dental caries that are not influenced by behaviours include dry mouth (a common side effect of many medications) and exposed root surfaces due to ageing and periodontal disease.
How fluoride prevents dental caries
Fluoride promotes the remineralisation process and makes remineralised enamel more resistant to de-mineralisation. Fluoride ions are strongly negatively charged, and when they are present in the intraoral fluids after an episode of de-mineralisation, they are adsorbed onto any exposed (positively charged) calcium ions on the tooth surface. The fluoride ions then attract further calcium and phosphate ions from saliva to create a new layer of dental enamel that contains fluoride. The fluoride-containing enamel is less soluble in an acidic environment than the original enamel, and so the tooth is made more resistant to future decay. 13,16 Regular topical exposure to fluoride is required so it can be present in the saliva immediately after an episode of de-mineralisation. When this is the case, fluoride slows down the progression of dental caries at a given level of sugar intake, delaying the formation of cavities and prolonging the life of teeth. 17
History of water fluoridation and other fluorides
The caries protective effect of fluoride was first discovered in the mid-20th century in the USA, where a series of studies throughout the 1930s and 1940s reported that drinking water which contained 1–1.2 mg F/l was associated with a 50% lower caries prevalence. 18–20 Since these pioneering studies, 25 countries across the world have added fluoride to parts of their public water supplies to a concentration of 1 mg F/l. 21 Countries with high population coverage of fluoridation in public water supplies include Singapore (100%), Australia (89%), the USA (74%), the Republic of Ireland (73%) and Chile (65%). 21,22 In the UK, only England currently has any water fluoridation programmes, and only around 10% of the population receive optimally fluoridated water. 21
Following the discovery of the effectiveness of fluoride in water in preventing dental caries, several other methods of applying fluoride to the teeth were developed. From the mid-1970s, fluoride toothpastes became widely available. Today, a range of topical fluoride products are available, including mouth rinses and dentist-prescribed gels, varnishes and higher-strength toothpastes. These topical self-applied or professionally applied products are highly effective, with Cochrane systematic reviews reporting significant reductions in the number of permanent tooth surfaces affected by decay, ranging from 24% [95% confidence interval (CI) 21% to 28%] for over-the-counter fluoride toothpaste,23 27% (95% CI 23% to 30%) for mouth rinses24 and 28% (95% CI 19% to 37%) for gels25 to 43% (95% CI 30% to 57%) for fluoride varnishes. 26 Professional guidance for dental teams in the UK recommends fluoride varnish application at least twice yearly for all children and quarterly for children and adults with additional risk factors, in addition to fluoride mouth rinses and prescription-strength fluoride toothpastes. 27
Fluoride has also been added to salt and milk as upstream delivery alternatives to water fluoridation in some countries, but evidence for the effectiveness of these delivery methods is less compelling than for water fluoridation or topical fluorides, and there are challenges related to implementation of these delivery methods. 28,29
The changing context of water fluoridation and dental disease
Dental caries in permanent teeth is consistently found to be the most prevalent health condition globally, affecting around 35% of the population in the 1995, 2010 and 2015 Global Burden of Disease studies. 30 However, largely because of the availability of highly effective topical fluoride products from the mid-1970s onwards, the epidemiology of this disease has changed substantially over the last 40 years in high-income countries, including the UK. 31,32 In 1973, the first Children’s Dental Health Survey for England and Wales found that by the age of 8, 65% of children already had an experience of decay in their permanent teeth (which would have been present in the mouth for just 2 years). By the age of 15, decay in permanent teeth was almost universal, affecting 97% of children. 33 Due to the chronic, progressive nature of dental caries, children with established decay are more likely to need teeth extracted as adults. In fact, tooth extractions were so common in 1968 that 37% of all adults over the age of 16 had no remaining natural teeth (were ‘edentulous’). 34 The most recent Children’s Dental Survey conducted in 2013 found the proportion of 15-year-olds who had experienced tooth decay in their permanent teeth had more than halved, to 46%. In the most recent Adult Dental Health Survey in 2009, just 6% of adults over the age of 16 were edentulous, demonstrating the significant improvement in oral health since the introduction of fluoride toothpastes. 35
Increased exposure to fluoride in toothpastes, gels, varnishes and mouthwashes has significantly improved oral health in both adults and children, but it is now becoming accepted that fluoride slows down the decay process rather than eliminating it. 36 Tooth decay has evolved from a rapidly progressing disease of childhood which results in early tooth loss, to a slowly progressing disease with the greatest burden experienced by adults. 8,36–39 Tooth loss has a negative impact on quality of life (QoL) and nutritional status. 1,40 The maintenance of natural teeth into old age is rightly celebrated, but the shifting trajectory of dental caries presents other challenges. With the number of people aged over 75 projected to double in the next 30 years,41 the greater burden of caries in older adults is expected to have major implications for dental services. 42 Medication-induced dry mouth, reduced dexterity, exposed root surfaces and reliance on others for mouth care can all drastically increase susceptibility to decay. Furthermore, after a lifetime of repair, the teeth of older people are often heavily filled and fragile (the restorative cycle). Restoring new cavities or replacing old fillings in such teeth can be technically demanding. In patients who may have multimorbidity, polypharmacy and reduced capacity to give consent, even routine dentistry can require specialist skills and facilities.
Community water fluoridation has the potential to offer preventive benefits for all age groups. This presents a significant advantage over targeted fluoride programmes which are delivered to children through schools and nurseries or practice-based interventions delivered by dentists which are costly and available only to regular dental attenders. Most of the research on water fluoridation was carried out at a time before the introduction of topical fluorides, when the primary focus was the prevention of caries in children. Recent evidence on the clinical and cost effectiveness of water fluoridation in contemporary populations who have benefitted from topical fluorides, particularly adults, is sparse.
Literature review: effectiveness of water fluoridation
Systematic reviews
There have been eight systematic reviews of the effectiveness of water fluoridation in preventing dental caries since the year 2000. 43–51 The first was carried out in the UK by the York Centre for Research and Dissemination and was commissioned by the Department of Health. 43 This review was assessed as high quality using the A MeaSurement Tool to Assess Systematic Reviews (AMSTAR 2) checklist for systematic reviews. 52 For the caries-effectiveness research question, 23 controlled before–after studies, 2 prospective and 1 retrospective cohort studies were included. Most studies demonstrated a protective effect of water fluoridation on dental caries in children, but the quality of the studies was low, mainly due to lack of consideration of confounding or effect modifying factors. As a result, in 2002, the UK Medical Research Council (MRC) was tasked by the Department of Health to identify priorities for future research. The recommendations of the working group included:
-
the effect of fluoridation on the dental health of adults
-
the impact of water fluoridation against a background of widespread topical fluoride use
-
economic impacts and the effects on health and well-being beyond the usual measures of decayed, missing and filledteeth (DMFT)
-
the effect of fluoridation on social disparities in dental caries.
The most recent systematic review to carry out a meta-analysis was conducted by Cochrane Oral Health in 2015. 47 The meta-analysis of 10 studies with 39,966 participants estimated that the initiation of water fluoridation was associated with a 26% relative reduction in the number of permanent teeth affected by decay [DMFT was reduced by 1.16 teeth in the fluoridated groups (95% CI 0.72 to 1.61)]. 47 Only prospective studies, with a concurrent control, and outcomes evaluated at two time points (before and after a change in fluoridation status) were included. 47 The quality of this review was assessed as ‘high’ using the AMSTAR 2 critical appraisal tool for systematic reviews. 52 The review authors had limited confidence in the size of the summary effect estimate due to the high risk of bias in most of the included studies. 47 The main issues were lack of consideration of confounding factors and non-blind outcome assessment. Furthermore, most of the included studies were carried out prior to the widespread introduction of fluoride toothpastes; none of the studies included adult participants, and there was insufficient evidence to evaluate the effectiveness of water fluoridation in reducing social inequalities in dental caries experience.
Three of the eight systematic reviews have included some adult participants. 45,49,51 The first was by Griffin et al. in 2007, which aimed to investigate the effectiveness of a range of fluorides for preventing caries in adults (gels, rinses, toothpastes and water fluoridation). 45 For the assessment of water fluoridation, they identified eight cross-sectional studies and one prospective cohort study. 45 A meta-analysis of seven of the cross-sectional studies that had assessed the effect of lifetime exposure found water fluoridation was associated with a 34.6% relative reduction in the number of permanent tooth surfaces affected by decay [decayed, missing and filled surfaces (DMFS)] (95% CI 12.6% to 51.0%). The quality of this review was assessed as being ‘critically low’ using the AMSTAR 2 tool. 52 The main issues were that the review did not include any evaluation of the quality of the primary studies or whether confounding was adequately accounted for. Additionally, the fluoride concentration of three of the studies included in the meta-analysis was above the maximum permitted level of 1.5 mg F/l in the UK which limits the applicability of the findings to the UK context, and some of the included studies were conducted in the pre-fluoride toothpaste era when caries levels were much higher. Furthermore, relative percentages can be misleading, as they make the difference seem larger than it is in clinical terms, particularly with such a finely graded outcome as tooth surfaces.
Two further systematic reviews that included adult participants were carried out on behalf of the Australian National Health and Medical Research Council (NHMRC) in 201649,50 and the Canadian Agency for Drugs and Technologies in Health (CADTH) in 2019. 53 The NHMRC review was an update to an earlier 2007 review but with much broader inclusion criteria. 44 The inclusion criteria for both reviews permitted any study design evaluating water fluoridation at concentrations of between 0.4 and 1.5 mg F/l that had conducted multivariable analysis to control for confounding (confounders not specified). Both reviews assessed dental and other health outcomes and were part of a wider examination of water fluoridation which also included ethical considerations53,54 and, in Canada, cost effectiveness. 55 The Canadian review included all of the systematic reviews45,47,56 and primary studies identified by the Australian review, a 2016 systematic review of the cessation of water fluoridation48 and any relevant primary studies published after the search period of the Australian review. The quality assessment for the Australian review50 using the AMSTAR 2 tool is ‘moderate’ and ‘high’ for the Canadian review. 51 Both reviews presented their findings as narrative summaries rather than meta-analyses due to the heterogeneous nature of the included studies. 50,51
The primary studies which included adult participants identified by the NHMRC review assessed caries57,58 and tooth loss. 59–62 Additional adult studies included in the Canadian review assessed caries severity (DMFT/S),63–66 caries prevalence,67 tooth loss68,69 and dental service use. 70,71 Of these 15 primary studies, 9 were assessed as being of ‘acceptable’ quality by the review authors and 7 as ‘low’ quality. 49,51 The studies were ecological57–62,65,67,70,71 and cross-sectional. 64–66,68 The authors of the Australian review concluded that the quality of the evidence was ‘low or very low’, but it was consistent and ‘indicates that water fluoridation improves the dental health of children and adults’. 50 The Canadian review also concluded that while the quality of the evidence was mixed, there was ‘consistent evidence that water fluoridation reduced caries in children and adults’, despite ‘widespread availability and use of fluoride toothpastes’. 51 With regard to other dental outcomes that included adult participants, the Canadian review authors found insufficient evidence for an association between water fluoridation and dental service use, limited evidence for reducing tooth loss and limited evidence for a reduction in social disparities. 51
In their recommendations for future research, the authors of the Canadian review noted that while the majority of studies identified for the caries outcomes were ecological or cross-sectional, these designs may be sufficient as long as appropriate adjustment for confounding is undertaken. 51 This is a view echoed by others who have highlighted that the quasi-experimental study designs specified as an inclusion criterion for the Cochrane systematic review were only possible during the initial implementation phase of water fluoridation (i.e. controlled before–after evaluations). 57,72 Most water fluoridation programmes were implemented in the 1950s and 60s, limiting the opportunities for such studies in more recent times. Adequately controlled cohort or cross-sectional studies have been suggested as more appropriate study designs in contemporary populations where it may not be possible to evaluate a change in water fluoridation status. 57,72 The Canadian review authors recommended that future research assess the impact of water fluoridation on reducing disparities in oral health and include populations that are varied in age, socioeconomic status, ethnicity and migration status. 51 The importance of conducting studies that can be generalised to the relevant national context was also noted.
Recent primary studies of the effectiveness of water fluoridation in adults and adolescents
The studies reviewed in this section are those 15 studies with adult participants, which were included in the 2019 CADTH review, as well as 4 studies published after the search end date of that review (December 2018). The search terms used to identify recent primary studies not included in the CADTH review were (Adult or Adolescent or Older or Elderly) AND (Caries or Decay or Tooth Loss or Dental Treatment) AND water fluorid$. The full search history is available in Appendix 1. The databases searched included: MEDLINE, EMBASE and all evidence-based medicine reviews (includes Cochrane and NHS Economic Evaluation Database). The search time frame was 1st of Jan 2018 to 21st of June 2022.
The identified studies were published between 2009 and 2022. The majority were carried out in Brazil59,61,64,67–69,73,74 and Australia. 57,58,60,63,65,66 All of the studies were of cross-sectional or ecological design. Most of the Brazilian studies were secondary analyses from the 200359,61 and 201067,68,73 SB Brazil national surveys of oral health, while two were the same cohort of participants at different time points64,69 and one was a school-based sample. 74 Four of the Australian studies were secondary analyses of the Australian National Survey of Adult Oral Health (ANSAOH) 2004–6,57,60,63,66 while one examined young offenders58 and one was an opportunistic follow-up of a childhood study sample. 65 Three of the studies utilised dental service data from South Korea,71 the USA75 and Sweden. 70 One study included Canadian Army recruits,76 and one was a secondary analysis of data from the telephone-based US Behavioural Risk Factors Survey 1995–9. 62 The outcomes assessed included self-reported number of lost teeth,62,69 clinically examined number of lost teeth,59,61,68,73 clinically examined prevalence and severity of decay (DMFT/S)57,60,63–67,74,76 and dental visits and/or treatments received. 70,71,75
All of the Brazilian studies reported that greater exposure to water fluoridation resulted in improved oral health, and the size of the differences between fluoridated and sub/non-optimally fluoridated groups was relatively large in comparison to the studies from Australia or the USA. For example, in the SB Brazil 2010, adults aged 35–44 living in sub/non-optimally fluoridated areas had a 27% higher mean ratio of missing teeth (MR 1.27, 95% CI 1.11 to 1.45). 73 In absolute terms, this was a large difference of three teeth per person, comparing the fluoridated and sub/non-optimally fluoridated municipalities,73 reflecting the high prevalence of missing teeth in this population. Another study which examined the SB Brazil 2010 data for 12–19-year-olds found that those living in sub/non-optimally fluoridated municipalities had 42% increased odds of having at least one decayed tooth (OR 1.42, 95% CI 1.08 to 1.86) and 57% increased odds of having at least one missing tooth (OR 1.57, 95% CI 1.16 to 2.14). 67 Both of these studies benefitted from calibrated examiners and a multilevel analysis which included a range of individual-level and contextual variables. 67,73
The Australian studies all found that increased exposure to water fluoridation resulted in fewer teeth affected by decay (DMFT/S) in younger adult age groups, but there were conflicting results for older adults. 57,58,60,63,65,66 The studies by Slade et al. (2013) and Do et al. (2017) both used data from the complete age range of participants in the ANSAOH 2004–6, but took a different analytical approach and reported different results. Slade et al. found those born pre-1960 who had more than 75% lifetime access to fluoridated water had 10% lower DMFT and 30% lower DFS (note ‘M’ component excluded) compared to those with < 25% lifetime access. In the cohort born 1960–90, for the same comparison, DMFT was 11% lower and DFS was 21% lower. 57 The estimates for the pre-1960 cohort are at risk of residual confounding due to age because of the relationship between per cent lifetime access to fluoridated water and age. That is, those in the pre-1960 cohort with > 75% lifetime access to fluoridated water were younger than those with a lower per cent lifetime access (due to the timing of water fluoridation implementation across Australia), but adjustment for age was only made by decade. Therefore, the analysis by Do et al. is preferred. 66
In the Do et al. study, adults aged 15–34 years of age who had the highest quartile of per cent lifetime access to fluoridated water had a 33% lower mean ratio of DMFS, compared to those with the lowest quartile of lifetime access (MR 0.67, 95% CI 0.48 to 0.92). 66 In the 35–44 age group, the mean ratio of DMFS scores was 22% lower (MR 0.78, 95% CI 0.66 to 0.93), but no difference was found in the age groups 45–54 and over 55. 66 In absolute terms, this is a difference of 3.1 tooth surfaces per person in the 15–34-year-olds and 5.7 surfaces per person in the 35–44-year-olds. These estimates were adjusted for age, sex, residential location, dental visit pattern, toothbrushing frequency, household income and oral hygiene. 66 The authors proposed that the absence of effect in the older age groups may be due to lack of variation in water fluoridation exposure in this age group and saturation of the DMFS score due to new caries being most likely to occur on previously treated surfaces (which would not increase the DMFS count). The main quality issue affecting the studies using ANSAOH data is that a large proportion of the original participants were excluded as a result of missing residential history information (26–31%, depending on the study). 57,66 An Australian follow-up study of young adults who had taken part in an earlier childhood study found that 20–35-year-olds with 0–74% lifetime access to fluoridated water had 26% higher rate of DMFS compared to those with 100% lifetime access [incidence rate ratio (IRR) 1.26, 95% CI 1.01 to 1.57],65 a difference of 1.6 tooth surfaces per person in absolute terms. 65 Finally, an Australian study of young offenders aged 13–21 found that those whose usual place of residence was sub/non-optimally fluoridated had a 77% higher rate of DMFT compared to those who usually resided in fluoridated areas (IRR 1.77, 95% CI 1.11 to 2.83). In both these studies, estimates were adjusted for important confounders such as age, sex and measures of socioeconomic status. 58,65
The Canadian study of Army recruits aged 16–45 found those recruited from areas with water fluoridation had a mean rate of DMFT that was 11% lower than the mean in recruits from sub/non-optimally fluoridated areas, after adjusting for age and gender [a difference in means of 0.67 fewer teeth (95% CI 0.79 to 0.55)]. 76 The mean rate of DMFS was 14% lower in those recruited from fluoridated areas [an absolute difference in means of 1.77 fewer tooth surfaces per person (95% CI 2.09 to 1.46)]. This study also evaluated if the effect of water fluoridation differed according to county-level income and education. No significant differences were identified, but the study population may not have had enough variation in terms of county-level income and education to fully examine this as all participants were Army recruits. 76 The US study of self-reported tooth loss in adults found those with a 100% probability of being exposed to water fluoridation at birth had 0.26 more teeth per person than those with a 0% probability (based on year of birth, county-level implementation date and population coverage of water fluoridation). 62 When stratified by social factors, the difference in the number of teeth was larger for black people (0.37 more teeth per person), those without a high-school diploma (0.61 more teeth per person) and high-school graduates (0.39 more teeth per person), whereas it was smaller for white people (0.18 more teeth per person) and was no longer statistically significant for college graduates (0.06 more teeth per person). 62 A range of county and individual-level covariates were included in the models for these estimates, including age, gender and education. 62 This is the only identified adult study to suggest water fluoridation may have a larger effect in more disadvantaged groups. 62
Three studies used routinely collected dental service monitoring data. 70,71,76 A South Korean study used National Health Insurance data to explore the effect of regional water fluoridation status in 2013 on dental outpatient visits between 2003 and 2013. 71 Patient records were obtained from a randomly selected National Health Insurance Sample Cohort. Patients were included in the analysis if they lived in non-metropolitan areas and had not had a caries diagnosis prior to 2003. The analytical sample was 472,250, and the average years of follow-up time was 9.12 years. Confounders included patient age, sex, income, health insurance funding bracket and dentist: population ratio and local government financial independence rate. The adjusted models found that patients living in fluoridated regions had a 5% lower risk of dental care visits (HR 0.95, 95% CI 0.93 to 0.97) over 9.2 years. The mean survival time to the first diagnosis of dental caries was 16 days longer in fluoridated regions (p < 0.0001). In those patients who did have a dental care visit, the number of visits per person was marginally lower in the fluoridated regions (β = −0.029, p = 0.04), but there was no difference in the costs of dental care. 71 This study illustrates that with the very large sample sizes afforded by routinely collected data sets, even very small differences will be statistically significant.
A Swedish study of adults aged 16 and older investigated the effect of lifetime water fluoridation exposure on aggregated area-level dental service data for 2008 and 2013. 70 The authors estimated in a regression analysis that if the fluoride level in a municipality increased by 1 mg F/l, the proportion of 20-year-olds who had a tooth repair in any given year would reduce by 0.6 percentage points (p < 0.01). 70 The regression included data from Swedish registers to allow for adjustment of confounders including sex, marital status, parent’s education, parent’s income, father’s cognitive and non-cognitive ability, parental immigration status and cohort mean education. A study of Medicare claims for children and adolescents aged 0–18 in Alaska was conducted to provide a comparator75 for an earlier study where water fluoridation had ceased. 77 The earlier study found that after the cessation of water fluoridation, the mean number of caries-related dental procedures per child aged 13–18 increased by 0.29 from 2003 (pre cessation) to 2012 (post cessation), and the costs increased by $145 per child. 77 The comparator study found that in a neighbouring area with similar census characteristics and ongoing water fluoridation, the mean number of caries-related dental procedures per child aged 13–18 decreased by 0.12 compared to 2003 and 2012, and the costs per child increased by $68.61. 75 The pre–post estimates in both studies were adjusted for sex, race and inflation, but as the studies were reported separately, no consideration of the effect of differences between areas was possible.
In summary, recent studies of the effectiveness of water fluoridation in adolescents and adults have found that water fluoridation is associated with improved oral health in adults aged up to 45 years. Most of the studies identified conducted multivariable analysis with consideration of confounders including a minimum of age, sex and socioeconomic status; only two studies did not. 75,76 However, much of the recent evidence comes from contexts which are not comparable to the UK and may have higher levels of dental disease and unmet treatment needs, including those studies conducted in Brazil59,61,64,67–69,73,74 and those with specific population subgroups such as young offenders or Army recruits. 58,76 The studies which are most applicable to the UK general population (i.e. Australia, the USA and Sweden) have demonstrated absolute differences so small that whether they are meaningful from the perspective of patients, public and clinicians is debatable. Additionally, there remains little evidence of the effect of water fluoridation on the oral health of older adults and its role in reducing social inequalities in adult oral health.
The NIHR CATFISH study: an evaluation of a water fluoridation scheme in Cumbria
A landmark 7-year prospective cohort study on the effectiveness and cost effectiveness of water fluoridation for preventing dental caries in children has recently been completed in the UK and is mentioned here because it is a contemporary, large UK study funded by National Institute for Health and Care Research (NIHR) and therefore highly relevant to the LOTUS study. 78 This study was made possible because of the replacement of water fluoridation dosing equipment after several years of inactivity. This created an opportunity to study the effects of reinstating the programme as if it were a new scheme. The study design was a controlled before-and-after study, with the start of the study being < 1 year since the change in fluoridation status. Two cohorts of children were followed, one from birth to 5 years and one from 5 to 11 years. The two cohorts allowed the effects of fluoride at different time points to be tested. There has long been debate over whether there is an important systemic effect of water fluoridation or whether the effect is primarily topical. For a systemic effect, fluoride must be present in the tissues when the teeth are developing in the jaws. For the deciduous (baby) teeth, this occurs from 30 weeks in utero up to 12 months of age. 79
This study found that, after adjusting for deprivation, age and sex, 5-year-old children who were exposed to fluoridated water from before birth (n = 1444) had 26% reduced odds of developing decay [odds ratio (OR) 0.74, 95% CI 0.56 to 0.98]. In absolute terms, the prevalence of any decay was 17.4% in children who had received fluoridated water and 21.4% in children who received sub/non-optimally fluoridated water. For the older cohort (n = 1192), while there was a lower prevalence of decay in the fluoridated group (19.1%) compared to the sub/non-optimally fluoridated group (21.9%), the 95% CI for the OR included the possibility of no effect (OR 0.80, 95% CI 0.58 to 1.09). There was no evidence of water fluoridation reducing the effect of social deprivation on either the prevalence of decay or the number of teeth affected by decay (DMFT, secondary outcome). The economic evaluation found that in the birth cohort there was a high probability of water fluoridation being cost-effective at a willingness-to-pay threshold of £20,000 per quality-adjusted life-year (QALY).
For comparison of effect sizes, the 2015 Cochrane systematic review meta-analysis estimated an absolute reduction in the prevalence of decay in deciduous teeth of 15% (95 CI 11% to 19%). 47 The Cumbrian Assessment of Teeth a Fluoride Intervention Study for Health (CATFISH) study authors concluded both the prevalence of decay and the size of the effect of water fluoridation were much smaller in this contemporary UK population than previously reported, and the clinical relevance of such a difference would need to be considered by policy-makers and the public. This study was designed and conducted in line with guidance on quality in water fluoridation studies and is highly relevant as it was carried out in the UK in a contemporary population. However, the shifting epidemiology of tooth decay into older age groups and the chronic long-term progression of this disease throughout life may mean the cohorts of young children included in this study were yet to experience the greatest risk of disease and therefore the greatest potential benefit of water fluoridation.
Conclusions and rationale for the LOTUS study
There have been some well-conducted observational studies of the effect of water fluoridation on the dental health of adults in recent years. However, much of this evidence comes from contexts that are not applicable to the UK (or indeed, other countries with low caries prevalence) in terms of disease levels and access to dental services. Studies which have been conducted in more applicable contexts have shown relatively small effect sizes or have been conducted with very specific populations, limiting their generalisability. Several of the key questions posed by the MRC in 2002 remain. Particularly, the effect of water fluoridation on the dental health of adults, the impact of water fluoridation on health and well-being outcomes that are meaningful to patients, the economic impacts of water fluoridation for the whole population and the impact on social disparities in oral health.
There are several reasons why most studies on water fluoridation to date have only included children. The first is that many of the water fluoridation studies were carried out before the introduction of topical fluorides, when the primary focus for prevention was to limit the extensive disease that was almost universal by adolescence. Secondly, from a practical perspective, it is much more difficult, and therefore costly, to recruit large numbers of adults for a clinical examination study. Children can be relatively easily accessed through school settings, but no comparable setting for the whole adult population exists. Thirdly, the most commonly used epidemiological measures of dental decay (DMFT and DMFS) are less sensitive in older people. 66 This is because the most susceptible teeth and tooth surfaces often experience recurrent decay after previous treatment. Recurrent decay results in more extensive cavities, larger dental fillings and weaker teeth, but it would not increase the count of the number of decayed teeth (DMFT) or surfaces (DMFS). Similarly, if a tooth that was previously filled is extracted due to further decay, the total DMFT count does not increase. In older people, it has been shown that the number of teeth that are missing is a more sensitive measure of social inequalities in oral health, but the causative role of periodontal disease in tooth loss increases from middle age, making tooth loss due to caries more difficult to identify. 6,80 Finally, gathering accurate information on where adults have lived throughout their lives, in order to determine their exposure to fluoridated water, is more challenging than it is for children, primarily due to the longer recall periods involved. Inability to obtain a complete residential history has resulted in large numbers of exclusions in recent population-based studies of the effectiveness of water fluoridation for adults. 60,66
The present study was designed with the recommendations of the MRC as well as the practical and methodological complexities outlined above in mind. The use of routinely collected NHS dental treatment data offered the opportunity to access information on the dental health and resource use of large numbers of adults from a wide cross section of society. This allowed us to examine the influence of water fluoridation on social inequalities in oral health. The fact that the data had already been collected meant the costs of conducting this study were much lower than a standard clinical epidemiological study, the time to obtain results was much shorter than for a prospective study of the same observation period and the problems of responder bias and loss to follow up were avoided. Utilising data on NHS dental treatments also offered the potential to avoid the ceiling effect encountered when using only the DMFT index to measure oral health in older adults. Repeated repairs to old fillings and an increased risk of secondary decay in previously filled teeth is one of the lifetime consequences of decay,81 but it is not captured by the standard epidemiological indices. Relatedly, measuring the impact of water fluoridation on NHS treatment costs necessarily requires real-world evidence. The use of a large data set also enables a larger number of potential confounding variables to be accounted for through statistical methods than when using a research-specific data set which would inevitably be much smaller due to the costs associated with data collection.
When designing the study, we spoke to NHS dental patients and the public about what was important to them in terms of oral health and preventing tooth decay. The majority referred to wishing to avoid uncomfortable and anxiety-provoking dental treatments that necessitated the use of local anaesthetic injections or ‘the drill’. They were also concerned about losing teeth and the potential impact that would have on their QoL, particularly in terms of eating and smiling. Avoiding the costs of dental treatment was also important to those who paid for their care. A recent analysis found the NHS dental charge for a course of treatment that includes any fillings or extractions (band 2) was 18.3% of the mean weekly gross household disposable income for those living in the most deprived 20% of neighbourhoods in England in 2018–9. 82 For any crowns or dentures, the NHS charge (band 3) rose to 79.4% of weekly gross disposable household income. 82 Our choice of outcome measures was informed by these conversations.
For our primary outcome, we chose the number of ‘invasive dental treatments’ received per person over 10 years. This includes any treatment on a tooth that is an immediate or longer-term consequence of tooth decay and would necessitate a local anaesthetic injection and/or the dental drill, for example, fillings, extractions, root canal treatments and crowns. We also included veneers because while the numbers provided by the NHS are expected to be very low, they are sometimes provided due to fluorosis (a cosmetic side effect of too much fluoride). Secondary outcomes are the number of natural remaining teeth, the total DMFT index as recorded from the dental charting and the cost per episode of invasive dental treatment avoided, for both patients and the NHS. Using these outcomes means we can address the MRC recommendation of using outcome measures that are meaningful to patients as well as dental professionals.
Chapter 2 Aim and objectives
Aim
Aim: To pragmatically assess the clinical and cost effectiveness of water fluoridation for preventing dental treatment and improving oral health in a contemporary population of adults, using a natural experiment design.
Objectives
The primary objective was to:
-
Compare the effect of 10-year exposure to fluoridated water with no exposure, on the number of invasive dental treatments received by adults attending NHS dental practices, over the same 10-year period.
The secondary objectives were to:
-
Compare the impact of 10-year exposure to community water fluoridation with no exposure on the oral health of adults attending NHS dental practices.
-
Measure the impact of 10-year exposure to water fluoridation on social inequalities in the oral health of adults attending NHS dentists.
-
Evaluate the cost effectiveness of water fluoridation in reducing the amount of invasive dental treatment in an adult population, taking a public sector perspective.
-
Estimate the return on investment (ROI) from a public sector perspective in terms of the change in the cost of providing dental treatments generated from an investment in water fluoridation.
For the purposes of this study, ‘adults’ includes adults and adolescents who have their adult, permanent teeth. This occurs from 12 years of age. 79
Chapter 3 Methods
Study design
The study is a pragmatic, retrospective cohort study using routinely collected NHS dental treatment claims data. These data were provided by the NHS Business Service Authority (BSA), which are responsible for processing all NHS dental treatment claims data from primary care dental services in England and Wales.
Study inclusion and exclusion criteria
Participants
Adults and adolescents aged 12 years and over, attending NHS dental practices in England in the last 10 years (prior to NHS BSA selecting the study cohort from within the database).
Adolescents aged 12 years and over were included as well as adults, as this is the age at which the permanent, adult teeth are usually present in the mouth (except for third molars or ‘wisdom teeth’). This age was also chosen to provide a useful continuation of information, following on from the cohort included in the (at the time, ongoing) NIHR PHR CATFISH study of water fluoridation in children.
Inclusion
-
Dental records that can be assigned to a unique individual using the combination of NHS BSA identifier (initial, surname, gender, date of birth) and NHS number.
The inclusion criteria were important because of the way that these data are stored within the NHS BSA database (as discreet claims relating to single courses of treatment rather than patients). The older claims (2010–5) in the NHS BSA database did not include the patient’s NHS number but rather used an NHS BSA patient identifier (made up of a combination of surname, first initial, date of birth and sex). These identifiers cannot be considered as unique at the national level, therefore a one-to-one match between BSA ID and NHS number was required to ensure all claims related to a single individual. Around 70% of the claims submitted into the new (post 2015) NHS BSA database can be assigned an NHS number. Further detail on this linkage process is provided in Data linkage and validation.
Exclusion
-
Individuals were excluded from further analysis if they did not have at least two episodes of dental attendance within the 10-year observation period.
The reason for this exclusion criteria was that we wanted to have at least two time points for information on residential address to strengthen the assignment of exposure to water fluoridation.
Selection of the study population by National Health Service Business Service Authority
In studies using routinely collected data, it is important to be clear about the source population, database population and study population. These are described below and illustrated in Figure 1.
FIGURE 1.
Diagram illustrating the relationship between the source, database and study populations.
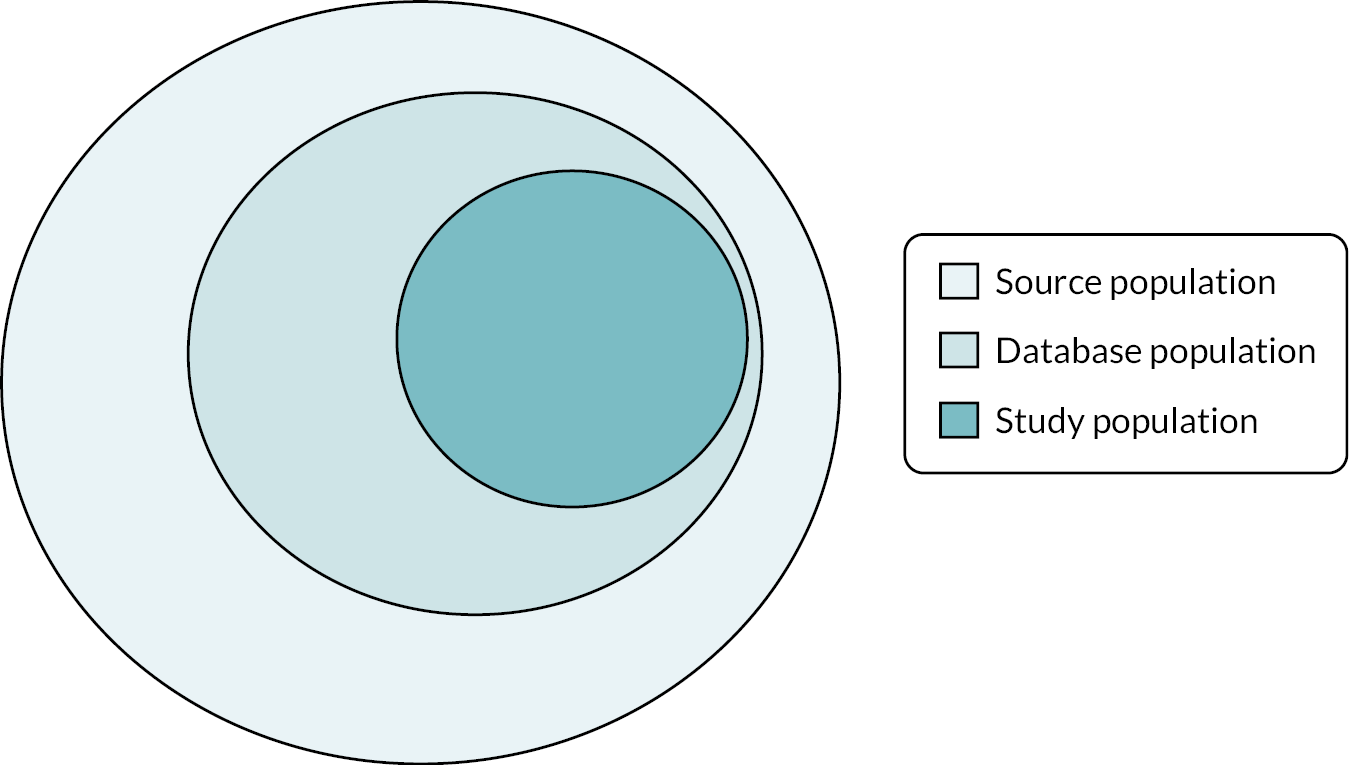
Source population
Users of NHS primary care dentistry services in England and Wales. This includes data on patients attending a range of different types of dental services: general dental services (GDSs; high-street practices), community dental services, prison dental services, urgent and out-of-hours services, specialist primary care referral services (oral surgery/sedation/orthodontics) and domiciliary care (for housebound patients).
Database population
Users of NHS primary care dentistry services in England and Wales between 22 November 2010 and 21 October 2020.
The retention period for NHS BSA dental data is 10 years, and the data were downloaded on 21 October 2020. The study team did not have access to the NHS BSA database population. The study population was selected by NHS BSA.
Study population
Users of NHS primary care dentistry services in England and Wales between 22 November 2010 and 21 October 2020, meeting the study inclusion and exclusion criteria, as well as NHS BSA selection criteria (Figure 2) and transferred to The University of Manchester. As per the s251 support from the Health Research Authority’s Confidentiality Advisory Group (CAG), all patients with an NHS data opt-out were excluded from the study population by NHSSA. NHS BSA also removed any treatment claims that related solely to orthodontic care.
FIGURE 2.
Summary of the pathway into the study population.
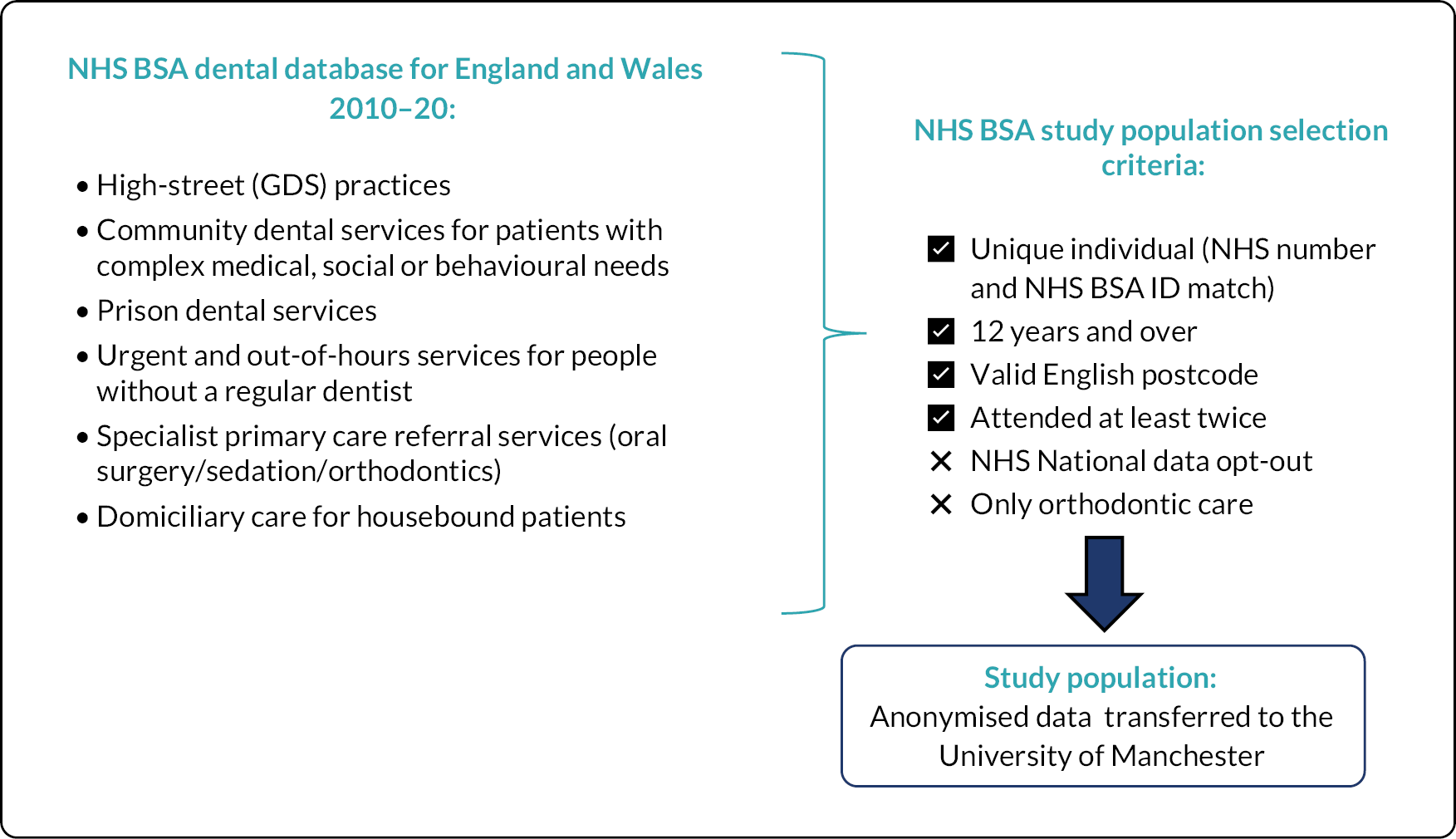
A schematic illustration giving an overview of pathway for inclusion in the study population is provided in Figure 2.
Data collection
Water fluoride concentration data
Routinely collected drinking water quality monitoring data were used to create the patient-level fluoride exposure variable.
In the original research proposal, we planned to use a binary postcode look-up table detailing which areas in England are ‘flagged’ as having a water fluoridation programme. We requested data on the coverage of water fluoridation and water fluoride concentrations from the Drinking Water Inspectorate (DWI) and Public Health England (PHE). Neither PHE nor DWI was able to provide a binary indicator of the coverage of water fluoridation programmes at a small area level, and they advised us to contact the water suppliers directly. We contacted the 24 water suppliers listed by the water regulator (Ofwat) in England and Wales. 83 The water companies did not have postcode-level mapping of fluoridation status or an indicator of which areas were intended to be included in a water fluoridation programme. Therefore, it became necessary to map water fluoride concentrations ourselves using water quality samples for fluoride.
Water quality regulations in England and Wales stipulate that water companies must monitor the concentration of fluoride to ensure it does not exceed the maximum permitted value of 1.5 mg F/l. 84 The drinking water network is mapped using water supply zones (WSZs) rather than standard UK census-based geographies. A WSZ is an area within which the water supply is of a ‘similar nature and treatment’ and the population supplied does not exceed 100,000. 84 Water quality samples are drawn from randomly selected households (customer taps/faucets) within a WSZ, or, if the nature and treatment of the water are the same, they can be taken from supply points higher up in the water distribution network. The number of samples that are taken in each WSZ in each year is correlated with population size, with more frequent samples taken in more populous areas. 84,85 The standard sampling frequency for fluoride is 8 samples per year, per 5000 population, per WSZ. 84 Minimum standards apply in relation to the sampling methodology and the accuracy of water quality samples (ISO 17025 and the Drinking Water Testing Specification). 86
We requested sample data on water fluoride concentration (mg F/l) for every WSZ, for every year from 2009 to 2020 under the provisions of the Environmental Information Regulations (2004). Requests were made through the ‘What Do They Know’ website87 and via direct e-mails between March 2020 and November 2020. We requested that the sample data be supplied as Microsoft Excel files containing supply zone names and zone codes. When consumer tap samples were unavailable, we requested supply point samples of water fluoride data and a corresponding list of WSZs supplied by these supply points. We sought raw sample data where possible, rather than the annual summaries. To enable the WSZ data to be mapped onto standard UK census-based small area geographies [lower super output areas (LSOAs)], we also requested geographic information system (GIS) shapefiles (maps) of the WSZ boundaries for every year from 2009 to 2020. GIS shapefiles show the WSZ boundaries as polygons. A polygon is a series of X (easting) and Y (northing) co-ordinate pairs that enclose a geographical area.
In total, we submitted 18 requests through the ‘What Do They Know’ website87 and sent 74 direct e-mails. In some cases, our initial requests were refused, with reasons cited including concerns about the potential for terrorist attacks on the water supply, samples being considered as the personal data of consumers and unreasonable burden or information not held. In such cases, we then proceeded to request an internal review and, in one case, made a referral to the Information Commissioner’s Office. One water company insisted that we sign a non-disclosure agreement to ensure that we did not publish the data in relation to WSZ geographies, whereas the others supplied the data as publicly available, in line with the EIR provisions.
Dental treatment and oral health data
Routinely collected NHS BSA dental treatment claims data were used to provide the study population and outcomes data.
These data were collected by the NHS BSA from primary care dental practices contracted to provide NHS dentistry within England and Wales between October 2010 and November 2020. NHS BSA is an executive non-departmental public body of the Department of Health and Social Care (sometimes known as an arms-length body). The BSA is responsible for monitoring dental patient charges, processing clinical activity against dental contracts and making payments to dentists in line with their NHS dental contract arrangements. The BSA also has a role in monitoring population-level access to dental services and guarding against fraudulent claims. 88
Data on the number and type of NHS dental treatments provided during each course of treatment are sent to NHS BSA within 2 months of the completion of a course of treatment. A ‘course of treatment’ is defined as all treatment that is deemed to be clinically necessary and which the patient consented to after each new dental examination. The claims for each course of treatment are submitted to the BSA using a form known as the ‘FP17’. These claim forms are completed electronically, and the required data are automatically populated using information from the dental electronic patient record (EPR) software.
Setting
National Health Service dental care
National Health Service dental care includes any treatment the dentist believes is clinically necessary to achieve and maintain good oral health. 89,90 It does not include treatments that are purely cosmetic, for example, teeth whitening. It also does not include more aesthetic treatment options where a conventional treatment option would be better from the perspective of maintaining oral health. An example of this would be a bonded porcelain crown on a molar tooth that is more aesthetic but involves removing more tooth tissue than a conventional (thinner) metal crown. Patients may opt to pay for cosmetic treatments or more aesthetic treatment options on a private basis, and it is permitted for dentists to mix NHS and private treatments on the same patient as part of the same course of treatment. Private dental care is not reported to NHS BSA and is therefore not recorded in the NHS dental treatment claims database.
Types of dental services
There are a range of different types of primary dental care services which submit FP17 treatment claims to the NHS BSA. Patients attending the following types of services are included in the study population data set.
General dental services
The majority of NHS primary dental care is provided in general dental practices who are contracted to provide ‘mandatory services’. This includes all routine dental care including examination, radiographs, treatment planning, prevention, conservative treatments (e.g. fillings, root canal treatments and crowns), surgical treatments, including extractions and periodontal treatments (for gum disease). 91 This type of general dental care is also provided in prisons and young offender facilities, and data from these settings are included in the study data set.
Advanced primary care dental services
Technically challenging dental care may be provided by primary care practices which hold a contract to provide ‘advanced mandatory services’ on a referral-only basis. This includes treatments that require additional skills, training and facilities, for example, complex tooth extractions or root canal treatments, minor surgical procedures, intravenous or inhalation sedation or domiciliary dental care. Once the specific treatment has been completed, the patient usually returns to the general dental practice that originally made the referral for their continued routine care.
Community dental services
Community dental services are a ‘special care’ dental service for patients with medical, social or behavioural needs that make their treatment more complex to provide. This may include patients with severe learning disabilities, autism or medical conditions that complicate dental treatments, for example, bleeding disorders or a compromised immune system. Medically compromised patients requiring domiciliary care or patients with physical disabilities that mean they need special equipment to receive care, such as hoists, wheelchair tippers or NHS ambulance transport services, may also be seen. 92 Other vulnerable groups, such as people experiencing homelessness, people with substance use disorders and children in care may also be treated depending on local arrangements. Patients may be referred for a single course of treatment or, depending on their needs, they may receive all their dental care within the community dental service.
Urgent dental care services
Urgent dental care services are contracted to provide in-hours and out-of-hours (evenings, weekends, bank holidays) dental care for people who do not currently have a dentist (in-hours) or whose dental practice is not currently open (out-of-hours). They provide one-off emergency care to relieve pain and infection.
National Health Service contracting and payment systems for primary care dentistry
National Health Service dental care is provided by independent contractors who hold a contract to provide an agreed scope and volume of NHS dental care. The three types of contracts that are currently in use are described below.
-
General dental services contract 2006. 91
This is the most frequently used type of dental contract and is the type used by most ‘high street’ general dental practices. 93 Contract holders must provide all ‘mandatory services’ and this clinical activity is commissioned and monitored using units of dental activity (UDAs). 91 The contract holder receives an agreed annual financial payment (total contract value) in return for providing a pre-specified number of UDAs. The total contract values and the target number of UDAs to be delivered are negotiated individually for each practice and are based on historical funding and activity levels. 94 Therefore, the financial value of 1 UDA varies between contracts (practices). The mean value of 1 UDA for GDS contracts in the financial year 2020–1 was £28.64. 95
When this contract was introduced in 2006, over 400 individual treatment fee-for-item treatment codes were replaced with just three ‘bands’ of treatment complexity for each course of treatment:
-
A band 1 course of treatment (examination, prevention, radiographs) attracts 1 UDA.
-
A band 2 course of treatment (all care in a band 1 course of treatment plus any number of direct restorations, endodontics and/or extractions) attracts 3 UDAs.
-
A band 3 course of treatment (all care in a band 1 and/or band 2 course of treatment plus any laboratory work including indirect restorations and dentures) attracts 12 UDAs.
The UDA system does not consider the number of treatments provided within each band. 94 For example, a course of treatment containing one direct restoration accrues the same number of UDAs as a course of treatment containing three direct restorations, two molar endodontic treatments and an extraction.
It is important to note that practices working under this contract may also provide services that are commissioned and monitored using metrics other than UDAs. For example, orthodontics, domiciliary care, sedation, dental public health or advanced mandatory services. Orthodontic courses of treatment are commissioned using units of orthodontic activity (UOAs), and for other services, specific key performance indicators (KPIs) may be outlined in a service level agreement accompanying the main contract. Example KPIs might include the number of clinical sessions to be provided, the number of patients to be treated or the maximum waiting times. This feature means it is not possible to determine the real-term value of contracts using only the total contract value and number of commissioned UDAs.
-
-
Personal dental services (PDS) contract 2006. 96
This type of contract is more commonly used for more specialised dental services that provide care on referral and even some secondary care services, such as treatment under general anaesthesia. 97 Services working under this type of contract may have a nominal number of UDAs to deliver, but they are more likely to be commissioned and monitored according to KPIs.
-
Personal dental services plus (PDS+) contract 2009. 96
This type of PDS contract was introduced with a view to increasing access to dentistry in underserved areas. Its payment mechanism is comprised of three payments in relation to services, access and performance. There are only 36 PDS+ contracts in England. 93
National Health Service dental patient charges
Patients in England pay for NHS dental care unless they are exempt from fees for a specified reason. In 2022, the patient charges are as follows:90
-
Band 1 (examination, radiographs, prevention, treatment plan): £23.80
-
Band 2 (band 1 treatments plus periodontal treatment, direct restorations, endodontics, extractions): £65.20
-
Band 3 (band 1 and/or band 2 treatments plus indirect restorations and dentures): £282.80
-
Urgent (temporary treatment to resolve acute pain or infection): £23.80.
The charge paid by the patient is based on the highest-complexity band that was provided during each particular course of treatment. It also covers any care provided in the less complex bandings. For example, a patient who had an examination, radiographs, two extractions and a partial denture would pay the band 3 charge of £282.80.
Patients who are currently fully exempt from NHS dental payment charges include those aged under 18, those aged under 19 and in qualifying full-time education, those who are pregnant or have given birth within the last 12 months, prisoners and those receiving qualifying low-income-related benefits or holding an income-assessed exemption certificate (HC2). 98 Some patients may be entitled to reduced NHS dental charges if they hold a partial NHS exemption certificate (HC3) through the NHS low-income scheme.
Approvals and registrations
The design of the study was peer-reviewed by the NIHR Public Health Research panel and was prospectively registered with ISRCTN on 13 January 2020 (ISRCTN96479279). The study received ethical approval from North East, Tyne and Wear South Research Ethics Committee on 27 May 2020 (20/NE/0144). The study protocol was published in 2021. 99
To allow NHS BSA to process patient identifiable data without individual patient consent (to select the study population and anonymise the data before transfer to the research team), section 251 support under Regulation 5 of the Health Service (Control of Patient Information) Regulations 2002 was required. This was granted by the Health Research Authority Confidentiality Advisory Group on 7 July 2020 (20/CAG/0072).
Data linkage and validation
Data linkage and validation to create the study population
The following is based on information provided by NHS BSA and describes their standard processes for data linkage and validation, followed by the additional steps that were necessary to provide the study population data set.
National Health Service number (a unique patient identifier) is infrequently populated on FP17 claims forms when they are submitted by dental practices, as it is not a mandatory component. In October 2019, only 6.8% of claims processed by NHS BSA had an NHS number recorded on submission. As a result, NHS BSA undertake a process of identification in order to link dental treatment claims for separate courses of treatment to an individual patient.
Since the creation of a new NHS BSA dental data warehouse in February 2016, the standard process to link FP17 claims to a unique individual patient is for all newly submitted claims to be checked using the following identifiers:
-
Surname
-
Forename
-
Sex
-
Date of birth
-
Last known postcode (patient)
-
Dental contract ID.
If this combination of details has not been recorded previously, the patient’s demographic details are sent to the Demographic Batching Services Spine (DBS) to trace the patient’s NHS number. If the FP17 claim form was submitted with an NHS number and the traced NHS number differs, the traced NHS number is used. Around 70% of the patient records in the new data warehouse have an associated NHS number.
To provide data that spanned 2010–20 for this study, NHS BSA needed to link patient records in the current data warehouse with an older, legacy data warehouse. Demographic tracing of NHS numbers did not take place for records held in the pre-2016 data warehouse; therefore, NHS numbers are not usually available in the older system.
Rather, patients were identified using an Index ID made up of a combination of surname, first initial, date of birth and sex. Index IDs cannot be considered as unique identifiers at the national level. To link the FP17 data across both databases, the Index IDs were matched to the post-2016 patient records. Only patients who had a 1 : 1 match between their NHS number and their Index ID were selected for the study population to ensure that the treatment claims were associated with a unique individual.
FP17 records for each selected patient were then validated. FP17s were removed where there was no information entered, where multiple forms had been submitted for one claim and where the claim was later withdrawn. Courses of treatment that contained only orthodontic treatment were removed.
Data access and storage
NHS BSA data were requested via the NHS BSA’s data for the research application form submitted on 23 July 2019. 100 The NHS BSA and the University of Manchester are joint controllers of the study population data set provided to the University of Manchester as per the terms of the data-sharing agreement. The study population data set was received by the University of Manchester on 16 April 2021. These data are securely held within the University of Manchester’s data safe haven, which is compliant with the NHS Data Security and Protection Toolkit (DSPT, 2020–1).
Bias
This is an observational study using routinely collected data. The primary potential sources of bias are confounding and non-differential errors of misclassification. This section details the approaches we took through the design and analysis of the study to reduce bias.
This study was designed to take a ‘natural experiment’ approach. This is a particular type of observational epidemiological study which utilises unplanned variation in the coverage and implementation of an intervention to strengthen causal inference and reduce bias when it is not possible to allocate the intervention randomly. 101 Guidance on natural experiment studies produced by the MRC provides two essential criteria for a study to be considered a natural experiment. 101
-
The intervention is not undertaken for the purposes of research (met for water fluoridation).
-
The variation in exposure and outcomes is analysed using methods that attempt to make causal inferences (met, as discussed below).
A tighter definition of a natural experiment study states that the intervention assignment should be ‘as-if randomised’. 101,102 In practice, it is very difficult to be sure that intervention allocation has been random without some form of randomisation. However, guidance on natural experiment studies advises that considering how well the ‘as-if randomised’ concept applies to the intervention under study can aid with identifying the potential impact of systematic bias in intervention group allocation and resultant confounding. As water fluoridation is a population-level intervention, it is not possible to randomly allocate individuals to receive it. In contrast, the delivery of optimally fluoridated drinking water is not perfectly correlated with decisions to implement water fluoridation programmes by policy-makers. Unplanned breaks in the supply of optimally fluoridated water can result from diversion of water supplies, equipment failures or suboptimal dosing. 103 This leads to some unplanned (and essentially, random) variation in the receipt of optimally fluoridated water at the level of regions and populations.
Further adding to the unplanned/random variation in intervention allocation, individuals move address, into and out of fluoridated areas. The combination of where an individual has lived between 2010 and 2020 (based on their recorded address in the NHS BSA dental database) and the annual water fluoride concentrations within those neighbourhoods (LSOAs) will affect their personal exposure to this intervention (and group allocation) in a way that is not correlated to the decision to implement it by policy-makers. Additionally, some individuals receive optimally fluoridated water as a result of natural geology rather than policy decisions. In contrast to many non-randomised studies of interventions, exposure to optimal water fluoridation does not rely on individual patients engaging with a particular service or intervention. Therefore, group allocation is less likely to be related to individual lifestyle or behavioural factors that could also be related to individual oral health outcomes (e.g. smoking, diet, oral hygiene behaviours). This reduces the potential for confounding of the effect of optimal water fluoridation due to lifestyle factors and behaviours.
Group assignment at the individual level rather than area level further strengthens the causal inferences that can be made because people living in the same regions or areas but assigned to different fluoridation groups on the basis of dose control or changes of address can be compared to each other, helping to disentangle area-based effects on oral health from the effect of receiving optimal water fluoridation. One example of this was that it is not possible to think of ‘optimally fluoridated’ or ‘sub/non-optimally fluoridated’ dental practices or local authorities in this study because most of the time each of these contained individuals from both groups.
Causal inference was further strengthened by considering which factors might have been important when policy-makers were deciding which areas to fluoridate in the 1960s and 70s and how these past decisions on the location of water fluoridation programmes could be related to the average composition of individuals in the optimally fluoridated and sub/non-optimally fluoridated groups in the study (how could the intervention and comparator groups deviate from being ‘as-if randomised’). 102 This thought process was documented using directed acyclic graphs (DAGs), which are formal diagrams illustrating the causal relationships between the exposures and outcomes of interest in an observational study. They include all common causes of the exposure and outcome of interest, based on expert knowledge and theory. 104,105
The DAGs were then used to inform the creation of optimally fluoridated and sub/non-optimally fluoridated groups for analysis that were equally balanced in terms of potential confounders and predictors of the outcomes through propensity score matching. The aim of matching is to achieve groups that are, on average, similar/equivalent in terms of all measured confounders and important outcome predictors. The latter are included to reduce the variance of the effect estimates, not to reduce bias. 106–109 A more detailed description of the process used to develop the DAG and select the variables for matching is included in (Appendix 2, Tables 33 and 34 and Appendix 2, Figures 16–21). The final DAG as used in the propensity score matching model is available online (https://dagitty.net/dags.html?id=O-tgYq) in interactive format. 110
As with any observational study, the method of accounting for confounding is dependent on the precision and accuracy with which the confounding variables are measured. Poorly measured or misclassified confounding variables will inevitably lead to some residual confounding. 111 Any confounding pathways that we did not consider when creating the DAGs could still lead to unmeasured confounding.
Non-differential misclassification
Another potential source of bias in this study is non-differential misclassification. This type of bias is caused by imprecision and errors in the measurement and classification of exposure and outcome variables. This could occur if a participant in our study was misclassified as being in the optimally fluoridated or sub/non-optimally fluoridated group, or if the dentist did not record the DMFT or numbers of treatments correctly. Non-differential means this bias is expected to affect both the optimally fluoridated and sub/non-optimally fluoridated groups equally. In situations where non-differential errors of misclassification of a binary exposure and outcome are independent (i.e. not connected, obtained from different sources, as in the present case), the effect of this type of bias is to obscure the treatment effect through random noise or ‘bias towards the null’. 111
Variables
The following variables were used during the analysis. A full list of all variables supplied by NHS BSA is available in Appendix 4.
Exposure: patient fluoridation status
Individuals within the NHS BSA data set were assigned a unique water fluoride concentration (mg F/l), based on the number of years they were assumed to have lived within each LSOA and the recorded water fluoride concentration for each LSOA. For further detail on how the 10-year grand mean water fluoride concentration was calculated for each patient, see Estimation of patient drinking water fluoride concentration.
The 10-year grand mean water fluoride concentration for each patient (mg F/l) was recategorised from a continuous into a binary exposure variable:
-
Optimally fluoridated: 10-year individual grand mean water fluoride concentration of ≥ 0.7 mg F/l.
-
Sub/non-optimally fluoridated: 10-year individual grand mean water fluoride concentration of < 0.7 mg F/l.
The threshold of 0.7 mg F/l and above was used to define ‘optimally fluoridated’ as a pragmatic cut-point because previous research has demonstrated that the 1 mg F/l target for water fluoridation programmes in England is not consistently achieved over the long term. 103,112 Furthermore, this is in line with the approach taken in the statutory 2018 health monitoring report for England, which used 0.7 mg F/l as the lower boundary in their binary analyses of caries-preventive effects. 112
Creating a binary exposure variable using continuous data will always present the difficulty that some individuals will be very close to the cut-point. Previous work by PHE found that between 2005 and 2015, 86% of the population of England lived in areas with low water fluoride concentrations (< 0.1 < 0.4 mg F/l), 4% lived in areas with between 0.4 < 0.7 mg F/l fluoride and 10% lived in areas with a water fluoride concentration ≥ 0.7 mg F/l. 112
Primary outcome
Number of invasive dental treatments (e.g. restorations, endodontics, extractions) received by adults attending NHS dental practices over 10 years of observation. The total quantity of such treatments per individual was summed to create a single-count variable. The following dental treatments were defined as invasive dental treatments:
-
permanent fillings and sealant restorations
-
inlays
-
endodontic treatment
-
veneers
-
crowns
-
extractions.
Secondary outcome variables
Oral health
-
Number of missing teeth.
-
Total number of teeth affected by decay (DMFT).
At each course of treatment, the number of teeth that are missing, decayed or filled is collected on the FP17 form. This requirement has been in place since the 2016–7 financial year. Patients whose last course of treatment was prior to the introduction of DMFT being collected were not expected to have this outcome measure (1 April 2016).
This measure is automatically extracted from the dental EPR software based on the dental charting that is completed by the dentist during the NHS examination. The data for these variables were provided by NHS BSA as separate counts of:
-
decayed teeth
-
missing teeth
-
filled teeth.
The number of missing teeth variable was used directly as a count variable taken from the patient’s most recent course of dental treatment (closest to 2020).
The DMFT variable was created by summing the individual D, M and F components as recorded at the patient’s most recent course of treatment (closest to 2020).
Variables used in the matching process
Guidance on propensity score matching states that all confounders and important predictors of the outcome that are unrelated to exposure should be included in the propensity score model. The latter predictors were included to increase the precision of the effect estimates rather than to reduce bias. 110 The following confounding and predictor variables were identified through the creation of a DAG. 113–115
Confounders
The variables below are ‘surrogate’ confounders, as it is not possible to match on the ‘true’ confounders of historic regional prosperity and past population oral health. Surrogate confounders are variables which when conditioned on (through matching, stratification or adjustment) block the confounding pathways caused by the true confounders. 105
-
Patient age
The age of the patient at the start of each course of treatment was added to the study by NHS BSA using the patient’s date of birth (prior to de-identification of the data). For our analysis, we assigned all patients an estimated ‘age in 2011’ as this was the first full year of data collection. This was created by adding or subtracting the number of years before/after 2011 from the age of the patient as recorded at their first course of dental treatment. Age was used as a continuous variable in the analysis.
-
Patient sex
Patient sex, as recorded at their first course of dental treatment, was used for the analysis. This is recorded on the FP17 as a binary male/female variable.
-
Patient ethnicity
The patient ethnicity variable, as supplied by NHS BSA, contained 18 categories at every course of dental treatment. These 18 categories were grouped into 6 categories as per 1–6 below. ‘Patient declined’ or ‘N/A’ were the most common responses at any single course of treatment. In cases where the patient’s ethnicity was recorded as ‘patient declined’ or ‘N/A’, ethnicity information from the next subsequent visit that was not ‘patient declined’ or ‘N/A’ was used to populate this variable.
-
White: White British, White Irish, other white background.
-
Mixed/multiple ethnic group: white and Black Caribbean, white and Black African, white and Asian, other mixed background.
-
Asian/Asian British: Asian or Asian British Indian, Asian or Asian British Pakistani, Asian or Asian British Bangladeshi, other Asian background, Chinese.
-
Black/African/Caribbean/Black British: black or Black British Caribbean, black or Black British African, other black background.
-
Any other ethnic group: any other ethnic group.
-
No information: patient declined or ‘N/A’ at all courses of treatment.
-
Patient deprivation [Index of Multiple Deprivation (IMD) 2015]
Area-level deprivation in England is assessed using a standardised methodology which ranks the 32,844 neighbourhood areas (LSOAs) in terms of relative deprivation, with 1 being the most deprived and 32,944 being the least deprived. Deprivation is assessed across seven domains: income, employment, education, health, crime, housing and living environment. NHS BSA added the IMD 2015 deprivation rank to each course of dental treatment, using the patient’s home postcode. The 2015 IMD data were used rather than the 2010 IMD because the boundaries of LSOAs were reorganised in 2011. The data used to create the IMD 2015 relate to the tax year 2012–3. 116
We transformed the deprivation ranks to deciles, using the rank cut-points provided by the Department for Communities and Local Government, with decile 1 being the most deprived 10% and decile 10 being the least deprived 10% of neighbourhoods (LSOAs) in England. 117 For the analysis, we linked the 2015 deprivation decile to the patient using their LSOA of residence as recorded at their first course of dental treatment.
-
Rural–urban classification (2011)
The Office for National Statistics (ONS) rural–urban classification is an official statistic which classifies LSOAs into eight categories, ranging from the most urban, ‘Major Conurbation’ to the most rural, ‘Village and Dispersed in a Sparse Setting’. 118 The patient’s LSOA of residence at their first course of treatment was used to assign a rural–urban classification to them.
-
Dental practice deprivation (IMD 2015)
The LSOA of the dental practice the patient attended for their first course of dental treatment was used to assign the dental practice deprivation variable. As with patient deprivation, this used the 2015 IMD deciles supplied by the Department for Communities and Local Government.
-
Local NHS dentistry provision (UDAs commissioned per person, by local authority)
The number of UDAs commissioned in the financial year 2015–6 by local authorities was supplied by NHS BSA in response to a request under the Freedom of Information Act (i.e. it was not derived from the main NHS BSA study data set). The year 2015–6 was the earliest year this information could be supplied for. For the analysis, we created the variable ‘UDAs commissioned per person, by local authority’ using the ONS mid-year 2015 population (all ages) as the denominator. The patient’s home LSOA at their first course of treatment was used to link ‘UDAs commissioned per person by local authority’, to individual patients using ONS LSOA-to-local authority look-up files.
Outcome predictors
The addition of the variables below was not deemed essential for the control of confounding. That is, these variables are in addition to the ‘minimally sufficient adjustment set’ and were added as outcome predictors (effect modifiers). 110
-
Type of dental contract
Primary care dental practices in England and Wales are contracted to provide NHS care using three different types of contracts, which are used for slightly different types of primary care dental services and have different blends of monitoring metrics (see Selection of the study population by National Health Service Business Service Authority for further detail). Reflecting these different contract types, this variable had three categories:
-
General dental service
-
Personal dental service
-
Personal dental service plus.
The type of dental contract that the dental practice was working at the patient’s first course of dental treatment was used to assign this variable.
-
Patient NHS Exemption Status
The reason for any NHS dental patient charge exemption at each course of treatment was supplied as a text variable by the NHS BSA. To create the patient’s NHS exemption status variable, the reasons for exemption were grouped into three categories, as listed below, and the patient’s exemption status at their first course of dental treatment was used.
-
Not exempt
-
Income-related exemptions: tax credit, income support, pension credit guarantee credit, NHS Tax credit exemption, Job seekers allowance, universal credit, income-related employment support allowance, full remission, HC2 certificate, partial remission, universal credit, income-based job seekers allowance, partial remission HC3 certificate, disability working allowance
-
Non-income-related exemptions: expectant mother, nursing mother, mother of child born in the last 12 months, 18 + further education, aged 18 in full-time education, patient under 18, prisoner, exam only, person of state retirement age.
Study size
The initial study population size was based on the maximum number of NHS BSA patient records that could be verified as relating to unique individuals. A data set with a large number of patients available is preferable for propensity score matching, as it increases the likelihood that a suitable match from the unexposed group will be found for every person in the exposed group. The matched (analytical) sample size was arrived at based on a wish to increase the generalisability of the study results to the population of regular users of NHS dentistry living in England. Therefore, the aim was to include every exposed patient and the maximum number of comparable unexposed patients in the analysis while still maintaining minimal differences in the characteristics of the optimally fluoridated and sub/non-optimally fluoridated groups.
Data validation and cleaning
Upon receipt of the study population data set from NHS BSA, an initial exploration of the data using graphs, frequency tables and summary statistics was carried out to identify any errors that had occurred during the transfer and to highlight any data entries that seemed implausible.
Based on expert clinical judgement, the following values were considered to be clinically implausible:
-
more than six endodontic treatments, veneers, crowns or inlays provided within a single course of treatment
-
more than 20 permanent fillings or sealant restorations provided within a single course of treatment
-
more than 32 extractions provided within a single course of treatment
-
more than 32 teeth recorded as either filled, missing or decayed, respectively (for the DMFT component).
Consequently, the clinically implausible values were replaced with the maximum clinically plausible values. The number of observations with clinically implausible data affecting the primary outcome was minimal (758 observations out of 6,370,280 in the matched data set) and evenly distributed across the exposed and non-exposed groups.
We had a meeting with NHS BSA to review some of the extreme values we observed for some variables, in particular UDA targets and total contract values. We sent NHS BSA a copy of the tabulated frequency counts, and they reran the same checks on their database. They confirmed that the values we identified were true values and reflected differences in the way in which contracts were commissioned, which related to very high-value contracts that were not commissioned or monitored using UDAs. For example, community dental services contracts providing care under general anaesthesia or contracts that were commissioned to provide orthodontic services.
Estimation of patient drinking water fluoride concentration
Some of the text in this section has been reproduced from Nyakutsikwa et al. 119 This is an Open Access article distributed in accordance with the terms of the CC BY 4.0 licence, which permits others to distribute, remix, adapt and build upon this work, for commercial use, provided the original work is properly cited. Some of the wording has been edited, and some is used verbatim.
Upon receipt of the water fluoride sample data files from each supplier, the data were cleaned so the water fluoride sample data could be matched with the GIS shapefiles. When discrepancies such as differences in WSZ names and/or codes between the files were detected, we made requests to the water supplier seeking clarity and subsequently modified the files to facilitate linkage. Annual descriptive summaries of the fluoride concentration (mg F/l) were then created for each WSZ [mean, median, standard deviation (SD), minimum, maximum, Q1, Q3] in statistical software, ‘R’. 120 In some cases, the water companies supplied the data already summarised as annual means, which were also uploaded into R. The summarised water fluoride concentration data were then merged with the shapefiles using the WSZ codes or names as the joining variable. The geographical projection of these shapefiles was adjusted to the OSGB 1936/British National Grid when necessary. 121,122
WSZ boundaries are not co-terminus with any standard UK geography. To convert the fluoride sample data into a format that could be assigned to individual patients, we mapped WSZs to their corresponding LSOAs. We linked the two geographies using the 2011 ONS LSOA population-weighted-centroids (LSOA-PWC) shapefile. 123 This shapefile contains a single geographic point on the ground where the median distance between all LSOA population members was lowest in the 2011 census (i.e. the weighted centre of where the population resides). 124 We used the spatial intersection method in R125 to combine the LSOA-PWC shapefile with the WSZ shapefiles and then assigned the annual mean water fluoride concentration (mg F/l) to each LSOA.
The individual files for each water company were then combined, resulting in a data set containing annual fluoride concentrations for English LSOAs from 2009 to 2020. The analysis did not discriminate between fluoride in the water as a result of geology (naturally fluoridated) or as a result of a public health programme (artificially fluoridated). Where possible (i.e. when raw sample data had been provided), this included mean, median, SD, minimum, maximum, Q1 and Q3. Maps were then created to allow visualisation of the data using the tmap package in R and the ONS LSOA 2011 and Local Authority District 2020 boundaries shapefiles. 126,127 This resulting data set of annual water fluoride concentration data by LSOA is now publicly available under a Creative Commons license. 128
The data set of annual water fluoride concentration (mg F/l) by LSOA was used to assign individuals in the study population to the optimally fluoridated and sub/non-optimally fluoridated groups of the unmatched study cohort. Using the raw data compiled above, we then carried out imputation for some of the years where there was missing data. Imputation was only carried out for areas where we did have some data for some of the years. In optimally fluoridated areas (based on the data that was present), imputation for the missing years was carried out by using an average of the other years (mean). In areas that were not optimally fluoridated, based on the data that we had, backwards or forwards imputation was used. This is because there has been shown to be greater variation over time in the fluoride concentration of optimally fluoridated water than sub/non-optimally fluoridated water. 103,119
Individual patients were assigned a grand mean water fluoride concentration (mg F/l), based on how many years they were estimated to have lived at each address. The LSOA of the patient’s home address was supplied at every course of treatment. From the start of the study observation period (November 2010) to the date of the patient’s first dental attendance, individuals were assumed to have lived at the same LSOA as was recorded at their first dental attendance (i.e. backwards imputation of LSOA of residence). In the period following each dental attendance, individuals were assumed to have remained living at the last recorded LSOA until a new LSOA was recorded (forwards imputation). Where a patient changed LSOAs within the same year, the annual fluoride concentration of each LSOA was weighted by the number of days the patient was estimated to be living there. The estimation of the number of days was carried out in the same way as described above, using backwards imputation of LSOA for the time before the patient’s first course of treatment and forwards imputation after every new LSOA.
Patients exposed to an individual grand mean water fluoride concentration of 0.7 mg F/l and above were assigned to the ‘optimally fluoridated’ group, and patients with a grand mean of < 0.7 mg F/l were assigned to the ‘sub/non-optimally fluoridated’ group.
Propensity score matching
Overview of propensity score matching
A key consideration in the design of this study was the selection of appropriate individuals residing in areas that were not exposed to water fluoridation to provide a comparator group in order to minimise bias and strengthen causal inference. In earlier observational studies, the selection of non-exposed individuals was sometimes carried out opportunistically, selecting individuals from neighbouring local authority areas or other, broadly comparable areas. We used propensity scores to formally select individuals in the sub/non-optimally fluoridated group based on their similarity to individuals in the optimally fluoridated group using a range of characteristics relating to exposure and outcome. A propensity score is a numerical value from 0 to 1 that reflects the probability of assignment to a particular study group (in this case, receipt or no receipt of optimal water fluoridation) based on their characteristics at the study baseline. 129 The characteristics the estimated propensity score is based on are those chosen by the study team as being important to consider to reduce confounding bias. In assigning each individual a propensity score, the overall ‘balance’ of the distribution of important characteristics in the exposed and non-exposed groups can be assessed using a single numerical value.
The aim is to achieve, as far as possible, an equal balance between the two groups in the pre-specified important characteristics upon which the propensity scores are based. 129 Once the propensity scores have been generated, individuals in the exposed (optimally fluoridated) group can be matched to those in the non-exposed (sub/non-optimally fluoridated) group based on the value of their propensity score. In this study, we used distance matching methods, whereby the distance (difference in propensity score for an individual in the exposed group and an individual in the non-exposed group) is used to form matched clusters of exposed and non-exposed individuals.
Guidance on covariate selection to estimate propensity scores106,129 suggests the best balance (and hence, reduction in confounding) is achieved by including baseline characteristics that, based on expert content knowledge:
-
are related to both the treatment and the outcome (e.g. receipt of water fluoridation and receipt of invasive NHS dental treatments)
or
-
are expected to be related to the outcome (receipt of invasive NHS dental treatments, DMFT and missing teeth)
and
-
are not on the causal pathway between exposure and outcome.
In the case of a very large number of potential variables, it is recommended to include those baseline characteristics related to both exposure and outcome and discard those expected to be only weakly associated with the outcome. 106–109 To ensure potential covariates meet the above criteria, we created DAGs as described in Bias and Appendix 2, Tables 33 and 34 and Appendix 2, Figures 16–21. These DAGs illustrate the hypothesised relationships between all common causes of exposure and outcomes. 110
To inform the selection of the propensity score variables, we had planned to hold a 1-day workshop where key stakeholders would be invited to consider potential confounders that needed to be addressed during the analysis. However, due to COVID restrictions, this was changed to a series of online consultations. See Variables for details of which variables were chosen for matching. Further information on the selection of variables is included in Appendix 2 (Appendix 2, Tables 33 and 34 and Appendix 2, Figures 16–21).
The following sections describe the steps taken to create the propensity scores, match exposed individuals to non-exposed individuals forming a data set for analysis and estimating the effect of exposure on the clinical outcomes.
Data were validated and cleaned prior to any analysis. The extent of missing data for the exposure variable, covariates used to estimate the propensity score and study outcome variables were documented. No imputation was undertaken for missing data. Given the size of the study data set, no meaningful loss of power was anticipated due to missing data. The level of imbalance in the complete data set from the BSA meeting the study inclusion criteria was ascertained through visual inspection and consideration of summary statistics for the variables on which the matching was based. We also reported summary statistics for the outcome variables for the unmatched data set and the matched data set, overall and by exposure status. The software packages R130 and Stata 14131 were used for all statistical analysis.
The R package MatchIt108,132 was used to estimate the propensity scores and undertake the matching of non-exposed individuals to exposed individuals. The propensity scores were estimated using logistic regression, where the response variable was exposure to water fluoridation taking a value of 0 for non-exposed (grand mean fluoride concentration of < 0.7 between 2010 and 2020, and a value of 1 for exposed). The model covariates were those identified by the methods described earlier. We planned to estimate the propensity scores initially in a parsimonious model using main effect terms only and restricting the inclusion of higher order and interaction terms unless adequate balance could not be achieved. This approach was undertaken to minimise the risk of overfitting. 106
Distance matching was used to match exposed and non-exposed individuals. We proposed to employ three methods of distance matching: nearest neighbour, optimal and full matching, with exact matching on the covariates sex and area level deprivation. The use of multiple matching algorithms has been recommended to select the match that has achieved ‘greatest’ covariate balance (where the distribution of the covariates is similar across the exposed and non-exposed groups) while maintaining an adequate sample size through minimisation of discarded observations. 133
Initially we planned to use a nearest neighbour matching algorithm. This is the simplest form of matching and involves finding and matching individuals in un-exposed group who have the closest propensity scores to those in the exposed group. 133 This method has the advantage that it works well for estimating the average treatment effect in the treated (ATT) (i.e. exposed to water fluoridation) when the number of treated cases is substantially smaller than the number of untreated cases available for matching, as is the case for our data set. The ATT in this study is the average effect that would have been expected if everyone in the exposed group received water fluoridation at the 0.7 threshold compared to if no one in this group received the exposure. 106 One limitation to the nearest neighbour matching approach, however, is that the best available match is sought without accounting for the resulting quality of the match of the entire treated sample.
We planned to refine the matching specification by considering the use of a calliper based on the propensity score, and by using a variable ratio matching as well as fixed one to one matching. A calliper is a maximum permissible distance in propensity scores for the match. It can be defined as ‘a fraction of the standard deviation of the logit of the propensity score’. 133 To remove at least 90% of bias, Rosenbaum and Rubin (1985) used a calliper of 0.25 SDs. 134 Any individuals whose propensity scores are not within the specified calliper (e.g. 0.25 SDs) of an individual in the comparison group are not selected for matching. The use of a calliper therefore increases the quality of the matches but risks reducing the matched sample size.
We also planned to undertake optimal pair matching and optimal full matching, which, in contrast to nearest neighbour matching, attempt to optimise the quality of the match for the overall matched data set, but these methods do require substantial processing power for the optimised matching of large data sets. Further description of these matching methods can be found in the publication by Lee and Little (2017). 106
Our proposed matching methods were:
-
Method 0: No matching
-
Method 1: Nearest neighbour, one to one matching
-
Method 2: Nearest neighbour, variable ratio matching (ratio = 3, maximum = 5)
-
Method 3: Nearest neighbour, variable ratio matching (ratio = 3, maximum = 5), with 0.25 calliper
-
Method 4: Optimal matching
-
Method 5: Optimal matching, variable ratio matching (ratio = 3, maximum = 5)
-
Method 6: Full matching
-
Method 7: Full matching with calliper.
Nearest neighbour matching with a fixed one-to-one match is the simplest model and was chosen as our initial approach, given the size of the data set and the number of exposed observations for whom we are seeking a match. An important limitation however is this method results in the smallest matched data set as potentially suitable non-exposed individuals are discarded once one non-exposed individual has been matched. Consequently, the total matched data set can only ever be as large as twice the size of the exposed group (but may be smaller).
This shortcoming was addressed with methods 2 and 3 using variable ratio matching. With variable ratio matching the number of matched non-exposed matches is permitted to vary, thus limiting the number of unsuitable matches compared to a fixed ratio approach. A larger sample size with more similar matches is thereby retained. As stated in Leite,133 research has shown variable ratio matching removes more bias than one-to-one matching135–137 and is particularly useful if the number of untreated (un-exposed) group cases is much larger than the number of treatment cases138 as is the case with the exposed and non-exposed groups in the study.
Method 2 was further refined in method 3, where a 0.25-unit calliper (a maximum permissible distance in propensity scores for the match) was added to the variable matching. In fixed and variable matching, the chance of unbalanced covariates may remain when the additional matches are not always as close as the first match. 108,132
In order to determine the ‘success’ of the different matching algorithms and to select the matched data set for analysis we considered the number of matched participants, including the number and impact of discarded observations from the exposed group, the overall sample size and the distribution of the covariates across the exposed and non-exposed groups. Evaluation of covariate balance was undertaken through visual inspection of their distributions in the unexposed and exposed groups, and examination of descriptive statistics. Visual inspection of covariate balance was undertaken through Love plots, empirical quantile–quantile (eQQ) plots for continuous covariates, bar charts for categorical covariates and kernel density plots for propensity score distribution and continuous variables. Balance was deemed to have been achieved on the eQQ plots where values fall on the 45o line, and for the empirical cumulative distribution function (eCDF) and kernel density plots where the lines of the exposed and non-exposed groups overlap.
Given the large sample size of the study data set, standardised mean differences (SMDs) were used in preference to hypothesis tests to determine covariate balance. 106 The SMD for a given covariate compares the difference in the means of the covariate (between the exposed and unexposed groups), divided by the pooled SD of the covariate. 139 SMD is also referred to as Cohen’s d,140 often used to frame effect sizes as small, medium or large in the psychological literature. 141 The large sample size meant it was conceivable that statistically significant differences in SMDs obtained through hypothesis testing for the covariates would be indicative of unimportant mean differences. In preference, absolute SMDs below a threshold of 0.1 SDs were considered to constitute adequate balance. 142
Lastly, we compared the distributions of the propensity scores for the exposed and non-exposed groups to ascertain the existence of common support for the treated observations through visual inspection of the linear propensity scores for the treated and untreated (jitter plots and histograms). If a substantial overlap is observed over the range of the propensity scores between the treatment and comparison groups, then this suggests a large area of common support over the participants. 106
Analysis of health outcomes
Once satisfactory balance on the covariates was achieved through propensity score estimation and matching and the preferred analytical data set selected, we estimated the effect of exposure on the primary and secondary clinical outcomes. As the primary and secondary outcomes were count responses, we used regression models depending on the distribution of the outcome under evaluation (Poisson or negative binomial models as appropriate), including matching weights and taking into account the dependency of the data using cluster robust standard errors (SEs). We planned to include the covariates used for the propensity score matching in the regression models of the clinical outcomes only if slight imbalances remained on the covariates (as indicated by a SMD between 0.1 and 0.25 units).
For the analysis of the primary outcome, we modelled the number of invasive dental treatments received per person as a count outcome. Results are reported as an IRR with 95% CI. The IRR is the ratio of the rate (count) of invasive dental treatments received over 10 years of observation in the optimally fluoridated group compared with the sub/non-optimally fluoridated group.
For the analysis of the secondary outcomes, we modelled the number of DMFT and missing teeth as count outcomes and reported the results as IRRs with 95% CIs.
To determine whether there was a differential effect of water fluoridation according to deprivation on the primary outcome, we undertook a regression analysis that included the main effects of water fluoridation and deprivation decile with an interaction term. Results are reported as IRR per course of treatment with 95% CIs. The marginal predicted number of invasive treatments according to combination of deprivation decile and fluoridation status was calculated and plotted using the margins command in Stata. 143
To determine whether there was a differential effect of water fluoridation according to deprivation on the secondary outcomes DMFT and missing teeth, we undertook regression analyses that included the main effects of water fluoridation and deprivation decile with an interaction term. Results are reported as IRR with 95% CIs. The marginal predicted numbers of DMFT and missing teeth according to combination of deprivation decile and fluoridation status were calculated and plotted using the margins command in Stata. 143
Ancillary analyses
-
An additional analysis was proposed by the Study Oversight Committee. We modelled the probability of receiving an invasive dental treatment according to exposure using logistic regression.
-
An offset term using the total number of courses of treatment was added to the regression model for the primary outcome of number of invasive dental treatments, so the exposure of the optimally fluoridated and sub/non-optimally fluoridated groups is proportional to the number of courses of treatment. Including an offset term in the model adjusts for the opportunity that an event has to occur. To determine whether there was a differential effect of water fluoridation according to deprivation on the primary outcome, when taking account of the number of courses of treatment received, we undertook an additional regression analysis that included the main effects of water fluoridation and deprivation decile with an interaction term and included an offset term for total number of courses of treatment.
Stakeholder views on a minimum ‘clinically’ relevant effect size
Given the large number of observations, from the outset we prioritised the magnitude of the treatment effect rather than statistical significance when interpreting the results of this study. In most research studies, the research team decides what effect size would be considered to be ‘clinically relevant’ when calculating the sample size. This decision is often pragmatic, balancing clinical experience, the resources available for recruitment and study duration, what is a realistic effect size considering previously published research. 144,145 Previous work has identified that different participant groups, for example, patients and medical professionals, may have differing views on what effect sizes are considered to be important. 146 Despite this, only a minority of studies in a recent systematic review of randomised controlled trials had sought opinions from patients and the public when specifying the target difference for the trial. 144 As water fluoridation is a universal population-level intervention, we felt it was important to gather views and opinions from a range of stakeholders, rather than make a decision solely within the research team. The intention through this involvement work was to explore the range of views and provide context for readers to help them to determine what they consider to be meaningful, rather than to provide a ‘hard cut-off’ for success.
We had originally planned to hold a face-to-face workshop with key stakeholders. Due to the impact of COVID-19, we instead held three online workshops of an hour each. Views from the following key stakeholder groups were sought using a range of invitation methods which included local and national routes:
-
patients and the public
-
dentists
-
dental therapists, hygienists and dental nurses
-
dental public health specialists and consultants
-
commissioners of NHS dental services
-
oral health promotion practitioners.
Patients and the public were invited to take part through two routes. The first was through a written request e-mailed to the University of Manchester’s PRIMER engagement group (Primary Care Research in Manchester Engagement Resource). The second was through an advertisement placed on the UK-wide NIHR People in Research website. All patient and public contributors were reimbursed for their time and use of home equipment either through expenses claim (PRIMER) or through a £30 shopping voucher (People in Research).
Dental care professionals were invited by e-mail invitation distributed by the Greater Manchester Local Dental Network and Local Dental Committees. Dental public health consultants and specialists were invited through the UK-wide British Association for the Study of Community Dentistry. Commissioners of NHS dental services were invited via e-mail through existing relationships and oral health promotion officers were invited through the North West Oral Health Promotion Network. Professional contributors were not financially reimbursed for their time, but if applicable, they were provided with a General Dental Council compliant certificate for 1 hour of continued professional development.
The workshops included an introductory talk on the background to the study and the concept of a ‘meaningful difference’ in our primary outcome (number of invasive dental treatments received over 10 years). The presentation was reviewed by the patient and public involvement (PPI) member of our Operational Management Group (OMG) and some changes were made to ensure the task was understandable and clearly explained. An example slide from the workshop is presented in Figure 3.
FIGURE 3.
Example slide from the meaningful difference workshops. ‘Tooth Filling’ icon created by Peter van Driel from thenounproject.com used under CC BY 4.0 licence.
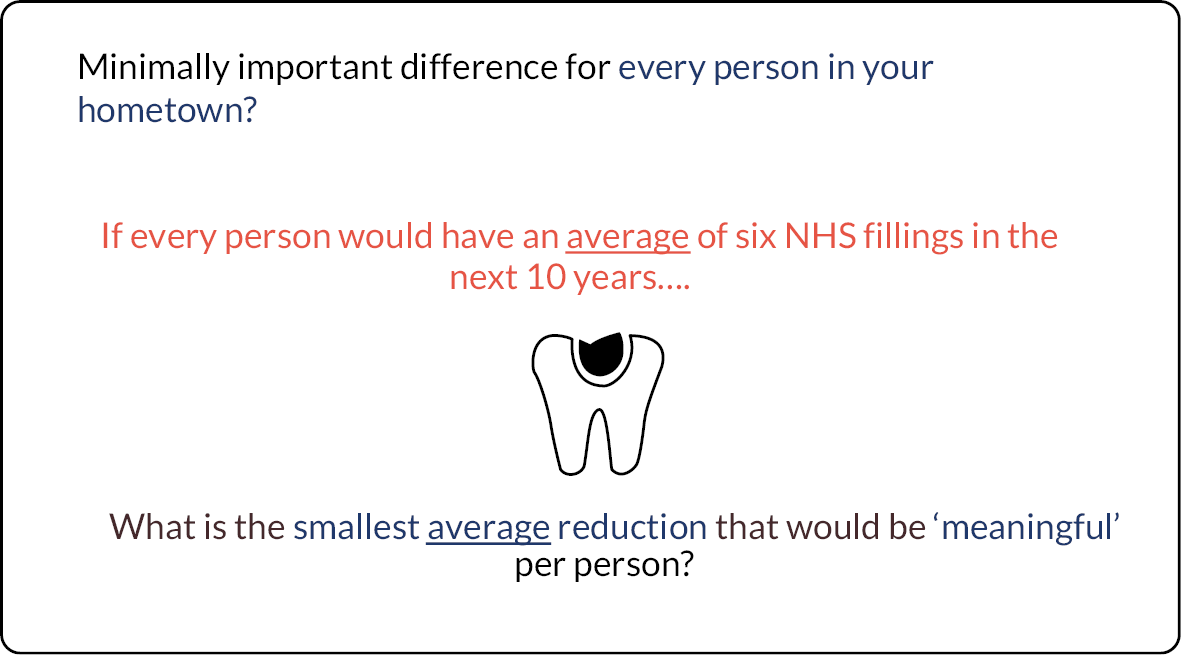
The concept of a meaningful difference was explained in terms of ‘what is the smallest reduction you would care about/think was worth having?’ and then attendees were presented with four scenarios which were designed to explore any differences in the threshold for fillings versus extractions, and differences when considered from the perspective of individuals versus populations:
-
If you would normally have six NHS fillings in the next 10 years …. What is the smallest reduction that would be ‘meaningful’ for you?
-
If you would normally have six NHS extractions in the next 10 years …. What is the smallest reduction that would be ‘meaningful’ for you?
-
If every person in your hometown would have an average of six NHS fillings in the next 10 years …. What is the smallest average reduction that would be ‘meaningful’ per person?
-
If every person in your hometown would have an average of six NHS extractions in the next 10 years …. What is the smallest average reduction that would be ‘meaningful’ per person?
For each scenario, contributors were requested to vote from a multiple-choice list (either via Zoom poll or Google forms, depending on which workshop). The options were presented in both absolute terms and relative terms (percentage reduction). The choice of six fillings and extractions for the ‘expected’ number of interventions without fluoridation was informed by longitudinal data suggesting that caries progresses at a rate of around 0.8–1.2 new surfaces per year in adults. 147,148 We planned in advance to prioritise the results as considered from the population perspective (home town) because water fluoridation is a population-level intervention.
The multiple-choice options for the minimum meaningful difference were:
-
three per cent reduction (0.18 of a filling/extraction fewer in 10 years)
-
five per cent reduction (0.3 of a filling/extraction fewer in 10 years)
-
eight per cent reduction (0.48 of a filling/extraction fewer in 10 years)
-
ten per cent reduction (0.6 of a filling/extraction fewer in 10 years)
-
sixteen per cent reduction (1 filling/extraction fewer in 10 years)
-
twenty-five per cent reduction (1.5 fillings/extractions fewer in 10 years)
-
thirty-five per cent reduction (2 fillings/extractions fewer in 10 years)
-
fifty per cent reduction (3 fillings/extractions fewer in 10 years).
Where the absolute number of fillings and extractions was < 1, contributors were asked to consider that for an individual, it would take a longer time to prevent one whole treatment.
Over the three workshops, held in April, May and August 2021, a total of 54 contributors took part. A summary of contributor numbers and recruitment route is presented in Table 1.
Contributors | Subgroup | Number |
---|---|---|
Dentists (16) | Dental public health specialists and consultants | 8 |
Clinical dental practitioners (GDS/CDS) | 8 | |
Oral health professionals (20) | Oral health promotion practitioner | 11 |
Dental care professional (dental nurse, therapist, hygienist) | 7 | |
Commissioner of NHS dental services | 2 | |
Public contributors (18) | NIHR People in Research | 10 |
PRIMER group | 8 | |
Total | 54 |
Simple frequency charts of the votes of all contributors for each of the multiple-choice options are presented in Figure 4 (for the results when considered from an individual perspective) and Figure 5 (for the results when considered from a population perspective). The frequency charts show the reductions considered meaningful were similar for fillings and extractions, and contributors were slightly more likely to consider smaller reductions to be meaningful when considering the effect size as an average applied to a whole population, as opposed to them as an individual.
FIGURE 4.
Votes of all contributors for the minimum reduction in the number of fillings or extractions that would be considered meaningful from an individual perspective.
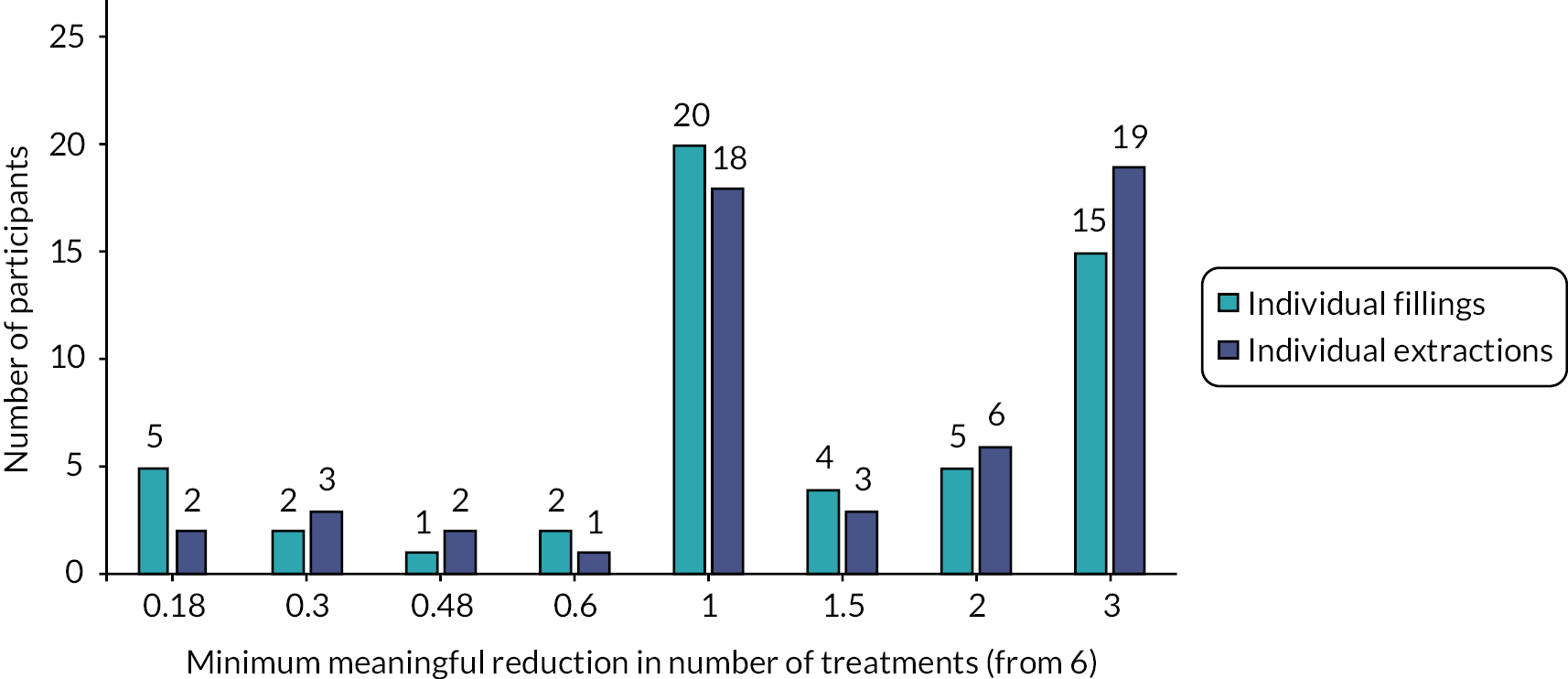
FIGURE 5.
Votes of all contributors for the minimum reduction in the number of fillings or extractions that would be considered meaningful from a population perspective.
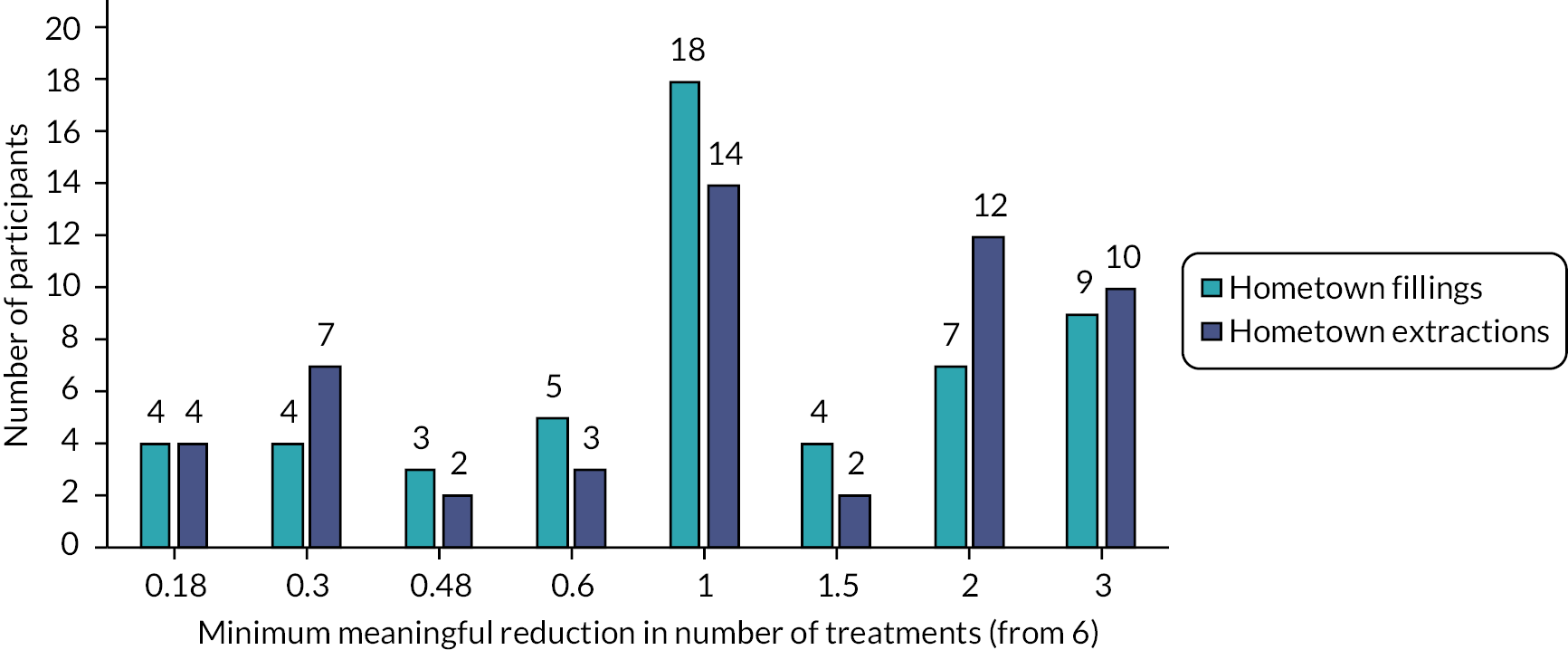
The most popular vote for what was meaningful in absolute terms was a reduction of one invasive dental treatment over 10 years; this was the same when participants were thinking about this for themselves as individuals and when thinking about everyone in their hometown (a population). In the example the participants were given, an absolute reduction of one filling or extraction (from an expected number of 6 over 10 years) equates to a 16% relative reduction.
Interestingly, the responses of contributors appeared to differ slightly by participant group. This is explored further in Figure 6, which presents the median of the votes, by participant group. Median is used as the measure of central tendency rather than the mean because the intention is to present the reduction that would satisfy most participants, and overall, the median responses for each scenario were closer to the modes (most popular) than the means. Dentists appeared to be more likely to consider smaller reductions in the numbers of fillings and extractions to be meaningful when considered from the population perspective, compared to other groups. Non-dentist oral health professionals were expecting a larger reduction in treatments for it to be considered meaningful when compared to dentists and patient and public contributors.
FIGURE 6.
Median of meaningful difference responses by contributor type. OHP, oral health professional.
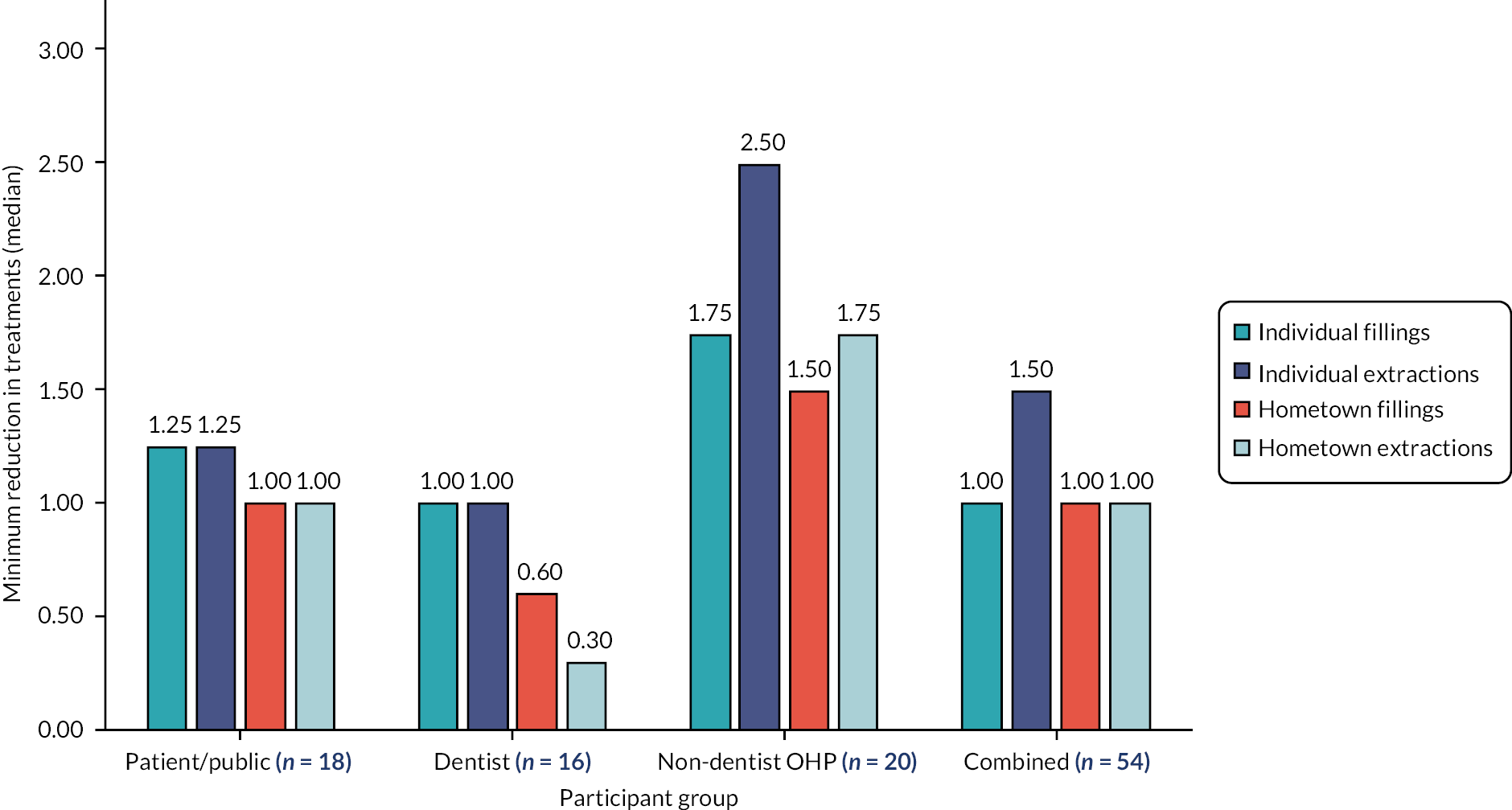
This involvement and engagement work illustrated there is a wide range of views regarding what would be a meaningful reduction in the number of invasive dental treatments received over 10 years as a result of water fluoridation. This appears to be a very subjective judgement, and this was the case even within the research team. However, as an overall summary, most non-dentist participants across the three engagement workshops felt an average reduction of at least one invasive NHS dental treatment per person over 10 years would be needed to be considered as meaningful to them (a 16% relative reduction in this scenario). On the other hand, the dentists present in the workshops voted for reductions as small as a third of a treatment per person (−0.30) to be meaningful (a 5% relative reduction in this scenario) when applied to a population. We refer back to this feedback from stakeholders when presenting the results of the analysis of health outcomes. Readers and policy-makers are encouraged to consider what they would view as a clinically or practically meaningful effect size.
Health economic evaluation methods
Approach
The health economics analysis consisted of calculating the ROI and a cost-effectiveness analysis. For these analyses, a public sector perspective was taken, which accounted for the direct costs and savings accrued to the relevant public sector bodies. The costs of water fluoridation are borne by PHE (for capital infrastructure costs), or local authorities (for operating costs), with any savings in treatment costs accrued by the NHS. Indirect public sector and/or wider societal costs were not included due to the pragmatic nature of the study and the limitations in the available data.
The ROI analysis allows for the financial costs for water fluoridation to be compared against the financial returns resulting from the NHS paying for fewer primary care dental treatments (all types of treatment). The cost-effectiveness analysis compares these same costs against avoiding invasive dental treatments; these being irreversible surgical procedures that often provoke anxiety and result in the loss of healthy tooth tissue.
Data collection
Costs of water fluoridation
A request for information on the annual costs of water fluoridation between 2009 and 2020 was made to the five water companies that have arrangements with the Secretary of State for Health and Social Care to provide fluoridated water. These are: Anglian Water, Northumbria Water, Severn Trent Water, South Staffordshire Water and United Utilities. Costs were requested in May 2021 using a questionnaire that requested information on the estimated population served, estimated total mega-litres of fluoridated water produced per year, capital and operating costs for each year from 2009 to 2020 and estimated lifespan of the water fluoridation dosing equipment. We requested the questionnaire be completed separately for each fluoride dosing installation. The information that was returned was not complete enough to inform the health economic analysis and one company did not return any information.
A further request for information on the costs of water fluoridation was submitted to PHE in July 2021. From April 2013 to October 2021, PHE was the public body responsible for delivering the functions of the Secretary of State for Health in relation to water fluoridation. This included monitoring and paying water company bills related to fluoridation. The operating costs were recovered from the local authorities whose populations are served by the fluoridation programmes. The costs of any capital expenditure were the responsibility of the Secretary of State for Health (in effect, PHE). From October 2021 these functions were transferred to the Office for Health Improvement and Disparities within the Department for Health and Social Care.
Operating and capital costs provided by PHE and relating to water fluoridation were combined to form total fluoridation costs. Annual costs cover financial years running from April to March. Operating costs included running costs such as workforce, transport and chemicals. Capital costs include replacement plants or repairs and upgrades to fluoride dosing equipment and facilities and are subject to annual fluctuations based on the required work. It is not possible to account for the depreciation in capital costs and PHE did not provide this. Furthermore, no data were available on the initial set-up costs of the current, or any future, water fluoridation schemes. Set-up costs vary widely between settings because of the different configurations and required capital investment. For example, water flows, road infrastructure and the arrangement of water treatment facilities are all location specific and need to be considered. 149
Fluoridation cost data from PHE for the 3 years 2010 to 2012 were not available, as this period pre-dates the existence of PHE and prior data could not be provided. Costs for these 3 years were imputed using the mean cost from 2013 to 2019. All fluoridation costs were inflated using the general dental practitioner (GDP) deflator and presented in 2020 prices. 150
Total fluoridation costs provided from PHE were used to calculate per-person water fluoridation costs; this first required an estimate of the population size receiving fluoridated water. It was not possible to source a formal indicator of which LSOAs within England are intended to receive water fluoridation and which receive it naturally, through geological sources. Because of this, we stated in our protocol we would not differentiate between natural and artificial water fluoridation when assigning individual patients to exposure groups and when evaluating health outcomes. Rather, assignment to the ‘exposed’ group was based on an individual grand mean water fluoride concentration of 0.7 mg F/l between 2010 and 2020. The ‘optimally fluoridated’ group therefore contains some individuals who receive water fluoridation without any cost to the public sector. The effect of this is to bias any estimates of cost effectiveness in favour of water fluoridation by including benefits that have not accrued any costs.
To avoid increasing this bias, when calculating the cost effectiveness and ROI for water fluoridation, we estimated the population receiving artificially fluoridated water based on published sources such as maps and descriptions of water fluoridation coverage in England. 21,151 Combining these published data with our own water fluoridation mapping work, we estimate that approximately 5% of the optimally fluoridated group in this study receive water fluoridation from natural sources. The estimated population receiving artificial water fluoridation was used to calculate water fluoridation cost per person, and to inform the scenarios for ROI and cost effectiveness.
Costs of National Health Service dental treatment
Information on the costs of NHS dental care was contained within the study population data set provided by NHS BSA. These data were submitted by dental practices in order to claim activity against their NHS dental contracts and to process patient charges.
The total financial value of each dental contract was provided, as well as the UDA target for the contract. NHS BSA were only able to provide the total contract value and the UDA target from 1 April 2014 because prior to this time the data were recorded in a different format and were not comparable. The average price per UDA in England was obtained from the NHS BSA under the Freedom of information Act (2000). 152 NHS BSA provided prices for financial years 2015–6 to 2019–20.
In addition, for every course of treatment, the ‘band’ of the treatment and the patient charge amount was provided. A band 1 course of NHS dental treatment (examination, prevention, radiographs) attracts 1 UDA. A band 2 course of treatment (restoration, endodontics, extractions) attracts 3 UDAs. A band 3 course of treatment (crowns, dentures) attracts 12 UDAs.
The cost of dental treatment was calculated using three approaches:
-
Patient charge: this amount was provided directly within the NHS BSA data and represents the charge to the patient for a specific course of treatment. Charges varied by treatment band, by year of treatment and by patient charge exemption status.
-
UDA treatment cost: the amount paid to the dental practice for the course of treatment. Treatment bands were first allocated the appropriate number of UDAs, and then multiplied by the average price per UDA sourced from the NHS BSA under the Freedom of information Act. NHS BSA could not provide average UDA prices for all years and therefore courses of treatment prior to April 2015 were allocated the 2015–6 amount while courses of treatment after March 2020 were allocated the 2019–20 amount. The average UDA value for England was used to cost the treatment (in preference to the contract-level UDA values contained within the study population data set) to increase the generalisability of the findings and to remove the effect of the very high-value contracts that are not commissioned and monitored solely using UDAs being included in the study data set (e.g. contracts where a proportion of the total financial contract value is monitored by specific KPIs rather than UDAs).
-
National Health Service treatment cost: the cost to the NHS for each course of treatment. Calculated as the UDA treatment cost minus the patient charge.
All dental treatment costs were inflated using the GDP deflator and presented in 2020 prices. 150
Data validation and cleaning
For 436,886 courses of treatment (approximately 0.67% of the total), the patient charge and the treatment band were not aligned, for example, a course of treatment allocated to band 1 but incurring a patient change associated with band 2. In these cases, both the treatment band and the patient charge were recoded to missing.
Return on investment
We calculated the public sector financial ROI for water fluoridation. The investments were captured by the costs of providing water fluoridation, while the returns were captured by differences in NHS treatment costs relating to reductions in dental service utilisation. For each patient in our matched cohort, the total NHS treatment cost over the study period was calculated by summing the NHS treatment cost across all courses of treatment. Differences in cost between patients from optimally fluoridated and sub/non-optimally fluoridated groups were estimated using a generalised linear model (GLM) with a log-link and a gamma distribution to account for right-skewed cost data. This choice of model specification was informed by a histogram showing the distribution of costs to have a long right-hand tail and by the low value of the Akaike information criterion compared to other possible specifications.
Per-patient differences in costs were extrapolated to the relevant population of regular users of NHS dental services living in optimally fluoridated areas using the following scenarios:
-
Scenario 1: estimated cost savings are attributed to the average optimally fluoridated population size 2010–20. This scenario will overestimate the ROI as it assumes our sample and the optimally fluoridated population have the same characteristics which we know is not the case. For example, our sample is constrained to those individuals aged 12 + who have attended an NHS dental practice at least twice during the study period.
-
Scenario 2: estimated cost savings are attributed to an estimate of the proportion of patients who have seen an NHS dentist during the study period. The proportion is estimated from responses to the GP Patient Survey 2019. Respondents were asked for reasons for not having an appointment in the last 2 years and could answer: prefer private; stayed with dentist when changed from private; tried but not successful; cannot remember if successful or not; and didn’t think they could get one. The proportion giving these reasons for not having an appointment summed to 24.40%; hence, we estimate the proportion having an appointment in this scenario to be 75.60%.
-
Scenario 3: estimated cost savings are attributed to the proportion of patients who have seen an NHS dentist in the National Institute for Health and Care Excellence (NICE) recommended recall period as reported by NHS BSA. Recall period is within 12 months for aged 12–18 and within 24 months for 18 +. The proportion is 50.30%.
-
Scenario 4: estimated cost savings are attributed to a proportion of patients lying equally between scenario 2 and 3. This scenario was suggested by PHE members on this study steering group and represents what they believed to be closest to the actual figure of individuals over the age of 12 who would be likely to attend an NHS dentist at least twice within 10 years. The proportion is 62.95%.
These different scenarios allow for us to explore the impact of the uncertainly that exists regarding the appropriate population our estimated effects should be applied.
Cost effectiveness
Cost effectiveness was based on the primary study outcome, assessed as the additional cost per avoided episode of invasive dental treatment. A public sector perspective was taken, as the costs were paid by PHE and the effectiveness is measured at NHS dental practices, and we estimated the incremental cost-effectiveness ratio (ICER). The ICER was measured by the difference in water fluoridation costs between optimally fluoridated and sub/non-optimally fluoridated groups (incremental costs), divided by the difference in the number of invasive dental treatments between optimally fluoridated or sub/non-optimally fluoridated groups (incremental effects).
The per-patient difference in the number of invasive dental treatments was provided by the statistical analysis detailed in Data validation and cleaning. Per-person differences estimated from our sample were extrapolated to the relevant population of regular users of NHS dental services living in optimally fluoridated areas using the same scenarios detailed above.
Reductions in dental service costs were not factored into the incremental costs of fluoridation (numerator in the ICER) because the reduction in invasive dental treatments is used as the measure of effects (denominator in the ICER). Deducting the costs of reduced dental treatments from the costs of water fluoridation would involve ‘double counting’ this change in treatment episodes.
The time horizon was the study duration. Costs and outcomes were not discounted as the difference in outcomes is observed once, at the end of study follow-up period and therefore the year in which differences occurred was not known.
Sensitivity analysis using fee-for-item costs
To afford greater sensitivity to the examination of relative differences in the use of NHS resources by patients in the optimally fluoridated and sub/non-optimally fluoridated groups, an alternative costing approach informed by the Scottish NHS fee-per-item system was also utilised as a sensitivity analysis. This is because in the English NHS dental contract, a course of treatment containing multiple invasive dental treatments attracts the same NHS payment (UDA allocation) as a course of treatment containing just one invasive dental treatment. It could be argued that the UDA system (with just three ‘bands’ of costs) could obscure cost differences as a result of lower treatment needs. Investigating the effect of using the Scottish fee-for-item costs was hypothesised to provide a more discriminatory approach.
It is important to note the intention here is not to estimate ‘equivalent’ Scottish NHS treatment costs, and the values attached to each treatment item are not intended to accurately quantify resource use in absolute terms. It is included for comparison purposes of relative differences only. This approach to costing dental care may also be more understandable to readers who are not familiar with the English system of remuneration for NHS dentistry.
For each course of treatment, the NHS BSA clinical data set contained the total number of treatments provided within each of the following treatment categories: examinations; permanent fillings and sealants; extractions; inlays; crowns; veneers; endodontic treatment and fluoride varnish.
The fee-for-item costs for each type of treatment in the Scottish Dental Remuneration schedule 142 November 2019153 were reviewed by two dentists who have practised in the NHS system in England and one dentist who has practised in the NHS system in Scotland. The Scottish treatment item that would best reflect the ‘middle’ level of complexity for each type of treatment recorded in the English FP17 clinical data set was chosen as the reference cost. The total number of treatments in each category for each patient was then multiplied by the chosen reference cost.
Changes from protocol
Substantial amendment 1 (NHS REC approved 17th of September 2020)
In the original proposal, the ‘fluoridated’ group were to be defined as individuals living in an area with an artificial water fluoridation programme (regardless of achieved water fluoride concentrations). The effect of achieved water fluoride concentrations were to be considered in a subgroup analysis investigating the differential effects of receiving optimally (≥ 0.7 mg F/l), or suboptimally fluoridated water (< 0.7 mg F/l). However, it was not possible to source an indicator of which areas in England were part of a fluoridation programme; therefore, we were unable to carry out the analysis as planned. The method for group assignment was changed to be based solely on measured water fluoride concentrations rather than programme coverage.
Other changes
In the protocol, the wording of the age range of the participants is stated as ‘adolescents and adults aged over 12 years’. We wish to clarify that the intended meaning should read as ‘adolescents and adults aged 12 years and over’ (i.e. beyond their 12th birthday).
For the secondary objective related to oral health, we had originally planned to use as ‘number of remaining teeth’ as an outcome, in addition to DMFT. The data are provided by NHS BSA as individual D, M and F components. During the analysis, we decided to avoid the planned transformation of 32-missing and to use the data as supplied by NHS BSA number of missing teeth. This was because the raw data had a better fitting distribution for the statistical models, and we could not be sure of the original number of teeth in the subtraction (32 vs. 28), as many people have congenitally missing third molars (wisdom teeth).
On 28 January 2022, the study end date changed to reflect a 6-month extension as a result of COVID-19 and the additional work involved in sourcing and compiling water fluoride concentration data.
Chapter 4 Results
Water fluoridation
Public Health England and the DWI were not able to provide information on which areas within England receive fluoridated water, either as part of a fluoridation programme or as a result of natural fluoridation. Of the 24 water suppliers listed for England and Wales on the Ofwat website, 17 companies were able to provide the requested fluoride concentration sample data and accompanying WSZ shapefiles. The data provided by the water companies comprised 164,111 individual fluoride concentration samples and 3027 annual mean records (mg F/l). Where raw sample data were provided, the mean number of samples provided for each WSZ, per year, was 11.07, but this ranged from 1 to 184.
There are 32,844 LSOAs in England. Table 2 illustrates the number and proportion of LSOAs which could be assigned an annual mean water fluoride concentration using the data supplied by the water companies, and those where imputation was undertaken for some of the years. Imputation was only carried out for areas where we had some data for some of the years. In areas that were optimally fluoridated based on the data present, imputation for the missing years was carried out by using an average of the other years (mean). In areas that were not optimally fluoridated based on the data present, backwards or forwards imputation was used. LSOAs where no corresponding WSZ shapefiles and fluoride sample data were obtained for any of the years were classified as missing. Areas that were not covered by the data we obtained included the Isles of Scilly, national parks, mountainous areas and private water supplies. These areas are shown as white on the maps (Figures 7 and 8).
Year | LSOAs with fluoride samples and WSZ map supplied (%) | LSOAs with simple imputation of annual fluoride concentration (%) | LSOAs assigned a fluoride status after simple imputation (%) |
---|---|---|---|
2010 | 32,019 (97.5) | 774 (2.4) | 32,793 (99.8) |
2011 | 32,553 (99.1) | 240 (0.7) | 32,793 (99.8) |
2012 | 32,565 (99.2) | 228 (0.7) | 32,793 (99.8) |
2013 | 32,566 (99.2) | 227 (0.7) | 32,793 (99.8) |
2014 | 32,720 (99.6) | 73 (0.2) | 32,793 (99.8) |
2015 | 32,709 (99.6) | 84 (0.3) | 32,793 (99.8) |
2016 | 32,667 (99.5) | 126 (0.4) | 32,793 (99.8) |
2017 | 32,668 (99.5) | 125 (0.4) | 32,793 (99.8) |
2018 | 32,722 (99.6) | 71 (0.2) | 32,793 (99.8) |
2019 | 32,789 (99.8) | 4 (0.0) | 32,793 (99.8) |
2020 | 26,384 (80.3) | 6409 (19.5) | 32,793 (99.8) |
FIGURE 7.
Map illustrating grand mean water fluoride concentration (mg F/l) in England between 2010 and 2020, with local authority boundaries overlaid.
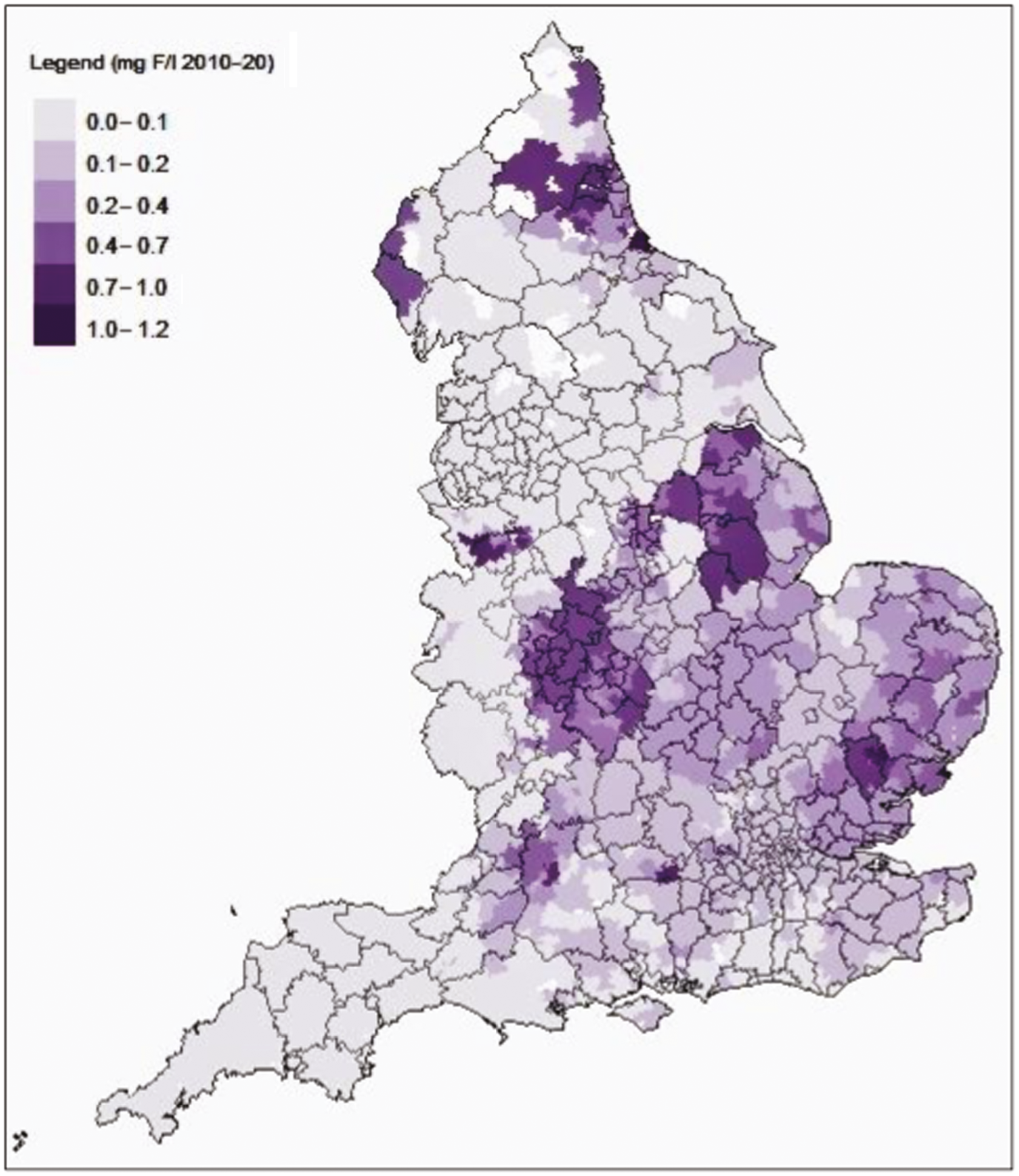
FIGURE 8.
Map illustrating which areas received optimally fluoridated (≥ 0.7 mg F/l) and sub/non-optimally fluoridated water (< 0.7 mg F/l) in England between 2010 and 2020, with local authority boundaries overlaid. Areas shown as white are those where we did not receive WSZ maps or samples.
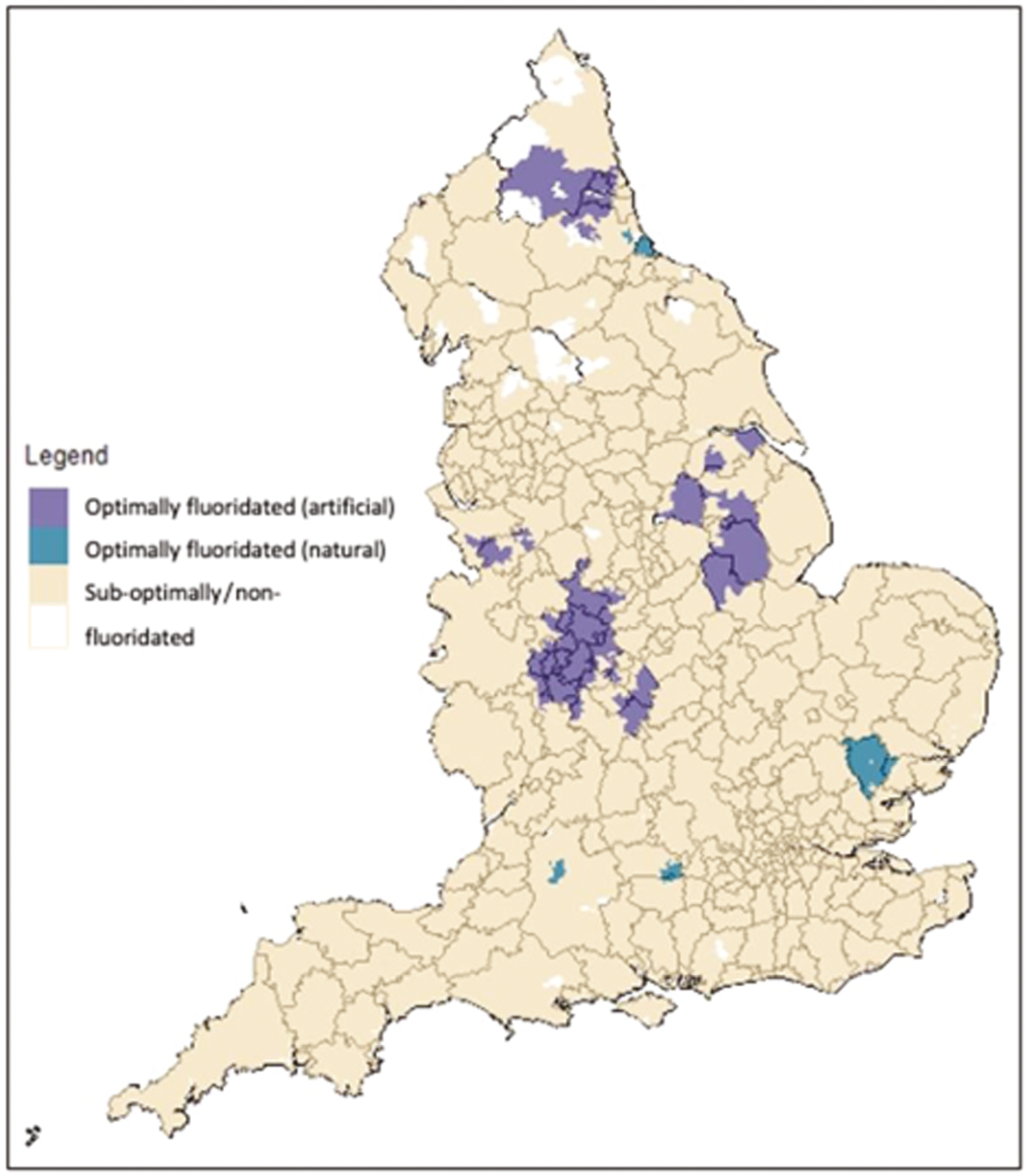
We were not able to source any formal indicator of which WSZs are intended to receive fluoridated water as a part of a public health programme (as opposed to receiving it naturally). The ‘naturally fluoridated’ indicator on the map in Figure 8 was created by visually comparing our map to published sources. 21,151
For the 32,793 LSOAs in England (99.8%), we were able to assign a water fluoridation status, the number and proportion that received water with an annual mean water fluoride concentration ≥ 0.7 mg F/l is presented in Table 3.
Year | Number of LSOAs receiving optimally fluoridated water (≥ 0.7 mg F/l) (%) | Population estimates for LSOAs receiving sub/non-optimally fluoridated water (≥ 0.7 mg F/l) |
---|---|---|
2010 | 2754 (8.4) | 4,427,925 |
2011 | 2730 (8.3) | 4,433,482 |
2012 | 3179 (9.7) | 5,185,052 |
2013 | 3408 (10.4) | 5,595,565 |
2014 | 3569 (10.9) | 5,880,623 |
2015 | 3371 (10.3) | 5,567,389 |
2016 | 2071 (6.3) | 3,414,311 |
2017 | 2696 (8.2) | 4,571,955 |
2018 | 3071 (9.4) | 5,192,260 |
2019 | 2402 (7.3) | 4,054,555 |
2020 | 2438 (7.4) | 4,142,775 |
The number and proportion of LSOAs that were optimally fluoridated in each year varied considerably over time. The difficulties of maintaining a consistent level of fluoridation have been previously documented. 103,119,154–157 A recent study of long-term dose monitoring records from a sample of eight water treatment works in England, spanning 18–35 years, found the period mean water fluoride concentration from the different treatment plants ranged from 0.53 (SD 0.47) to 0.93 (SD 0.22) mg F/l, and was within the optimal range (0.7–1.0 mg F/l) in 27.7% to 77.8% of samples, depending on site. Reasons proposed for suboptimal performance of water fluoride dosing are flooding, failures in equipment, fires and shortages in the supply of fluoridation chemicals. 156,158,159
It is because of this variability in the coverage of optimal water fluoridation over time that we created an individual-level measure of exposure for each patient, rather than assuming the area they lived in was ‘fluoridated’.
The annual mean water fluoride concentration (mg F/l) for the LSOAs which were recorded in the patient’s residential history were combined, to create a ‘grand mean’ water fluoride concentration for each patient. Patients who had an individual water fluoride concentration ≥ 0.7 mg F/l were assigned to the optimally fluoridated group (exposed). Patients whose individual grand mean water fluoride concentration was < 0.7 mg F/l were assigned to the sub/non-optimally fluoridated group (unexposed). This method of exposure assignment overcomes the variability in water fluoridation dosing over time.
Study population
The flow diagram below (Figure 9) details the stages involved in the selection of the study population within the NHS BSA database, prior to transfer of the data set to the University of Manchester. One limitation of the pre-2016 patient identification methods used by BSA is that it is not possible to ensure the NHS BSA IDs represent a unique individual patient (prior to 1 : 1 matching between NHS number and NHS BSA index identifier).
FIGURE 9.
National Health Service BSA data validation and selection of the study population prior to transfer of data to the research team.
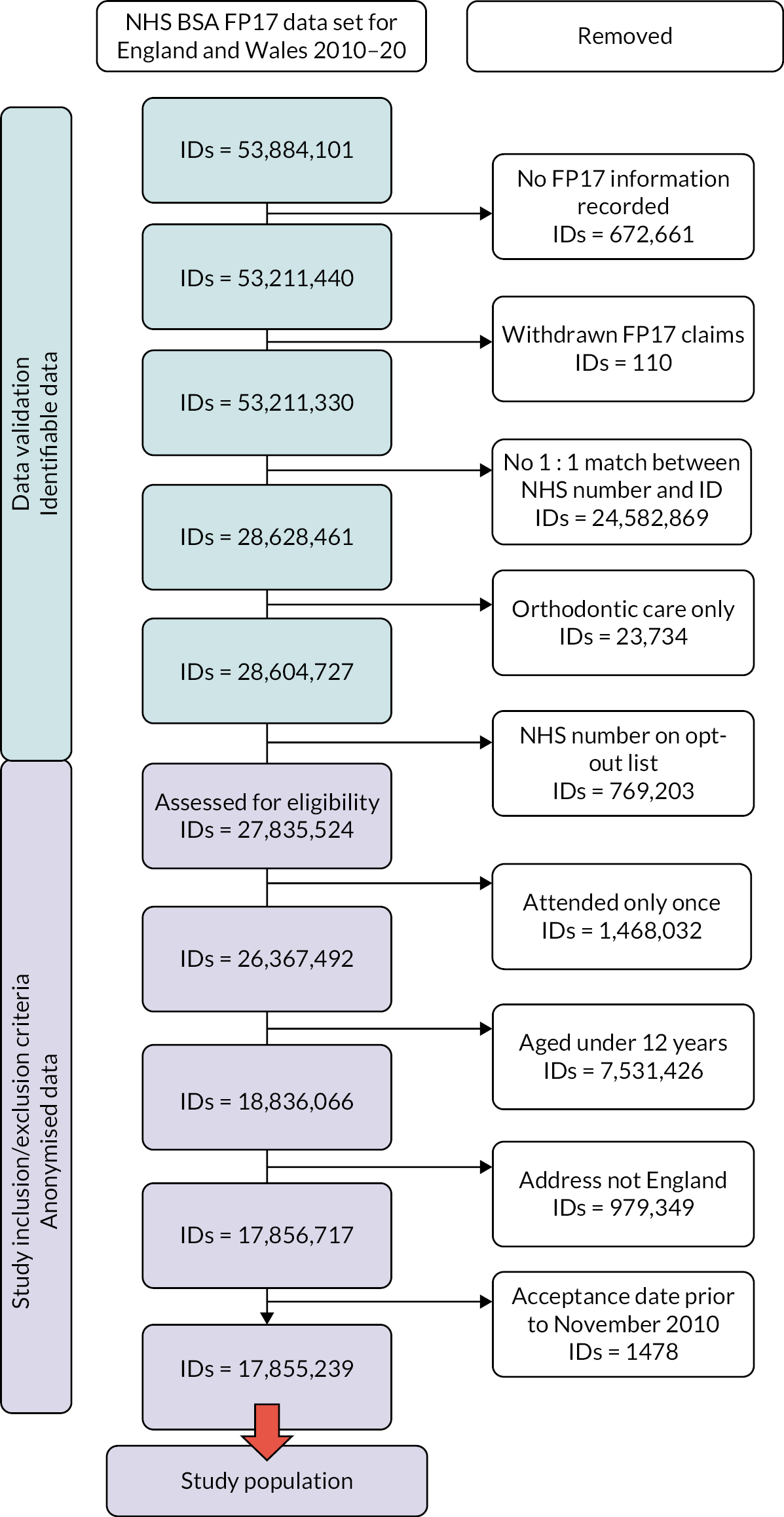
Propensity score matching results
We proposed to undertake seven different methods of propensity score matching:
-
Method 0: No matching
-
Method 1: Nearest neighbour, one to one matching
-
Method 2: Nearest neighbour, variable ratio matching (ratio = 3, maximum = 5)
-
Method 3: Nearest neighbour, variable ratio matching (ratio = 3, maximum = 5), with 0.25 calliper
-
Method 4: Optimal matching
-
Method 5: Optimal matching, variable ratio matching (ratio = 3, maximum = 5)
-
Method 6: Full matching
-
Method 7: Full matching with calliper.
The propensity score estimation and matching processes were conducted using the University of Manchester’s computationally intensive research platform (iCSF, aka Incline). The iCSF is a cluster of computer nodes that utilises a Linux system. Each node has 256 GB of RAM and uses two 14-core Intel Broadwell CPUs. Estimation and matching using nearest neighbour methods took, on average, 6 days to complete. Due to the increased complexity involved in estimation and matching using optimal and full matching (Methods 4–7), these methods failed to execute to completion.
Our matched analytical data set was based therefore on nearest neighbour matching. Of the three variants explored, nearest neighbour matching was selected based on our pre-specified criteria of greatest covariate balance and the number of observations/participants remaining in the exposed and non-exposed groups after matching.
Full details of all the completed estimation and matching methods are provided in Appendix 4, Tables 35–37. Results of the different methods are described briefly below with respect to the selection of the final matched data set for analysis.
Resulting matched data sets
-
Method 0 The unmatched data set consisted of 17,796,185 observations, with 1,592,570 individuals ascribed optimally fluoridated status and 16,203,615 individuals ascribed sub/non-optimally fluoridated status.
-
Method 1 (nearest neighbour, one to one matching): The use of nearest neighbour matching with a one-to-one match of controls (sub/non-optimally fluoridated) to treated individuals (optimally fluoridated) resulted in the smallest sample size of the nearest neighbour methods. Matches were found for all 159,270 exposed individuals and common support was found for all treated cases.
-
Method 2 (nearest neighbour, variable ratio matching): The use of nearest neighbour matching with a one-to-three variable ratio match of controls (sub/non-optimally fluoridated) to treated individuals (optimally fluoridated) resulted in a total sample size of 6,370,280, the largest of all nearest neighbour matches. Matches were found for all 159,270 exposed individuals and common support was found for all treated observations. No exposed observations were discarded.
-
Method 3 (nearest neighbour, variable ratio matching, calliper): The use of nearest neighbour with variable ratio matching and the addition of a 0.25 calliper resulted in a total sample size of 6,369,406. Four hundred and thirty-seven observations from the intervention (optimally fluoridated) group could not be matched within this calliper and were subsequently discarded from the matched data set. Consequently, the estimand using this method no longer corresponds to the ATT (exposed), but rather an undefined matched population.
The sample sizes for the three matching methods are reported in Table 4. The total sample size, with the numbers of matched and unmatched (discarded) individuals for the exposed and non-exposed groups are presented.
Matching method | 0 (No matching) | 1 | 2 | 3 |
---|---|---|---|---|
Sample size | 17,796,185 | 3,185,140 | 6,370,280 | 6,369,406 |
Matched optimally fluoridated | – | 1,592,570 | 1,592,570 | 1,592,133 |
Matched sub/non-optimally fluoridated | – | 1,592,570 | 4,777,710 | 4,777,273 |
Unmatched optimally fluoridated | 592,570 | 0 | 0 | 437 |
Unmatched sub/non-optimally fluoridated | 16,203,615 | 14,611,045 | 11,425,905 | 11,426,342 |
Matched optimally fluoridated (effective sample size) | 0 | 0 | 1,592,570 | 1,592,133 |
Matched sub/non-optimally fluoridated (effective sample size) | 0 | 0 | 2,654,283 | 2,654,041 |
Evaluation of covariate balance for methods 1 through 3 was undertaken through visual inspection and examination of descriptive statistics. Visual inspection of covariate balance was undertaken through Love plots, eQQ and kernel density plots for continuous covariates, and bar charts for categorical covariates. Balance was deemed to have been achieved on the eQQ plots where values fall on the 45° line, and for the kernel density plots where the lines of the exposed and non-exposed groups overlap. Additionally, values of absolute SMDs were used in preference to hypothesis tests to determine covariate balance, with SMDs below a threshold of 0.1 SDs considered to constitute adequate balance. Based on all the above information Model 2 was chosen to take forwards for further analysis.
Results indicated that methods 1 and 2 had worked well to produce a matched data set, convincingly meeting the threshold values for absolute SMD and used all available information from the exposed observations. With method 3, 437 treated cases were discarded from the matched data set, while the observed percentage balance improvement over the unmatched data set was the same as for method 2 (99.9%). Consequently, the matched data set resulting from method 3 was not taken forwards for analysis. Based on the larger sample size and the fact that variable ratio matching has been shown to remove more bias than one-to-one matching, we elected to take forwards method 2 as the matched data set for analysis.
Balance of the selected matched cohort
A Love plot showing the SMDs for the unmatched and matched analytical cohorts is shown in Figure 10. The dashed vertical lines in the Love plot represent a SMD of 0.1 SDs (the recommended threshold for adequate covariate balance without further regression-based adjustments). The solid vertical line represents a SMD of zero. The red indicators are the SMDs in the unmatched cohort and the blue indicators represent the SMDs in the selected matched cohort (method 2). All absolute standardised effect sizes (SMDs) of the matched sample were below the threshold of 0.1 SDs and were therefore considered to constitute adequate balance. Additional graphs evaluating covariate balance for method 2 are presented in Appendix 5, Figures 22–33. Table 5 presents summary balance statistics for the selected matched analytical cohort.
FIGURE 10.
Love plot illustrating SMD for the unmatched cohort and the matched cohort taken forwards for analysis (method 2).
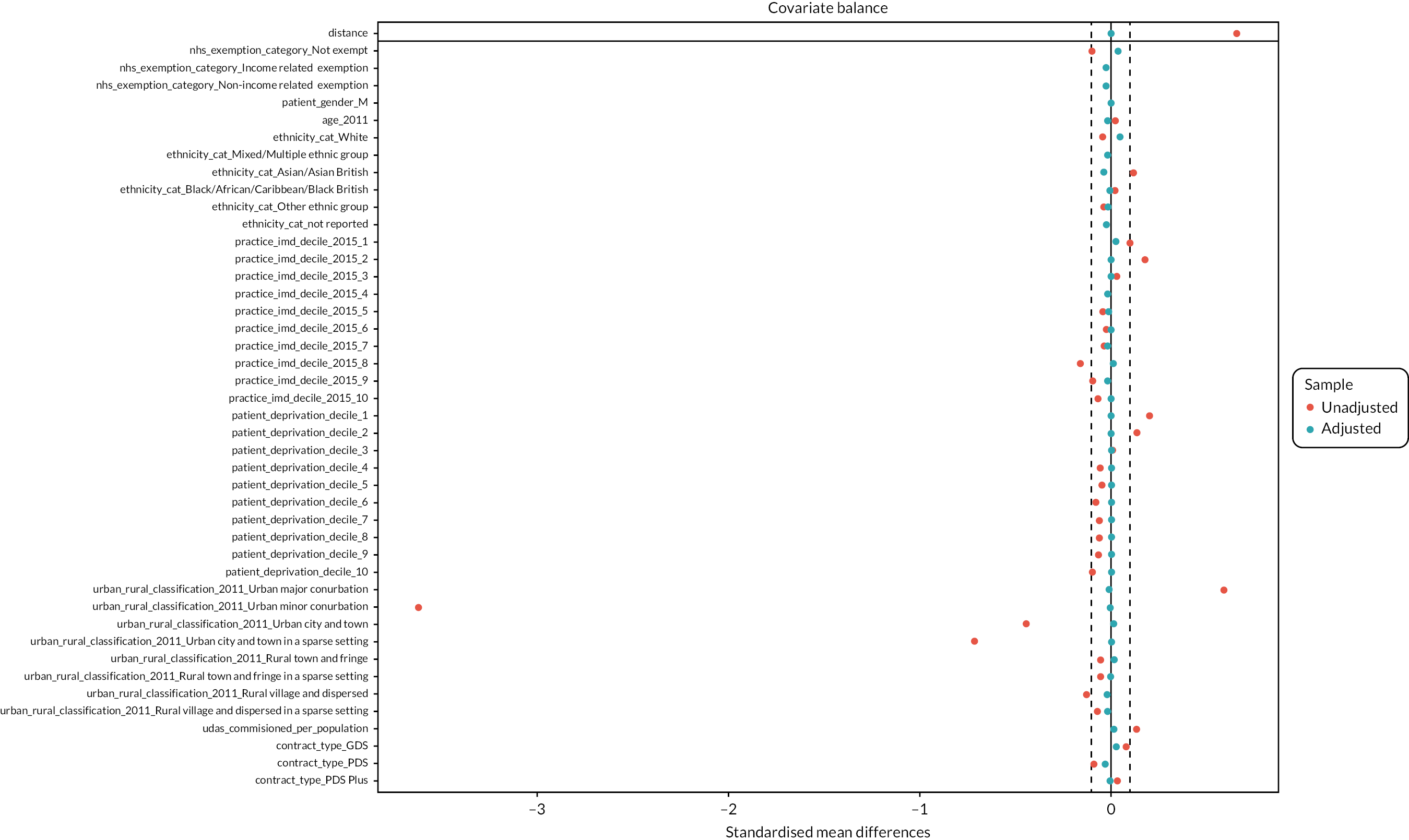
Matching variable | SMD | SMD improvement (%) | Matching variable | SMD | SMD improvement (%) |
---|---|---|---|---|---|
Overall distance | 0.0005 | 99.9 | |||
Female | 0 | 100 | Urban major conurbation | −0.0106 | 98.2 |
Male | 0 | 100 | Urban minor conurbation | −0.0005 | 100 |
Age | −0.02 | 19.7 | Urban city and town | 0.0126 | 97.2 |
Patient IMD Q1 | 0 | 100 | Urban city and town in a sparse setting | 0.0007 | 99.9 |
Patient IMD Q2 | 0 | 100 | Rural town and fringe | 0.0152 | 73.6 |
Patient IMD Q3 | 0 | 100 | Rural town and fringe in a sparse setting | −0.006 | 88.8 |
Patient IMD Q4 | 0 | 100 | Rural village and dispersed | −0.0169 | 86.8 |
Patient IMD Q5 | 0 | 100 | Rural village and dispersed in a sparse setting | −0.0151 | 77.9 |
Patient IMD Q6 | 0 | 100 | UDAs commissioned per person | 0.0104 | 92.2 |
Patient IMD Q7 | 0 | 100 | Dental contract type: GDS | 0.0308 | 60.4 |
Patient IMD Q8 | 0 | 100 | Dental contract type: PDS | −0.0301 | 66.8 |
Patient IMD Q9 | 0 | 100 | Dental contract type: PDS+ | −0.0063 | 81.5 |
Patient IMD Q10 | 0 | 100 | Practice IMD Q1 | 0.0259 | 74.2 |
White ethnicity | 0.0466 | −11.9 | Practice IMD Q2 | −0.0003 | 99.9 |
Mixed/multiple ethnicity | −0.0157 | −157.4 | Practice IMD Q3 | 0.0049 | 82.7 |
Asian/Asian British ethnicity | −0.038 | 66.1 | Practice IMD Q4 | −0.0194 | −67.8 |
Black/African/Caribbean/Black British ethnicity | −0.0006 | 96.4 | Practice IMD Q5 | −0.0109 | 70.3 |
Other ethnic group | −0.017 | 49.1 | Practice IMD Q6 | 0.0017 | 90.8 |
No ethnicity recorded (%) | −0.0217 | −31.7 | Practice IMD Q7 | −0.0168 | 47.3 |
NHS charges: not exempt | 0.0358 | 62.5 | Practice IMD Q8 | 0.0131 | 92 |
NHS charges: income-related exemption | −0.0254 | 77.1 | Practice IMD Q9 | −0.0145 | 84.7 |
NHS charges: non-income-related exemption | −0.0252 | −13.8 | Practice IMD Q10 | 0.0037 | 94.8 |
As shown in Table 5 the SMDs between the exposed and unexposed group were all below the recommended threshold for adequate covariate balance of 0.1. 142 The SMDs for the patient deprivation and sex variables are zero because exact matching was used for these variables. The per cent balance improvement is also shown which indicates how improved the SMD was by the matching procedure (compared to the unmatched data set).
Table 6 illustrates the variance ratio (VR) for the continuous variables of age and UDAs commissioned per person (local authority level). VR is the ratio of the variance of a covariate in one group to the variance of the same covariate in the comparator group. VRs close to one are considered to indicate good balance. 141
Matching variable | VR | VR improvement (%) |
---|---|---|
Overall distance | 1.0033 | 99.3 |
Age | 0.9733 | −381.5 |
UDAs commissioned per person | 1.2337 | −40.6 |
Selection of participants for analysis
Figure 11 illustrates the participant flow from receipt of the study population data set by The University of Manchester, to the final groups for analysis. A total of 17,855,239 patients were selected by NHS BSA for the study population and their anonymised dental treatment claims data were securely transferred to the University of Manchester data safe haven for further analysis by the research team. From this study population, there were 17,637 individuals who could not be assigned to an LSOA with an identified water fluoridation status. This is because there were 51 LSOAs that we did not receive any fluoride concentration data for (0.2% of LSOAs in England). Areas that were not covered included the Isles of Scilly, national parks, mountainous areas and private water supplies.
FIGURE 11.
Participant flow diagram.
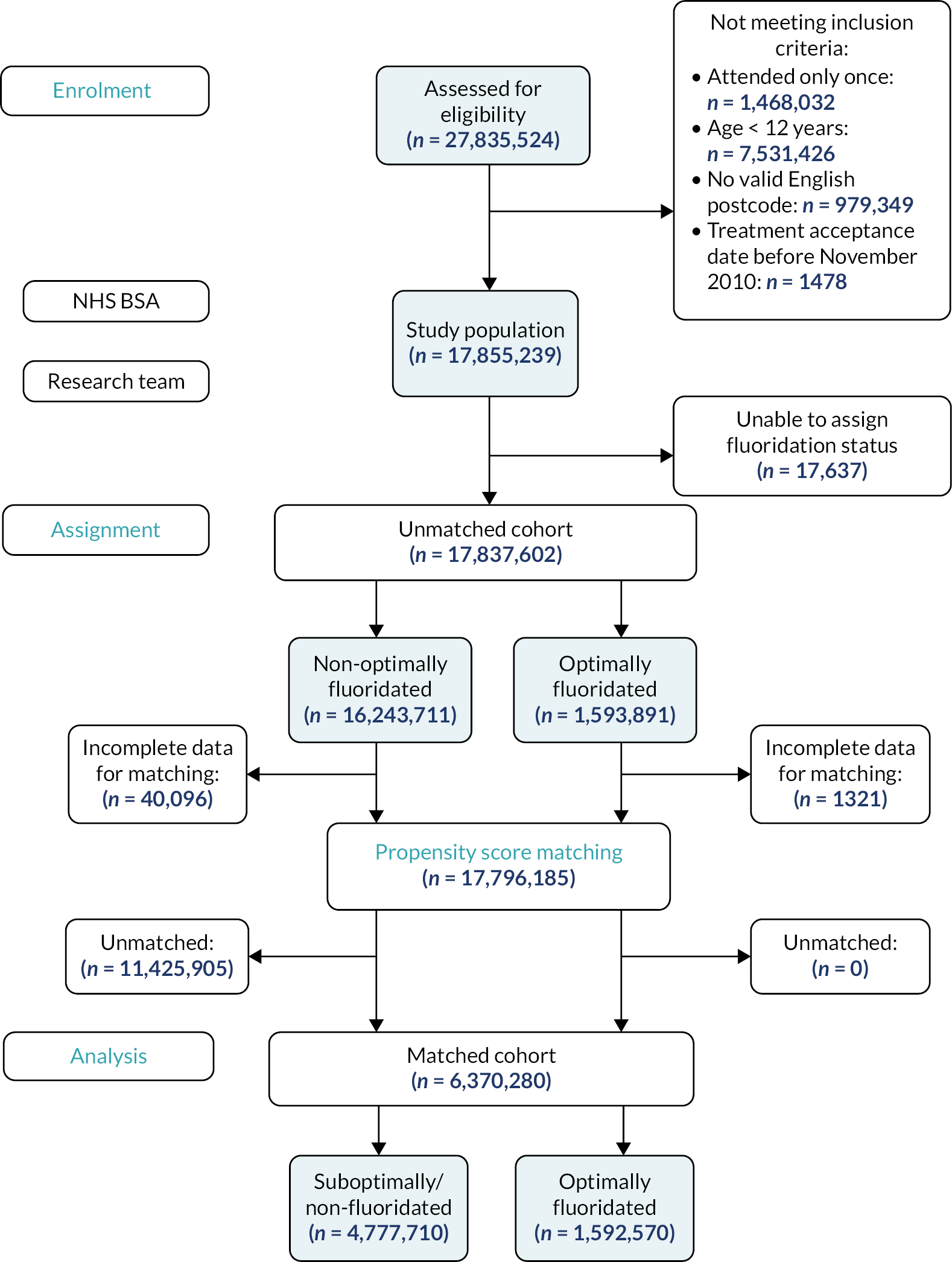
After assignment of 17,837,602 individuals to the full unmatched cohort, a total of 41,417 individuals (although living in England) had attended a dental practice in Wales for their first course of treatment. These individuals were excluded from the matching procedure because it was not possible to assign a ‘dental practice deprivation decile’ using the English Indices of Deprivation.
The matching procedures were carried out on a total of 17,796,185 individuals. Of these, 11,425,905 were excluded from the final matched cohort because they were not chosen as a suitable match. All excluded participants were from the sub/non-optimally fluoridated control group.
Baseline characteristics of the unmatched and matched cohorts
Table 7 presents the baseline individual-level characteristics of the unmatched (full) study cohort and the selected matched study cohort, by fluoridation group. Table 8 presents the baseline contextual characteristics for the same cohorts. The individual and contextual baseline characteristics presented are those which the propensity score matching was based on. All individual level and contextual baseline characteristics were derived from information recorded at the patient’s first course of dental treatment in the study observation period.
Cohort | Unmatched | Matched | ||
---|---|---|---|---|
Characteristics | Sub/non-optimally fluoridated | Optimally fluoridated | Sub/non-optimally fluoridated | Optimally fluoridated |
Total participants | 16,243,711 | 1,593,891 | 4,777,710 | 1,592,570 |
Sex | ||||
Male (%) | 7,227,473 (44.49) | 707,093 (44.36) | 2,119,584 (44.36) | 706,528 (44.36) |
Female (%) | 9,016,238 (55.51) | 886,798 (55.64) | 2,658,126 (55.64) | 886,042 (55.64) |
Age: mean (SD) | 42.66 (18.07) | 43.11 (18.03) | 43.47 (18.27) | 43.11 (18.03) |
Deprivation quintile | ||||
IMD Q1 (%) | 1,508,826 (9.29) | 271,545 (17.04) | 813,723 (17.03) | 271,241 (17.03) |
IMD Q2 (%) | 1,549,589 (9.54) | 228,849 (14.36) | 685,104 (14.34) | 228,368 (14.34) |
IMD Q3 (%) | 1,625,795 (10.01) | 164,686 (10.33) | 493,686 (10.33) | 164,562 (10.33) |
IMD Q4 (%) | 1,665,910 (10.26) | 138,048 (8.66) | 413,940 (8.66) | 137,980 (8.66) |
IMD Q5 (%) | 1,669,258 (10.28) | 141,958 (8.91) | 425,658 (8.91) | 141,886 (8.91) |
IMD Q6 (%) | 1,682,393 (10.36) | 130,876 (8.21) | 392,451 (8.21) | 130,817 (8.21) |
IMD Q7 (%) | 1,664,049 (10.24) | 135,444 (8.50) | 406,113 (8.50) | 135,371 (8.50) |
IMD Q8 (%) | 1,663,949 (10.24) | 136,154 (8.54) | 408,306 (8.55) | 136,102 (8.55) |
IMD Q9 (%) | 1,634,394 (10.06) | 131,645 (8.26) | 394,791 (8.26) | 131,597 (8.26) |
IMD Q10 (%) | 1,579,548 (9.72) | 114,686 (7.20) | 343,938 (7.20) | 114,646 (7.20) |
Ethnicity | ||||
White (%) | 11,112,050 (68.41) | 1,058,370 (66.40) | 3,068,228.4 (64.22) | 1,057,826 (66.42) |
Mixed/multiple (%) | 170,337 (1.05) | 15,792 (0.99) | 54,712.8 (1.15) | 15,762 (0.99) |
Asian/Asian British (%) | 655,708 (4.04) | 109,810 (6.89) | 374,725.2 (7.84) | 109,605 (6.88) |
Black/African/Caribbean/Black British (%) | 268,743 (1.65) | 29,894 (1.88) | 89,608.2 (1.88) | 29,748 (1.87) |
Other ethnic group (%) | 214,010 (1.32) | 15,763 (0.99) | 55,264.8 (1.16) | 15,744 (0.99) |
No information recorded (%) | 3,822,863 (23.53) | 364,262 (22.85) | 1,135,170.6 (23.76) | 363,885 (22.85) |
NHS exemption status | ||||
Not exempt (%) | 11,884,863 (73.17) | 1,095,157 (68.71) | 3,204,184.8 (67.07) | 1,094,527 (68.73) |
Income-related exemption (%) | 3,497,084 (21.53) | 421,828 (26.47) | 1,317,265.2 (27.57) | 421,219 (26.45) |
Non-income-related exemption (%) | 861,764 (5.31) | 76,906 (4.83) | 256,260 (5.36) | 76,824 (4.82) |
Cohort | Unmatched | Matched | ||
---|---|---|---|---|
Characteristic | Sub/non-optimally fluoridated | Optimally fluoridated | Sub/non-optimally fluoridated | Optimally fluoridated |
Total participants | 16,243,711 | 1,593,891 | 4,777,710 | 1,592,570 |
Rural–urban classification of patient residence | ||||
Urban major conurbation (%) | 5,027,780 (30.95) | 958,498 (60.14) | 2,896,921.2 (60.63) | 957,372 (60.11) |
Urban minor conurbation (%) | 726,672 (4.47) | 243 (0.02) | 757.2 (0.02) | 243 (0.02) |
Urban city and town (%) | 7,566,731 (46.58) | 429,286 (26.93) | 1,260,981.6 (26.39) | 429,197 (26.95) |
Urban city and town in a sparse setting (%) | 35,744 (0.22) | 15 (0.00) | 34.8 (0.00) | 15 (0.00) |
Rural town and fringe (%) | 1,548,791 (9.53) | 126,808 (7.96) | 360,641.4 (7.55) | 126,760 (7.96) |
Rural town and fringe in a sparse setting (%) | 65,096 (0.40) | 2661 (0.17) | 9157.8 (0.19) | 2661 (0.17) |
Rural village and dispersed (%) | 1,180,994 (7.27) | 72,808 (4.57) | 235,089 (4.92) | 72,750 (4.57) |
Rural village and dispersed in a sparse setting (%) | 91,903 (0.57) | 3572 (0.22) | 14,127 (0.30) | 3572 (0.22) |
Local dental services commissioning | ||||
Number of UDAs per person within home LA: mean (SD) | 1.63 (0.32) | 1.68 (0.34) | 1.67 (0.31) | 1.68 (0.34) |
Dental contract type | ||||
GDS (%) | 15,025,375 (92.50) | 1,503,660 (94.34) | 4,473,114 (93.62) | 1,502,389 (94.34) |
PDS (%) | 1,185,080 (7.30) | 83,400 (5.23) | 282,129 (5.91) | 83,350 (5.23) |
PDS+ (%) | 33,246 (0.20) | 6831 (0.43) | 22,467 (0.47) | 6831 (0.43) |
Dental practice deprivation quintile | ||||
IMD Q1 (%) | 1,904,514 (11.72) | 244,842 (15.36) | 689,879.4 (14.44) | 244,842 (15.37) |
IMD Q2 (%) | 1,773,540 (10.92) | 285,554 (17.92) | 857,146.2 (17.94) | 285,554 (17.93) |
IMD Q3 (%) | 1,816,828 (11.18) | 193,197 (12.12) | 572,013 (11.97) | 193,197 (12.13) |
IMD Q4 (%) | 1,755,013 (10.80) | 166,862 (10.47) | 528,919.2 (11.07) | 166,862 (10.48) |
IMD Q5 (%) | 1,567,532 (9.65) | 137,666 (8.64) | 427,604.4 (8.95) | 137,666 (8.64) |
IMD Q6 (%) | 1,270,244 (7.82) | 116,938 (7.34) | 348,634.8 (7.30) | 116,938 (7.34) |
IMD Q7 (%) | 1,183,216 (7.28) | 103,791 (6.51) | 331,149.6 (6.93) | 103,791 (6.52) |
IMD Q8 (%) | 2,762,265 (17.01) | 187,508 (11.76) | 542,346.6 (11.35) | 187,508 (11.77) |
IMD Q9 (%) | 1,148,166 (7.07) | 79,924 (5.01) | 254,931.6 (5.34) | 79,924 (5.02) |
IMD Q10 (%) | 1,022,297 (6.29) | 76,288 (4.79) | 225,085.2 (4.71) | 76,288 (4.79) |
Clinical results
Clinical characteristics and health outcomes for the unmatched and matched cohorts
Table 9 presents descriptive summary statistics for the clinical characteristics of the unmatched and matched cohorts overall and by water fluoridation status. The clinical characteristics of the matched and unmatched cohorts are relatively similar. This provides reassurance the findings from the matched cohort can be generalised to the wider population of NHS dental service users.
Unmatched cohort | Matched cohort | |||||
---|---|---|---|---|---|---|
Sub/non-optimally fluoridated | Optimally fluoridated | Total | Sub/non-optimally fluoridated | Optimally fluoridated | Total | |
Participants (n) | 16,243,711 | 1,593,891 | 17,837,602 | 4,777,710 | 1,592,570 | 6,370,280 |
Number of courses of treatment: mean (SD) | 10.31 (6.20) | 9.92 (6.11) | 10.27 (6.19) | 10.13 (6.31) | 9.92 (6.11) | 10.08 (6.26) |
Number of fillings received: mean (SD) | 3.87 (4.90) | 3.84 (4.92) | 3.87 (4.90) | 3.95 (5.03) | 3.84 (4.92) | 3.92 (5.00) |
Number of extractions received: mean (SD) | 1.00 (1.99) | 1.10 (2.13) | 1.01 (2.01) | 1.10 (2.11) | 1.10 (2.13) | 1.10 (2.11) |
Number of invasive treatments: mean (SD) | 5.41 (6.08) | 5.44 (6.09) | 5.41 (6.08) | 5.62 (6.26) | 5.44 (6.09) | 5.57 (6.02) |
Received invasive treatments: n (%) | 13,604,955 (83.76) | 1,346,391 (84.47) | 14,951,346 (83.82) | 4,040,138 (84.56) | 1,345,328 (84.48) | 6,370,280 (84.54) |
Participants (n)160 | 15,782,449 | 1,547,114 | 17,329,563 | 4,097,227 | 1,419,879 | 5,517,106 |
DMFT: mean (SD) | 13.13 (8.70) | 13.12 (8.72) | 13.13 (8.70) | 13.36 (8.86) | 13.15 (8.73) | 13.31 (8.83) |
Number of missing teeth: mean (SD) | 6.24 (6.75) | 6.63 (7.10) | 6.28 (6.78) | 6.65 (7.16) | 6.65 (7.12) | 6.65 (7.15) |
We carried out an analysis of DMFT and missing teeth by age group, to investigate the possibility that differences in the number of missing teeth may be more sensitive to the effects of water fluoridation in older people, whereas decayed and filled teeth may be more sensitive in younger people. This was informed by recent studies from the UK and Australia which suggest this may be the case. 6,66,80 Age 45 was chosen as the cut-point because this is the age at which differences have been reported previously. 66
These analyses were carried out on the full unmatched cohort because it would not be possible to split the matched cohort by age without splitting the matched subclasses.
Tables 10 and 11 show that the mean DMFT and mean number of missing teeth are very similar between the optimally fluoridated and sub/non-optimally fluoridated groups, in both the under 45 and 45 and over age groups.
N = 17,329,563 | Unmatched cohort DMFT (mean, SD) | ||
---|---|---|---|
Age group | Sub/non-optimally fluoridated | Optimally fluoridated | Total |
Under 45 | 8.63 (6.62) | 8.38 (6.42) | 8.61 (6.60) |
45 and over | 18.54 (7.78) | 18.56 (7.79) | 18.54 (7.78) |
Total | 13.13 (8.70) | 13.12 (8.72) | 13.13 (8.70) |
N = 17,329,563 | Unmatched cohort missing teeth (mean, SD) | ||
---|---|---|---|
Age group | Sub/non-optimally fluoridated | Optimally fluoridated | Total |
Under 45 | 3.40 (3.84) | 3.45 (3.90) | 3.40 (3.85) |
45 and over | 9.67 (7.82) | 10.72 (8.12) | 9.73 (7.85) |
Total | 6.24 (6.75) | 6.63 (7.10) | 6.28 (6.78) |
Primary outcome: invasive dental treatments
The primary research question of interest was whether water fluoridation reduced the number of invasive dental treatments (restorations, endodontics, extractions) received by adults attending NHS dental practices over 10 years of observation (22 November 2010 to 21 October 2020).
Outcome information was available for 6,370,280 observations in total, with 4,777,710 observations in the sub/non-optimally fluoridated group and 1,592,570 in the optimally fluoridated group. The mean number of invasive treatments per person in the matched study sample overall was 5.573 treatments (SE 0.003), with a similar number of mean treatments per person in the sub/non-optimally fluoridated (5.616, SE 0.005) and optimally fluoridated groups (5.443, SE 0.005). The median number of invasive treatments was four, with a maximum number of invasive dental treatments of 271 in a distribution that was positively skewed (Figure 12 and Table 12). Overall, 84.5% patients in the matched sample received at least one invasive dental treatment in the 10-year period of observation.
FIGURE 12.
Histogram illustrating the distribution of the total number of invasive dental treatments per patient.
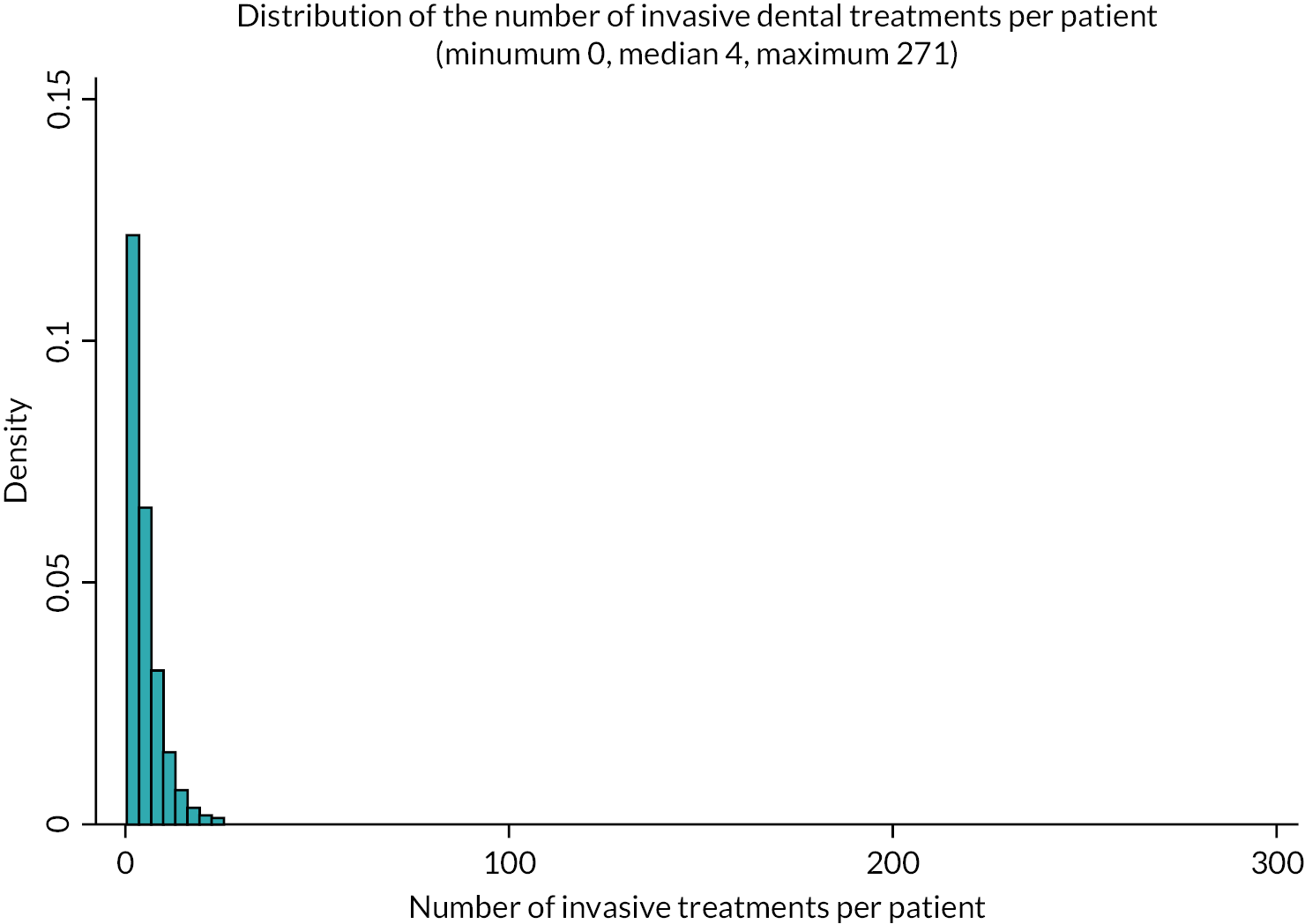
Primary outcome: number of invasive dental treatments | Sub/non-optimally fluoridated | Optimally fluoridated | Total |
---|---|---|---|
Secondary outcome: DMFT | Sub/non-optimally fluoridated | Optimally fluoridated | Total |
Secondary outcome: number of missing teeth | Sub/non-optimally fluoridated | Optimally fluoridated | Total |
N | 4,777,710 | 1,592,570 | 6,370,280 |
Mean (SE) | 5.616 (0.004) | 5.443 (0.005) | 5.573 (0.003) |
Q1, Q50, Q75 | 1, 4, 8 | 1, 4, 8 | 1, 4, 8 |
Minimum, maximum | 0, 271 | 0, 248 | 0, 271 |
N | 4,097,227 | 1,419,879 | 5,517,106 |
Mean (SE) | 13.361 (0.006) | 13.149 (0.007) | 13.308 (0.005) |
Q1, Q50, Q75 | 6, 13, 20 | 6, 13, 20 | 6, 13, 20 |
Minimum, maximum | 0, 32 | 0, 32 | 0, 32 |
N | 4,097,227 | 1,419,879 | 5,517,106 |
Mean (SE) | 6.646 (0.005) | 6.652 (0.006) | 6.647 (0.004) |
Q1, Q50, Q75 | 2, 4, 9 | 2, 4, 9 | 2, 4, 9 |
Minimum, maximum | 0, 32 | 0, 32 | 0, 32 |
As the outcome is a count of the total number of invasive treatments received, we used a negative binomial regression to compare the groups and determine the effect estimate, accounting for the clustering of the data through cluster robust SE and weighting the data. A negative binomial regression was chosen rather than a Poisson regression to account for the substantial overdispersion in the distribution for this outcome.
The IRR was 0.969 (95% CI 0.967 to 0.971), indicating the rate of invasive treatments in the optimally fluoridated group was 3% less than that of the sub/non-fluoridated group. The predicted count of the number of invasive treatments from this model was 5.616 (SE 0.004) for the sub/non-optimally fluoridated group and 5.443 (SE 0.005) for the optimally fluoridated group. There was a predicted mean difference of 0.173 fewer invasive dental treatments per person (95% CI 0.185 fewer to 0.161 fewer) in the optimally fluoridated group compared to the sub/non-optimally fluoridated group.
To aid with readers with the interpretation of the relevance of any differences in the primary outcome, we carried out stakeholder engagement to identify what would be considered to be a meaningful ‘per person’ reduction in the number of invasive dental treatments (for everyone in a hypothetical town), as a result of water fluoridation. In the engagement workshops we presented a scenario that six fillings or extractions would be received in the sub/non-optimally fluoridated group over 10 years and sought views on what size of reduction would be considered meaningful. The scenario presented during the workshops was in fact very similar to what we found, with the mean number of invasive dental treatments received in the sub/non-optimally fluoridated group in our matched cohort being 5.6. This makes the comparison of the effect size for our primary outcome against stakeholder views fairly straightforward with regard to relative and absolute differences.
During the engagement workshops, most participants felt that an average reduction of at least one invasive NHS dental treatment per person over 10 years would be needed to be considered as meaningful to them (a 16% relative reduction in the scenario). The dentists present did consider smaller reductions of 0.30 fewer invasive dental treatments per person to be meaningful (a 5% relative reduction in the example scenario), but only a minority of all stakeholders (4 out of 54) felt the smallest reduction presented in the scenarios (0.18 fewer treatments, a relative difference of 3%) would be considered meaningful. Effects sizes of 3% relative difference or smaller have previously been referred to as ‘exceedingly small’ in the epidemiological literature. 161
Secondary outcomes
The secondary outcomes of interest in this study were the number of DMFT and the number of missing teeth.
Secondary outcome: DMFT
As recording of DMFT was only mandated on the FP17 form from April 2016, DMFT data are only available from 2016 to 2020. The most recent DMFT value, that is, DMFT recorded at the dental visit closest to the end of the data collection period 2020, was used for data analysis.
Outcome information was available for a total of 5,517,106 patients: 4,097,227 in the sub/non-optimally fluoridated group and 1,419,879 in the optimally fluoridated group. The mean DMFT in the subset of the matched study sample for which DMFT was recorded was 13.308 (SE 0.005), a mean DMFT in the sub/non-optimally fluoridated group of 13.361 (SE 0.006) and 13.149 (SE 0.007) in the optimally fluoridated group (see Table 12).
We used a negative binomial regression to compare the groups and determine the effect estimate, accounting for the clustering of the data through cluster robust SE and weighting the data. A negative binomial regression was chosen rather than a Poisson regression to account for the substantial overdispersion in the data for this outcome. The IRR was 0.984 (95% CI 0.983 to 0.985), indicating the rate of DMFT in the optimally fluoridated group was 2% less than in the sub/non-optimally fluoridated group.
The predicted mean count of DMFT in the negative binomial model was 13.361 (SE 0.006) per person in the sub/non-optimally fluoridated group and 13.149 (SE 0.007) per person in the optimally fluoridated group. There was a predicted mean difference of 0.212 fewer DMFT (95% CI 0.229 fewer to 0.194 fewer) in the optimally fluoridated group compared to the sub/non-optimally fluoridated group.
Secondary outcome: number of missing teeth
As recording of DMFT was only mandated on the FP17 from April 2016, the M (missing tooth) component of DMFT data is only available from 2016 to 2020. The most recent missing component score, that is, number of missing teeth recorded at the dental visit closest to the end of the data collection period 2020, was used for data analysis.
Outcome information was available for a total of 5,517,106 observations: 4,097,227 observations in the sub/non-optimally fluoridated group and 1,419,879 in the optimally fluoridated group. The mean number of missing teeth in the subset of the matched study sample for which DMFT was recorded was 6.647 (SE 0.004), a mean number of missing teeth in the sub/non-optimally fluoridated group of a mean of 6.646 (SE 0.005) and 6.652 (SE 0.006) in the optimally fluoridated group (see Table 12).
We used a negative binomial regression to compare the groups and determine the effect estimate, accounting for the clustering of the data through cluster robust SE and weighting the data. A negative binomial regression was chosen rather than a Poisson regression to account for the substantial overdispersion in the data for this outcome. The IRR was 1.001 (95% CI 0.999 to 1.003), indicating an equivocal effect of water fluoridation. The predicted mean number of missing teeth in the sub/non-optimally fluoridated group was 6.646 (SE 0.005) missing teeth per person, compared to 6.652 (SE 0.006) missing teeth per person in the optimally fluoridated group. There was a predicted mean difference of 0.006 more missing teeth (95% CI 0.008 fewer to 0.021 more) in the optimally fluoridated group compared to the sub/non-optimally fluoridated group.
Secondary objective: social inequalities
In order to determine whether there was any differential effect of water fluoridation according to social deprivation on the primary and secondary outcomes we repeated the previous analysis, but this time added an interaction term to the regression analysis that included the main effects of water fluoridation and deprivation decile. This allows the effect of water fluoridation within each decile of deprivation to be evaluated. To facilitate interpretation the predicted counts for the primary and secondary outcomes were calculated and plotted using the margins command in Stata. For all analyses, initial Poisson regression models indicated substantial overdispersion, that was accounted for by negative binomial Regression models that proved a better model fit to the data.
Primary outcome: invasive dental treatments
The summary estimates for number of invasive dental treatments are presented in Table 13 by water fluoridation status and deprivation decile. There was a clear pattern to the mean number of invasive dental treatments according to area-level deprivation for this outcome, overall and by group. From the most deprived groups (IMD decile 1) to the least deprived groups (IMD decile 10), the mean number of invasive treatments received decreases. This pattern is evident in both the optimally fluoridated and sub/non-optimally fluoridated groups.
Deprivation decile (IMD) | Sub/non-fluoridated N, mean (SE) |
Optimally fluoridated N, mean (SE) |
Total N, mean (SE) |
---|---|---|---|
1 (most deprived) | 813,723 6.324 (0.012) |
271,241 5.988 (0.012) |
1,084,964 6.241 (0.010) |
2 | 685,104 6.056 (0.012) |
228,368 5.788 (0.013) |
913,472 5.989 (0.010) |
3 | 493,686 5.756 (0.013) |
164,562 5.675 (0.015) |
658,248 5.736 (0.010) |
4 | 413,940 5.565 (0.012) |
137,980 5.455 (0.016) |
551,920 5.537 (0.010) |
5 | 425,658 5.459 (0.012) |
141,886 5.278 (0.016) |
567,544 5.414 (0.010) |
6 | 392,451 5.357 (0.011) |
130,817 5.171 (0.016) |
523,268 5.310 (0.009) |
7 | 406,113 5.286 (0.011) |
135,371 5.175 (0.016) |
541,484 5.259 (0.009) |
8 | 408,306 5.159 (0.011) |
136,102 5.097 (0.016) |
544,408 5.143 (0.009) |
9 | 394,791 5.057 (0.011) |
131,597 5.001 (0.016) |
526,388 5.043 (0.009) |
10 (least deprived) | 343,938 4.985 (0.012) |
114,646 4.867 (0.017) |
458,584 4.956 (0.010) |
Total | 4,777,710 5.616 (0.004) |
1,592,570 5.443 (0.005) |
6,370,280 5.573 (0.003) |
We modelled the effects of water fluoridation and area-level deprivation on the number of invasive dental treatments using a negative binomial regression model with main and interaction effects, cluster robust SE and accounting for weighting. The number of observations for this outcome was 6,370,280.
The conditional effect estimate for the rate of invasive dental treatments according to water fluoridation status from the model with the interaction term was IRR 0.976 (95% CI 0.968 to 0.984). The predicted marginal mean values for the number of invasive dental treatments were 5.443 (SE 0.005) in the optimally fluoridated group and 5.616 (SE 0.004) in the sub/non-optimally fluoridated group. The effect estimates from the regression model for the main and interaction terms along with their CIs are reported in Appendix 6, Tables 38–40
Figure 12 illustrates the mean predicted number of invasive dental treatments for the optimally fluoridated and sub/non-optimally fluoridated groups at each deprivation decile, from the negative binomial regression model. The mean predicted number of invasive dental treatments for the matched sample overall is highest for the most deprived decile (IMD decile 1), decreasing steadily to the least deprived decile (IMD decile 10). Compared to the effect of water fluoridation in deprivation decile 10, the reference category of the least deprived, the effect of water fluoridation is greatest in most deprived deciles 1 and 2.
Table 14 shows the discrete changes in the predicted number of invasive treatments (the difference in the predicted values of the mean number of invasive treatments in the optimally fluoridated group and the mean number in the sub/non-optimally fluoridated group) at each deprivation decile. In IMD decile 1 (most deprived), the predicted mean number of invasive dental treatments received is 0.337 lower (95% CI −0.371 to −0.302) in the optimally fluoridated group compared to the sub/non-optimally fluoridated group. In IMD decile 2, the predicted mean number of invasive dental treatments received is 0.268 lower (95% CI −0.304 to −0.233) lower in the optimally fluoridated group compared to the sub/non-optimally fluoridated group (Figure 13 and Table 11). In the remaining deciles the differences are smaller. The effects of water fluoridation do not bring the mean number of predicted invasive dental treatments in the more deprived deciles in line with those of the least deprived deciles.
Deprivation decile (IMD) | Difference in the predicted mean number of invasive dental treatments (95% CI) (negative values favour water fluoridation) | p > |z| |
---|---|---|
1 (most deprived) | −0.337 (−0.371 to −0.302) | 0.000 |
2 | −0.268 (−0.304 to −0.233) | 0.000 |
3 | −0.080 (−0.119 to −0.042) | 0.000 |
4 | −0.110 (−0.149 to −0.070) | 0.000 |
5 | −0.181 (−0.220 to −0.143) | 0.000 |
6 | −0.186 (−0.224 to −0.147) | 0.000 |
7 | −0.111 (−0.149 to −0.074) | 0.000 |
8 | −0.062 (−0.099 to −0.025) | 0.001 |
9 | −0.055 (−0.093 to −0.018) | 0.004 |
10 (least deprived) | −0.118 (−0.158 to −0.078) | 0.000 |
FIGURE 13.
Predicted number of invasive dental treatments with 95% CIs by water fluoridation status and patient deprivation decile from negative binomial regression model.
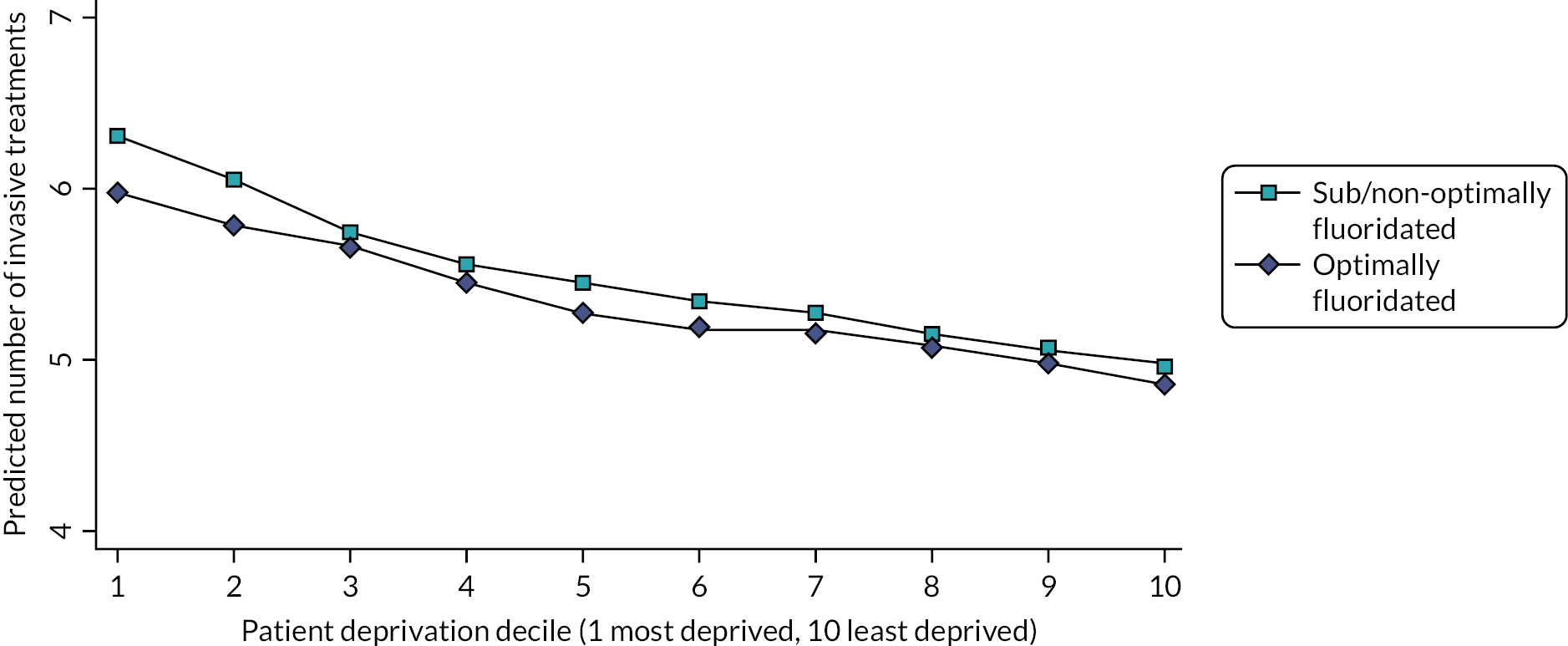
The predicted effects of optimal water fluoridation on the number of invasive dental treatments received within each decile would not have been considered meaningful by most stakeholders that we engaged with, though the dentists we consulted did consider differences as small as 0.3 fewer invasive dental treatments to be meaningful.
Secondary outcome: DMFT
The summary estimates for DMFT are presented in Table 15 by water fluoridation status and deprivation decile for the subset of the matched sample post 2016. There was no clear association of DMFT count with area-level deprivation overall or by water fluoridation status.
Deprivation decile (IMD) | Sub/non-optimally fluoridated (n = 4,097,227) N, mean (SE) |
Optimally fluoridated (n = 1,419,879) N, mean (SE) |
Total (n = 5,517,106) N, mean (SE) |
---|---|---|---|
1 (most deprived) | 349,879 13.009 (0.017) |
250,347 12.024 (0.018) |
600,226 12.763 (0.014) |
2 | 399,225 13.229 (0.018) |
207,813 12.804 (0.019) |
607,038 13.123 (0.015) |
3 | 396,660 13.189 (0.019) |
146,300 13.262 (0.022) |
542,960 13.207 (0.016) |
4 | 418,761 13.182 (0.019) |
120,457 13.315 (0.025) |
539,218 13.216 (0.016) |
5 | 403,014 13.331 (0.019) |
125,162 13.506 (0.024) |
528,176 13.375 (0.016) |
6 | 437,699 13.606 (0.018) |
113,687 13.616 (0.026) |
551,386 13.608 (0.015) |
7 | 439,602 13.654 (0.018) |
118,650 13.722 (0.025) |
558,252 13.671 (0.015) |
8 | 443,079 13.595 (0.018) |
119,691 13.774 (0.025) |
562,770 13.639 (0.015) |
9 | 434,143 13.634 (0.018) |
115,859 13.637 (0.025) |
550,002 13.635 (0.015) |
10 (least deprived) | 375,165 13.789 (0.019) |
101,913 13.346 (0.027) |
477,078 13.676 (0.017) |
Total | 4,097,227 13.361 (0.006) |
1,419,879 13.149 (0.007) |
5,517,106 13.308 (0.005) |
We modelled the effects of water fluoridation and area-level deprivation on DMFT using a negative binomial model with main and interaction effects, cluster robust SE and accounting for weighting. The number of observations for this outcome was 5,517,106. The conditional effect estimate for the effect of water fluoridation was IRR 0.968 (95% CI 0.964 to 0.973), with predicted mean values for the DMFT count of 13.149 (SE 0.007) in the optimally fluoridated group and 13.361 (SE 0.006) in the sub/non-optimally fluoridated group. The effect estimates for the main and interaction terms along with their CIs are reported in Appendix 6, Tables 38–40.
Figure 13 illustrates the predicted mean DMFT count for the optimally fluoridated and sub/non-optimally fluoridated groups at each deprivation decile from the negative binomial model. Table 16 shows the discrete changes in the predicted mean DMFT count (the difference in the predicted values of the mean DMFT count in the optimally fluoridated group and the mean number in the sub/non-optimally fluoridated group) at each deprivation decile.
Deprivation decile (IMD) | Difference in the predicted mean DMFT count (95% CI) (negative values favour water fluoridation) | p > |z| |
---|---|---|
1 (most deprived) | −0.986 (−1.033 to −0.939) | 0.000 |
2 | −0.426 (−0.476 to −0.375) | 0.000 |
3 | 0.073 (0.016 to 0.13) | 0.012 |
4 | 0.132 (0.072 to 0.192) | 0.000 |
5 | 0.174 (0.116 to 0.233) | 0.000 |
6 | 0.01 (−0.049 to 0.069) | 0.740 |
7 | 0.068 (0.01 to 0.126) | 0.022 |
8 | 0.179 (0.121 to 0.236) | 0.000 |
9 | 0.004 (−0.054 to 0.062) | 0.894 |
10 (least deprived) | −0.440 (−0.501 to −0.378) | 0.000 |
Unlike the analyses for the primary outcome, where a social gradient in the expected direction from most to least deprived was evident, there was no such pattern for DMFT. In IMD decile 1, the predicted mean DMFT count is 0.986 lower (95% CI 1.033 lower to 0.939 lower) in the optimally fluoridated group compared to the sub/non-optimally fluoridated group. In IMD decile 2, the predicted mean DMFT count is 0.426 lower (95% CI 0.476 lower to 0.375 lower) in the optimally fluoridated group compared to the sub/non-optimally fluoridated group (Figure 14 and Table 16). However, the direction of effect is not consistent across the deciles. In IMD deciles 3–9, the mean predicted DMFT count is either very similar in both groups (IMD deciles 6, 7 and 9) or is slightly higher in the fluoridated group than in the sub/non-optimally fluoridated group (IMD deciles 3, 4, 5 and 8).
FIGURE 14.
Predicted number of DMFT with 95% CIs by water deprivation status and deprivation decile from negative binomial regression model.
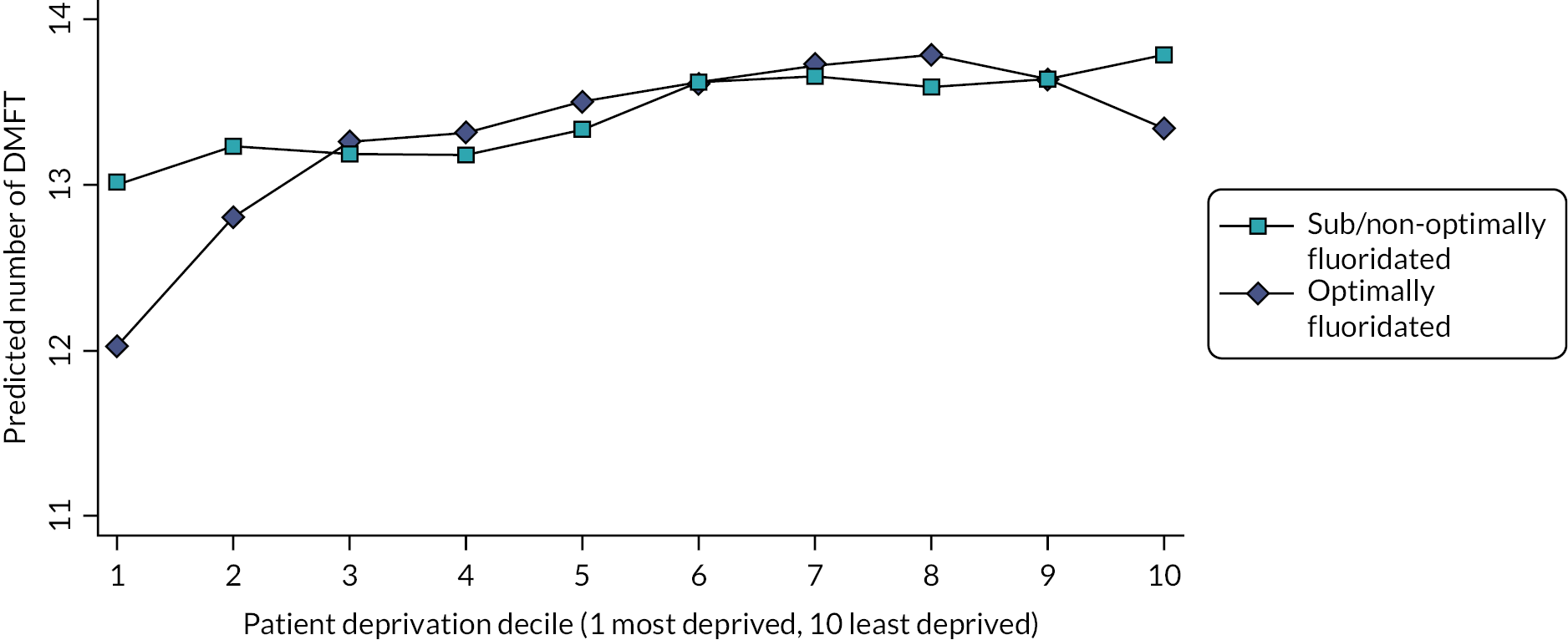
Secondary outcome: number of missing teeth
The summary estimates for the number of missing teeth are presented in Table 17 by water fluoridation and deprivation decile. Similar to the mean number of invasive dental treatments but different to DMFT, there was evidence of a clear pattern according to area-level deprivation on this outcome for the subset of the matched sample post 2016 overall and by water fluoridation status. As level of deprivation decreases, from the most deprived (IMD decile 1) to the least deprived groups (IMD decile 10), the mean number of missing teeth decreases, and this pattern is evident in the sub/non-optimally fluoridated group and for most deciles of the optimally fluoridated group.
Deprivation decile (IMD) | Sub/non-fluoridated (n = 4,097,227) N, mean (SE) |
Optimally fluoridated (n = 1,419,879) N, mean (SE) |
Total (n = 5,517,106) N, mean (SE) |
---|---|---|---|
1 (most deprived) | 349,879 7.199 (0.015) |
250,347 6.724 (0.015) |
600,226 7.081 (0.012) |
2 | 399,225 7.160 (0.016) |
207,813 6.987 (0.017) |
607,038 7.117 (0.013) |
3 | 396,660 6.839 (0.016) |
146,300 6.978 (0.019) |
542,960 6.874 (0.013) |
4 | 418,761 6.571 (0.016) |
120,457 6.827 (0.021) |
539,218 6.345 (0.013) |
5 | 403,014 6.499 (0.015) |
125,162 6.708 (0.019) |
528,176 6.551 (0.013) |
6 | 437,699 6.476 (0.014) |
113,687 6.625 (0.020) |
551,386 6.513 (0.012) |
7 | 439,602 6.349 (0.014) |
118,650 6.533 (0.019) |
558,252 6.395 (0.012) |
8 | 443,079 6.199 (0.014) |
119,691 6.524 (0.019) |
562,770 6.281 (0.011) |
9 | 434,143 6.091 (0.013) |
115,859 6.255 (0.019) |
550,002 6.132 (0.011) |
10 (least deprived) | 375,165 5.914 (0.014) |
101,913 5.819 (0.019) |
477,078 5.891 (0.012) |
Total | 4,097,227 6.646 (0.005) |
1,419,879 6.652 (0.006) |
5,517,106 6.647 (0.004) |
We modelled the effects of optimal water fluoridation and area level deprivation on the number of missing teeth using a negative binomial model with main and interaction effects, cluster robust SE and accounting for weighting. The number of observations for this outcome was 5,517,106. The conditional effect estimate for the effect of optimal water fluoridation was IRR 0.984 (95% CI 0.977 to 0.992), with predicted mean values for the number of missing teeth of 6.652 (SE 0.006) in the optimally fluoridated group and 6.646 (SE 0.005) in the sub/non-optimally fluoridated group. The effect estimates for the main and interaction terms along with their CIs are reported in Appendix 6.
Figure 14 illustrates the predicted mean number of missing teeth for the optimally fluoridated and sub/non-optimally fluoridated groups at each deprivation decile. Table 18 shows the discrete changes in the predicted mean number of missing teeth (the difference in the predicted values of the mean number of missing teeth in the fluoridated group and the mean number in the sub/non-optimally fluoridated group) at each deprivation decile.
Deprivation decile (IMD) | Difference in the predicted mean number of missing teeth (95% CI) (negative values favour water fluoridation) | p > |z| |
---|---|---|
1 | −0.476 (−0.517 to −0.435) | 0.000 |
2 | −0.174 (−0.217 to −0.130) | 0.000 |
3 | 0.139 (0.091 to 0.187) | 0.000 |
4 | 0.256 (0.206 to 0.306) | 0.000 |
5 | 0.209 (0.162 to 0.257) | 0.000 |
6 | 0.149 (0.101 to 0.196) | 0.000 |
7 | 0.184 (0.138 to 0.229) | 0.000 |
8 | 0.325 (0.279 to 0.369) | 0.000 |
9 | 0.164 (0.120 to 0.208) | 0.000 |
10 | −0.094 (−0.139 to −0.049) | 0.000 |
In IMD decile 1, the predicted mean number of missing teeth is 0.476 lower (95% CI 0.517 lower to 0.435 lower) in the optimally fluoridated group compared to the sub/non-optimally fluoridated group. In IMD decile 2, the predicted mean number of missing teeth is 0.174 lower (95% CI 0.217 lower to 0.130 lower) in the optimally fluoridated group compared to the sub/non-optimally fluoridated group (Figure 15 and Table 18). However, as with DMFT, the direction of effect is not consistent across the deciles. In IMD deciles 3–9, the predicted mean number of missing teeth is higher in the optimally fluoridated group than in the sub/non-optimally fluoridated group; for example, in IMD decile 8 the optimally fluoridated group is predicted to have 0.325 more missing teeth per person than the sub/non-optimally fluoridated group.
FIGURE 15.
Predicted mean number of missing teeth with 95% CIs by water fluoridation status and deprivation decile from negative binomial regression.
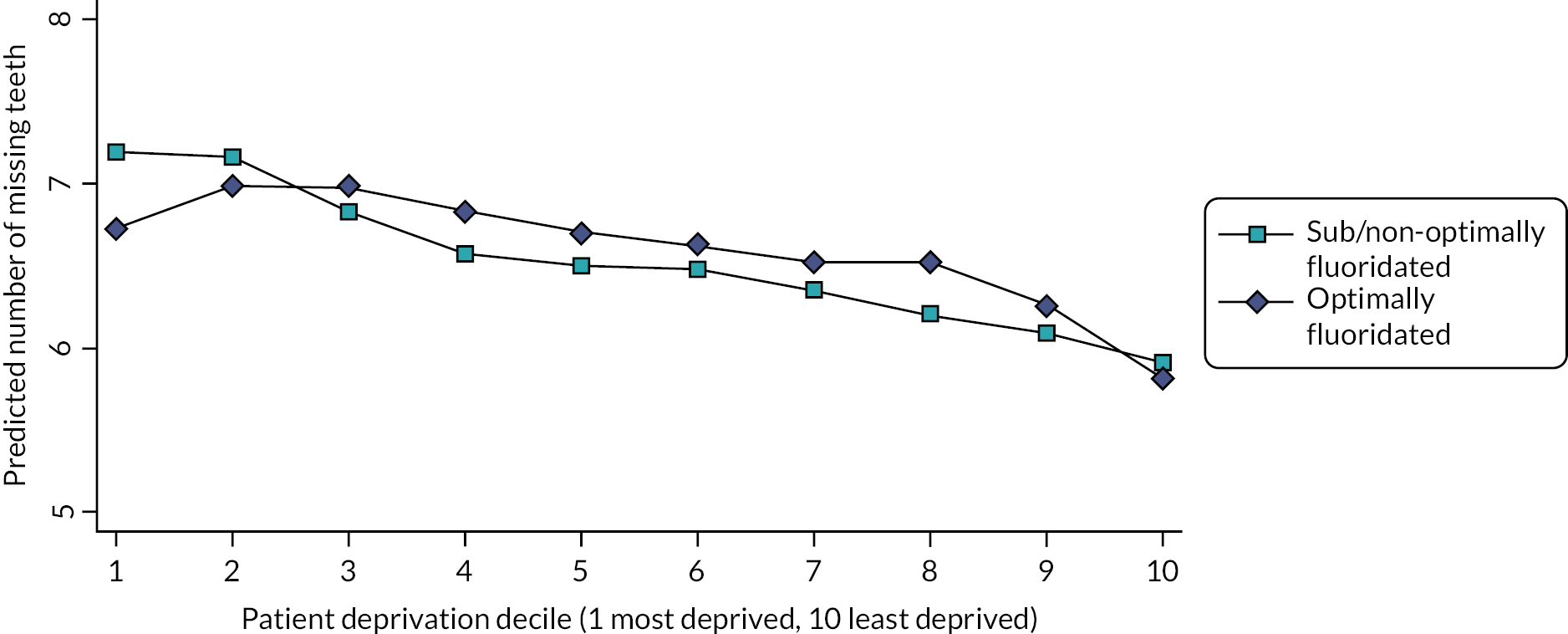
Ancillary analysis
Primary outcome: receipt of any invasive dental treatment(s) during the study period
An additional analysis considering the primary outcome expressed as the presence or absence of receipt of one or more invasive treatments during the study period was proposed by the Study Oversight Committee. The proportion of patients who received any invasive treatments for the matched study cohort overall was 0.845 (SE 0.0002). For the sub/non-optimally fluoridated group, the proportion was 0.846 (SE 0.0002) and for the optimally fluoridated group, it was 0.845 (SE 0.0003). A logistic regression was used to model the probability of receiving an invasive dental treatment according to water fluoridation status. The odds of receiving at least one invasive treatment for the optimally fluoridated group were 0.99 times the odds of receiving at least one invasive treatment for the sub/non-optimally fluoridated group (OR 0.993, 95% CI 0.988 to 0.999). The predicted values for the probability of receiving at least one invasive dental treatment was 0.845 (SE 0.0003) for the optimally fluoridated group and 0.846 (SE 0.0002) for the sub/non-optimally fluoridated group.
Primary outcome: invasive dental treatments taking into account the number of courses of treatment per patient
For the analysis of the primary outcome, we modelled the rate of invasive dental treatments per course of treatment to account for the fact that the number of courses of treatment varied from person to person, and the number of invasive treatments received is proportional to the number of courses of treatments completed. An offset term using the total number of courses of treatment was added to the regression model, so the exposure of the optimally fluoridated and sub/non-optimally fluoridated groups is proportional to the number of courses of treatment. Including an offset term in the model adjusts for the opportunity that an event has to occur.
The IRR for the effect of water fluoridation from this model was 1.013 (95% CI 1.011 to 1.016), indicating the rate of invasive dental treatments per course of treatment in the optimally fluoridated group was 1% higher than that of the sub/non-optimally fluoridated group. The predicted mean number of invasive treatments from this model was 5.940 (SE 0.004) for the sub/non-optimally fluoridated group and 6.020 (SE 0.005) for the optimally fluoridated group.
Further details on the ancillary analysis taking into account the number of courses of treatment received by each patient, including analysis by deprivation decile and the proportion of patients who received any invasive dental treatments, is available in Appendix 7, Tables 40 and 42 and Appendix 7, Figure 34.
Health economic results
Water fluoridation costs
Data on water fluoridation expenditure (provided by PHE) are summarised in Table 19 alongside the estimated population receiving artificially fluoridated water (≥ 0.7 mg F/l) and per person expenditure. The population receiving artificially fluoridated water was estimated by combining the water fluoridation sample data and maps used to assign patient to optimally fluoridated or sub/non-optimally fluoridated groups (see Water fluoridation), with published estimates and maps detailing the extent of natural and artificial water fluoridation in England. 21,151
Year | Population receiving artificially fluoridated water (≥ 0.7 mg F/l) | Capital expenditure | Operating expenditure | Total expenditure | Total expenditure in 2020 prices | Expenditure per person in 2020 prices |
---|---|---|---|---|---|---|
2010a | 4,148,557 | £1,395,729 | £2,674,849 | £4,070,577 | £5,048,465 | £1.22 |
2011a | 4,114,777 | £1,395,729 | £2,674,849 | £4,070,577 | £4,946,083 | £1.20 |
2012a | 4,915,098 | £1,395,729 | £2,674,849 | £4,070,577 | £4,867,772 | £0.99 |
2013 | 5,312,695 | £3,012,400 | £2,364,160 | £5,376,560 | £6,288,889 | £1.18 |
2014 | 5,627,931 | £314,800 | £2,436,282 | £2,751,082 | £3,167,406 | £0.56 |
2015 | 5,316,818 | £1,582,200 | £2,504,335 | £4,086,535 | £4,680,918 | £0.88 |
2016 | 3,168,016 | £1,261,800 | £2,821,102 | £4,082,902 | £4,589,696 | £1.45 |
2017 | 4,156,021 | £998,100 | £2,599,496 | £3,597,596 | £3,971,865 | £0.96 |
2018 | 4,901,454 | £1,977,600 | £2,995,309 | £4,972,909 | £5,382,635 | £1.09 |
2019 | 3,780,350 | £623,200 | £3,003,257 | £3,626,457 | £3,847,659 | £1.02 |
Capital expenditure includes replacement plants or repairs and upgrades to fluoride dosing equipment and facilities and is subject to annual fluctuations based on the required work. Operating expenditure included running costs such as workforce, transport and chemicals. Expenditure data could not be provided by PHE for the years 2010–2 as this is a period before PHE existed. For these years the mean expenditure was used, calculated over the period 2013–9. The estimated size of the population receiving artificial fluoridation at the required dose was used to calculate expenditure per person. The GDP deflator is used to express these costs in 2020 prices. Total expenditure between 2010 and 2019 was £46,791,388 in 2020 prices. The average size of the artificially optimally fluoridated population between 2010 and 2019 was 4,544,172 yielding a per person optimal fluoridation cost over 10 years of £10.30 in 2020 prices.
Dental resource use and costs
The sample consists of 6,370,280 patients who are split between 1,592,570 in the optimally fluoridated group and 4,777,710 in the sub/non-optimally fluoridated group. Table 20 summarises the total courses of treatment per patient from 2010 to 2020. Mean total courses of treatment were 0.22 lower in the optimally fluoridated group, 9.91 versus 10.13. This difference is driven by very high service uses and the percentiles in the distribution are the same until the 99th percentile.
Total courses of treatment | Mean courses of treatment per patient | SD | p10 | p25 | p50 | p75 | p90 | p95 | p99 | |
---|---|---|---|---|---|---|---|---|---|---|
Sub/non-optimally fluoridated | 48,818,541 | 10.13 | 6.31 | 3 | 5 | 9 | 14 | 18 | 21 | 29 |
Optimally fluoridated | 15,789,515 | 9.91 | 6.11 | 3 | 5 | 9 | 14 | 18 | 21 | 27 |
Total | 64,608,056 | 10.08 | 6.26 | 3 | 5 | 9 | 14 | 18 | 21 | 28 |
Table 21 shows how treatment bands are distributed between those in the optimally fluoridated and sub/non-optimally fluoridated groups. Optimally fluoridated patients had a greater percentage of band 1 treatments and slightly lower percentage of band 2 and band 3 treatments. Urgent treatments were also lower for optimally fluoridated patients.
Band 1 | Band 2 | Band 3 | Free | Urgent | Total | |
---|---|---|---|---|---|---|
Sub/non-optimally fluoridated | 23,993,922 | 14,791,403 | 3,675,986 | 485,554 | 5,440,868 | 48,387,733 |
49.59 | 30.57 | 7.60 | 1.00 | 11.24 | 100.00 | |
Optimally fluoridated | 8,311,682 | 4,735,564 | 1,164,471 | 167,454 | 1,404,267 | 15,783,437 |
52.66 | 30.00 | 7.38 | 1.06 | 8.90 | 100.00 | |
All | 32,305,604 | 19,526,967 | 4,840,457 | 653,008 | 6,845,134 | 64,171,170 |
50.34 | 30.43 | 7.54 | 1.02 | 10.67 | 100 |
Table 22 shows NHS patient changes per patient for the period 2010–20. This includes patients who qualified for NHS charge exemptions at some/all of their courses of treatments. Optimally fluoridated patients had lower patient charges, but the mean difference is relatively small at £7.64. From the 75th percentile the differences become greater, and at the 99th percentile the difference is £50.
Mean | SD | p10 | p25 | p50 | p75 | p90 | p95 | p99 | |
---|---|---|---|---|---|---|---|---|---|
Sub/non-optimally fluoridated | £376.64 | £336.25 | £45.50 | £125.18 | £292.57 | £531.23 | £827.75 | £1039.80 | £1507.21 |
Optimally fluoridated | £369.00 | £325.34 | £44.93 | £124.34 | £290.26 | £521.77 | £806.47 | £1009.46 | £1457.24 |
Total | £374.73 | £333.58 | £45.28 | £124.98 | £292.12 | £528.56 | £822.36 | £1032.35 | £1495.00 |
Dental practices are reimbursed for the care provided using UDAs and the financial value of a UDA is negotiated. There is considerable variation in the financial value of 1 UDA between contracts. Our analysis uses the mean cost per UDA for all practices in England between financial years 2015–6 and 2019–20, obtained from NHS BSA via a freedom of information (FOI) request (Table 23). Treatment bands have different UDA multipliers: Band 1 × 1 UDA, Band 2 × 3 UDA, Band 3 × 12 UDA and Urgent × 1.2 UDA.
Year | Mean cost per UDA |
---|---|
2010–1* | £27.50 |
2011–2* | £27.50 |
2012–3* | £27.50 |
2013–4>* | £27.50 |
2014–5* | £27.50 |
2015–6 | £27.50 |
2016–7 | £27.70 |
2017–8 | £28.20 |
2018–9 | £28.70 |
2019–20 | £29.80 |
2020–1* | £29.80 |
The average cost per UDA and treatment band multiplier are used to generate total UDA treatment costs shown in Table 24. Each course of treatment is assigned the UDA cost paid to the practice by the NHS and then totalled for each patient from 2010 to 2020. Patients in fluoridated areas had a lower UDA treatment cost at £755.75 versus £784.34, a difference of £28.59. As with patient charges, the difference increases for those with higher service use and reaches £191.63 at the 99th percentile.
Mean | SD | p10 | p25 | p50 | p75 | p90 | p95 | p99 | |
---|---|---|---|---|---|---|---|---|---|
Sub/non-optimally fluoridated | £784.34 | £663.72 | £166.24 | £321.10 | £600.81 | £1040.05 | £1622.93 | £2073.56 | £3187.53 |
Optimally fluoridated | £755.75 | £624.48 | £169.84 | £319.23 | £580.44 | £995.87 | £1547.66 | £1970.58 | £2995.90 |
Total | £776.94 | £654.26 | £167.10 | £320.55 | £597.34 | £1028.50 | £1604.43 | £2048.31 | £3141.45 |
For each course of treatment, the NHS pays the dental practice the UDA treatment costs but also recovers the patient charge amount, where these charges are applicable. Table 25 presents the NHS treatment cost, where patient charges have been deducted from UDA treatment costs to give the total cost to the NHS. Patients in optimally fluoridated areas had a lower NHS treatment cost at £385.75 versus £407.70, a difference of £21.95. This difference increases to £135.96 for the 99th percentiles.
Mean | SD | p10 | p25 | p50 | p75 | p90 | p95 | p99 | |
---|---|---|---|---|---|---|---|---|---|
Sub/non-optimally fluoridated | £407.70 | £426.82 | £69.43 | £138.85 | £271.53 | £517.43 | £902.53 | £1244.43 | £2104.55 |
Optimally fluoridated | £385.75 | £396.23 | £70.51 | £136.78 | £260.78 | £488.62 | £842.31 | £1159.75 | £1968.59 |
Total | £402.22 | £419.49 | £69.67 | £138.30 | £268.71 | £509.70 | £887.69 | £1223.74 | £2069.80 |
The difference in NHS treatment costs between optimally fluoridated and sub/non-optimally fluoridated patients is estimated using a GLM and the regression results are shown in Table 26. The marginal effects estimate reveals a saving in treatment costs for optimally fluoridated patients over the study period of £22.26 per person with a narrow 95% CI of £21.43 to £23.09. This represents a relative reduction in NHS treatment costs of 5.46% per person (95% CI −5.26% to −5.66%) in the optimally fluoridated group.
Total NHS treatment cost | Coefficient | 95% CI |
---|---|---|
Optimally fluoridated | −0.055* | −0.057 to −0.053 |
Constant | 6.011* | 6.009 to 6.012 |
Observations | 6,370,280 | |
Marginal effects | 95% CI | |
Optimally fluoridated | −22.26* | −23.09 to −21.43 |
Return on investment
The return on investing in water fluoridation is estimated using the cost provided by PHE and the estimated NHS cost saving from Table 20. As the cost saving is estimated on a sample, we present four alternative scenarios to estimate the proportion of the optimally fluoridated population these cost savings can be attributed.
-
Scenario 1: estimated cost savings are attributed to the average optimally fluoridated population size 2010–20. This scenario will overestimate the ROI as it assumes our sample and the fluoridated population have the same characteristics which we know is not the case. For example, our sample is aged 12 + and have received at least two courses of NHS dental treatment in the study period.
-
Scenario 2: estimated cost savings are attributed to an estimate of the proportion of patients who have received at least two courses of NHS dental treatment in the study period. The proportion is estimated from responses to the GP Patient Survey 2019. Respondents were asked for reasons for not having an appointment in the last 2 years and could answer: prefer private; stayed with dentist when changed from private; tried but not successful; cannot remember if successful or not; and didn’t think they could get one. The proportion giving these reasons for not having an appointment summed to 24.40%; hence we estimate the proportion who may have received at least two courses of NHS dental treatment within 10 years to be 75.60%.
-
Scenario 3: estimated cost savings are attributed to the proportion of patients who have seen an NHS dentist within the NICE maximum recommended recall period, as reported by NHS BSA. The NICE recommended recall period is within 12 months for those aged 12–18 years and within 24 months for 18 +. This will be the lower boundary of estimate of the true proportion because the time frames are shorter than the 10 years. This proportion is 50.30%.
-
Scenario 4: estimated cost savings are attributed to a proportion of patients lying equally between scenario 2 and 3. This scenario was suggested by members on this study steering group and represents what they believed to be closest to the actual figure to an estimate of the proportion of the 12 + population who would receive at least two courses of NHS dental treatment in 10 years. The proportion is 62.95%.
The ROI for each scenario is shown in Table 27.
Scenario | A | B | C | D | E | F |
---|---|---|---|---|---|---|
Population size | Total fluoridation costs | Estimated savings in total NHS treatment cost per person | Estimated savings in total NHS treatment cost [A*C] | Estimated total ROI [D–B] | Estimated percentage total ROI [E/B*100] | |
1 | 4,544,172 | £46,791,388 | £22.19 | £101,153,269 | £54,361,881 | 116% |
2 | 75.60% of A 3,435,394 | £46,791,388 | £22.19 | £76,471,870 | £29,680,482 | 63% |
3 | 50.30% of A 2,285,718 | £46,791,388 | £22.19 | £50,880,083 | £4,088,695 | 9% |
4 | 62.95% of A 2,860,556 | £46,791,388 | £22.19 | £63,675,983 | £16,884,595 | 36% |
Taking scenario 4 as an example, we see the investment in fluoridation costing £46.8 million and reducing NHS treatment cost by £63.7 million over the study period. This yields a ROI of £16.9 million, representing 36% of the total investment. In this calculation, the only benefit is achieved via reducing NHS treatment costs for the scenario population. No other plausible benefits are factored, which could include lower treatment costs outside of the population (i.e. those aged under 12) or benefits other than NHS treatment costs such as reduced school or work absences.
Cost effectiveness
The cost effectiveness of water fluoridation is estimated using the cost provided by PHE and the estimated number of invasive treatments avoided from Clinical results (the primary outcome).
As with the ROI, four scenarios are used and calculations for cost effectiveness are shown in Table 28.
Scenario | A | B | C | D | E | F | G |
---|---|---|---|---|---|---|---|
Population size | Total fluoridation costs | Estimated number of invasive treatments sub/non-optimally fluoridated | Estimated number of invasive treatments optimally fluoridated | Estimated number of invasive treatments avoided per patient [C–D] | Estimated number of invasive treatments avoided for population [A*E] | Cost per invasive treatments avoided [B/F] | |
1 | 4,544,172 | £46,791,388 | 5.616 | 5.443 | 0.173 | 786,142 | £59.52 |
2 | 75.60% of A 3,435,394 | £46,791,388 | 5.616 | 5.443 | 0.173 | 594,323 | £78.73 |
3 | 50.30% of A 2,285,718 | £46,791,388 | 5.616 | 5.443 | 0.173 | 395,429 | £118.33 |
4 | 62.95% of A 2,860,556 | £46,791,388 | 5.616 | 5.443 | 0.173 | 494,876 | £94.55 |
The incremental costs are £46.8 million as the fluoridation costs are zero in the sub/non-optimally fluoridated group. The incremental benefits vary depending on the scenario chosen, taking scenario 4 as an example, we see the number of invasive treatments decreasing by 494,876. This leads to a cost of £94.55 per invasive treatment avoided. ICER=£46.8m−£05.616−5.443=£94.55
This ICER of £94.55 cannot alone be used to determine if the water fluoridation scheme were cost-effective or not, when evaluated using the study period and study population. For that determination a threshold value would be needed, a monetary value below which the ICER would represent a cost-effective decision. It should be noted that the benefit of avoiding an invasive dental treatment cannot be solely measured by the cost to the NHS of that treatment, for example the patient has avoided the need for a treatment which may have been uncomfortable and permanently affected the integrity of a tooth.
Sensitivity analysis using fee-for-item costs
It could be argued that the English UDA system (with just three ‘bands’ of costs) could obscure cost differences as a result of lower treatment needs. Investigating the effect of using the Scottish fee-for-item costs was hypothesised as a sensitivity analysis, to provide a more discriminatory approach.
The intention here is not to estimate ‘equivalent’ Scottish NHS treatment costs and the values attached to each treatment item are not intended to accurately quantify resource use in absolute terms. It is included for comparison purposes of relative differences only.
The Scottish treatment item in the Scottish Dental Remuneration schedule 142 November 2019153 thought to best reflect the ‘middle’ level of complexity for each type of treatment recorded in the English FP17 clinical data set was chosen as the reference cost (Table 29). The reference costs are multiplied by the number of treatments for each patient recorded in the NHS BSA dental data set, to calculate the totals shown in Table 30.
Treatment | Cost |
---|---|
Examination | £15 |
Permanent fills and sealants | £20 |
Extractions | £10 |
Inlays | £120 |
Crowns | £170 |
Veneers | £105 |
Endodontic treatment | £80 |
Fluoride varnish | £6 |
Mean | SD | p10 | p25 | p50 | p75 | p90 | p95 | p99 | |
---|---|---|---|---|---|---|---|---|---|
Sub/non-optimally fluoridated | £292.98 | £260.70 | £60.00 | £120.00 | £220.00 | £380.00 | £611.00 | £800.00 | £1270.00 |
Optimally fluoridated | £283.16 | £248.78 | £60.00 | £120.00 | £215.00 | £365.00 | £585.00 | £760.00 | £1210.00 |
Total | £290.52 | £259.33 | £60.00 | £120.00 | £220.00 | £376.00 | £605.00 | £790.00 | £1255.00 |
Using a GLM and the regression results shown in Table 31, the difference in treatment cost is estimated as £9.89 lower per person in the optimally fluoridated group (95% CI −£9.39 to −£10.41). This is a relative reduction in treatment costs of 3.38% (95% CI 3.20% to 3.55%). Despite using a completely different philosophy to costing dental treatment, this estimate is remarkably similar to the relative reduction of 5.46% per person (95% CI −5.26% to −5.66%) we found in the main analysis (using average UDA values for England).
Total NHS treatment cost | Coefficient | 95% CI |
---|---|---|
Marginal effects | 95% CI | |
Optimally fluoridated | −0.034* | −0.036 to −0.032 |
Constant | 5.680* | 5.679 to 5.681 |
Observations | 6,370,280 | |
Optimally fluoridated | −9.89* | −10.41 to −9.39 |
This provides reassurance that we are unlikely to have missed a large cost differential as a result of the banded approach to costing used in England, as compared to the more detailed fee-for-item costings used in Scotland.
Sensitivity analysis on units of dental activity values
In the main health economic analysis, the mean UDA value for contracts in England, obtained from NHS BSA, is used to calculate treatment costs. In Table 32 we present descriptive statistics using the UDA target, contract value and resulting UDA value calculated using contract specific values from the study sample. In each case, the mean is first calculated for each patient over all their courses of treatment, then the mean for both groups is calculated over all patients in that group.
UDA target | Mean | SD | p10 | p25 | p50 | p75 | p90 | p95 | p99 |
---|---|---|---|---|---|---|---|---|---|
Total financial contract value | Mean | SD | p10 | p25 | p50 | p75 | p90 | p95 | p99 |
Single UDA value | Mean | SD | p10 | p25 | p50 | p75 | p90 | p95 | p99 |
Sub/non-optimally fluoridated | 22,461 | 15,867 | 8000 | 12,709 | 19,165 | 28,268 | 39,000 | 47,905 | 85,709 |
Optimally fluoridated | 23,297 | 15,094 | 8071 | 12,531 | 20,000 | 30,175 | 42,273 | 51,236 | 75,835 |
Sub/non-optimally fluoridated | £676,725 | £508,809 | £233,970 | £370,029 | £570,323 | £844,730 | £1,200,897 | £1,467,401 | £2,702,661 |
Optimally fluoridated | £662,940 | £477,426 | £223,935 | £352,178 | £551,132 | £853,473 | £1,182,106 | £1,562,259 | £2,595,334 |
Sub/non-optimally fluoridated | £1658.15 | £59,504.54 | £24.82 | £26.87 | £29.35 | £32.30 | £35.75 | £39.22 | £1530.21 |
Optimally fluoridated | £404.15 | £13,041.81 | £23.81 | £25.58 | £27.65 | £30.48 | £33.38 | £34.90 | £113.49 |
Patients in the sub/non-optimally fluoridated group are more likely to have attended practices whose contracts have a very high financial value and a low UDA target. The resulting ‘UDA values’ (total financial contract value divided by UDA target) can be very high. It is likely that such contracts have a proportion of their financial value/activity allocated to a type of care that is not commissioned using UDAs. Orthodontic care is commissioned using UOAs, and sedation services are commissioned using specific KPIs, for example, number of patients treated, or waiting times. Community Dental Services are sometimes commissioned and funded to provide treatment under general anaesthesia in secondary care using a PDS (primary care) contract, with a very high contract value but very few UDAs allocated. We did not have access to these alternative commissioning/monitoring metrics, or the proportion of financial contract value that they apply to; therefore, it is not possible to know how much of the total financial contract value is actually allocated to UDAs, and what proportion is allocated to non-UDA-based activities.
Extreme differences in the contract-specific ‘UDA values’ as shown in Table 32 likely result from the fact that sub/non-optimally fluoridated patients are sampled in larger numbers and from a wider geographical catchment, to facilitate the one-to-three matching approach. Using the contract-specific UDA values contained within the study sample results in very extreme cost differences which do not appear to be driven by similar differences in clinical activity, but rather the different contracts and mechanisms available to commission dental services. This is why we elected to use an average UDA value obtained from NHS BSA to apply costs to clinical activity.
Chapter 5 Patient and public involvement
Aim
The aim of the PPI in this research was to ensure the work produced would be relevant to and understandable by patients and the public as funders and consumers of research.
Objectives
-
To gain insights about what matters to patients and the public about their oral health when developing the original proposal for the bid.
-
To seek ongoing input and invite challenge into the conduct and governance of the study.
-
To gather views from patients and the public on what they would consider to be a meaningful difference in our primary outcome.
-
To develop dissemination materials that are engaging and clear.
Methods
Objective 1
In designing the research proposal, the views of 16 members of the public were sought using one-to-one conversations at a dental practice in a deprived location in central Salford. Volunteers were reimbursed for their time with a £10 gift voucher, funded by a bursary from the North West Research Design Service. This group were considered to be appropriate because they were drawn from the population of patients we were planning to gather data on, and they include a wide cross section of society with the potential to benefit from water fluoridation. With many having seen the dentist that day, they were also expected to be focused on the topic for the study application.
Objective 2
Embedded PPI members were recruited to both the OMG and the Study Steering Committee (SSC). The OMG met once every 2 months and the SSC met four times. The embedded PPI members were recruited through the NIHR ‘People in Research’ website. We budgeted for PPI involvement throughout the study, using the NIHR INVOLVE calculator. One staff member was assigned as the main contact person for both the PPI members of the team. This staff member was responsible for recruiting the PPI members, undertaking an initial training needs analysis, offering training options, introducing the PPI members to the study team, guiding, supporting and working with them throughout the study.
Objective 3
Three online workshops were held with a view to gathering views on what would be considered to be a meaningful reduction in the number of invasive NHS dental treatments received over 10 years, as a result of water fluoridation. Ten patient and public contributors were recruited through the ‘People in Research’ website, and eight were recruited from the existing University of Manchester Primary Care PPI group (PRIMER). The first 10 contributors to respond to the invitation to take part on the ‘People in Research’ website were included. The findings from the day were distributed to the interested participants via e-mail after the event. Further information on the content of and findings from the workshop are available in Chapter 3, Stakeholder views on a minimum ‘clinically’ relevant effect size.
Objective 4
All public-facing materials were codeveloped with the PPI member of the study OMG, and this chapter and the plain language summary of this report were written together. The full study report has also been reviewed by the PPI member. PPI members will be involved in developing further dissemination materials, and in sharing outputs through their wider networks and during our planned engagement workshop for policy-makers. We also plan to share the public-facing dissemination materials yet to be developed (the video animation and infographic) with groups from a range of backgrounds for comment and feedback during their development process.
Results
Objective 1
These conversations revealed that researching ways to improve dental health was seen as very important because it is something that affects everyone. The out-of-pocket costs to patients of dental treatment were seen as a major issue, as well as the wish to avoid anxiety-provoking treatments. As a result, more information on the impact of patient charges and dental anxiety was added to the ‘background’ section of the funding application. The majority of people wrongly assumed they already had fluoride in their drinking water and seemed shocked to find that while some areas were potentially benefiting from this intervention, their area wasn’t. Some stated if this study identified a reduction in the number of unpleasant dental treatments required, or costs for patients, they would be asking their local council why they don’t fund water fluoridation.
The patients felt that reducing the need for dental treatment was important, firstly because it is unpleasant and, secondly, because it is costly to patients because of patient charges. These views helped to solidify our plan to use ‘invasive dental treatments received’ as the primary outcome measure, rather than the usual epidemiological index for dental studies, ‘number of decayed, missing and filled teeth’ (DMFT). One person suggested that a good way to share the results with the public would be to create a video which could be shared through social media. This was subsequently added to the dissemination package arising from the public feedback.
Objective 2
We had a very good response to the advertisement for embedded PPI contributors on the ‘People in Research’ website. The PPI member of the OMG had experience on committees and belongs to a number of networks, but aside from being a dental patient herself she had little existing knowledge about dental health. When applying for the role she was interested in the potential of dental research to help give a person a better QoL, free from dental pain, more confidence and a more positive self-image. She also hoped to increase her understanding about the potential health impact of water fluoridation. We developed a bespoke package of training, made up of a slide deck and key documents related to oral health. These helped to increase her confidence in contributing to this topic area and helped her to achieve her aim of increasing her understanding about the potential health impact of water fluoridation. This PPI member prepared a plain English summary for us to add to the current version of the protocol and helped to refine the text for the study website. She also assisted with developing the slides for the meaningful difference workshops. Through her involvement with this project, she has become more knowledgeable about dental issues and has also contributed to other funding applications within the dental school.
A second PPI member was recruited to the SSC. His training needs analysis revealed that he was a very experienced PPI contributor and was also a member of the University of Manchester Primary Care PPI group (PRIMER). He did not feel any particular training would be needed. His attendance at the steering committee meetings resulted in changes to the steering committee terms of reference and the study protocol.
Objective 3
A total of 18 public contributors attended the three workshops. The findings showed for most patients and the public, a minimum of one invasive dental treatment per person, over 10 years, would need to be avoided as a result of receiving water fluoridation for the effect to be viewed as meaningful (a 16% relative reduction). Interestingly, in contrast, dentists felt even very small reductions of less than half a treatment per person over 10 years (5% relative reductions) were meaningful. This finding was used to contextualise the results of this study and contributors reported they valued receiving a summary of the findings from the day and would like to continue to receive information about the study and our results. A negative incident in one of the workshops was that one contributor (while not ‘muted’) on the online call made a disparaging remark about another contributor. This was very unfortunate, and as it was not possible to identify which person had made the remark, it was not possible to remove them.
Objective 4
The public-facing study website, the plain English summary of the study protocol, this chapter and the full report have been codeveloped with or reviewed by the PPI member of the OMG. This has increased the readability of the documents and has also had additional benefits such as allowing us to see the particular elements of the research which are unique, surprising and most interesting to members of the public. Through the PPI member, the staff members of the research team have also learnt about patient engagement networks we were not aware of previously, that could be further routes for dissemination, such as the ‘use MY data’ and ‘Understanding Patient Data’ networks.
Discussion and conclusions
The PPI component has strongly influenced the design and conduct of the study from the outset. The PPI members of the study team have been important contributors to the work, have brought fresh perspectives to decisions and have functioned as ‘boundary spanners’, alerting us to helpful new developments, conventions and networks in other disciplines. Involving patients and the public in considering what would be a meaningful difference in our primary outcome was challenging because the concept is not straightforward and requires somewhat of a thought experiment. However, we feel it was very worthwhile and determining the threshold for ‘success’ in a study is a very important aspect that is not often considered as an area for PPI input.
Reflections/critical perspective
The embedded PPI element of the study enriched the study more than the staff members anticipated, and both the PPI members and staff enjoyed the experience and have grown and developed as a result of this work. We feel there were several elements that contributed to the success of this element of the research. Initially, when having conversations with potential PPI members who had expressed an interest in joining the team, it was useful to consider what qualities and attributes we were looking for. We valued existing networks and experience of working on committees because we wanted the PPI members to be able to feel confident to take part in meetings and assist with dissemination through their networks.
From the perspective of a PPI member, having a nominated professional contact to act as a ‘buddy’ was essential. The presence of a familiar person who could provide simple explanations of complex or technical information, and when needed, undertake debriefing sessions after meetings, was particularly appreciated and helped to make the PPI member feel more comfortable. Having a chance to meet with the full research team face to face (before the COVID pandemic necessitated a move to online meetings) also helped to increase interaction. It was important the professional members of the team were friendly and welcoming and treated the PPI member as an equal. The PPI member felt empowered to ask questions as they would always receive a serious response.
The one-off PPI activities were intended to gather a range of views from people with different perspectives and including those with perhaps less experience as contributors. Having adequate funding to support the activities was crucial and we feel this is partly why we got a good response to all requests. As the incident involving an inappropriate remark during one of the workshops illustrates, this type of engagement with no advance screening of participants is more unpredictable. A lesson learnt is to ensure participants receive ground rules before any events and the expectation that everyone involved should be treated respectfully is reiterated at the start. Additionally, in future we would plan to undertake more evaluation of the PPI activities as they are happening. A potential tool that could be useful is the Public and Patient Engagement Evaluation Tool (Version 2) developed by McMaster University. 162
Chapter 6 Discussion
Introduction
This study was undertaken to better understand the impact of water fluoridation on the dental health of adults. Water fluoridation studies, when considering benefit, assume any benefit seen in childhood continues through life. However, there are very few studies reporting the impact of water fluoridation on adults due to the complexities of data collection in this group versus the relatively simpler data collection from children attending school.
The recent UK context of low caries prevalence, social mobility, variability in water fluoridation dosing performance103 and increased additional sources of fluoride27 has not been extensively studied.
Key results
Primary objective
The findings of this study indicate the rate of invasive dental treatments in the optimally fluoridated group was 3% lower than that of the sub/non-optimally fluoridated group (IRR 0.969, 95% CI 0.967 to 0.971).
The predicted count of the number of invasive treatments from this model was 5.616 (SE 0.004) for the sub/non-optimally fluoridated group and 5.443 (SE 0.005) for the optimally fluoridated group. There was a predicted mean difference of 0.173 fewer invasive dental treatments per person (95% CI 0.185 fewer to 0.161 fewer) in the optimally fluoridated group compared to the sub/non-optimally fluoridated group.
Most stakeholders we engaged with (50/54) did not consider this magnitude of reduction in the number of invasive dental treatments received per person to be meaningful.
Secondary objectives
Oral health
We found the mean number of teeth with any experience of decay (DMFT) in the optimally fluoridated group was 2% lower than in the sub/non-optimally fluoridated group (IRR 0.984, 95% CI 0.983 to 0.985). The predicted mean count of DMFT in the negative binomial model was 13.361 (SE 0.006) per person in the sub/non-optimally fluoridated group and 13.149 (SE 0.007) per person in the optimally fluoridated group. There was a predicted mean difference of 0.212 fewer DMFT (95% CI 0.229 fewer to 0.194 fewer) in the optimally fluoridated group compared to the sub/non-optimally fluoridated group.
We did not specifically engage with stakeholders regarding what size of reduction in DMFT would be considered meaningful. However, as with the primary outcome, this size of effect has previously been classified as exceedingly small. 161
We found no difference in the mean number of missing teeth between the optimally fluoridated group and the sub/non-optimally fluoridated group (IRR 1.001, 95% CI 0.999 to 1.003). The predicted mean number of missing teeth in the sub/non-optimally fluoridated group was 6.646 (SE 0.005) missing teeth per person, compared to 6.652 (SE 0.006) missing teeth per person in the optimally fluoridated group. There was a predicted mean difference of 0.006 more missing teeth (95% CI 0.008 fewer to 0.021 more) in the optimally fluoridated group compared to the sub/non-optimally fluoridated group.
Effect of water fluoridation on social inequalities
There was evidence of a clear and consistent social inequality gradient in the number of NHS invasive dental treatments received, with patients in the most deprived decile of matched cohort receiving on average 1.285 more NHS invasive dental treatments per person over 10 years than those in the least deprived decile. The number of treatments received reduced consistently, from the most to the least deprived deciles.
In all deciles, the predicted number of invasive treatments was lower in the optimally fluoridated group than in the sub/non-optimally fluoridated, but the size of the reductions was very small. The largest predicted reduction of 0.337 invasive dental treatments per person (95% CI 0.371 fewer to 0.302 fewer) was in the most deprived decile (IMD 1), a relative reduction of 5.3%. This size of reduction would not have been considered meaningful by most stakeholders we engaged with, though the dentists we consulted considered differences as small as 0.3 fewer invasive dental treatments to be meaningful, a relative reduction of 5%.
The relationship between deprivation and the total number of teeth affected by decay (DMFT) was unclear and did not demonstrate the expected social inequalities gradient. Therefore, we are unable to conclude whether optimal water fluoridation reduced social inequalities in the total number of teeth affected by decay (DMFT). Small differences were evident in each decile, but the direction of effect was not consistent.
There was evidence of a clear and consistent social inequalities gradient in the expected direction in the number of missing teeth per person. Patients in the most deprived decile of the full-matched cohort had 1.19 more missing teeth per person than those in the least deprived decile and the number of missing teeth reduced consistently, from the most to the least deprived deciles. Small differences in the number of missing teeth per person in the optimally fluoridated and sub/non-optimally fluoridated groups were evident in each decile, but the direction of effect was not consistent.
Overall, we could find no compelling evidence to demonstrate that water fluoridation reduces socioeconomic disparities in oral health in the adult population.
Health economics
The cost-effectiveness analysis estimates the investment required (in the cost of water fluoridation) to produce a particular unit of health gain (avoiding one invasive dental treatment). This analysis does not include any reductions in the costs of NHS dentistry because those are considered in the ROI analysis. When evaluating whether water fluoridation is ‘cost-effective’ at preventing invasive dental treatments, the cost per invasive dental treatment avoided needs to be weighed against the patient experience and health impact of having an invasive dental treatment, rather than any potential monetary savings. At present, there is no cost-effectiveness threshold for the avoidance of invasive dental treatments.
The estimated cost of optimal water fluoridation to avoid one invasive dental treatment ranged from £59.52 to £118.33, depending on the proportion of the population estimated to use NHS dental services at least twice within 10 years (from 100% to 50.3% respectively). Using the scenario thought to be most likely by our steering committee (that 62.9% of the population aged 12 years and over will use NHS dental services at least twice in 10 years), we estimate the cost per invasive dental treatment avoided (the ICER) was £94.55 in 2020 prices.
In contrast, the ROI analysis calculates the net public sector return from a purely monetary perspective, balancing the costs of water fluoridation against any reductions in total NHS dental treatment costs. It is important to remember that any estimated savings in NHS treatment costs may not be realised if the dentist time saved is used to treat other NHS patients, as opposed to leading to a reduction in dentist time spent on NHS dental care. While this might help improve access to NHS dental care and/or reduce waiting times to receive care, those outcomes would not reduce the overall cost of NHS dental care.
We estimate that over the study period, total NHS treatment costs per patient were £22.26 lower (95% CI £21.43 to £23.09 lower) in patients receiving optimally fluoridated water (a relative reduction in total NHS dental treatment costs per person of 5.5%). The total cost of optimal water fluoridation over the study period was estimated to be £10.30 per person (2020 prices). When these per-patient costs were attributed to a population, the public sector ROI varied between 9% and 116% depending on the size of the population estimated to utilise NHS dental services (from 50.3% to 100% of the population respectively). All scenarios represented a positive return. Using the scenario thought to be most likely by our SSC, that 62.9% of the population aged over 12 would use NHS dental services at least twice in 10 years, the predicted ROI for optimal water fluoridation in England over our period of observation (November 2010 to October 2020) was estimated to be £16,884,595 (a 36% ROI).
National Health Service dental treatment costs were lower in the optimally fluoridated group because of slightly fewer courses of treatment, a greater proportion of band 1 treatments and a slightly lower proportion of band 2 and band 3 treatments. The proportion of urgent courses of treatments was also lower (11.24% in the sub/non-optimally fluoridated group and 8.90% in the optimally fluoridated group). The differences between NHS treatment costs were greater for patients who were high users of services at the top of the distribution, beyond the 95th percentile. This creates a skewed distribution which is typical for costs data. This distribution is also likely to explain why a small difference in the mean number of courses of treatments received per person over 10 years (9.91 in the optimally fluoridated group vs. 10.13 in the sub/non-optimally fluoridated group) led to a greater difference in NHS treatment costs than 0.22 fewer courses of treatments per person would imply.
Total NHS dental changes paid by patients were lower in the optimally fluoridated group, but the difference was relatively small, at £7.64 per patient. This includes patients who qualified for NHS charge exemptions at some or all of their courses of treatments.
How the results sit with the wider literature
Our findings of a very small effect of optimally fluoridated water align with those of the recently published landmark UK prospective cohort study in children (CATFISH). 78 This high-quality study was designed to meet the recommendations of the MRC in terms of study design, and was made possible because of the replacement of water fluoridation dosing equipment in Cumbria, after several years of inactivity. 163 Two cohorts of children were followed, one from birth to 5 years and one from 5 to 11 years, which allowed the effects of fluoride before and after tooth eruption to be investigated. In 5-year-old children (n = 1444), after adjusting for deprivation, age and sex, the prevalence of any decay was 17.4% in those who had received fluoridated water and 21.4% in those who received non-fluoridated water, an absolute difference of 4% (OR 0.74, 95% CI 0.56 to 0.98). For the 11-year-old children (n = 1192), while there was a slightly lower prevalence of decay in the fluoridated group (19.1%) compared to the non-fluoridated group (21.9%), the 95% CI for the OR included the possibility of no effect (OR 0.80, 95% CI 0.58 to 1.09). There was no evidence of water fluoridation reducing the effect of social deprivation in either the prevalence of decay or the number of teeth affected by decay (DMFT).
Despite a small absolute reduction in caries prevalence, like the present study, the economic evaluation for the CATFISH study found that in the 5-year-old children (exposed to water fluoridation from birth) there was a high probability of water fluoridation being cost-effective at a willingness-to-pay threshold of £20,000 per QALY. 164 The study authors concluded that the prevalence of decay and therefore the absolute size of the effect of water fluoridation was much smaller in this contemporary UK population than previously reported, and the clinical relevance of such a difference would need to be considered by policy-makers and the public. For comparison, the 2015 Cochrane systematic review and meta-analysis (which mostly contained studies conducted before 1975) estimated an absolute reduction in the prevalence of decay in deciduous teeth of 15% (95% CI 11% to 19%). For permanent teeth, the Cochrane systematic review estimated that DMFT was reduced by 1.16 teeth per person (95% CI 0.72 fewer to 1.61 fewer). 47 The Cochrane review did not include any studies containing adults and the length of follow-up was between 2 and 3 years, which are important differences to bear in mind.
Similar findings of small clinical differences in absolute terms have been identified in recent studies in Australia, the USA and Sweden. 62,66,70 Contemporary studies in adults that have found larger absolute differences in oral health as a result of water fluoridation have been carried out in contexts that are not transferrable to the UK general population due to higher levels of dental disease and very different dental care provision, for example, studies conducted in Brazil59,61,64,67–69,73,74 and those with specific population subgroups such as young offenders or Army recruits. 58,76 A recent study of Australian adults found no evidence of any benefit of water fluoridation in adults over the age of 45, and proposed that this finding could be explained by a ‘ceiling effect’ in measures of caries experience. 66 A ceiling effect is where after a certain point increased levels of disease cannot be captured by the measure. For example, with DMFT, new decay cannot be captured on teeth that have previously been decayed, are missing, or have been filled. We did not find such a ceiling effect in our data and DMFT in both our unmatched and matched cohorts increased at each age group beyond age 45.
Strengths and limitations
Strengths
There are several strengths to this research study. We published an a priori and peer-reviewed protocol and the study was designed to address some of the evidence gaps and key recommendations for water fluoridation research made by the MRC in 2002. 163 In particular:
-
the effect of water fluoridation on the dental health of adults
-
the effects of fluoridation on health and well-being beyond the usual measures of decayed, missing or filled teeth
-
the economic impacts of water fluoridation
-
how the effects of water fluoridation vary with social class.
There have been few studies on the effects of water fluoridation on the dental health of adults, because of the logistical difficulties in recruiting large number of representative adults into an epidemiological study. Routinely collected NHS dental claims data offered an innovative solution but have been a relatively underused resource for research, particularly in comparison to other NHS activity data sets, for example, Hospital Episode Statistics (HES), or the general medical practice-based Clinical Practice Research Datalink database. 165 In working with NHS BSA we have increased our knowledge and understanding of the potential for these data sets to be used for research. These reflections inform our recommendations for the further development of these data sets, and the utility of NHS dental data sets across the four UK home countries has been explored in more detail in a separate paper. 165 The benefits of using these routinely collected data are that very large samples are available and the data provide an ability to follow patients over long time periods. Once the necessary permissions are secured, the data are available at minimal costs and within a relatively short time frame. Important demographic variables are routinely collected, including age, sex, ethnicity and postcode. 165 Postcode is crucial as it enables the LSOA in which each patient lives and its level of deprivation to be assigned via the IMD index. This feature, and the large sample size, allowed us to address the MRC recommendation to investigate how the effect of water fluoridation deprivation varied with social class (patient deprivation decile and NHS exemption status used as proxy measures for social class).
Having access to the LSOA of each recorded patient address over the period of observation also allowed us to assign an individual level of exposure to water fluoridation when combined with address history recorded in the NHS BSA database. This element strengthens the natural experiment design of the study, which retains the unplanned, and essentially random variation in receipt of the intervention at the individual level. This feature helps to reduce the effect of area-based confounding related to factors which influenced decisions on which areas to fluoridate in the 1960s and 70s. While not perfect, the method of exposure assignment is much more robust than has been utilised in many previous studies on the effects of water fluoridation. We were also able to create an unplanned additional resource for future research, through the publication of LSOA level water fluoride concentrations. 128 The geodemographic variables in the NHS dental services data set also enabled sophisticated matching of exposed and non-exposed (comparator) samples. The propensity score matching procedure was carried out according to a pre-planned set of covariates which had been identified through the use of a DAG, in order to make the theoretical assumptions transparent and avoid the introduction of collider bias. An additional benefit of propensity score matching is that the method of addressing confounding is carried out before the final effect estimation (unlike with regression-based methods).
Patient, public and stakeholder involvement and engagement has been an important part of this project throughout and is a significant strength of this work. With regard to the MRC recommendation to consider the effects of water fluoridation on health and well-being beyond DMFT, we engaged with patients and the public to identify what was important to them about their oral health. They stated that avoiding invasive dental treatments (the ‘drill’ and the ‘injection’) was very important, as was keeping their natural teeth, and paying less in NHS dental charges. These conversations were not intended to provide a representative or generalisable view as they were involvement and engagement activities, but they did help to inform our decision to use number of invasive dental treatments as our primary outcome. We also hypothesised that this choice of primary outcome would have greater utility to be able to capture the effects of repeated treatments for decay on the same tooth (the restorative cycle), than the traditional measure of DMFT. The three online stakeholder involvement and engagement workshops we held also helped us to understand the range of effect sizes that patients, the public and dental experts would consider to represent a meaningful reduction in invasive dental treatments as a result of water fluoridation. The range of views we encountered was broad, and this was reflected even within the research team. It is possible that the views of readers and policy-makers may differ from the majority view that we found in our workshops.
Using a more formal approach, a minimum set of patient-centred outcomes for adult oral health has recently been developed in a collaboration between the World Dental Federation (FDI) and the International Consortium for Health Outcomes Measurement (ICHOM). 166 The authors report that the aim is for the outcome set to be used to measure the value of healthcare outcomes, in clinical, research, evaluation and community settings. 166 Although ICHOM is designed to capture the outcomes of healthcare services, rather than preventive interventions, the outcomes reflect what patients and the public said was important to them during those early conversation in Salford, including the ability to eat and smile. Interestingly, the initial iteration of potential outcomes for ICHOM did not include the financial impact of dental care on individuals, but this outcome was added as a result of feedback from an advisory group of eight patients, and aligns with what we heard during our engagement work. 166 Avoidance of irreversible treatment is an obvious goal for preventive interventions, but this was not included due to the nature of the ICHOM project, which is specifically to develop measures which represent end results of health care. 166 The core outcome measures in effectiveness trials (COMET) initiative collates core outcome sets specifically for intervention research purposes. To date, there remains no formally agreed core outcome set for the primary prevention of dental caries on the COMET database. 167 Developing one using the COMET methodology, which includes a systematic review of previous outcomes and Delphi consensus methods, is a necessary area of future research for caries prevention studies. 168
The health economic analysis provides contemporary information on the economic impacts of water fluoridation, from both the perspective of the public sector and NHS dental patients. We were able to source capital and revenue costs of water fluoridation centrally from PHE based on their responsibility for funding capital investment and recovering operating costs from local authorities, meaning they were accurate as they are what has been charged to the public sector. We also have high confidence in the costs to the NHS of dental treatment, as our data are based on claims that have already been processed and paid and are subject to independent scrutiny with regard to the detection of fraud. A sensitivity analysis based on the Scottish fee-per-item system of costings was also undertaken to provide a more discriminatory approach to costs than the simplified ‘banded’ cost levels used in the English system. Reassuringly, the relative differences in costs using these very different philosophies and approaches to costings were remarkably similar. We found a reduction in costs to the NHS of 5.46% per person (95% CI −5.66% to −5.26%) in the main analysis (using average UDA values for England), compared to a reduction of 3.38% (95% CI -3.55% to -3.20%) in the fee-per-item sensitivity analysis. Finally, we benefitted from independent oversight and scrutiny through the study steering group and were able to utilise their judgement and expertise when considering the most likely ROI and cost-effectiveness scenarios.
Limitations
There are several limitations to this work. It must be remembered that the study sample is drawn from the population of users of NHS dentistry living in England between November 2010 and October 2020. Population groups not included are those who prefer private dentistry, cannot get access to NHS dentistry, or have not felt the need for any dental care within this time frame. For NHS BSA to be able to link treatment claims together for a single, unique patient, a 1 : 1 match between NHS BSA Index ID and NHS number was required. The majority of treatment claims (FP17s) do not include NHS number when they are submitted, but NHS BSA are able to trace NHS numbers for 70% of submitted claims through the NHS demographic batching service. Published work using NHS HES data has demonstrated that missing data (and missed matches in NHS data linkage studies) more frequently affect ethnic minority patients, those living in deprived areas, foreign nationals and those with ‘no fixed abode’. 169,170 Missing data from specific population subgroups have the potential to bias estimates of effect, for example by eliminating higher need groups which could underestimate the effectiveness an intervention. More complete data on NHS numbers and ethnicity in the NHS BSA system would be welcomed to increase the ability to undertake data linkage. It must also be remembered that children aged below 12 years were not included in our cost-effectiveness estimates here and we were also unable to include societal benefits such as reduced time off work/school with toothache, attending dentist for treatment, reduction in pain and suffering from toothache, etc. Although these impacts fall outside of our public sector perspective, they do generate value for society.
A further limitation in the analysis of cost effectiveness is, as we were not able to secure an indicator of which areas were fluoridated as part of a public health programme, and which received natural fluoridation, we included all individuals who had a personal water fluoridation exposure > 0.7 mg F/l in the exposed group, regardless of source. While the proportion of the population receiving fluoridation through natural sources is relatively small (~5%),21 this means any benefits of natural fluoridation have been included in our analysis despite there being no costs associated with this exposure. This will have the tendency to overestimate the cost effectiveness of water fluoridation as a public health intervention. Additionally, we were not able to source capital costs earlier than 2013, which means the costs of setting up a new water fluoridation treatment programme have not been included in our cost-effectiveness estimates, as none were commissioned within that time period. These costs are potentially significant and include the costs of public consultations, feasibility studies, detailed engineering proposals and costs of new buildings, dosing equipment and service roads. Therefore, our approach considers the current impact of existing schemes. Individual local authorities can use our findings together with the setup costs they are presented with for any potential future water fluoridation investments. We provide information on the likely impact should a scheme be introduced and not the cost of its introduction. This is a pragmatic approach because the costs of investment will vary widely between settings because of the different engineering configurations required (water flows, road infrastructure and the arrangement of water treatment facilities). 149
The primary outcome measure of ‘invasive dental treatments received’ was chosen because it was stressed as being important to patients and the public during our engagement work. However, it has some limitations which are important to consider. Firstly, there is no financial incentive for dentists to accurately record the number of treatment items provided on the FP17 form (beyond one treatment in each ‘band’ of care). On the other hand, the number and types of treatment provided are automatically recorded from the electronic dental clinical notes system. As the regulator of dentists in the UK, the General Dental Council states that dentists must ‘make and keep contemporaneous, complete and accurate patient records’ to comply with the expected standards of the profession. While there may be some inaccuracies in the reporting of the number and types of treatments, we do not feel that this is likely to be a significant problem. Secondly, receipt of dental treatment is influenced by a range of factors unrelated to water fluoridation and dental caries, including the clinical decision-making of dentists, the presence of non-caries-related dental problems such as periodontal disease and trauma, and the patient’s ability to pay privately for some treatments (dental care provided privately following an NHS examination will not be captured). In the same vein, for the DMFT and ‘missing’ teeth outcome, we do not have information on the reasons why teeth were extracted, and some will have been extracted as a result of periodontal disease rather than caries. We have controlled for sociodemographic factors that may be related to non-caries-related treatments including periodontal disease and ability to pay privately (matching on age, sex, deprivation, ethnicity and NHS dental charge exemption status); therefore, we see no reason why non-caries-related treatments should vary between groups. Any relative differences in caries-related treatments would still be expected to have been apparent ‘over and above’ the non-caries-related treatments, but it is important to remember this measure is not a measure of ‘caries-related treatment’. The inclusion of a diagnosis code within the routinely collected NHS dental data sets would be a welcome step to provide greater sensitivity in future research studies and would aid epidemiological surveillance.
The DMFT outcome did not demonstrate a clear social inequalities gradient, which inevitably raises questions regarding the validity of these data. However, recent secondary analyses of UK and Australian epidemiological surveys have indicated that measures of total caries experience (DMFT/S) may not be sensitive to socioeconomic inequalities in adults, other than in the youngest adult age groups, and that missing teeth are more sensitive. 6,66,73 Indeed, the missing teeth outcome did demonstrate a clear social inequalities gradient in the present study. It should be remembered that DMFT in this study is not the same measure as the commonly used epidemiological index. In the NHS BSA data set, the number of DMFT variables are automatically extracted from the dental charting completed at the time of examination. 171,172 NHS BSA guidance for dentists states that teeth should only be recorded as missing if the dentist is ‘confident that the tooth was extracted’. This is in contrast to recent UK epidemiological surveys of adult oral health where any tooth not present in the mouth is charted as missing (i.e. including unerupted or congenitally missing teeth). 171–173 The accuracy of the DMFT data in this clinical context depends on the thoroughness of the dentist’s examination and the accuracy of their record keeping (as opposed to training and calibration as is used in epidemiological surveys). Previous audits have shown that the accuracy of NHS dental charts is variable when compared with a reference standard. 174
It cannot be ruled out that the absence of a clear effect of water fluoridation on DMFT or missing teeth may in part be due to the potentially lower sensitivity of clinical chartings carried out by busy GDPs, as opposed to trained and calibrated examiners. On the other hand, dental practitioners are able to dry the teeth, take radiographs and have access to more powerful lighting than is used in epidemiological studies, where examinations are often carried out in schools; thus, it could be argued that the sensitivity of the clinical examinations may be higher. Missing teeth are also a fairly obvious feature which do not require a thorough dental examination to detect. It seems unlikely that if the effect of water fluoridation had remained the same as previously reported (26% relative reduction in DMFT in the Cochrane systematic review),47 that it would go undetected solely as a result of a lack of sensitivity in these measures. It is also reassuring that the proportion of adults estimated to be edentulous in our full unmatched cohort (1.3%) is very similar to the proportion recorded in the 2017 National Dental Epidemiology Programme survey of adults attending general dental practices which used calibrated examiners (1.1%). 175 However, a study to investigate the validity of the DMFT data recorded by GDPs and transferred to NHS BSA as compared to trained and calibrated examiners would help to unpick this further.
For the assignment of individual water fluoridation exposure, we linked water fluoride concentrations between 2010 and 2020 to each patient’s residential history as recorded at the time of each course of treatment. Errors of misclassification could have occurred during the mapping of water fluoridation, due to the conversion from WSZs to LSOAs, and as a result of patients moving address in between courses of treatments. NHS BSA dental data are retained for a maximum period of 10 years; therefore, we were not able to assign water fluoridation exposure to individuals earlier than this date. Thus, the effects observed cannot be said to result from only 10 years of exposure, rather a ‘minimum of’ 10 years exposure. Additionally, measurement of exposure was concurrent with measurement of outcomes in this study, but reverse causality is not a concern as we see no mechanism whereby the number of treatments received could influence a patient’s fluoridation status. The variability in achieved water fluoride concentrations over time is such that it is important to have this information when assigning exposure to water fluoridation. However, the method we needed to use to obtain this information [sourcing water quality sampling data from each water company and converting the information to useable (LSOA) geographies] was very time-consuming and not one we would recommend to other research teams.
As with any observational study, the likely effect of unmeasured and residual confounding must be considered. Unmeasured confounding results from confounding that cannot be taken into account during the design or analysis stage of the study as the variables cannot be, or were not, measured. We did not have any data on the behaviours of participants, for example, tap water consumption, smoking, sugar consumption, fluoride use and oral hygiene. However, as exposure to optimally fluoridated water does not rely on individuals seeking out a particular service or intervention (as with many other health promotion interventions), the potential for confounding due to behavioural factors is significantly reduced. Behavioural characteristics of individuals are unlikely to have also influenced their group assignment. We propose that patient behaviours could only plausibly be linked to water fluoridation group assignment through the effect of sociodemographic characteristics clustering by geography and influencing decisions on which areas to fluoridate in the 1960s (which we accounted for by matching on age, rurality, ethnicity, deprivation and NHS charge exemption status). We also propose that data on sociodemographic characteristics are more likely to be accurate than self-reported behaviours.
An example of a potential source of unmeasured confounding is if there were historic ‘cultural’ variations in dietary or oral hygiene behaviours (not explained by age, rurality, ethnicity, deprivation and NHS charge exemption status) that influenced decisions on which areas to fluoridate in the 1960s, and which still persist today and influence oral health outcomes. We would not be able to remove the confounding effect of ‘culture’ because it is unmeasured. Concerns about unmeasured confounding are reduced in this study because of the natural experiment study design, which (through individual-level assignment of receipt of optimal water fluoridation) reduces the correlation between historic decisions to implement water fluoridation and group assignment (due to breaks in water fluoridation supply, suboptimal dosing, or individuals moving into and out of fluoridated areas). While still an observational study, there is some unplanned variation in receipt of the intervention, which takes the group assignment of individuals in this study closer to being ‘as-if randomised’, strengthening causal inference and reducing the likely impact of unmeasured confounding. 101,102
Residual confounding is when the variables included do not fully remove the confounding effect because they are poor proxies or are poorly measured or completed. The principal areas of concern in this study relate to socioeconomic status and ethnicity. Our analysis used two methods of accounting for socioeconomic status: an area-based deprivation measure (IMD) linked to the individual patient via postcode and NHS exemption status at baseline. It is possible that some residual confounding could occur as a result of facets of socioeconomic status not captured by these measures, for example through level of education, or as a result of people of higher socioeconomic status living in deprived areas (or vice versa). This could limit our ability to fully account for the effect of socioeconomic status. The categories used for ethnicity in this study are also relatively broad, which could limit the ability of this measure to truly account for the impact of ethnicity for all ethnic groups. Furthermore, we did not have any ethnicity information recorded during any course of treatment for 20% of our sample. While the direction and effect of ethnicity on oral health outcomes in the UK are not clear at present, it is likely that these missing data could have led to residual confounding due to ethnicity. 176 Greater completion of ethnicity data in NHS dental records is needed.
We did not take account of variation in UDA values across different contracts, and how this may influence the behaviour of dentists. This was because we did not have access to any other KPIs that those contracts were commissioned to deliver; therefore, UDA values calculated by dividing total financial contract value by UDA target cannot be assumed to be reflective of the true payment: targets ratio of any given service. Furthermore, operating costs for dental surgeries do vary in different regions of the country; therefore, it is not possible to know how much of the observed variation in UDA values is actually appropriate given the variation in overheads.
Equality, diversity and inclusion
The study uses routinely collected data from a range of different types of NHS dental services. By including data from urgent and out-of-hours dental services, community/special care dental services and prison dental care, in addition to GDSs (high-street practices), we widened the study population to include groups which are less likely to be regular attenders to high-street practices. In doing so, we obtained a study sample that was relatively close to the general population of England in terms of deprivation, albeit with slight under-representation in the most and least deprived deciles. In terms of age, the study population reflects those who we know are more likely to use NHS dental services and the age groups 25–75 were somewhat over-represented compared to those aged under 25 and over 75. In terms of ethnicity, 23.5% of patients in the full study population did not have any ethnicity information recorded at any course of treatment over the 10-year period, so comparisons on the alignment of the study sample with the general population are limited. However, of those who did have ethnicity data recorded at least once, the proportion of white ethnicity was over-represented compared to England 2011 census population (89.4% compared to 85.4% white in England), while all other ethnic groups were under-represented. This may be a true reflection of users of NHS dental services, but it could also be caused by variation in ethnicity data completeness by ethnic group. This is a limitation of the ethnicity data collected by NHS dental services and any steps that can be taken to improve the recording of this information would be welcomed.
Our preliminary PPI work (when preparing the original research proposal) was undertaken in Salford, an ethnically diverse and socioeconomically deprived inner-city location in the North of England. This location was specifically chosen to involve a potentially underserved group in setting the research agenda and choice of primary outcome. We have sought to involve a diverse range of PPI voices throughout the study and using different methods of recruitment at different stages has been helpful in this regard. This included both national and local channels. We are cognisant that when developing and testing our patient-facing dissemination materials, we will need to seek opinions from people with a range of backgrounds and will endeavour to ensure the materials meet readability guidelines177 and use easy-read images and symbols rather than text where possible. The study website was also developed with these principles in mind and the colours are suitable for those with colour blindness and the page is designed to be readable by text-reader software. Similarly, we hope that throughout this report we have made complex technical language as accessible as possible by including explanations or using a more familiar word where possible. The report has been proofread by our patient and public engagement representative and another non-clinical colleague with the aim of increasing readability. Finally, the research team does include people of different ethnicities, genders and ages, and development opportunities have been provided for more junior staff members through this study.
Interpretation
Introduction
This study is not a traditional evaluation of water fluoridation. Historically, epidemiological evaluations of dental caries prevention interventions usually use clinical measures of disease (DMFT/S) as the primary outcome measure and try to recruit representative samples of the exposed and unexposed comparator populations. Follow-up of the exposed and comparator samples is usually prospective and ideally for a minimum of 3 years. 47 The MRC (2002) report on water fluoridiation163 and systematic reviews of water fluoridation point to the lack of high-quality studies examining the effect of water fluoridation on caries in adults. 47 The rationale for our approach in using NHS activity data is that it enabled an affordable, contemporary and initial assessment of the effect of water fluoridation on an adult population in England.
While the clinical results suggest very small effects of water fluoridation that stakeholders may not consider to be meaningful, the health economic analysis suggests that water fluoridation represents a positive ROI for the public sector. These apparently conflicting findings can be understood in the following ways. Firstly, the ROI analysis used total NHS treatment costs and includes all types of NHS dental treatment, not just invasive dental treatments (e.g. urgent dental care). Secondly, the per-person reduction in overall NHS dental treatment costs was of a similarly small magnitude to the clinical results in relative terms (5.5% lower NHS treatment costs compared to a 3% lower rate of NHS invasive dental treatments). However, because the costs of NHS dentistry are so much higher than the costs of water fluoridation (£402.22 vs. £10.30 per person over 10 years in 2020 prices), even relatively small reductions in NHS dental service utilisation will result in positive returns. Finally, it must be remembered the estimated ROI is for the period of observation only and does not include the significant capital investment that would be required if a new water fluoridation programme was established. It was not possible to include the original set-up costs for the schemes currently operating in England because they were commissioned around 60 years ago.
If water fluoridation continues to provide effective disease suppression in adults at a similar level to that reported for children in the most recent Cochrane systematic reviews (mean DMFT 1.16 teeth lower per person in the optimally fluoridated group (95% CI −1.61 to −0.72)),47 one would expect that a large difference in invasive treatments between exposed and non-exposed populations would be readily found. The observed reduction in the number of invasive dental treatments received per person by those in the optimally fluoridated group was smaller than what the majority of stakeholders we consulted with (patients, the public and dental professionals) felt would be meaningful. The stakeholders were drawn from both within the North West and across the UK, but it is important to remember that the workshops were an involvement and engagement activity rather than an attempt to produce a generalisable cut-off. Nonetheless, relative effect sizes of 3% or less have previously been referred to as ‘exceedingly small’ effects, which warrant cautious interpretation as they ‘could be eliminated with even minimal biases and their importance is uncertain’. 161
Potential explanations for the failure to find a definitively meaningful difference in clinical outcomes between exposed and non-exposed groups include:
-
a smaller effect size for water fluoridation in contemporary populations in which fluoride toothpaste use is ubiquitous than has been previously reported in historical studies (without widespread use of fluoride toothpaste and higher disease levels) and by systematic reviews made up predominantly of historical studies. This is supported by more recently published studies as outlined in How the results sit with the wider literature.
-
lack of a clear relationship between individual levels of dental disease and treatment provision due to perverse contracting incentives within NHS dentistry which provide no additional remuneration over and above one treatment within each payment ‘band’.
While we feel it is unlikely that we have missed a large/meaningful effect, we cannot rule out some underestimation of the effect of water fluoridation, as a result of using data from NHS dental services. The current contracting model for dental services incentivises dentists to modify their treatment patterns to hit contract activity targets (which are not aligned to population need). 94,178 Dentists working in deprived areas with patients that have a higher need for care are required to undertake more clinical work for the same UDA credit. Under this contracting system, there is no financial incentive for a dentist to provide three fillings compared to one. This disincentive to ‘find’ both patients and teeth that need treatment could have resulted in an underestimation of the true effect of water fluoridation. There are major concerns about NHS dental services and it is now over 13 years since publication of the Steele Review and the promise of a new NHS dental contract in England. 179 A fresh consideration of how the resources available for oral health should be allocated to provide a service based on population need is long overdue.
-
Limitations in our ability to detect a meaningful difference as a result of using routinely collected and uncalibrated data for outcomes.
We do not feel that this is the case, because we were able to detect a meaningful difference in the number of invasive NHS dental treatments received as a result of deprivation (1.285 more per person comparing the most to the least deprived decile). Similarly, we were able to detect a difference in the number of missing teeth per person as a result of deprivation (1.19 more per person comparing the most to the least deprived decile). This indicates that with this very large sample size, these outcome measures are sufficiently sensitive to detect even small effects (i.e. the finely graded small differences between each decile), when they were present.
With regard to generalisability, there are some slight differences in the characteristics of our analytical (matched) cohort compared to the general population of NHS dental service users as a result of the matching process (and the small proportion of the English population receiving water fluoridation). In the interests of increased internal validity, the matched analytical cohort was made more similar to the optimally fluoridated population of England than the sub/non-optimally fluoridated population. Our matched analytical cohort is therefore slightly more urban, more ethnically diverse, more deprived and has a lower proportion of NHS fee-paying patients than the sub/non-optimally fluoridated population of England. 180 Despite these demographic differences, the clinical characteristics of the unmatched and matched cohorts were very similar and we are confident that our findings are generalisable to the wider population of NHS dental service users in England (the 2009 Adult Dental Health Survey found that 58% of adults had tried to make an NHS dental appointment in the previous 3 years and of these 92% successfully received and attended an appointment). 181
Implications for policy, public health practice and research
This is one of the few studies on water fluoridation that has included adults and adolescents. Using routinely collected data and an innovative design meant it was possible to address some of the MRC research priorities for water fluoridation which were first articulated in 2002. However, triangulation and the use of ‘interlocking evidence’ are recommended in guidance on the evaluation of population health interventions. 101,182 The results of this study and the recently published NIHR CATFISH study in children both support the hypothesis that water fluoridation appears to be producing less dramatic improvements in oral health in contemporary UK populations than it did in historical studies. Fluoride toothpastes and professionally applied fluorides are now ubiquitous, and as a result the prevalence and severity of dental caries have greatly reduced compared to when the majority of studies were carried out. Similar findings of small clinical differences in absolute terms have been found in recent studies of adult populations in Australia, the USA and Sweden. 62,66,70 Water fluoridation may now represent a classic ‘prevention paradox’; a preventive measure which can bring benefits for populations and services but ‘offers little to each participating individual’. 183 This was not the case when water fluoridation was originally discovered and implemented, when the early studies identified noticeable reductions in caries of around 50% per person. 184,185 Whether the case for water fluoridation can be made based solely on the potential for reductions in NHS dental service utilisation and savings in NHS treatment costs, rather than health gains that are noticeable and meaningful to patients and the public, needs to be considered by stakeholders. Participatory consultation methods, for example, citizen juries, may be beneficial to explore this issue further.
Decision-making context must be considered when evaluating the transferability of our findings internationally. Factors of relevance include the prevalence and severity of dental caries in the population, access to alternative sources of fluoride and universal preventive dental care, costing arrangements for both dental care and water fluoridation, and current coverage of community drinking water and water fluoridation. Settings with high caries rates and limited access to preventive dentistry and alternative sources of fluoride may find that water fluoridation is still producing large health impacts in their population. Decisions regarding expansion of water fluoridation versus the cessation of existing schemes will also need to be approached very differently. Both the present study and the recent CATFISH study of the effectiveness of water fluoridation in children support the conclusion that existing schemes in the UK are cost-saving. 164 On the other hand, new schemes can require significant capital investment which may not be recovered during their lifespan in (increasingly) low caries populations.
The costs of any new water fluoridation programmes are extremely variable and depend on local water supply configurations. 186 Any cost estimates for new water fluoridation programmes or significant investment in existing schemes should be viewed in the context of our estimate that receipt of optimal water fluoridation (≥ 0.7 mg F/l) over 10 years reduced NHS dental treatment costs by 5.5% per person. Breaks in the supply of optimally fluoridated water and suboptimal dosing are relatively common103 and would result in a smaller impact of water fluoridation programmes on NHS treatment costs. To provide some context on the potential costs of a new scheme, a 2009 feasibility report estimated that the capital works to establish a programme to fluoridate the whole of the North West of England would be £102,124,677 in 2007 prices (± 30%). For Greater Manchester and Merseyside the estimate was £35,447,837 (± 30%). 149 In 2021 prices, these estimates would be £139,288,507.19 for the North West and £48,347,534.05 for Greater Manchester and Merseyside. 187 We received estimates on the projected lifespan of such equipment from two of the five water companies that supply fluoridated water in England. Based on their experience, the fluoridation dosing equipment was estimated to last between 15 and 20 years and the civil infrastructure (e.g. building, roads, pipes) was estimated to have a lifespan of 60 years.
There is no doubt that population-level, ‘mass preventive’ interventions for dental caries are still required. While disease levels have fallen dramatically in high-income countries over the last 40 years, dental caries remains almost universal by adulthood, even in populations that have had access to fluoride toothpastes and fluoridated water from birth. 5,38 We may be reaching the limit of improvements that can be achieved through fluorides alone. A dose–response relationship between free sugars and dental caries is evident at all levels of intake above zero and fluorides merely attenuate this relationship. 8,36–39 Average consumption of free sugars in the UK is more than double the recommended level for adolescents, and it is almost double for adults. 9,10 Only 7% of adolescents aged 11–18 and 17% of adults aged 19–64 meet the recommendation that free sugars should make up no more than 5% of total energy intake. 10 In populations with widespread access to fluoride toothpastes and topical fluorides, policy interventions related to the social and commercial determinants of health which change the food environment, improve diet quality and reduce sugar consumption may offer greater improvements for the dental health of populations and individuals than further expansion of water fluoridation. 188 Dental and dental public health professionals who seek to prevent caries have a great deal in common with those seeking to promote healthy weight and prevent other nutrition-related chronic diseases. 15 These relationships have been underdeveloped in the past but working together on sugar reduction may help us to move beyond the fluoride era and achieve further advances in caries prevention.
Recommendations for future research
-
National Health Service primary care dental data are a potentially valuable resource for research, surveillance and epidemiology. Consideration should be given as to how to develop this resource further. Suggestions include: commissioning enhanced data collection through a network of dental providers (similar to the medical G.P. Clinical Practice Research Datalink network), mandatory use of NHS numbers, recording the reason for treatment (caries, periodontal disease, trauma, repair of old restoration), steps to increase the completeness of patient ethnicity data and validation of the DMFT data.
-
Routinely collected water quality monitoring data should be made publicly available. A central, annually updated database of water fluoride concentrations (mg F/l) at small area level is required to support future research and monitoring of water fluoridation.
-
To broaden the evidence base for triangulation, the effect of water fluoridation on the dental health of adults should be investigated in any future national dental epidemiology surveys.
-
To determine if the estimated cost to avoid one invasive dental treatment (the ICER) represents good value for money, further work is required to identify a threshold level for ‘good value’ for this outcome.
Conclusions
Optimal water fluoridation produced limited heath gains in adults and adolescents which may not be considered meaningful by stakeholders. There were very small reductions in the number of invasive dental treatments received and DMFT, and no difference in the mean number of missing teeth, per person. There was no compelling evidence that water fluoridation reduced social inequalities in health.
Slightly lower dental service utilisation in the optimally fluoridated group led to a positive ROI for water fluoridation during the period of observation, but this did not include the significant costs of setting up a new water fluoridation programme.
Whether the case for water fluoridation programmes can be made solely on predicted ROI rather than health improvements that are meaningful to patients and the public may need to be considered by stakeholders.
Additional information
Acknowledgements
The Patient Data Citation:
This work uses data provided by patients and collected by the NHS as part of their care and support
The patient data citation was created by the members of use MY data, a movement of patients, relatives, carers who campaign for patient data to be to save lives and improve treatments. Understanding Patient Data adopted the citation and have been instrumental in helping to spread the message.
The research team would like to thank the patients who contributed their data to this work and those who continue to support the use of patient data for public good.
The research team would like to thank Dr Richard Hoskins, infrastructure analyst at the University of Manchester, for his expertise and generous support.
The research team would like to thank Nikki Dodds, NHS Business Services Authority, for her hard work in selecting the LOTUS study population.
The research team would like to thank all NHS Business Services Authority for their assistance in accessing these data.
The research team would like to thank the members of the North East, Tyne and Wear Research Ethics Committee and the members of the HRA Confidentiality Advisory Group.
The research team would like to thank Lynne Macrae and Dr Ben Pietras at the University of Manchester for their support with research governance and research I.T.
The research team would like to thank the peer reviewers and the editorial team at NIHR Public Health Research. We are indebted to you for your suggestions which have made this report a stronger piece of work.
LOTUS Study Steering Committee members
Chair: Dr Girvan Burnside, Senior Lecturer in Biostatistics, University of Liverpool.
Professor Mike Kelly, Senior Visiting Fellow, University of Cambridge.
Dr Alexander John Morris, Senior Lecturer in Dental Public Health, University of Birmingham.
Mr Shazad Saleem, General Dental Practitioner, Glodwick Dental Centre, Oldham, OL8 2BS.
Mr Mohsan Ahmad, General Dental Practitioner, Glodwick Dental Centre, Oldham, OL8 2BS.
Dr Philip Bell, Patient and Public Representative.
Dr Jing Shen, Senior Lecturer in Health Economics, Takeda Pharmaceutical Company Limited and The University of Newcastle.
Mrs Anna Ireland, National lead for Dental Public Health, Public Health England/Office for Health Improvement and Disparities, Department of Health and Social Care.
Professor Tjeerd Van Staa, Professor of Health e-Research, The University of Manchester.
Former LOTUS Study Steering Committee members:
Mrs Sandra White, National lead for Dental Public Health, Public Health England.
Mr David Regan, Director of Public Health, Manchester City Council.
We would like to thank the current and former members of the LOTUS Study Steering Committee for giving generously of their time and expertise.
Contributions of authors
Deborah Moore (https://orcid.org/0000-0001-9965-9371) (Research Associate, Dental Public Health) was involved in the study design, data collection, conduct and analysis and reporting phases. Lead areas included: Coordination of the funding application, project management and approvals, literature review, choice of matching variables and directed acyclic graphs, patient and public engagement, and coordination of the drafting and submission of this report.
Blessing Nyakutsikwa (https://orcid.org/0000-0002-9050-7926) (Research Associate, Biostatistics) was involved in the data collection, conduct and analysis and reporting phases. Lead areas included: Water fluoridation GIS mapping, data preparation and statistical analysis.
Thomas Allen (https://orcid.org/0000-0002-2972-7911) (Research Fellow, Health Economics) was involved in the study design, data collection, conduct and analysis and reporting phases. Lead areas included: Design and conduct of the health economics analysis and interpretation and reporting of the health economic results.
Emily Lam (Patient and Public Engagement representative) was involved in the conduct and reporting phases and contributed to the design of the meaningful difference workshops, drafting the PPI chapter, reviewing this report and the development of the dissemination materials.
Stephen Birch (https://orcid.org/0000-0002-1372-0440) (Professor, Health Economics) was involved in was involved in the study design, data collection, conduct and analysis and reporting phases. Lead areas included: Provision of senior health economics advice.
Martin Tickle (https://orcid.org/0000-0001-5348-5441) (Emeritus Professor, Dental Public Health) was involved in the study design, data collection, conduct and analysis and reporting phases. Lead areas included: Provision of senior dental public health advice.
Iain A Pretty (https://orcid.org/0000-0001-6298-4189) (Professor, Dental Public Health) was involved in the study design, data collection, conduct and analysis and reporting phases. Lead areas included: Co-PI providing senior leadership of the project, senior dental public health advice and supervision of DM and BN.
Tanya Walsh (https://orcid.org/0000-0001-7003-8854) (Professor, Healthcare Evaluation) was involved in the study design, data collection, conduct and analysis and reporting phases. Lead areas included: Co-PI providing senior leadership of the project, design of the statistical analysis and reporting of results and supervision of DM and BN.
Disclosure of interests
Full disclosure of interests: Completed ICMJE forms for all authors, including all related interests, are available in the toolkit on the NIHR Journals Library report publication page at https://doi.org/10.3310/RFQA3841.
Primary conflicts of interest: Iain A Pretty reports funding from Colgate-Palmolive Company (New York, NY, USA), grants from NIHR and editorial board member payments from Wiley-Blackwell (Hoboken, NJ, USA). Deborah Moore reports working at the Colgate-Palmolive Dental Health Unit 2017–23, which received grant funding from the Colgate-Palmolive Company (New York, NY, USA). Tanya Walsh reports grant funding from NIHR, institutional consulting fees from FDI and panel membership for NIHR Research for Patient Benefit North West. Martin Tickle reports grant funding from NIHR. Emily Lam reports panel and committee memberships for NIHR.
Data-sharing statement
Due to the terms of the data-sharing agreement with NHS Business Services Authority, there are no NHS patient data that can be shared. All data requests should be sent to the corresponding author in the first instance.
The raw (non-imputed) water fluoride concentration data are available to download on Figshare: https://figshare.manchester.ac.uk/articles/dataset/Water_fluoride_concentrations_mgF_L_per_LSOA_in_England_2009_-_2020_/15104730
Ethics statement
The design of the study was peer reviewed by the NIHR Public Health Research panel and was prospectively registered with ISRCTN on 13/01/2020 (ISRCTN96479279). The study received ethical approval from North East, Tyne & Wear South Research Ethics Committee on 27.05.2020 (20/NE/0144). The study protocol was published in 2021. 99
To allow NHS BSA to process patient identifiable data without consent (to select the study population and anonymise the data before transfer to the research team), section 251 support under Regulation 5 of the Health Service (Control of Patient Information) Regulations 2002 was required. This was granted by the Health Research Authority Confidentiality Advisory Group on 07.07.2020 (20/CAG/0072).
Information governance statement
The University of Manchester is committed to handling all personal information in line with the UK Data Protection Act (2018) and the General Data Protection Regulation (EU GDPR) 2016/679.
Under the Data Protection legislation, The University of Manchester and The NHS Business Services Authority are joint data controllers in relation to the NHS data used in this study and these data are handled according to a joint controller arrangement and data sharing agreement.
You can find out more about how The University of Manchester handles personal data, including how to exercise your individual rights and the contact details for The University of Manchester’s Data Protection Officer here. Information about what personal data the NHS Business Services Authority hold and how they process it is available here.
Department of Health and Social Care disclaimer
This publication presents independent research commissioned by the National Institute for Health and Care Research (NIHR). The views and opinions expressed by authors in this publication are those of the authors and do not necessarily reflect those of the NHS, the NIHR, MRC, NIHR Coordinating Centre, the PHR programme or the Department of Health and Social Care.
This monograph was published based on current knowledge at the time and date of publication. NIHR is committed to being inclusive and will continually monitor best practice and guidance in relation to terminology and language to ensure that we remain relevant to our stakeholders.
Accessibility of supplemental information
Data on annual water fluoride concentrations (mg F/l) per LSOA in England (2009–20) are available via the University of Manchester’s open data repository, Figshare. This data set does not contain any imputed values: https://doi.org/10.48420/15104730.v2
The patient-level data sets generated during and/or analysed during the current study are not expected to be made available due to the terms of the data-sharing agreement with the NHS Business Services Authority (data provider and joint data controller).
Publications
Moore D, Allen T, Birch S, Tickle M, Walsh T, Pretty IA. How effective and cost-effective is water fluoridation for adults? Protocol for a 10-year retrospective cohort study. BDJ Open 2021;7:3.
Nyakutsikwa B, Allen T, Walsh T, Pretty I, Tickle M, Birch S, Moore D. Who are the 10%? Characteristics of the populations and communities receiving fluoridated water in England. Community Dent Heal 2022;39:247–53.
Nyakutsikwa B, Walsh T, Pretty I, Moore D. Water fluoride concentrations in England, 2009–2020. Community Dent Heal 2022;39:106–12.
Moore D, Nyakutsikwa B, Allen T, Lam E, Birch S, Tickle M, et al. How effective and cost-effective is water fluoridation for adults and adolescents? The LOTUS 10-year retrospective cohort study. Community Dent Oral Epidemiol. 2024 Jan 8. https://doi.org/10.1111/cdoe.12930. Epub ahead of print. PMID: 38191778.
Disclaimers
This article presents independent research funded by the National Institute for Health and Care Research (NIHR). The views and opinions expressed by authors in this publication are those of the authors and do not necessarily reflect those of the NHS, the NIHR, the PHR programme or the Department of Health and Social Care. If there are verbatim quotations included in this publication the views and opinions expressed by the interviewees are those of the interviewees and do not necessarily reflect those of the authors, those of the NHS, the NIHR, the PHR programme or the Department of Health and Social Care.
References
- Abajobir AA, Abate KH, Abbafati C, Abbas KM, Abd-Allah F, Abdulkader RS, et al. Global, regional, and national incidence, prevalence, and years lived with disability for 328 diseases and injuries for 195 countries, 1990–2016: a systematic analysis for the Global Burden of Disease Study 2016. Lancet 2017;390:1211-59.
- Peres MA, Macpherson LMD, Weyant RJ, Daly B, Venturelli R, Mathur MR, et al. Oral diseases: a global public health challenge. Lancet 2019;394:249-60.
- The Information Centre for Health and Social Care . 8: Access and Barriers to Care – A Report from the Adult Dental Health Survey 2009 2011. https://digital.nhs.uk/data-and-information/publications/statistical/adult-dental-health-survey/adult-dental-health-survey-2009-summary-report-and-thematic-series (accessed 11 January 2021).
- NHS England . Improving Dental Care and Oral Health – A Call to Action 2014. www.england.nhs.uk/wp-content/uploads/2014/02/imp-dent-care.pdf (accessed 16 March 2020).
- Broadbent JM, Foster Page LA, Thomson WM, Poulton R. Permanent dentition caries through the first half of life. Br Dent J 2013;215:1-6.
- Steele J, Shen J, Tsakos G, Fuller E, Morris S, Watt R, et al. The interplay between socioeconomic inequalities and clinical oral health. J Dent Res 2015;94:19-26.
- Scientific Advisory Committee on Nutrition. Carbohydrates and Health 2015. https://www.gov.uk/government/publications/sacn-carbohydrates-and-health-report (accessed 5 January 2024).
- Sheiham A, James WPT. A reappraisal of the quantitative relationship between sugar intake and dental caries: the need for new criteria for developing goals for sugar intake. BMC Public Health 2014;14.
- World Health Organization . Guideline: Sugars Intake for Adults and Children 2015. www.who.int/publications/i/item/9789241549028 (accessed 6 January 2023).
- Public Health England, Food Standards Agency . National Diet and Nutrition Survey. Rolling Programme Years 9 to 11 (2016 2017 to 2018 2019). Vol. 11 2020. https://assets.publishing.service.gov.uk/government/uploads/system/uploads/attachment_data/file/943114/NDNS_UK_Y9-11_report.pdf (accessed 14 December 2021).
- Aoba T. Solubility properties of human tooth mineral and pathogenesis of dental caries. Oral Dis 2004;10:249-57.
- Fejerskov O. Concepts of dental caries and their consequences for understanding the disease. Community Dent Oral Epidemiol 1997;25:5-12.
- Featherstone JDB. Dental caries: a dynamic disease process. Aust Dent J 2008;53:286-91.
- Solar O, Irwin A. A Conceptual Framework for Action on the Social Determinants of Health. Social Determinants of Health Discussion Paper 2 (Policy and Practice). Geneva; 2010.
- Watt RG, Sheiham A. Integrating the common risk factor approach into a social determinants framework. Community Dent Oral Epidemiol 2012;40:289-96.
- Ten Cate J, Featherstone J. Mechanistic aspects of the interactions between fluoride and dental enamel. Crit Rev Oral Biol Med 1991;2:283-96.
- Duggal MS, Toumba KJ, Amaechi BT, Kowash MB, Higham SM. Enamel demineralization in situ with various frequencies of carbohydrate consumption with and without fluoride toothpaste. J Dent Res 2001;80:1721-4.
- Dean H, Jay P, Arnold F, Elvove E. Domestic water and dental caries. II. A study of 2,832 white children, aged 12–14 years, of 8 suburban Chicago communities, including Lactobacillus acidophilis studies of 1,761 children. Public Health Rep 1941;56:761-92.
- Dean HT, Arnold FA, Elvove E. Domestic water and dental caries. V. Additional studies of the relation of fluoride domestic waters to dental caries experience in 4,425 white children, aged 12 to 14 years, of 13 cities in 4 States. Public Health Rep 1942;57:1155-79.
- Dean H, Arnold F, Jay P, Knutson J. Studies on mass control of dental caries through fluoridation of the public water supply. Public Health Rep 1950;65:1403-8.
- British Fluoridation Society . The Extent of Water Fluoridation 2012. https://bfsweb.org/extent/.
- Australian Government National Health and Medical Research Council . Water Fluoridation and Human Health in Australia: Questions and Answers 2017. www.nhmrc.gov.au/sites/default/files/documents/attachments/water-fluoridationqa.pdf (accessed 11 January 2021).
- Walsh T, Worthington HV, Glenny AM, Marinho VCC, Jeroncic A. Fluoride toothpastes of different concentrations for preventing dental caries (review). Cochrane Database Syst Rev 2019;3. www.cochranelibrary.com/cdsr/doi/10.1002/14651858.CD007868.pub3/full (accessed 11 January 2023).
- Marinho VCC, Chong LY, Worthington HV, Walsh T. Fluoride mouthrinses for preventing dental caries in children and adolescents. Cochrane Database Syst Rev 2016;2016:1-6. www.cochranelibrary.com/cdsr/doi/10.1002/14651858.CD002284.pub2/full (accessed 14 December 2021).
- Marinho VCC, Worthington HV, Walsh T, Chong LY. Fluoride gels for preventing dental caries in children and adolescents (review). Cochrane Database Syst Rev 2015;2015. http://doi.wiley.com/10.1002/14651858.CD002280.pub2 (accessed 11 January 2023).
- Marinho VCC, Worthington HV, Walsh T, Clarkson JE. Fluoride varnishes for preventing dental caries in children and adolescents (review). Cochrane Database Syst Rev 2013. www.cochranelibrary.com/cdsr/doi/10.1002/14651858.CD002279.pub2/full (accessed 14 December 2021).
- Department for Health and Social Care, Office for Health Improvement and Disparities, NHS England, NHS Improvement . Delivering Better Oral Health: An Evidence-Based Toolkit for Prevention 2021. www.gov.uk/government/publications/delivering-better-oral-health-an-evidence-based-toolkit-for-prevention (accessed 14 December 2021).
- Marthaler TM. Salt fluoridation and oral health. Acta Med Acad 2013;42:140-55.
- Yeung C, Chong L, Glenny A. Fluoridated milk for preventing dental caries (review). Cochrane Database Syst Rev 1976. www.cochranelibrary.com/cdsr/doi/10.1002/14651858.CD003876.pub4/full (accessed 11 January 2023).
- Kassebaum NJ, Smith AGC, Bernabé E, Fleming TD, Reynolds AE, Vos T, et al. GBD 2015 Oral Health Collaborators . Global, regional, and national prevalence, incidence, and disability-adjusted life years for oral conditions for 195 Countries, 1990–2015: a systematic analysis for the global burden of diseases, injuries, and risk factors. J Dent Res 2017;96:380-7.
- Bratthall D, Hänsel-Petersson G, Sundberg H. Reasons for the caries decline: what do the experts believe?. Eur J Oral Sci 1996;104:416-22; discussion 423.
- Marthaler TM. Changes in dental caries 1953–2003. Caries Res 2004;38:173-81.
- Murray JJ, Vernazza CR, Holmes RD. Forty years of national surveys: an overview of children’s dental health from 1973–2013. Br Dent Joural 2015;219:281-5.
- Steele JG, Treasure ET, O’Sullivan I, Morris J, Murray JJ. Adult Dental Health Survey 2009: transformations in British oral health 1968–2009. Br Dent J 2012;213:523-7.
- NHS Digital . Adult Dental Health Survey 2009 – Summary Report and Thematic Series 2011. https://digital.nhs.uk/data-and-information/publications/statistical/adult-dental-health-survey/adult-dental-health-survey-2009-summary-report-and-thematic-series (accessed 11 January 2023).
- Bernabé E, Sheiham A. Extent of differences in dental caries in permanent teeth between childhood and adulthood in 26 countries. Int Dent J 2014;64:241-5.
- Bernabe E, Vehkalahti MM, Sheiham A, Lundqvist A, Suominen AL. The shape of the dose-response relationship between sugars and caries in adults. J Dent Res 2016;95:167-72.
- Broadbent JM, Thomson WM, Poulton R. Progression of dental caries and tooth loss between the third and fourth decades of life: a birth cohort study. Caries Res 2006;40:459-65.
- Sheiham A, James WPT. A new understanding of the relationship between sugars, dental caries and fluoride use: implications for limits on sugars consumption. Public Health Nutr 2014;17:2176-84.
- Cousson PY, Bessadet M, Nicolas E, Veyrune JL, Lesourd B, Lassauzay C. Nutritional status, dietary intake and oral quality of life in elderly complete denture wearers. Gerodontology 2012;29:e685-92.
- Office for National Statistics . One Third of Babies Born in 2013 Are Expected to Live to 100 2015. www.ons.gov.uk/ons/rel/lifetables/historic-and-projected-data-from-the-period-and-cohort-life-tables/2012-based/sty-babies-living-to-100.html (accessed 11 August 2022).
- Thomson WM, Ma S. An ageing population poses dental challenges. Singapore Dent J 2014;35:3-8.
- McDonagh M, Bradley M, Cooper J, Whiting P, Sutton A, Chestnutt I, et al. A Systematic Review of Public Water Fluoridation 2000. www.york.ac.uk/media/crd/crdreport18.pdf (accessed 11 January 2023).
- National Health and Medical Research Council . A Systematic Review of the Efficacy and Safety of Fluoridation. Part A: Review Methodology and Results 2007. https://www.nhmrc.gov.au/sites/default/files/documents/attachments/A-systematic-review-of-the-Efficacy-and-safety-of-Fluoridation-part-a%20.pdf (accessed 25 March 2024).
- Griffin SO, Regnier E, Griffin PM, Huntley V. Effectiveness of fluoride in preventing caries in adults. J Dent Res 2007;86:410-5.
- Community Preventive Services Task Force . Preventing Dental Caries: Community Water Fluoridation. Task Force Finding and Rationale Statement 2013. www.thecommunityguide.org/findings/dental-caries-cavities-community-water-fluoridation.html (accessed 11 January 2023).
- Iheozor-Ejiofor Z, Worthington H, Walsh T, O’Malley T, Clarkson J, Macey R, et al. Water fluoridation for the prevention of dental caries. Cochrane Database Syst Rev 2015;6:1-273. www.cochranelibrary.com/cdsr/doi/10.1002/14651858.CD010856.pub2/full (accessed 29 September 2021).
- McLaren L, Singhal S. Does cessation of community water fluoridation lead to an increase in tooth decay? A systematic review of published studies. J Epidemiol Community Health 2016;70:934-40.
- Jack B, Aysson M, Lewis S, Irving A, Agresta B, Ko H, et al. Health Effects of Water Fluoridation: Technical Report 2016. www.nhmrc.gov.au/about-us/publications/water-fluoridation-dental-and-other-human-health-outcomes#block-views-block-file-attachments-content-block-1 (accessed 12 November 2023).
- Jack B, Ayson M, Lewis S, Irving A, Agresta B, Ko H, et al. Health Effects of Water Fluoridation. Evidence Evaluation Report 2016. www.nhmrc.gov.au/about-us/publications/water-fluoridation-dental-and-other-human-health-outcomes#block-views-block-file-attachments-content-block-1 (accessed 11 January 2023).
- Canadian Agency for Drugs and Technologies in Health . Community Water Fluoridation Programs: A Health Technology Assessment-Review of Dental Caries and Other Health Outcomes. CADTH Technology Review No. 12 2019. www.cadth.ca (accessed 19 November 2020).
- Shea BJ, Reeves BC, Wells G, Thuku M, Hamel C, Moran J, et al. AMSTAR 2: a critical appraisal tool for systematic reviews that include randomised or non-randomised studies of healthcare interventions, or both. BMJ 2017;358:j4008-9.
- Canadian Agency for Drugs and Technologies in Health . Evidence Highlights: Community Water Fluoridation Programs 2019. www.cadth.ca (accessed 19 November 2020).
- Australian Government National Health and Medical Research Council (NHMRC) . Information Paper – Water Fluoridation: Dental and Other Human Health Outcomes 2017. www.nhmrc.gov.au/sites/default/files/documents/reports/fluoridation-info-paper.pdf (accessed 11 January 2023).
- Canadian Agency for Drugs and Technologies in Health . Community Water Fluoridation Programs: A Health Technology Assessment-Budget Impact Analysis. CADTH Technology Review No. 13 2019. www.cadth.ca (accessed 12 January 2023).
- Rugg-Gunn AJ, Do L. Effectiveness of water fluoridation in caries prevention. Community Dent Oral Epidemiol 2012;40:55-64.
- Slade GD, Sanders AE, Do L, Roberts-Thomson K, Spencer AJ. Effects of fluoridated drinking water on dental caries in Australian adults. J Dent Res 2013;92:376-82.
- Haysom L, Indig D, Byun R, Moore E, Van Den Dolder P. Oral health and risk factors for dental disease of Australian young people in custody. J Paediatr Child Health 2015;51:545-51.
- Barbato P, Peres MA. Tooth loss and associated factors in adolescents: a Brazilian population-based oral health survey. Rev Saúde Pública 2009;43:1-11.
- Crocombe LA, Brennan DS, Slade GD, Stewart JF, Spencer AJ. The effect of lifetime fluoridation exposure on dental caries experience of younger rural adults. Aust Dent J 2015;60:30-7.
- Koltermann AP, Giordani JMA, Pattussi MP. The association between individual and contextual factors and functional dentition status among adults in Rio Grande do Sul State, Brazil: a multilevel study TT – A associação entre aspectos individuais e contextuais na dentição funcional de adultos do R. Cad Saude Publica 2011;27:173-82.
- Neidell M, Herzog K, Glied S. The association between community water fluoridation and adult tooth loss. Am J Public Health 2010;100:1980-5.
- Crocombe LA, Brennan DS, Slade GD. Does lower lifetime fluoridation exposure explain why people outside capital cities have poor clinical oral health?. Aust Dent J 2016;61:93-101.
- Peres MA, Peres KG, Barbato PR, Höfelmann DA. Access to fluoridated water and adult dental caries. J Dent Res 2016;95:868-74.
- Spencer AJ, Liu P, Armfield JM, Do LG. Preventive benefit of access to fluoridated water for young adults. J Public Health Dent 2017;77:263-71.
- Do L, Ha D, Peres M, Skinner J, Byun R, Spencer AJ. Effectiveness of water fluoridation in the prevention of dental caries across adult age groups. Community Dent Oral Epidemiol 2017;45:225-33.
- Aguiar VR, Pattussi MP, Celeste RK. The role of municipal public policies in oral health socioeconomic inequalities in Brazil: a multilevel study. Community Dent Oral Epidemiol 2018;46:245-50.
- Chalub LLFH, Martins CC, Ferreira RC, Vargas AMD. Functional dentition in Brazilian adults: an investigation of social determinants of health (SDH) using a multilevel approach. PLOS ONE 2016;11:e0148859-18.
- Barbato PR, Peres MA, Höfelmann DA, Peres KG. Contextual and individual indicators associated with the presence of teeth in adults. Rev Saude Publica 2015;49:1-10.
- Aggeborn L, Öhman M. The effects of fluoride in drinking water. J Polit Econ 2017;129:465-91.
- Cho MS, Han KT, Park S, Moon KT, Park EC. The differences in healthcare utilization for dental caries based on the implementation of water fluoridation in South Korea. BMC Oral Health 2016;16.
- Rugg-Gunn AJ, Spencer AJ, Whelton HP, Jones C, Beal JF, Castle P, et al. Critique of the review of ‘Water fluoridation for the prevention of dental caries’ published by the Cochrane Collaboration in 2015. Br Dent Joural 2016;220:335-40.
- Roberto LL, Silveira MF, De Paula AMB, Ferreira E Ferreira E, Martins A, Haikal D. Contextual and individual determinants of tooth loss in adults: a multilevel study. BMC Oral Health 2020;20:1-10.
- Marques RB, Lima CCB, de Abreu Costa MLV, de Deus Moura de Lima M, de Fátima Almeida de Deus Moura L, Tabchoury CPM, et al. Fluoridated water impact on tooth decay and fluorosis in 17–20-year-olds exposed to fluoride toothpaste. J Public Health Dent 2021;82:385-94.
- Meyer J, Margaritis V, Jacob M. The impact of water fluoridation on Medicaid-eligible children and adolescents in Alaska. J Prev 2022;43:111-23.
- Batsos C, Boyes R, Mahar A. Community water fluoridation exposure and dental caries experience in newly enrolled members of the Canadian Armed Forces 2006–2017. Can J Public Heal 2021;112:513-20.
- Meyer J, Margaritis V, Mendelsohn A. Consequences of community water fluoridation cessation for Medicaid-eligible children and adolescents in Juneau, Alaska. BMC Oral Health 2018;18:1-10.
- Goodwin M, Emsley R, Kelly M, Sutton M, Tickle M, Walsh T, et al. Evaluation of water fluoridation scheme in Cumbria: the CATFISH prospective longitudinal cohort study. Public Heal Res 2022;10:1-106.
- AlQahtani SJ. Atlas of Human Tooth Development and Eruption. London: Barts and London School of Medicine and Dentistry; 2009.
- Mejia G, Jamieson LM, Ha D, Spencer AJ. Greater inequalities in dental treatment than in disease experience. J Dent Res 2014;93:966-71.
- Henry DB. The consequences of restorative cycles. Oper Dent 2009;34:759-60.
- Dale V, Gutacker N, Bloor K. NHS Dental Charges and the Effect of Increases on Access: An Exploration. York; 2021.
- Ofwat . Contact Details for Your Water Company 2021. www.ofwat.gov.uk/households/your-water-company/contact-companies/ (accessed 11 May 2021).
- The National Archives . The Water Supply (Water Quality) Regulations 2016 614 2016. www.legislation.gov.uk/uksi/2016/614/contents/made (accessed 11 January 2021).
- Drinking Water Inspectorate . Guidance on the Implementation of the Water Supply (Water Quality) Regulations 2016 (as Amended) in England and the Water Supply (Water Quality) Regulations (Wales) 2018 2018. www.dwi.gov.uk/water-companies/guidance-and-codes-of-practice/guidance-on-implementing-the-water-supply-water-quality-regulations/ (accessed 12 January 2023).
- United Kingdom Accreditation Service . LAB 37: Accreditation Requirements for Sampling and Testing in Accordance With the Drinking Water Testing Specification (DWTS) 2013. https://www.ukas.com/resources/publications/laboratory-accreditation/ (accessed 25 March 2024).
- mySociety . What Do They Know? 2020. www.whatdotheyknow.com (accessed 29 September 2021).
- The National Archives . The Functions of the National Health Service Commissioning Board and the NHS Business Services Authority (Awdurdod Gwasanaethau Busnes Y GIG) (Primary Dental Services) (England) Regulations 2013 469 2013. www.legislation.gov.uk/uksi/2013/469/contents/made (accessed 11 January 2021).
- The National Archives . The National Health Service (Dental Charges) Regulations 2005 3477 2005. www.legislation.gov.uk/uksi/2005/3477/contents/made (accessed 7 April 2021).
- NHS . Understanding NHS Dental Charges 2020. www.nhs.uk/using-the-nhs/nhs-services/dentists/understanding-nhs-dental-charges/ (accessed 6 March 2020).
- The National Archives . The National Health Service England (General Dental Services Contracts) Regulations 2005 3361 2005. www.legislation.gov.uk/uksi/2004/291/pdfs/uksi_20040291_en.pdf (accessed 7 April 2022).
- NHS England . Special Care Dentistry Clinical Standard PR1641 2022. https://www.england.nhs.uk/wp-content/uploads/2022/10/B1641-clincial-standard-special-care-dentistry.pdf (accessed 25 March 2024).
- National Audit Office . Dentistry in England 2020. www.nao.org.uk/wp-content/uploads/2020/03/Dentistry-in-England.pdf (accessed 18 March 2021).
- Harris R, Perkins E, Holt R, Brown S, Garner J, Mosedale S, et al. Contracting with General Dental Services: a mixed-methods study on factors influencing responses to contracts in English general dental practice. Health Soc Care Deliv Res 2015;3:1-194.
- British Dental Association . UDA Value Checker. 2022. Report No.: V2.3 n.d. https://bda.org/advice/Pages/UDA-value-checker.aspx (accessed 3 November 2023).
- The National Archives . The National Health Service (Personal Dental Services Agreements) Regulations 2005 3373 2005:1-90. www.legislation.gov.uk/uksi/2004/291/pdfs/uksi_20040291_en.pdf (accessed 21 April 2022).
- NHS England . Introductory Guide for Commissioning Dental Specialties 2015. https://england.nhs.uk/commissioning/wp-content/uploads/sites/12/2015/09/intro-guide-comms-dent-specl.pdf (accessed 5 January 2024).
- NHS . Get Help With Dental Costs n.d. www.nhs.uk/nhs-services/dentists/dental-costs/get-help-with-dental-costs/ (accessed 17 August 2022).
- Moore D, Allen T, Birch S, Tickle M, Walsh T, Pretty IA. How effective and cost-effective is water fluoridation for adults? Protocol for a 10-year retrospective cohort study. BDJ Open 2021;7:1-9.
- NHS Business Services Authority . Patient Identifiable Data Requests 2020. www.nhsbsa.nhs.uk/access-our-data-products/request-data/patient-identifiable-data-requests (accessed 25 May 2022).
- Medical Research Council . Using Natural Experiments to Evaluate Population Health Interventions: Guidance for Producers and Users of Evidence 2011. https://mrc.ukri.org/documents/pdf/natural-experiments-guidance/ (accessed 11 January 2021).
- Vocht FD, Katikireddi SV, Mcquire C, Tilling K, Hickman M, Craig P. Conceptualising natural and quasi experiments in public health. BMC Med Res Methodol 2021;21:1-8.
- Moore D, Goodwin M, Pretty I. Long-term variability in artificially and naturally fluoridated water supplies in England. Community Dent Oral Epidemiol 2020;48:49-55.
- Greenland S, Pearl J, Robins JM. Causal diagrams for epideimiologic research. Epidemiology 1999;10:37-48.
- Hernán M. Causal knowledge as a prerequisite for confounding evaluation: an application to birth defects epidemiology. Am J Epidemiol 2002;155:176-84.
- Lee J, Little TD. A practical guide to propensity score analysis for applied clinical research. Behav Res Ther 2017;98:76-90.
- Brookhart MA, Schneeweiss S, Rothman KJ, Glynn RJ, Avorn J, Stürmer T. Variable selection for propensity score models. Am J Epidemiol 2006;163:1149-56.
- Ho DE, Imai K, King G, Stuart EA. Matching as nonparametric preprocessing for reducing model dependence in parametric causal inference. Polit Anal 2007;15:199-236.
- Imbens GW. Nonparametric estimation of average treatment effects under exogeneity: a review. Rev Econ Stat 2004;86:4-29.
- Textor J, Hardt J, Knüppel S. DAGitty: a graphical tool for analyzing causal diagrams. Epidemiology 2011;22.
- Rothman K, Greenland S. Handbook of Epidemiology. New York: Springer Reference; 2014.
- Public Health England . Water Fluoridation: Health Monitoring Report for England 2018 2018. www.gov.uk/government/publications/water-fluoridation-health-monitoring-report-for-england-2018 (accessed 11 January 2021).
- Textor J, van der Zander B, Gilthorpe MS, Liśkiewicz M, Ellison GT. Robust causal inference using directed acyclic graphs: The R package ‘dagitty’. Int J Epidemiol 2016;45:1887-94.
- Ferguson KD, McCann M, Katikireddi SV, Thomson H, Green MJ, Smith DJ, et al. Evidence synthesis for constructing directed acyclic graphs (ESC-DAGs): a novel and systematic method for building directed acyclic graphs. Int J Epidemiol 2020;49:322-9.
- Tennant PWG, Murray EJ, Arnold KF, Berrie L, Fox MP, Gadd SC, et al. Use of directed acyclic graphs (DAGs) to identify confounders in applied health research: review and recommendations. Int J Epidemiol 2020;50:620-32.
- Smith AT, Noble M, Noble S, Wright G, Mclennan D. The English Indices of Deprivation 2015 Research Report 2015. www.gov.uk/government/publications/english-indices-of-deprivation-2015-research-report (accessed 15 January 2023).
- Department for Communities and Local Government . The English Indices of Deprivation 2015 2015. www.gov.uk/government/statistics/english-indices-of-deprivation-2015 (accessed 10 June 2021).
- Office for National Statistics . Rural Urban Classificiation (2011) of Lower Layer Super Output Areas in England and Wales 2018. https://data.gov.uk/dataset/b1165cea-2655-4cf7-bf22-dfbd3cdeb242/rural-urban-classification-2011-of-lower-layer-super-output-areas-in-england-and-wales (accessed 20 April 2022).
- Nyakutsikwa B, Walsh T, Pretty I, Moore D. Water fluoride concentrations in England, 2009-2020. Community Dent Health 2022;39:106-12.
- R Core Team . R: A Language and Environment for Statistical Computing 2018. www.r-project.org/ (accessed 12 January 2021).
- Lansley G, Cheshire J. Practical 7: Using R As a GIS. An Introduction to Spatial Data Analysis and Visualisation in R 2016. https://spatialanalysisonline.com/An Introduction to Spatial Data Analysis in R.pdf (accessed 1 September 2020).
- Brunsdon C, Comber L. An Introduction to R for Spatial Analysis and Mapping. Los Angeles, CA: Sage; 2019.
- Office for National Statistics . Lower Layer Super Output Areas (December 2011) Population Weighted Centroids 2020. https://geoportal.statistics.gov.uk/datasets/ons::lower-layer-super-output-areas-december-2011-population-weighted-centroids/about (accessed 1 August 2020).
- Office for National Statistics . Information Note: Population Weighted Centroids Guidance 2017. https://data.gov.uk/dataset/2c5695f2-39d0-457f-a03c-1f4d3617bb48/population-weighted-centroids-guidance (accessed 1 July 2020).
- Brunsdon C, Comber L. An Introduction to R for Spatial Analysis and Mapping. Thousand Oaks, California: Sage Publications, Inc; 2019.
- Office for National Statistics . Local Authority Districts (December 2020) UK BFC 2020. https://geoportal.statistics.gov.uk/datasets/ons::local-authority-districts-december-2020-uk-buc/about (accessed 25 March 2024).
- Office for National Statistics . Lower Layer Super Output Areas (December 2011) Boundaries Super Generalised Clipped (BSC) EW V2 2020. https://geoportal.statistics.gov.uk/search?collection=Dataset&sort=name&tags=all(BDY_LSOA%2CDEC_2011) (accessed 16 June 2020).
- Nyakutsikwa B, Walsh T, Pretty I, Moore D. Water Fluoride Concentrations (mg F L) Per LSOA in England (2009–2020). Dataset 2021. https://doi.org/10.48420/15104730.v2 (accessed 12 August 2021).
- Bergstra SA, Sepriano A, Ramiro S, Landewé R. Three handy tips and a practical guide to improve your propensity score models. RMD Open 2019;5:e000953-8.
- R Core Team . R: A Language and Environment for Statistical Computing 2021. https://R-project.org/ (accessed 01 November 2023).
- StataCorp . Stata Statistical Software: Release 14 2015.
- Ho DE, King G, Stuart EA, Imai KM. Nonparametric preprocessing for parametric causal inference. J Stat Softw 2011;42:1-28.
- Leite W. Practical Propensity Score Methods Using R. Thousand Oaks: Sage Publications; 2017.
- Rosenbaum P, Rubin DB. Constructing a control group using multivariate matched sampling models that incorporate the propensity score. Am Stat 1985;39:33-8.
- Cepeda M, Boston R, Farrar J, Strom B. Optimal matching with a variable number of controls vs. a fixed number of controls for a cohort study: trade-offs. J Clin Epidemiol 2003;56:230-7.
- Gu X, Rosenbaum P. Comparison of multivariate matching methods: structures, distances, and algorithms. J Comput Graph Stat 1993;2:405-20.
- Ming K, Rosenbaum P. Substantial gains in bias reduction from matching with a variable number of controls. Biometrics 2000;56:118-24.
- Ming K, Rosenbaum P. A note on optimal matching with variable controls using the assignment algorithm. J Comput Graph Stat 2001;10:455-63.
- Flury B, Riedwyl H. Standard distance in univariate and multivariate analysis. Am Stat 1986;40:249-51.
- Cohen J. Statistical Power Analysis for the Behavioral Sciences. Hillsdale, NJ: Lawrence Erlbaum Associates; 1988.
- Austin PC. Balance diagnostics for comparing the distribution of baseline covariates between treatment groups in propensity-score matched samples. Stat Med 2009;28:3083-107.
- Austin PC. An introduction to propensity score methods for reducing the effects of confounding in observational studies. Multivariate Behav Res 2011;46:399-424.
- StataCorp . Stata Statistical Software 2021.
- Hislop J, Adewuyi TE, Vale LD, Harrild K, Fraser C, Gurung T, et al. DELTA Group . Methods for specifying the target difference in a randomised controlled trial: the difference elicitation in trials (DELTA) systematic review. PLOS Med 2014;11.
- Rothwell JC, Julious SA, Cooper CL. A study of target effect sizes in randomised controlled trials published in the Health Technology Assessment journal. Trials 2018;19:1-13.
- Allison DB, Elobeid MA, Cope MB, Brock DW, Faith MS, Vander Veur S, et al. Sample size in obesity trials: patient perspective versus current practice. Med Decis Mak 2010;30:68-75.
- Broadbent JM, Thomson WM, Poulton R. Trajectory patterns of dental caries experience in the permanent dentition to the fourth decade of life. J Dent Res 2008;87:69-72.
- Thomson WM. Dental caries experience in older people over time: what can the large cohort studies tell us?. Br Dent J 2004;196:89-92.
- Rowe P. Report of the North West PCTs Fluoridation Evaluation Group 2008. https://documents.manchester.ac.uk/display.aspx?DocID=65164 (accessed 23 January 2023).
- HM Treasury . DDP Deflators at Market Prices, and Money GDP December 2021 (Quarterly National Accounts) 2022. www.gov.uk/government/statistics/gdp-deflators-at-market-prices-and-money-gdp-december-2021-quarterly-national-accounts (accessed 29 July 2022).
- Lowery G, Bunn S. Water Fluoridation and Dental Health 2021. https://post.parliament.uk/water-fluoridation-and-dental-health/ (accessed 10 May 2022).
- The National Archives . Freedom of Information Act 2000 2020. www.legislation.gov.uk/ukpga/2000/36/contents (accessed 12 January 2021).
- The Scottish Government . Statement of Dental Remuneration. 2019. Report No.: Amendment No. 142 n.d. https://scottishdental.org/professionals/statement-of-dental-remuneration/ (accessed 27 November 2020).
- Roberts DJ, Morris J, Wood A, Verlander NQ, Leonardi GS, Fletcher T. Use of public water supply fluoride concentration as an indicator of population exposure to fluoride in England 1995–2015. Environ Monit Assess 2020;192:1-16.
- Moimaz SAA, Saliba NA, Saliba O, Sumida DH, DeSouza NP, Chiba FY, et al. Water fluoridation in 40 Brazilian cities: 7 year analysis. J Appl Oral Sci 2002;21:13-9.
- Kuthy RA, Naleway C, Durkee J. Factors associated with maintenance of proper water fluoride levels. J Am Dent Assoc 1985;110:511-3.
- Pelletier AR. Maintenance of optimal fluoride levels in public water systems. J Public Health Dent 2004;64:237-9.
- Buzalaf MAR, Granjeiro JM, Damante CA, Ornelas F. Fluctuations in public water fluoride level in Bauru, Brazil. J Public Health Dent 2002;62:173-6.
- CDC . Shortages of Fluoridation Additives 2015. www.cdc.gov/fluoridation/engineering/engineering-shortages.htm (accessed 16 September 2022).
- Goldthorpe J, Walsh T, Tickle M, Birch S, Hill H, Sanders C, et al. An evaluation of a referral management and triage system for oral surgery referrals from primary care dentists: a mixed-methods study. Health Soc Care Deliv Res 2018;6:1-126.
- Siontis GCM, Ioannidis JPA. Risk factors and interventions with statistically significant tiny effects. Int J Epidemiol 2011;40:1292-307.
- McMaster University . Public and Patient Engagement Evaluation Tool (PPEET) V2 2018. https://healthsci.mcmaster.ca/ppe/our-products/public-patient-engagement-evaluation-tool/download (accessed 23 March 2022).
- Medical Research Council . Water Fluoridation and Health 2002. https://webarchive.nationalarchives.gov.uk/ukgwa/20220207162925/http://mrc.ukri.org/publications/browse/water-fluoridation-and-health/ (accessed 5 January 2024).
- National Institute for Health and Care Excellence (NICE) . Carrying NICE Over the Threshold. News 2015. www.nice.org.uk/news/blog/carrying-nice-over-the-threshold (accessed 8 November 2022).
- Moore D, Allen T, Boyers D, Mckenzie K, Thompson W, Nyakutsikwa B, et al. Unlocking the potential of NHS primary care dental datasets. Br Dent J 2022;232:241-50.
- Riordain RN, Glick M, Mashhadani SSAA, Aravamudhan K, Barrow J, Cole D, et al. Developing a standard set of patient-centred outcomes for adult oral health – an international, cross-disciplinary consensus. Int Dent J 2021;71:40-52.
- COMET Initiative . COMET Initiative (Core Outcome Measures in Effectiveness Trials). Search the COMET Database 2023. www.comet-initiative.org (accessed 27 January 2023).
- Cantrill JA, Sibbald B, Buetow S. The Delphi and nominal group techniques in health services research. Int J Pharm Pract 1996;4:67-74.
- Hagger-johnson G, Harron K, Goldstein H, Hagger-johnson G. Innovation in health informatics. J Innov Heal Informatics 2017;24:234-46.
- Hagger-Johnson G, Harron K, Fleming T, Gilbert R, Goldstein H, Landy R, et al. Data linkage errors in hospital administrative data when applying a pseudonymisation algorithm to paediatric intensive care records. BMJ Open 2015;5:e008118-8.
- Public Health England . Dental Public Health Epidemiology Programme. Oral Health Survey of Adults Attending General Dental Practices 2017 18 National Protocol. First 2017.
- NHS Business Services Authority . NHS Dental Services – Completion of Form Guidance FP17 – England 2023. https://www.nhsbsa.nhs.uk/activity-payment-and-pension-services/dental-activity-processing (accessed 25 March 2024).
- O’Sullivan I, Lader D, Beavan-Seymour C, Chenery V, Fuller E, Sadler K. Foundation Report: Adult Dental Health Survey 2009 (Technical Information). Health and Social Care Information Centre; 2011:1-138. http://doc.ukdataservice.ac.uk/doc/6884/mrdoc/pdf/6884foundation_report_and_technical_information.pdf (accessed 1 May 2024).
- Brown NL, Jephcote VEL, Morrison JN, Sutton JE. Inaccurate dental charting in an audit of 1128 general dental practice records. Dent Update 2017;44:254-60.
- Public Health England . National Dental Epidemiology Programme for England. Oral Health Survey of Adults Attending General Dental Practices 2018 2020. https://assets.publishing.service.gov.uk/government/uploads/system/uploads/attachment_data/file/891208/AiP_survey_for_England_2018.pdf (accessed 11 February 2021).
- Public Health England . Inequalities in Oral Health in England 2021. https://assets.publishing.service.gov.uk/government/uploads/system/uploads/attachment_data/file/970380/Inequalities_in_oral_health_in_England.pdf (accessed 11 May 2021).
- NIACE . Readability: How to Produce Clear Written Materials for a Range of Readers 2009. https://learningandwork.org.uk/resources/research-and-reports/readability-how-to-produce-clear-written-materials-for-a-range-of-readers/ (accessed 8 August 2015).
- Tickle M, McDonald R, Franklin J, Aggarwal VR, Milsom K, Reeves D. Paying for the wrong kind of performance? Financial incentives and behaviour changes in National Health Service dentistry 1992–2009. Community Dent Oral Epidemiol 2011;39:465-73.
- Steele J. NHS Dental Services in England. An Independent Review Led by Professor Jimmy Steele 2009. www.sigwales.org/wp-content/uploads/dh_101180.pdf (accessed 12 January 2023).
- Nyakutsikwa B, Allen T, Walsh T, Pretty I, Tickle M, Birch S, et al. Who are the 10%? Characteristics of the populations and communities receiving fluoridated water in England. Community Dent Heal 2022;39:247-53.
- Hill KB, Chadwick BL, Freeman R, O’Sullivan I, Murray JJ. Adult Dental Health Survey 2009: relationships between dental attendance patterns, oral health behaviour and the current barriers to dental care. Br Dent J 2013;214:25-32.
- Vandenbroucke JP, Broadbent A, Pearce N. Causality and causal inference in epidemiology: the need for a pluralistic approach. Int J Epidemiol 2016;45:1776-86.
- Rose G. Strategy of prevention: lessons from cardiovascular disease. Br Med J (Clin Res Ed) 1981;282:1847-51.
- Arnold F, Likens R, Russell A, Scott D. Fifteenth year of the Grand Rapids fluoridation study. J Am Dent Assoc 1962;65:780-5.
- Dirks OB, Houwink B, Kwant GW. The results of 6 1/2 years of artificial fluoridation of drinking water in The Netherlands. The Tiel-Culemborg experiment. Arch Oral Biol 1961;5:284-300.
- Public Health England . Improving Oral Health. A Community Water Fluoridation Toolkit for Local Authorities. GW-1634 2020. https://assets.publishing.service.gov.uk/government/uploads/system/uploads/attachment_data/file/953333/Fluoridation_Toolkit_V1.7.pdf (accessed 5 March 2021).
- Bank of England . Inflation Calculator 2022. www.bankofengland.co.uk/monetary-policy/inflation/inflation-calculator (accessed 26 September 2022).
- Public Health England . Sugar Reduction: The Evidence for Action 2015. www.gov.uk/government/uploads/system/uploads/attachment_data/file/470179/Sugar_reduction_The_evidence_for_action.pdf (accessed 25 March 2024).
- Morgan S, Winship C. Counterfactuals and Causal Inference: Methods and Principles for Social Research. New York: Cambridge University Press; 2007.
- Cole SR, Platt RW, Schisterman EF, Chu H, Westreich D, Richardson D, et al. Illustrating bias due to conditioning on a collider. Int J Epidemiol 2010;39:417-20.
- Williams TC, Bach CC, Matthiesen NB, Henriksen TB, Gagliardi L. Directed acyclic graphs: a tool for causal studies in paediatrics. Pediatr Res 2018;84:487-93.
- Hernán MA. The C-word: Scientific euphemisms do not improve causal inference from observational data. Am J Public Health 2018;108:616-9.
- Leite W. Practical Propensity Score Methods Using R. Thousand Oaks: Sage Publications, Inc; 2017.
- Greenland S, Morgenstern H. Confounding in Health Research. Annu Rev Public Health 2001;22:189-212.
- Ali MS, Prieto-Alhambra D, Lopes LC, Ramos D, Bispo N, Ichihara MY, et al. Propensity score methods in health technology assessment: principles, extended applications, and recent advances. Front Pharmacol 2019;10:1-19.
- Wanyonyi KL, Radford DR, Gallagher JE. Dental treatment in a state-funded primary dental care facility: contextual and individual predictors of treatment need?. PLOS ONE 2017;12:e0169004-17.
- Jo O, Kruger E, Tennant M. Disparities in the geographic distribution of NHS general dental care services in England [published online ahead of print May 27 2021]. Br Dent J 2021. https://doi.org/10.1038/s41415-021-3005-0.
- Chadwick BL, White D, Lader D, Pitts N. 5: Preventive Behaviour and Risks to Oral Health – A Report from the Adult Dental Health Survey 2009 2011. https://digital.nhs.uk/data-and-information/publications/statistical/adult-dental-health-survey/adult-dental-health-survey-2009-summary-report-and-thematic-series (accessed 5 January 2024).
- Weston-Price S, Copley V, Smith H, Davies GM. A multi-variable analysis of four factors affecting caries levels among five-year-old children; deprivation, ethnicity, exposure to fluoridated water and geographic region. Community Dent Health 2018;35:217-22.
- NHS England . G.P. Patient Survey – January to March 2019 2019. www.england.nhs.uk/statistics/2019/07/11/gpps_dent_8492_822742/ (accessed 25 March 2024).
- Delgado-Angulo EK, Mangal M, Bernabé E. Socioeconomic inequalities in adult oral health across different ethnic groups in England. Health Qual Life Outcomes 2019;17:3-9.
- Shah S, Wordley V. An overview of adult dental fee exemptions in NHS primary dental care in England. Br Dent J 2021;230:1-5.
- Thomas E, Serwicka I, Swinney P. Urban Demographics: Why People Live Where They Do. Centre for Cities; 2015.
- De Noronha N. Housing and the Older Ethnic Minority Population in England 2019. https://www.housinglin.org.uk/_assets/Resources/Housing/Support_materials/Briefings/HLIN_Briefing_BME_Housing.pdf (accessed 5 January 2024).
- Innes NPT, Schwendicke F. Restorative thresholds for carious lesions: systematic review and meta-analysis. J Dent Res 2017;96:501-8.
Appendix 1 Full search history for literature review
15 February 2022
Database: EBM Reviews – Cochrane Database of Systematic Reviews <2005 to 9 February 2022>, EBM Reviews – ACP Journal Club <1991 to January 2022>, EBM Reviews – Database of Abstracts of Reviews of Effects <1st Quarter 2016>, EBM Reviews – Cochrane Clinical Answers <January 2022>, EBM Reviews – Cochrane Central Register of Controlled Trials <January 2022>, EBM Reviews – Cochrane Methodology Register <3rd Quarter 2012>, EBM Reviews – Health Technology Assessment <4th Quarter 2016>, EBM Reviews – NHS Economic Evaluation Database <1st Quarter 2016>, EMBASE <1974 to 11 February 2022>, Ovid MEDLINE(R) and Epub Ahead of Print, In-Process, In-Data-Review & Other Non-Indexed Citations and Daily <1946 to 14 February 2022>
Search strategy:
----------- ------------ ----------- ------------- ------------ ---------- -----------
-
water fluorid$.mp. [mp = ti, ot, ab, tx, kw, ct, sh, hw, tn, dm, mf, dv, kf, fx, dq, nm, ox, px, rx, an, ui, sy] (3701)
-
(Adult or Adolescent or Older or Elderly).mp. [mp = ti, ot, ab, tx, kw, ct, sh, hw, tn, dm, mf, dv, kf, fx, dq, nm, ox, px, rx, an, ui, sy] (18,021,535)
-
(Caries or Decay or Tooth Loss or Dental Treatment).mp. [mp = ti, ot, ab, tx, kw, ct, sh, hw, tn, dm, mf, dv, kf, fx, dq, nm, ox, px, rx, an, ui, sy] (325,372)
-
limit 3 to yr=‘2018 -Current’ [Limit not valid in DARE; records were retained] (65,596)
-
limit 4 to humans [Limit not valid in CDSR,ACP Journal Club,DARE,CCA,CCTR,CLCMR; records were retained] (36,177)
-
1 and 2 and 3 and 4 and 5 (98)
-
remove duplicates from 6 (63)
-
from 7 keep 10,12,15-17,29,31,33,38,40,56,62 (12)
21 June 2022 update
Database: EBM Reviews – Cochrane Database of Systematic Reviews <2005 to 15 June 2022>, EBM Reviews – ACP Journal Club <1991 to May 2022>, EBM Reviews – Database of Abstracts of Reviews of Effects <1st Quarter 2016>, EBM Reviews – Cochrane Clinical Answers <May 2022>, EBM Reviews – Cochrane Central Register of Controlled Trials <May 2022>, EBM Reviews – Cochrane Methodology Register <3rd Quarter 2012>, EBM Reviews – Health Technology Assessment <4th Quarter 2016>, EBM Reviews – NHS Economic Evaluation Database <1st Quarter 2016>, EMBASE <1974 to 2022 Week 24>, Ovid MEDLINE(R) and Epub Ahead of Print, In-Process, In-Data-Review & Other Non-Indexed Citations, Daily and Versions <1946 to 20 June 202022>
Search strategy:
-------- --------- --------- ---------- -------- --------- -------- --------- ----------
-
water fluorid$.mp. [mp = ti, ot, ab, tx, kw, ct, sh, fx, hw, tn, dm, mf, dv, kf, dq, nm, ox, px, rx, an, ui, sy] (3722)
-
(Adult or Adolescent or Older or Elderly).mp. [mp = ti, ot, ab, tx, kw, ct, sh, fx, hw, tn, dm, mf, dv, kf, dq, nm, ox, px, rx, an, ui, sy] (18,341,528)
-
(Caries or Decay or Tooth Loss or Dental Treatment).mp. [mp = ti, ot, ab, tx, kw, ct, sh, fx, hw, tn, dm, mf, dv, kf, dq, nm, ox, px, rx, an, ui, sy] (331,355)
-
limit 3 to human [Limit not valid in CDSR,ACP Journal Club,DARE,CCA,CCTR,CLCMR; records were retained] (190,064)
-
limit 4 to yr=‘2022 -Current’ [Limit not valid in DARE; records were retained] (4183)
-
1 and 2 and 3 and 4 and 5 (11)
-
remove duplicates from 6 (9)
Appendix 2 Selection of covariates for matching
A key goal in studies of intervention effectiveness is to remove the spurious associations introduced by common causes, known as confounding. 105 Confounders are variables that when ‘conditioned on’ (through stratification, adjustment, or matching) can remove spurious associations created by common causes of a given exposure and outcome. 105 The identification of common causes (and therefore, confounding) can be aided by the drawing of DAGs. 104,105 DAGs are a diagrammatic representation of the hypothesised relationships between all common causes of the exposure and outcome of interest. 104,105
DAGs link study variables by arrows that represent direct causal effects, which can be protective or causative. There are two basic rules for creating a DAG:
-
For a diagram to represent a causal system, all shared causes of any two variables in the DAG must be included. 114
-
Causes must come before effects. This means the diagrams are ‘acyclic’: It should not be possible to follow the arrows to end up back at the same variable where you started.
Judgements about which variables should be included in a DAG and the direction of cause and effect through the connecting arrows are based on subject matter knowledge. It is important to include all plausible common causes of exposure and outcome. 115 This includes those that are measurable and available within the study data set, and those that are unmeasurable and/or not included in the study data set. 115
Practical guidance on creating DAGs suggests the following steps may be useful:114,115
-
Identify which variables have been used to control for confounding in similar studies by conducting a literature review.
-
After reviewing the literature and using subject knowledge, are all plausible common causes of exposure and outcome identified?
-
It is easier to interpret and read DAGs that flow in one consistent direction (e.g. from left to right or top to bottom). This makes the direction of temporality and causality clearer.
-
To illustrate the relationships between the possible confounders, it is best to start with a DAG that is ‘saturated’. This means each variable is assumed to cause all possible future variables.
-
Connections between variables should only be removed when they are deemed to be implausible based on expert knowledge or relevant theory. The absence of an arrow is a strong claim of no direct effect. 189
-
Decisions to remove arrows should be recorded in a decision log. 114 Possible reasons for removing an arrow include: temporality, lack of face validity, supporting theory of no direct effect and/or a thought experiment confirms the value of the putative ‘cause’ variable would not be expected to alter the value of the putative ‘effect’ variable. 114
-
Variables that are subcategories of a concept and whose input and output arrows start and end in the same places may be combined into one ‘supervariable’. 114 This makes the diagram less cluttered. 115
Paths on a DAG are collections of arrows, facing in any direction, for example -> -> <-. They can be open or closed. Open paths transmit statistical associations and closed paths do not. 115 Open paths can be closed by conditioning on any variable along the path. This will block the transmission of statistical associations through that path. Paths are also blocked and do not transmit statistical associations when they contain a ‘collider variable’. 115 A collider variable is a variable that receives two arrows (i.e. has two common causes). Care must be taken not to condition on collider variables or their descendants, because this can inadvertently open paths that were previously being blocked by the collider. This ‘overadjustment’ would introduce a spurious association between the two (previously unrelated) common causes of the collider. 104,105 Understanding this type of bias is not intuitive, but several clear worked examples are available which can help to illuminate collider bias further for the interested reader. 190
The purpose of drawing a DAG is to identify a ‘minimal sufficient adjustment set’. 114,115 This is a set of variables that when conditioned on will provide an un-confounded estimate of the causal effect of interest, by closing all open paths that would otherwise transmit spurious associations. 115 Open paths that can transmit confounding associations are also referred to as ‘backdoor paths’, or ‘biasing paths’. 191 In addition to identifying confounders, DAGs can also allow us to check that addition of further important prognostic variables to the adjustment set does not inadvertently open previously closed paths through conditioning on a collider variable. 104
Drawing DAGs can be done by hand. However, they can become very complex; a recent review of the use of DAGs115 reported that the number of variables ranged from 3 to 28 and the number of connections ranged from 3 to 99. To draw the DAGs and identify the minimum sufficient adjustment sets required to close confounding pathways, an online software package named Dagitty was used. 110
The causal effect192 that we wish to estimate in the present study is the effect of ‘receiving water fluoridation ≥ 0.7 mg F/L’ (exposure) on the ‘number of NHS dental treatments received’ (outcome). The causal effect of interest is also known as the study estimand. 115
The hypothesised DAG for our study is available online (https://dagitty.net/dags.html?id=O-tgYq) in interactive form and is presented as figures below illustrating six stages:
-
Figure 16 illustrates the hypothesised relationships between important variables related to our exposure and outcome in the real world. This includes both measured and unmeasured/unmeasurable variables.
-
Figure 17 is the same as Figure 16 but the variables that are unmeasurable or unmeasured in our study are represented with a grey oval.
-
Figure 18 illustrates the minimal sufficient adjustment set needed to close the open backdoor (confounding) pathways. These are the confounders (or ‘surrogate’ confounders) in our causal diagram. The variables that are being conditioned on to block the confounding pathways (through restriction, matching, stratification or adjustment) are represented with a white oval.
-
Figure 19 illustrates the addition of further variables that are ancestors of the outcome (also known as competing exposures or outcome predictors) to the adjustment set. This is to check that matching on variables hypothesised to be predictors of the outcome will not inadvertently create problems as a result of opening previously closed backdoor pathways through collider bias or conditioning on intermediates along the causal pathway. Propensity score matching guidance suggests the inclusion of predictor variables to increase precision of the effect estimates (though not reduce bias).
-
Figure 20 illustrates the effect of increased bias when fluoride varnish is added to the matching set.
-
Figure 21 presents the final DAG as used in the matching process.
FIGURE 16.
Directed acyclic graph illustrating the hypothesised causal pathways connecting exposure to drinking water containing ≥ 0.7 mg F/l and the number of NHS invasive dental treatments received and oral health 2010–20 before any variables are conditioned on.
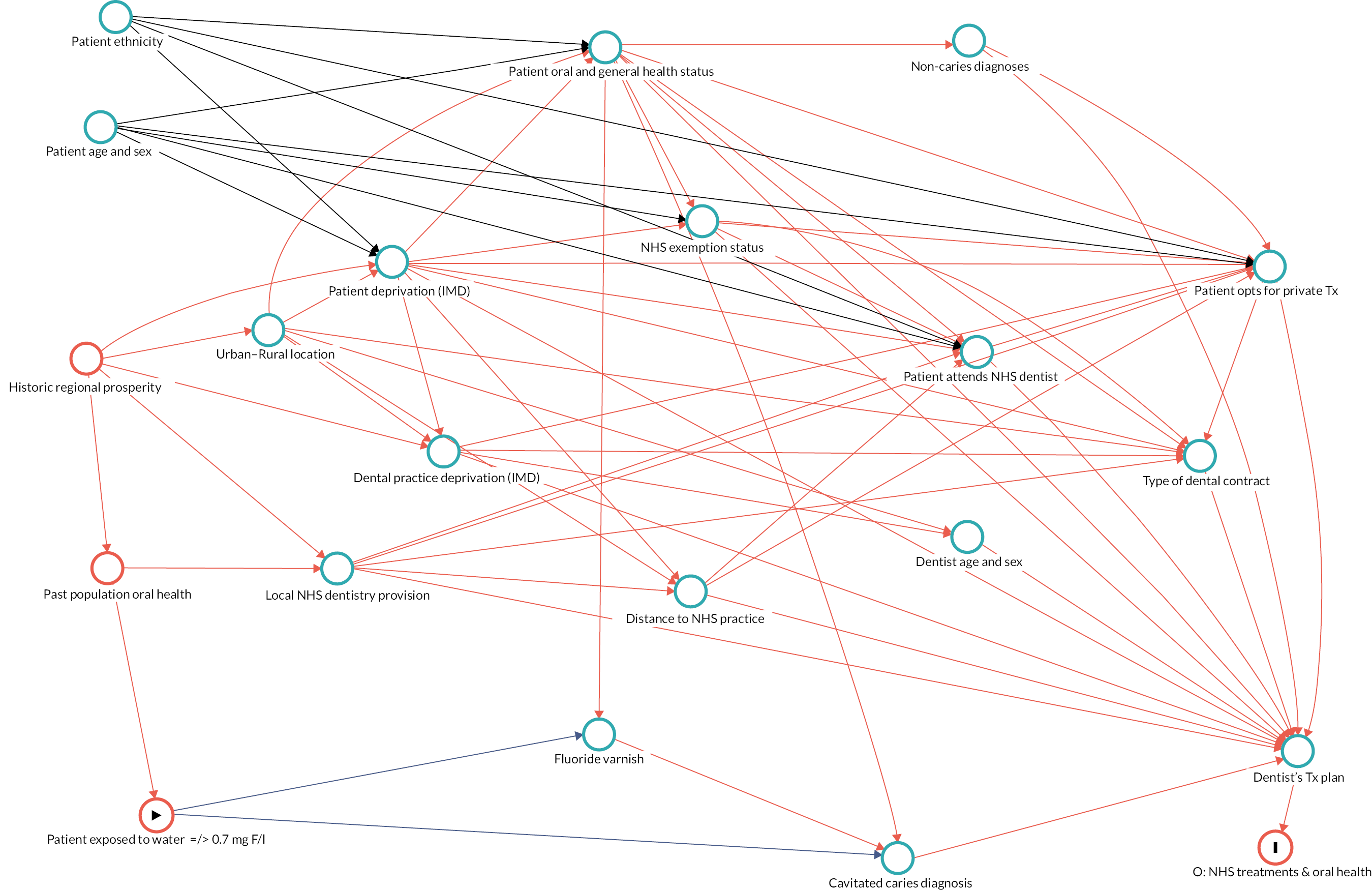
FIGURE 17.
Directed acyclic graph illustrating the unmeasured variables in the LOTUS study in grey.
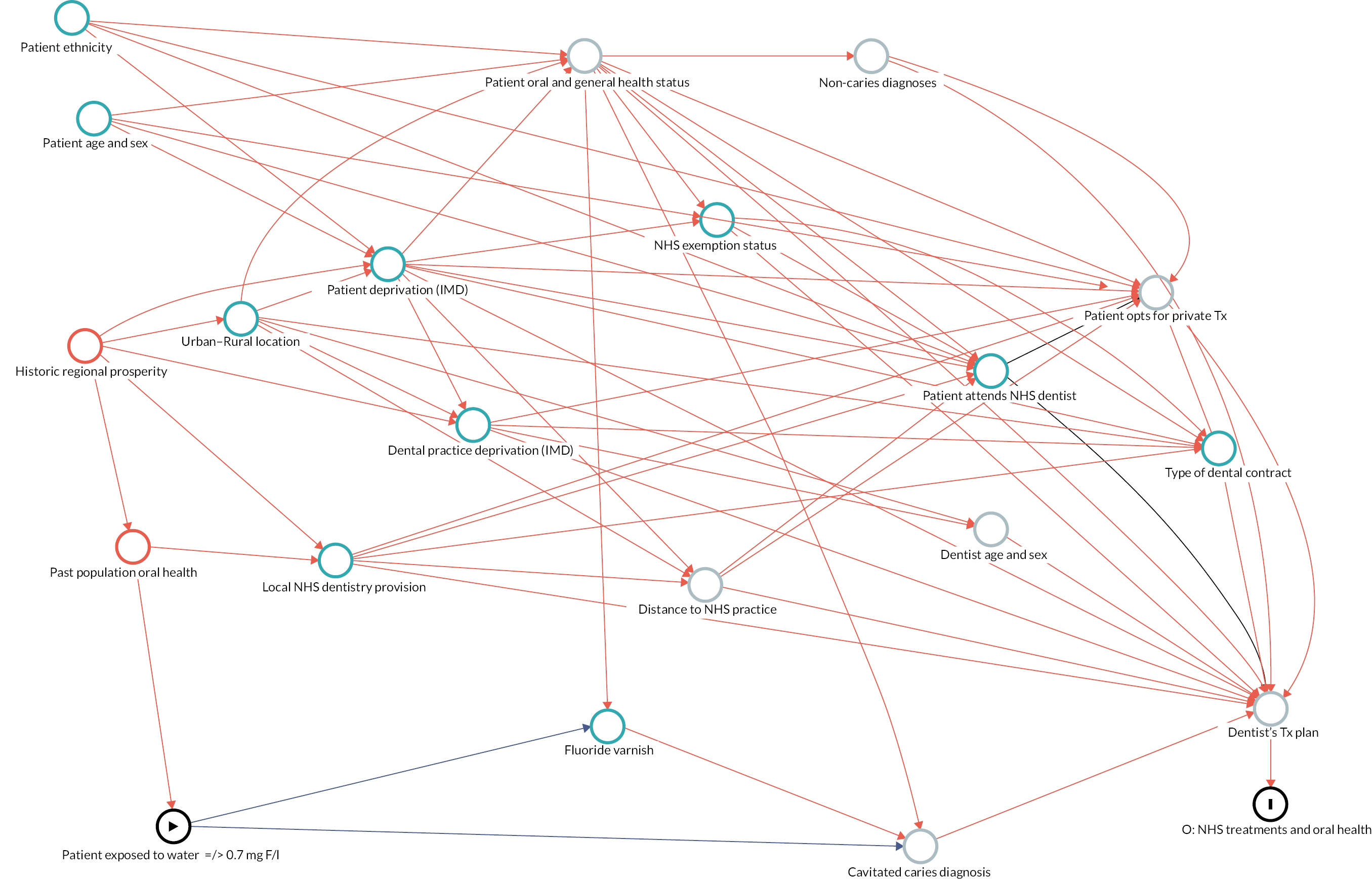
FIGURE 18.
Directed acyclic graph illustrating closure of all hypothesised confounding pathways by matching on surrogate confounders.
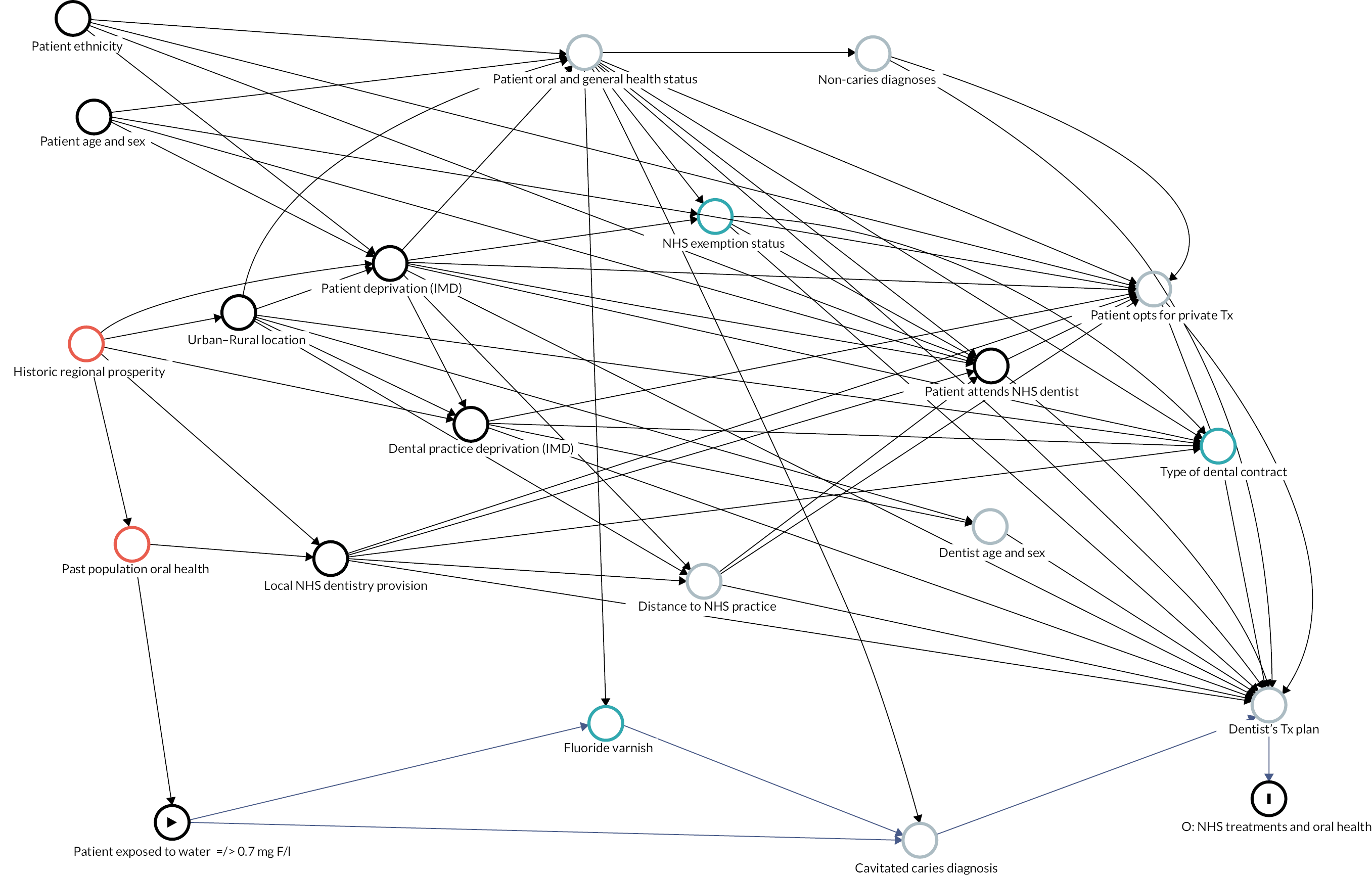
FIGURE 19.
Directed acyclic graph illustrating that potential additional matching on ancestors of the outcome (outcome predictors) that are not on the causal pathway will not introduce any collider bias.
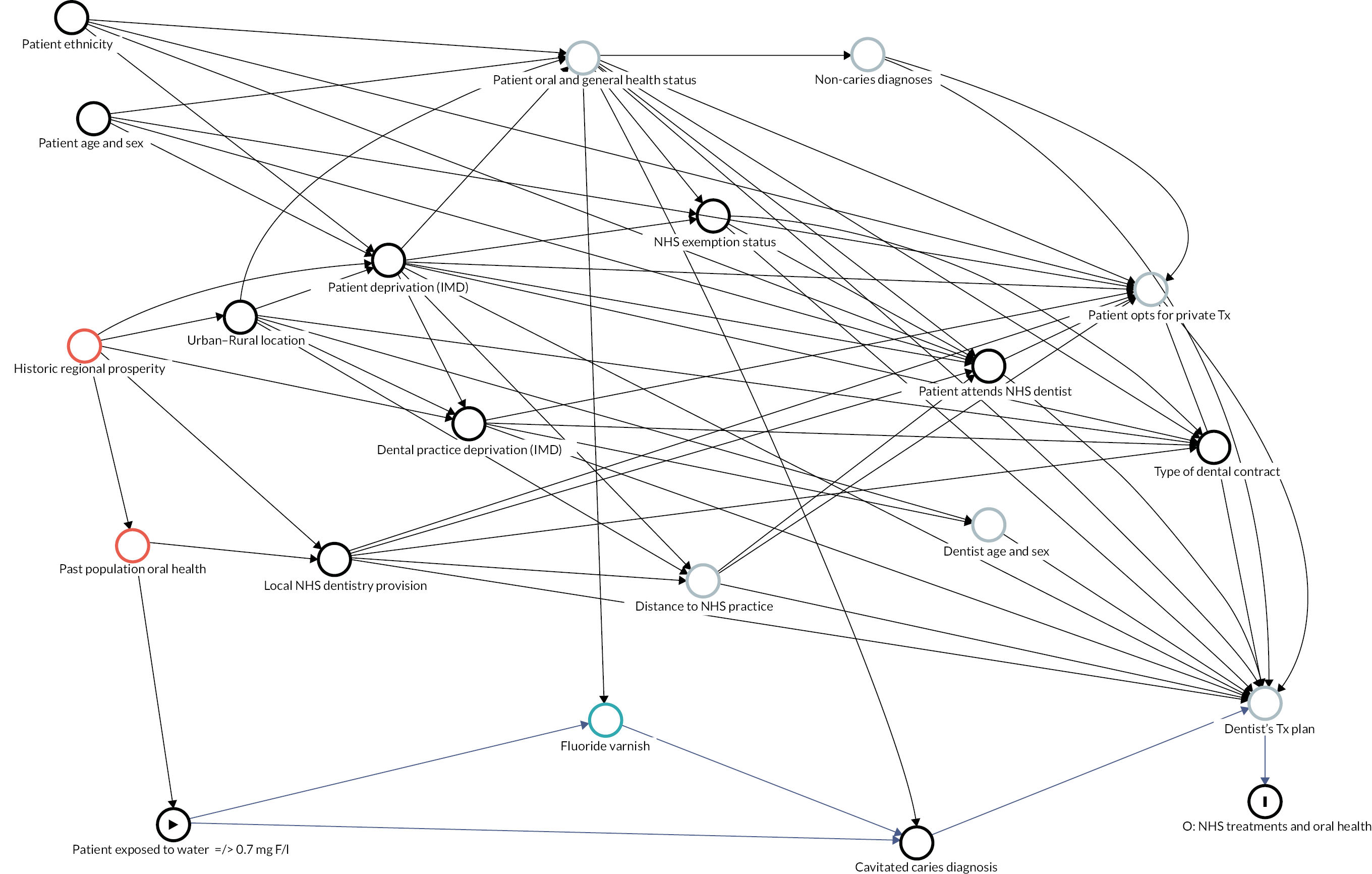
Legend Figures 16 to 21:
The study exposure variable is an oval highlighted with a black triangular ‘play’ symbol.
The study outcome variable is an oval highlighted with a vertical black line.
The causal pathway of interest (the study estimand) is shown using a dark blue arrow.
The variable ‘Patient Attends NHS Dentist’ is shown as being conditioned on (black oval) because this is an essential criterion to be included in the study data set. By design, we are conditioning on this variable through selection (restriction).
Open, biasing (confounding) pathways are illustrated in red. Ancestors of both the exposure and the outcome (also termed true confounders)115 are represented with a red oval.
Ancestors of the outcome (also called competing exposures)115 are represented with a turquoise oval.
Unmeasured and/or unmeasurable variables are shown with a grey oval.
Figure 16 shows that the only hypothesised potential true confounders (i.e. possible common cause of both the exposure and the outcome) are: population oral health before water fluoridation was introduced and long-term regional prosperity. The hypothesised mechanism by which these factors could be a true common cause of both an individual’s exposure to fluoridated water and the number of invasive dental treatments they received between 2010 and 2020 is that these factors could potentially have influenced both decisions to implement water fluoridation, and where dentists historically chose to set up dental practices in the past (before commissioning was introduced in 2006), and where practices have been commissioned (post 2006).
Figure 17 illustrates it is not possible to condition on the true confounders of population oral health or regional prosperity, because they are unmeasurable, or unmeasured, in our study. Unmeasured variables are illustrated with a grey oval. However, this is not unusual and is not a terminal problem, because it is still possible to close any potential confounding pathways by conditioning on ‘surrogate confounders’,115 more proximally located on the backdoor pathway. This will stop the transmission of any spurious association which is being caused by the true confounders.
Figure 18 shows that it is possible to close all confounding pathways by conditioning on the surrogate confounders115 of:
-
patient ethnicity
-
patient age.
-
patient sex
-
patient socioeconomic status (deprivation decile of LSOA of residence at first course of treatment)
-
urban–rural location
-
dental practice deprivation (deprivation decile of dental practice at first course of treatment)
-
local NHS dentistry provision (UDAs commissioned per person in local authority of residence at first visit).
The confounding variables that are being conditioned on (through matching) are illustrated in Figure 19 using a white oval. This set of seven variables is referred to as the ‘minimum sufficient adjustment set’. While it is possible to remove all confounding pathways through the minimal sufficient adjustment set, guidance on propensity score methods suggests that in addition to true confounders (in this case, surrogate confounders), predictors of the outcome that are not related to treatment assignment should also be included as variables in the propensity matching model. 193 This is because they reduce variance of treatment effect estimates, but they do not reduce bias. 193
However, as with traditional regression modelling approaches, when using propensity scores it is important not to include collider variables and those which are intermediates on the causal pathways between the exposure and the outcome. 194,195 Including such variables will tend to increase, rather than reduce, bias. 195 The impact of adding further potential variables to the propensity score model is illustrated in Figures 20 and 21.
FIGURE 20.
Directed acyclic graph illustrating that addition of fluoride varnish to the matching set opens previously closed backdoor pathways and that fluoride varnish is an intermediate on the causal pathway.
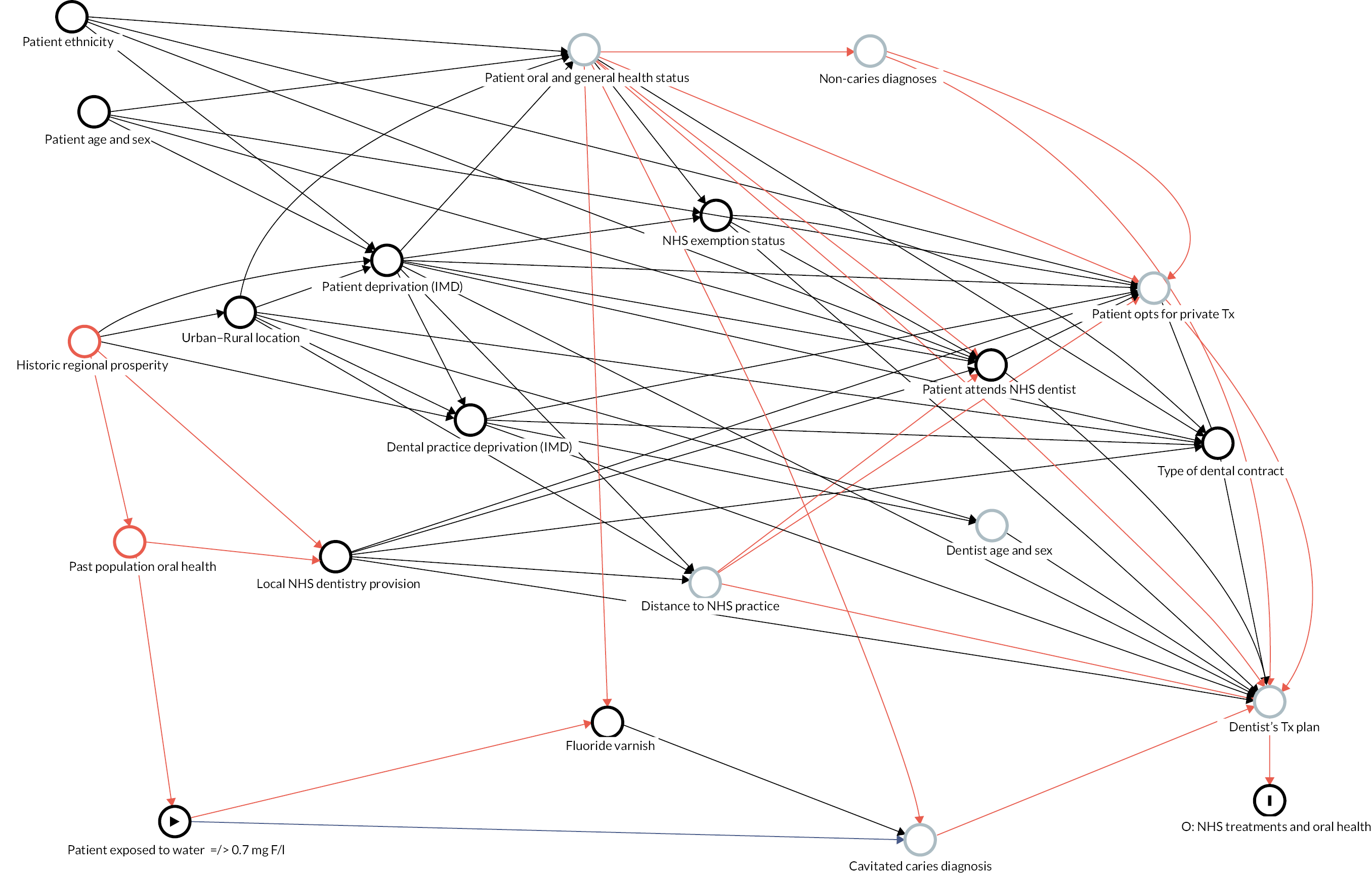
FIGURE 21.
Directed acyclic graph illustrating the final set of covariates for matching and the closure of all confounding pathways.
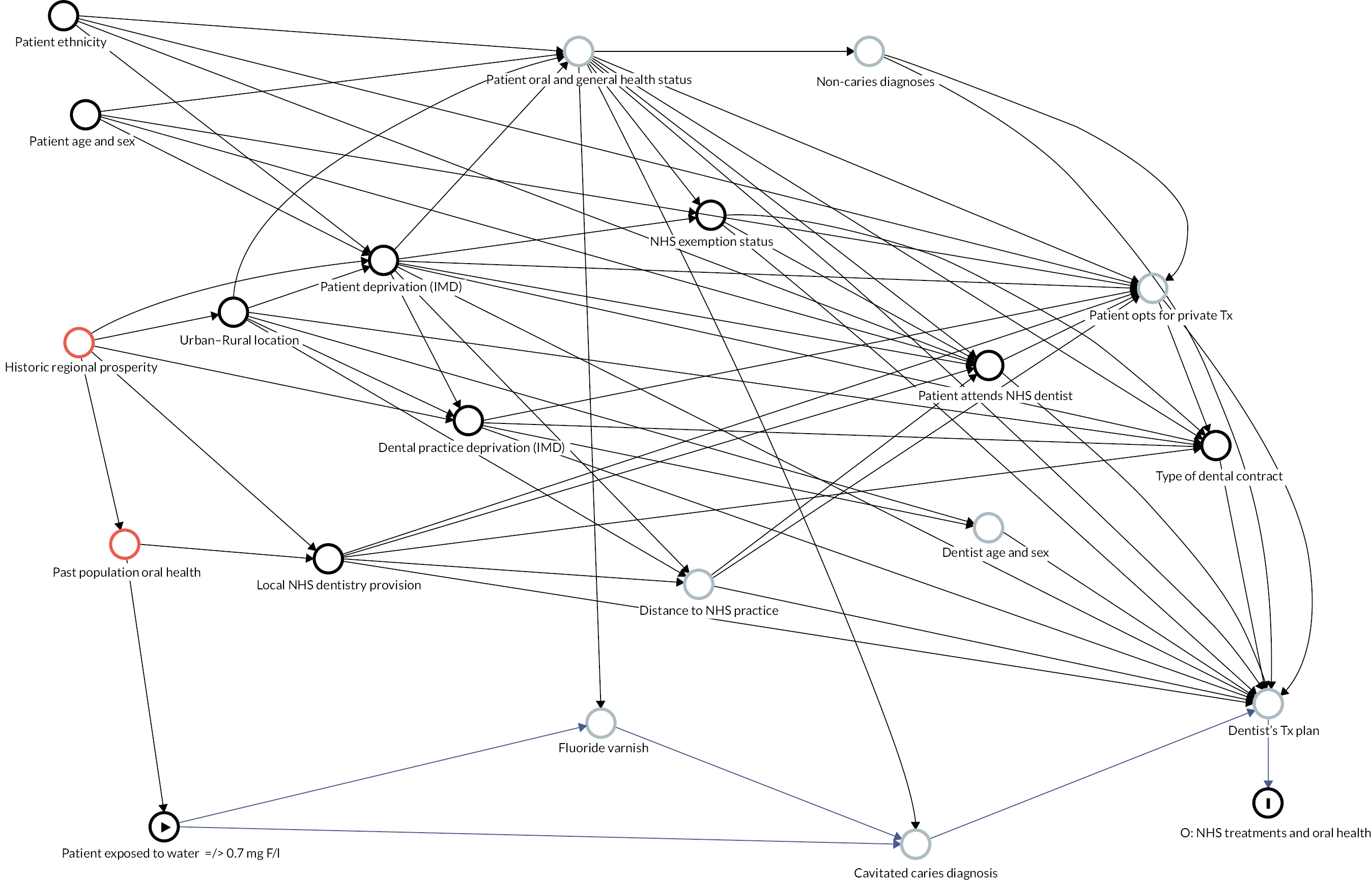
Figure 19 shows the DAG after further inclusion of the following ancestors of the outcome. 115 It confirms that including these variables in the propensity matching model as predictors of the outcome would not introduce collider bias through opening previously closed backdoor pathways and they are not on the causal pathway between exposure and outcome:
-
NHS exemption status
-
type of dental contract
-
dentist age and sex.
Figure 20 shows the further addition of ‘fluoride varnish’ to the propensity score model as a hypothesised predictor of the outcome causes problems. This is because it could also be hypothesised to be an intermediate on the causal pathway between exposure and outcome. The hypothesised role for fluoride varnish on the causal pathway is that knowledge of a patient’s fluoridation status could influence how likely a dentist is to have applied fluoride varnish for caries prevention. 27 Conditioning on fluoride varnish applications would inadvertently remove the (hypothesised) true protective effect of more frequent fluoride varnish applications for patients in sub/non-optimally fluoridated areas. Moreover, fluoride varnish is a collider variable (also receiving a causal arrow from the patient’s oral and general health status). Conditioning on it therefore inadvertently opens previously closed backdoor paths and increases bias. Whether higher rates of fluoride varnish application in sub/non-optimally fluoridated patients could explain any lack of effect of water fluoridation is an interesting question for further analysis, but it represents a different research question to that of the present study. As such it was decided that fluoride varnish should not be used in the propensity score model for this analysis.
Figure 21 presents the final DAG as used in the matching process. It illustrates that all confounding pathways have been closed by the inclusion of the matching variables. Note that dentist age and sex is not included as an outcome predictor in the final matching set for reasons outlined in Table 33.
Variable | Decision and rationale | Choice of format of variable |
---|---|---|
Patient age | Include: Caries and other oral disease such as periodontal disease are known to progress over time.148,176 The odds of receiving dental treatment increases with each increasing year of age.196 |
Match on year of age as continuous covariate |
Patient IMD | Include: Caries experience and other oral and general health conditions are strongly related to deprivation.176 Travel distance to a dental practice (accessibility) is related to deprivation.197 | Categorical based on deciles of LSOA deprivation rank (from patient postcode at first visit) Exact matching within decile |
Patient sex | Include: Sex is related to both oral health behaviours (risk and protective) and uptake of dental services.181,198 | Binary: M/F Exact matching |
Patient ethnicity | Include: Direction and effect of ethnicity on dental disease and receipt of dental treatment are not clear at present. This variable is included because an effect cannot be ruled out in light of theories regarding the underlying social mechanisms of health inequalities.14,199-201 | Categorical:
|
NHS exemption | Include: The number and types of NHS dental treatment received have been demonstrated to vary according to exemption status of the patient.202 Furthermore, income-related exemptions are a proxy measure for income. | Categorical: exemption status based on status at first visit:
|
Local NHS dentistry provision | Include: There are known variations in the volume of services commissioned by geographic region.176 These variations may reflect historical patterns in relation to deprivation and oral health. | Continuous: UDAs commissioned per person in 2015–6 by Local Authority. This is the earliest date that a measure of UDAs commissioned was available. |
We chose the above as a proxy for dental service availability rather than:
|
||
Because UDAs delivered may be more likely to be influenced by water fluoridation (e.g. if it is easier to complete courses of treatment in fluoridated areas due to lower caries burden). Dentists per 100,000 population does not take account of full-time/part-time working and different levels of NHS commitments. | ||
Urban–rural | Include: Distance from a service is related to its use and people living in urban areas are more likely to be closer to NHS dental practices.197 Demographic characteristics such as age and ethnicity are known to vary by rural–urban geography203,204 Inclusion of this variable for matching is also hypothesised to account for any potential imbalances between fluoridated and sub/non-optimally fluoridated groups in access to secondary care dental services, training for dentists, level of experience and demographic characteristics of locally available dentists (dental hospitals and schools are located in major cities). Recruitment of dentists is more difficult in rural areas. | Categorical based on patient’s LSOA at their first visit
|
Dental practice deprivation | Include: It is hypothesised that dental practices in more deprived areas will have less potential for private income and will have a higher need population. This may influence the chances of an individual patient receiving an NHS (vs. private) dental treatment and the availability of appointments. | Categorical based on deciles of LSOA deprivation rank (from dental practice LSOA at patient’s first visit). IMD 2015. |
Type of dental contract | It is hypothesised that the contracting mechanism may influence the types of treatment that are carried out and the types of patients that attend the service (e.g. PDS contracts are often used for Community Dental Services with patients with more complex treatment needs). | Three categories based on contract type at patients first visit:
|
Dentist age and sex | Not included: No available evidence to suggest an effect on treatment thresholds in the UK setting.205 Patients may see many dentists over time, so this would not be a stable factor to match on. If there are systematic differences in dentist composition by area, it is hypothesised that this would be accounted for by matching patients on dental practice deprivation decile and urban: rural classification at baseline (e.g. older more experienced dentists in rural areas, newer graduates in cities closer to dental schools). | |
Receipt of fluoride varnish | Not included: It is hypothesised that fluoride varnish is an intermediate on the causal pathway between water fluoridation and dental caries/invasive treatment. Including it as a matching variable would remove the hypothesised protective effect of more frequent fluoride varnish application in sub/non-optimally fluoridated areas, and it is also a collider variable, receiving arrows from both patient water fluoridation exposure status and patient oral and general health status. Matching on it would open previously closed backdoor paths and increases bias. |
A summary of the hypothesised reasons for inclusion or exclusion of the variables assessed as potential matching variables is presented in Table 33.
Variable (subconcepts) | Connects to (forwards, left to right) | Doesn’t connect to (forwards, left to right) | Rationale for no direct connection |
---|---|---|---|
Patient ethnicity |
|
|
|
Patient age and sex |
|
|
|
Historic regional prosperity |
|
|
|
Past population oral health (before water fluoridation) |
|
No other plausible connections |
|
Urban–rural location |
|
|
|
Patient deprivation (IMD 2015) |
|
|
|
Dental practice deprivation (IMD 2015) |
|
|
|
Local NHS dentistry provision (Proxy: UDAs commissioned per person by Local Authority in 2015) |
|
|
|
Exposure (> 0.7 mg F/l) |
|
No other plausible connections | |
Patient O&G Health Status [Health behaviours (diet, smoking etc.) risks and health status] |
|
|
|
Fluoride varnish (as a preventive intervention, in the past, prior to the current treatment plan) |
|
|
|
NHS exemption status |
|
|
|
Distance to practice |
|
No other plausible connections | |
Non-caries diagnosis (Trauma, orthodontic need, periodontal disease) |
|
No other plausible connections | |
Cavitated caries diagnosis |
|
No other plausible connections | |
Patient attends NHS dentist (and has NHS number) |
|
|
|
Type of dental contract (PDS/GDS/PDS-Plus) |
|
|
|
Dentist’s age and sex |
|
No other plausible connections | |
Pt opts for private Tx |
|
|
|
Dentist’s Tx plan |
|
No other plausible connections |
Appendix 3 Full list of variables supplied by National Health Service Business Service Authority
Patient demographic data
-
Age at treatment acceptance date
-
ID number
-
Postcode (subsector level)
-
Gender
-
Ethnicity
-
IMD at home address at each course of treatment
-
LSOA at home address at each course of treatment
-
National Health Service exemption category at each course of treatment
Patient clinical treatment data
-
Treatment start and end dates
-
Examination
-
Fluoride varnish
-
Endodontic treatment (including number of teeth)
-
Permanent fillings and sealant restorations (including number of teeth)
-
Extractions (including number of teeth)
-
Crowns provided (including number of teeth)
-
Veneers applied (including number of teeth)
-
Inlays (including number of teeth)
Patient oral health data
-
Number of decayed teeth permanent
-
Number of missing teeth permanent
-
Number of filled teeth permanent
Health economic information
-
Treatment band
-
Patient charge amount (£)
-
Patient charge collected (£)
Dental practice information
-
Pseudonymised contract number
-
Annual activity (number of UDAs commissioned) – from 1 April 2014
-
Contract value (total financial value of contract) – from 1 April 2014
-
Type of contract (PDS/GDS)
-
Practice LSOA name
-
IMD of practice LSOA
Data provided by NHS BSA but not utilised during this analysis
-
Dentist age at treatment acceptance date
-
Dentist gender
-
Dentist place of qualification
-
Dentist year of registration
-
Performer ID of the dentist (pseudonymised)
-
Population per dentist, per year (CCG level)
-
Arrest bleed count
-
Scale and polish (item 1)
-
Radiographs taken (including number)
-
Upper denture – acrylic (item 9, including number of teeth)
-
Lower denture – acrylic (item A, including number of teeth)
-
Upper denture – metal (item B, including number of teeth)
-
Lower denture – metal (item C, including number of teeth)
-
Bridges fitted (item F, including number of teeth)
-
Antibiotic items prescribed (item J, including number of items)
-
Referral for advanced mandatory services (item G, including band)
-
Other treatment (item K)
-
Best practice prevention according to delivering better oral health offered (item L)
-
Advanced mandatory services/count forms with that treatment present
-
Bridge repair
-
Denture repair
-
‘Urgent’ box ticked
-
Suture removed
-
Count of forms with a Dental Regulation 11 (REG 11) Replacement
-
Fissure sealants (including number of teeth)
-
Unique patients seen (on contract) per 12 and 24 months – from 1 April 2016
-
Radiographs taken per 100 courses of treatment (on contract) – for full financial years (i.e. April 2011–April 2020 only)
-
Local authority name
-
Dentist status: foundation dentists/performer/provider
-
Years only (i.e. from 1 April 2011 to 31 March 2020)
-
Number of days since last visit to any dental contract
-
NICE recall interval
-
Treatment complete or incomplete
-
Duration of treatment (time between acceptance date and treatment end date)
-
Practice PCT and CCG code
Appendix 4 Additional information on propensity score matches not taken forwards for analysis
This section presents summary statistics on the balance between fluoridated and sub/non-optimally fluoridated groups for the unmatched sample, and matching using methods 1 and 3.
Method 0: No matching
Standardised mean difference | SMD |
---|---|
distance | 0.6595 |
nhs_exemption_category_Not exempt | −0.0956 |
nhs_exemption_category_Income-related exemption | 0.1112 |
nhs_exemption_category_Non-income-related exemption | −0.0221 |
patient_gender_F | 0.0026 |
patient_gender_M | −0.0026 |
age_2011 | 0.0248 |
ethnicity_cat_White | −0.0417 |
ethnicity_cat_Mixed/Multiple ethnic group | −0.0061 |
ethnicity_cat_Asian/Asian British | 0.1121 |
ethnicity_cat_Black/African/Caribbean/Black British | 0.0157 |
ethnicity_cat_Other ethnic group | −0.0334 |
ethnicity_cat_not reported | −0.0165 |
practice_imd_decile_2015_1 | 0.1004 |
practice_imd_decile_2015_2 | 0.1821 |
practice_imd_decile_2015_3 | 0.0281 |
practice_imd_decile_2015_4 | −0.0115 |
practice_imd_decile_2015_5 | −0.0366 |
practice_imd_decile_2015_6 | −0.019 |
practice_imd_decile_2015_7 | −0.0318 |
practice_imd_decile_2015_8 | −0.1636 |
practice_imd_decile_2015_9 | −0.0947 |
practice_imd_decile_2015_10 | −0.0711 |
patient_deprivation_decile_1 | 0.2055 |
patient_deprivation_decile_2 | 0.1365 |
patient_deprivation_decile_3 | 0.0103 |
patient_deprivation_decile_4 | −0.0564 |
patient_deprivation_decile_5 | −0.0472 |
patient_deprivation_decile_6 | −0.0778 |
patient_deprivation_decile_7 | −0.0624 |
patient_deprivation_decile_8 | −0.0606 |
patient_deprivation_decile_9 | −0.0655 |
patient_deprivation_decile_10 | −0.0973 |
urban_rural_classification_2011_Urban major conurbation | 0.5946 |
urban_rural_classification_2011_Urban minor conurbation | −3.6182 |
urban_rural_classification_2011_Urban city and town | −0.443 |
urban_rural_classification_2011_Urban city and town in a sparse setting | −0.7152 |
urban_rural_classification_2011_Rural town and fringe | −0.0575 |
urban_rural_classification_2011_Rural town and fringe in a sparse setting | −0.0538 |
urban_rural_classification_2011_Rural village and dispersed | −0.1279 |
urban_rural_classification_2011_Rural village and dispersed in a sparse setting | −0.0683 |
udas_commissioned_per_population | 0.134 |
contract_type_GDS | 0.0779 |
contract_type_PDS | −0.0909 |
contract_type_PDS Plus | 0.0342 |
Variance ratio | VR |
distance | 1.609 |
age_2011 | 0.9944 |
udas_commissioned_per_population | 1.1611 |
Method 1: Nearest neighbour, one to one matching
Standardised mean difference | SMD | Per cent balance improvement in SMD |
---|---|---|
distance | 0.0006 | 99.9 |
nhs_exemption_category_Not exempt | 0.0625 | 34.6 |
nhs_exemption_category_Income-related exemption | −0.0468 | 57.9 |
nhs_exemption_category_Non-income-related exemption | −0.0389 | −75.7 |
patient_gender_F | 0 | 100 |
patient_gender_M | 0 | 100 |
age_2011 | −0.0173 | 30.4 |
ethnicity_cat_White | 0.0645 | −54.7 |
ethnicity_cat_Mixed/Multiple ethnic group | −0.0283 | −364.5 |
ethnicity_cat_Asian/Asian British | −0.0437 | 61 |
ethnicity_cat_Black/African/Caribbean/Black British | 0.0007 | 95.4 |
ethnicity_cat_Other ethnic group | −0.0263 | 21.3 |
ethnicity_cat_not reported | −0.0335 | −103.3 |
practice_imd_decile_2015_1 | 0.0286 | 71.5 |
practice_imd_decile_2015_2 | 0.0044 | 97.6 |
practice_imd_decile_2015_3 | 0.0047 | 83.5 |
practice_imd_decile_2015_4 | −0.0258 | −124 |
practice_imd_decile_2015_5 | −0.0238 | 35 |
practice_imd_decile_2015_6 | 0.002 | 89.5 |
practice_imd_decile_2015_7 | −0.0214 | 32.8 |
practice_imd_decile_2015_8 | 0.0284 | 82.7 |
practice_imd_decile_2015_9 | −0.0247 | 73.9 |
practice_imd_decile_2015_10 | 0.0096 | 86.4 |
patient_deprivation_decile_1 | 0 | 100 |
patient_deprivation_decile_2 | 0 | 100 |
patient_deprivation_decile_3 | 0 | 100 |
patient_deprivation_decile_4 | 0 | 100 |
patient_deprivation_decile_5 | 0 | 100 |
patient_deprivation_decile_6 | 0 | 100 |
patient_deprivation_decile_7 | 0 | 100 |
patient_deprivation_decile_8 | 0 | 100 |
patient_deprivation_decile_9 | 0 | 100 |
patient_deprivation_decile_10 | 0 | 100 |
urban_rural_classification_2011_Urban major conurbation | −0.0086 | 98.5 |
urban_rural_classification_2011_Urban minor conurbation | −0.0003 | 100 |
urban_rural_classification_2011_Urban city and town | 0.0306 | 93.1 |
urban_rural_classification_2011_Urban city and town in a sparse setting | 0.001 | 99.9 |
urban_rural_classification_2011_Rural town and fringe | −0.0066 | 88.4 |
urban_rural_classification_2011_Rural town and fringe in a sparse setting | −0.0132 | 75.4 |
urban_rural_classification_2011_Rural village and dispersed | −0.0307 | 76 |
urban_rural_classification_2011_Rural village and dispersed in a sparse setting | −0.0126 | 81.5 |
udas_commissioned_per_population | 0.0121 | 90.9 |
contract_type_GDS | 0.0428 | 45 |
contract_type_PDS | −0.0416 | 54.3 |
contract_type_PDS Plus | −0.0098 | 71.3 |
Variance ratio | VR | Per cent balance improvement in VR |
distance | 1.0032 | 99.3 |
age_2011 | 0.9666 | −505.5 |
udas_commissioned_per_population | 1.1899 | −16.4 |
Method 3: Nearest neighbour, variable ratio matching (ratio = 3, maximum = 5), with calliper
Standardised mean difference | SMD | Per cent balance improvement in SMD |
---|---|---|
distance | 0.0003 | 99.9 |
nhs_exemption_category_Not exempt | 0.0359 | 62.5 |
nhs_exemption_category_Income-related exemption | −0.0255 | 77.1 |
nhs_exemption_category_Non-income-related exemption | −0.0252 | −14.1 |
patient_gender_F | 0 | 100 |
patient_gender_M | 0 | 100 |
age_2011 | −0.0197 | 20.9 |
ethnicity_cat_White | 0.0468 | −12.3 |
ethnicity_cat_Mixed/Multiple ethnic group | −0.0157 | −157.6 |
ethnicity_cat_Asian/Asian British | −0.0381 | 66 |
ethnicity_cat_Black/African/Caribbean/Black British | −0.0006 | 96.4 |
ethnicity_cat_Other ethnic group | −0.017 | 49.1 |
ethnicity_cat_not reported | −0.0218 | −32.3 |
practice_imd_decile_2015_1 | 0.0254 | 74.7 |
practice_imd_decile_2015_2 | 0.0002 | 99.9 |
practice_imd_decile_2015_3 | 0.0049 | 82.6 |
practice_imd_decile_2015_4 | −0.0194 | −68 |
practice_imd_decile_2015_5 | −0.0109 | 70.3 |
practice_imd_decile_2015_6 | 0.0017 | 91.1 |
practice_imd_decile_2015_7 | −0.0168 | 47.3 |
practice_imd_decile_2015_8 | 0.0131 | 92 |
practice_imd_decile_2015_9 | −0.0145 | 84.7 |
practice_imd_decile_2015_10 | 0.0037 | 94.8 |
patient_deprivation_decile_1 | 0 | 100 |
patient_deprivation_decile_2 | 0 | 100 |
patient_deprivation_decile_3 | 0 | 100 |
patient_deprivation_decile_4 | 0 | 100 |
patient_deprivation_decile_5 | 0 | 100 |
patient_deprivation_decile_6 | 0 | 100 |
patient_deprivation_decile_7 | 0 | 100 |
patient_deprivation_decile_8 | 0 | 100 |
patient_deprivation_decile_9 | 0 | 100 |
patient_deprivation_decile_10 | 0 | 100 |
urban_rural_classification_2011_Urban major conurbation | −0.0106 | 98.2 |
urban_rural_classification_2011_Urban minor conurbation | −0.0005 | 100 |
urban_rural_classification_2011_Urban city and town | 0.0126 | 97.2 |
urban_rural_classification_2011_Urban city and town in a sparse setting | 0.0007 | 99.9 |
urban_rural_classification_2011_Rural town and fringe | 0.0152 | 73.6 |
urban_rural_classification_2011_Rural town and fringe in a sparse setting | −0.006 | 88.8 |
urban_rural_classification_2011_Rural village and dispersed | −0.0169 | 86.8 |
urban_rural_classification_2011_Rural village and dispersed in a sparse setting | −0.0151 | 77.9 |
udas_commissioned_per_population | 0.0109 | 91.9 |
contract_type_GDS | 0.0319 | 59.1 |
contract_type_PDS | −0.0302 | 66.8 |
contract_type_PDS Plus | −0.01 | 70.9 |
Variance ratio | VR | Per cent balance improvement in VR |
distance | 1.002 | 99.6 |
age_2011 | 0.9734 | −380.7 |
udas_commissioned_per_population | 1.2354 | −41.5 |
Appendix 5 Additional charts for assessing the balance of the selected matched cohort (matching method 2)
FIGURE 22.
Distribution of propensity scores for the unmatched cohort, illustrating common support of the propensity scores between fluoridated and sub/non-optimally fluoridated groups.
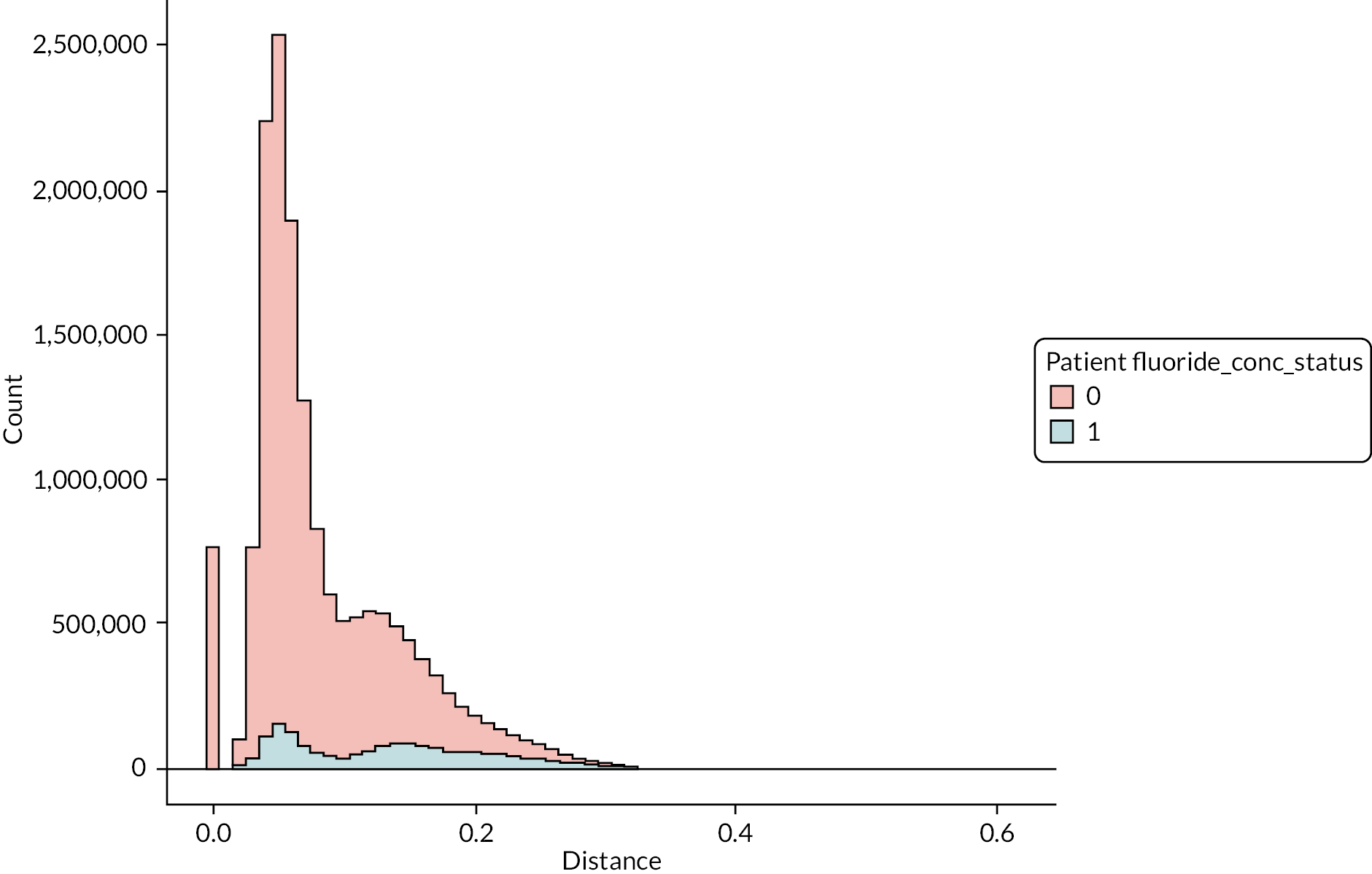
FIGURE 23.
Distribution of propensity scores for the matched cohort (matching method 2).
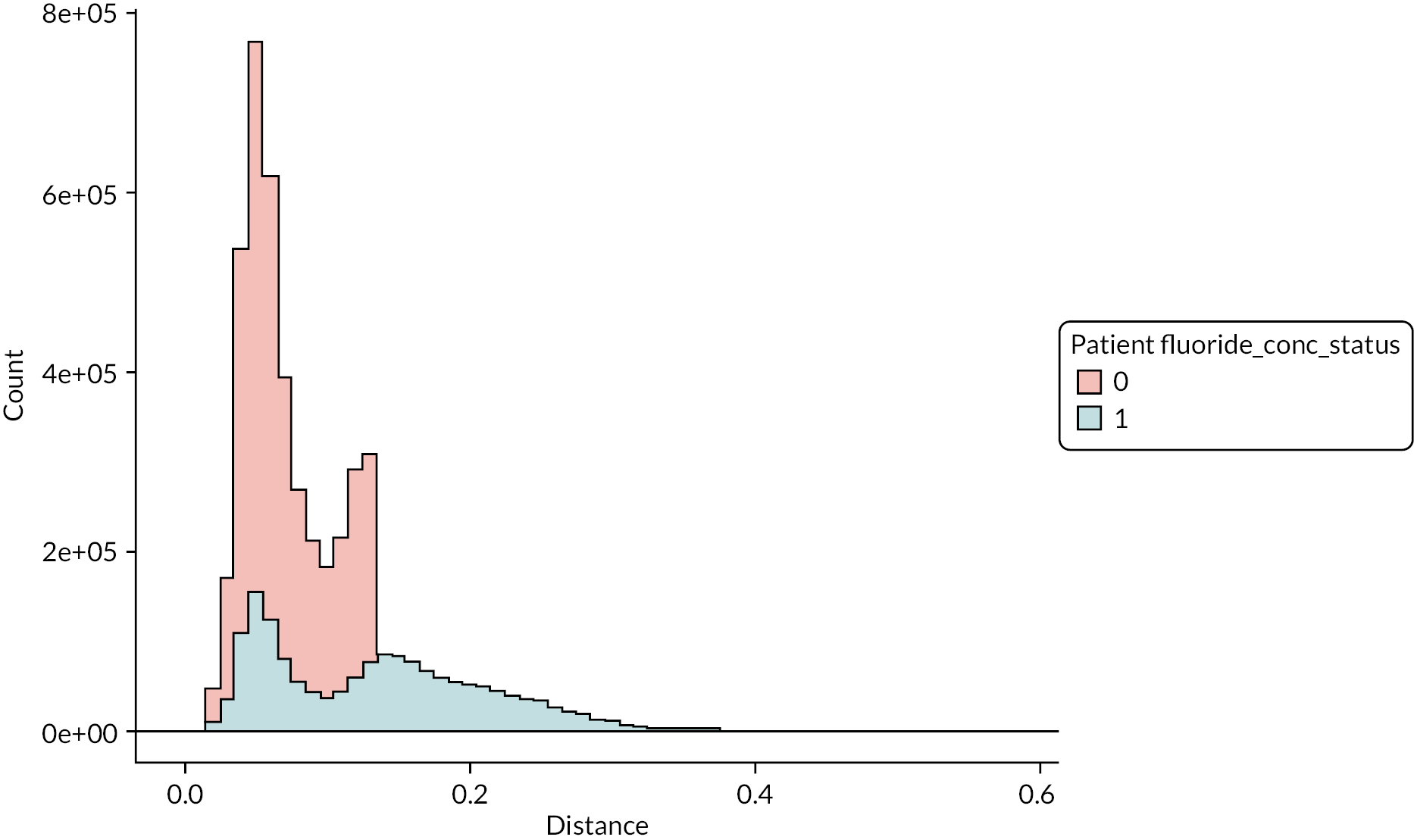
FIGURE 24.
Empirical quantile–quantile plots for age and UDAs commissioned per person for the matched cohort (matching method 2).
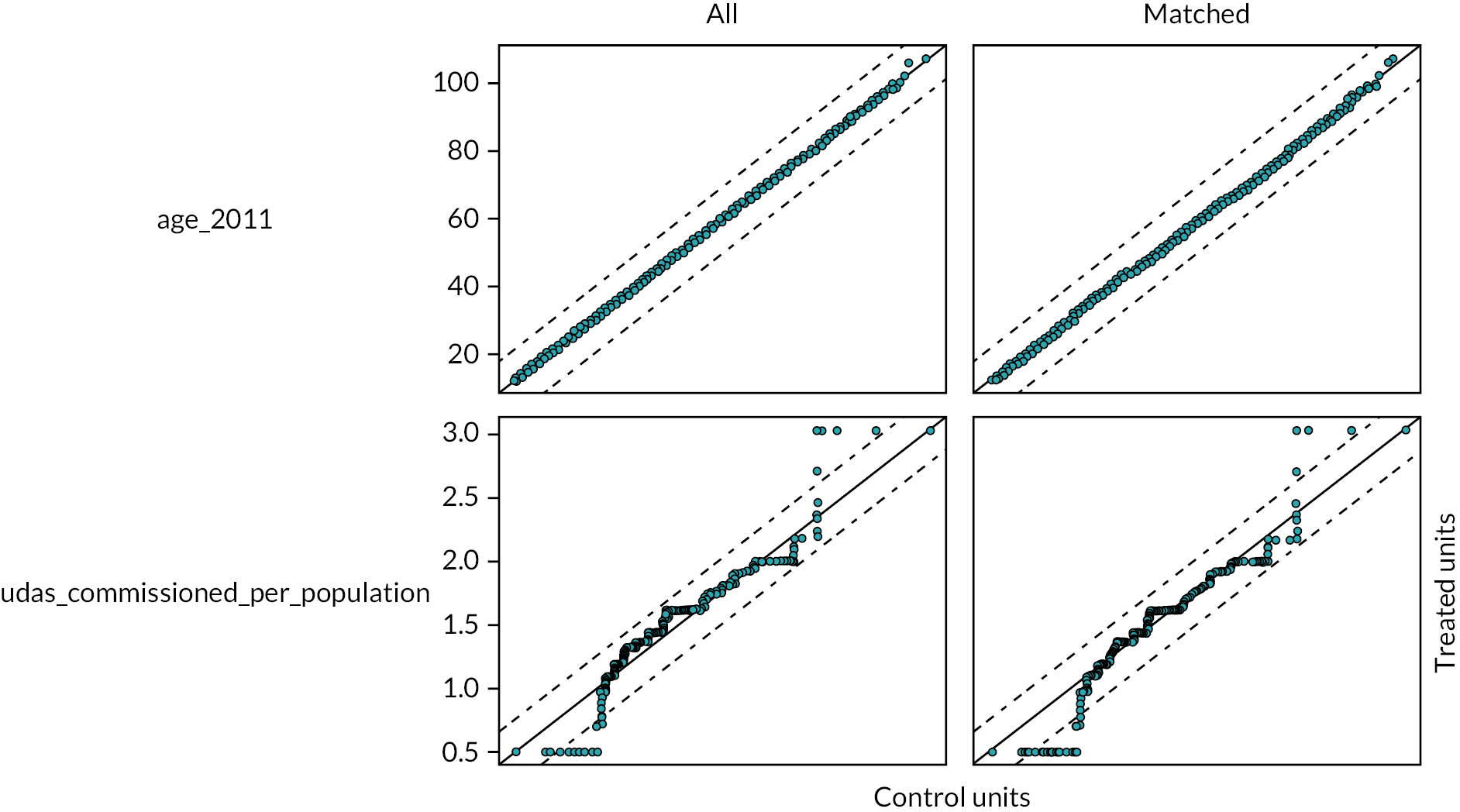
FIGURE 25.
Kernel density plot for age in the selected matched cohort (matching method 2).
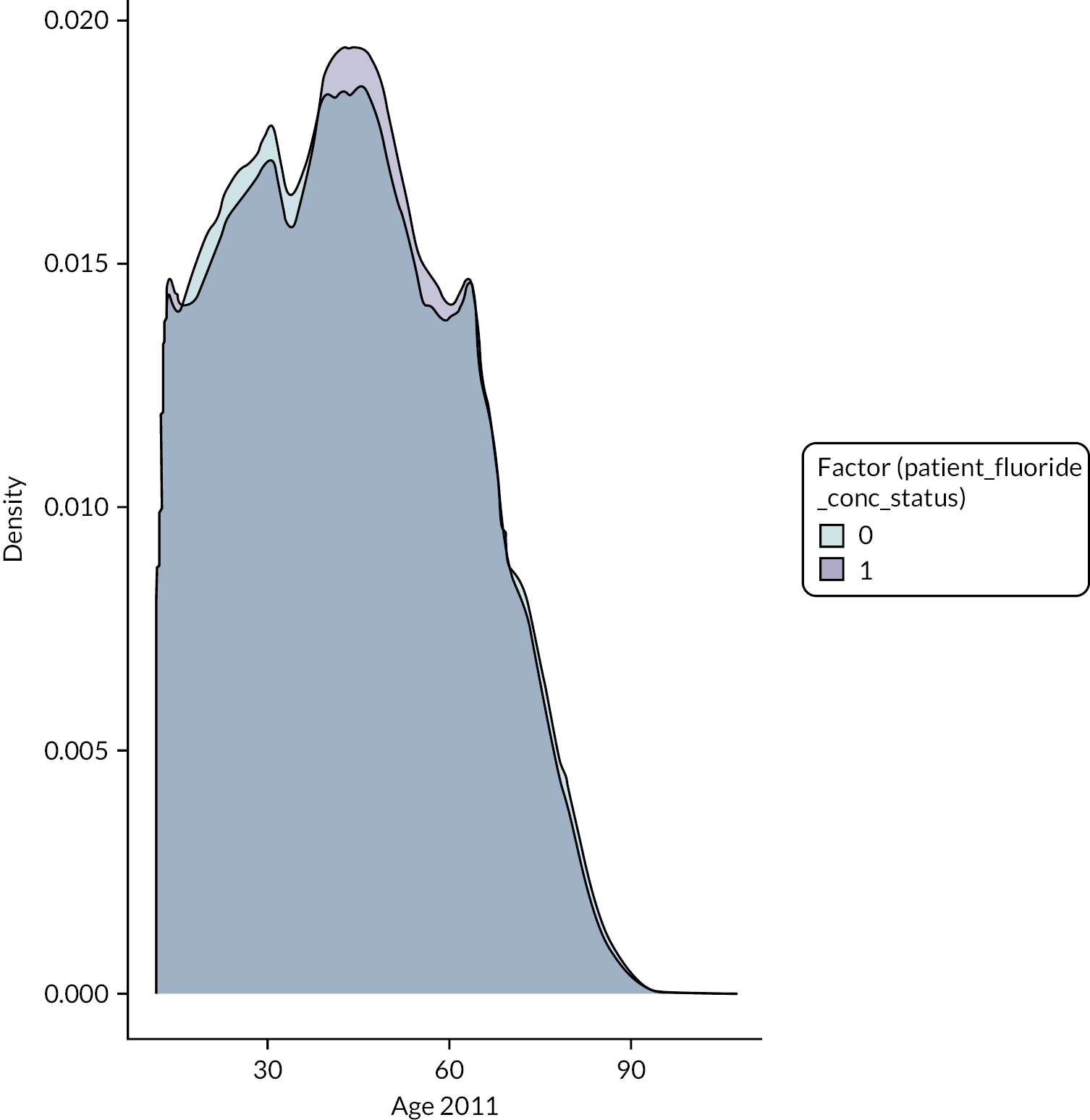
FIGURE 26.
Kernel density plot of the number of UDAs commissioned per person (per population) in the selected matched cohort (matching method 2).
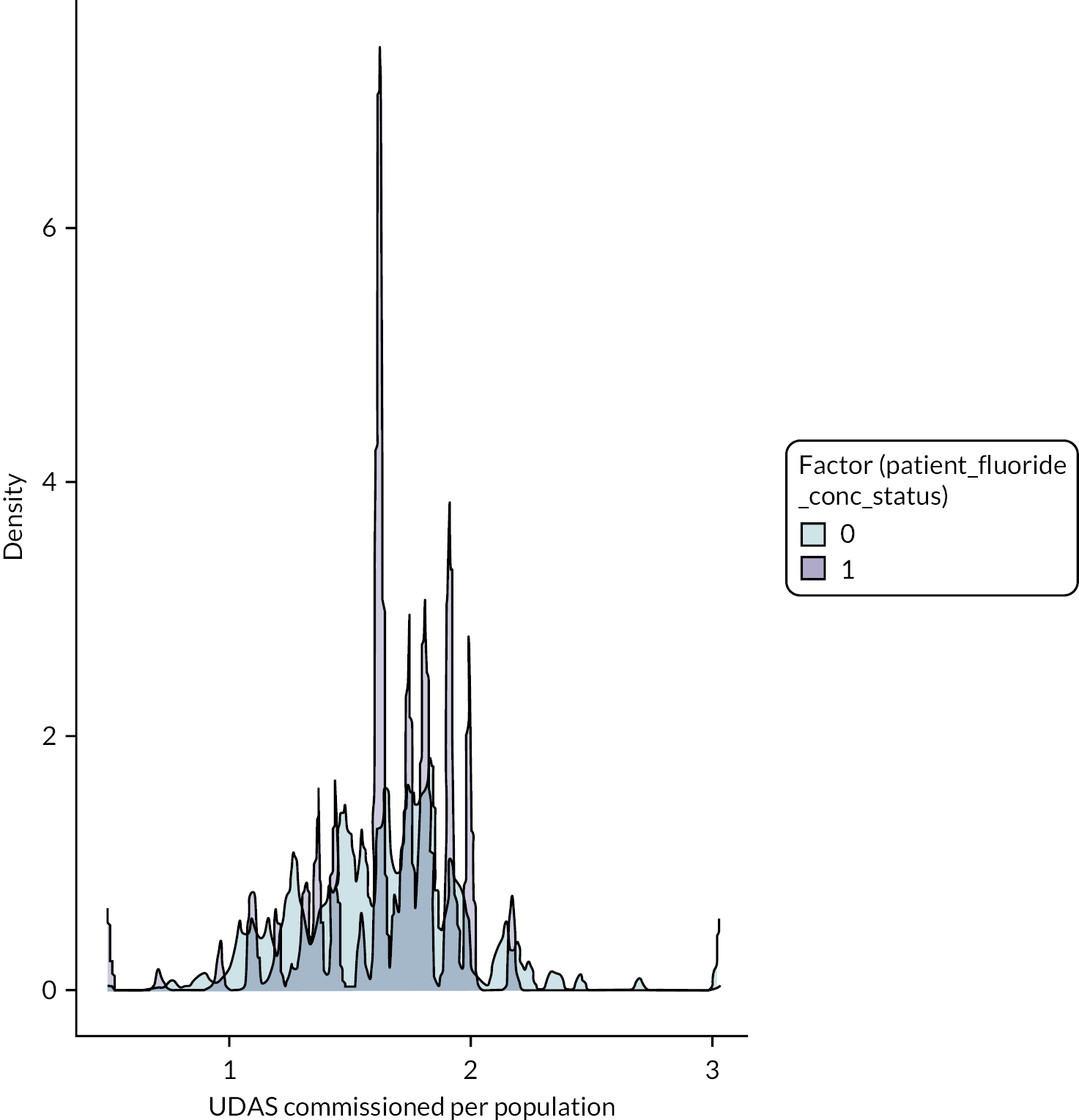
FIGURE 27.
Distributional balance for dental practice contract type in the selected matched cohort (matching method 2).
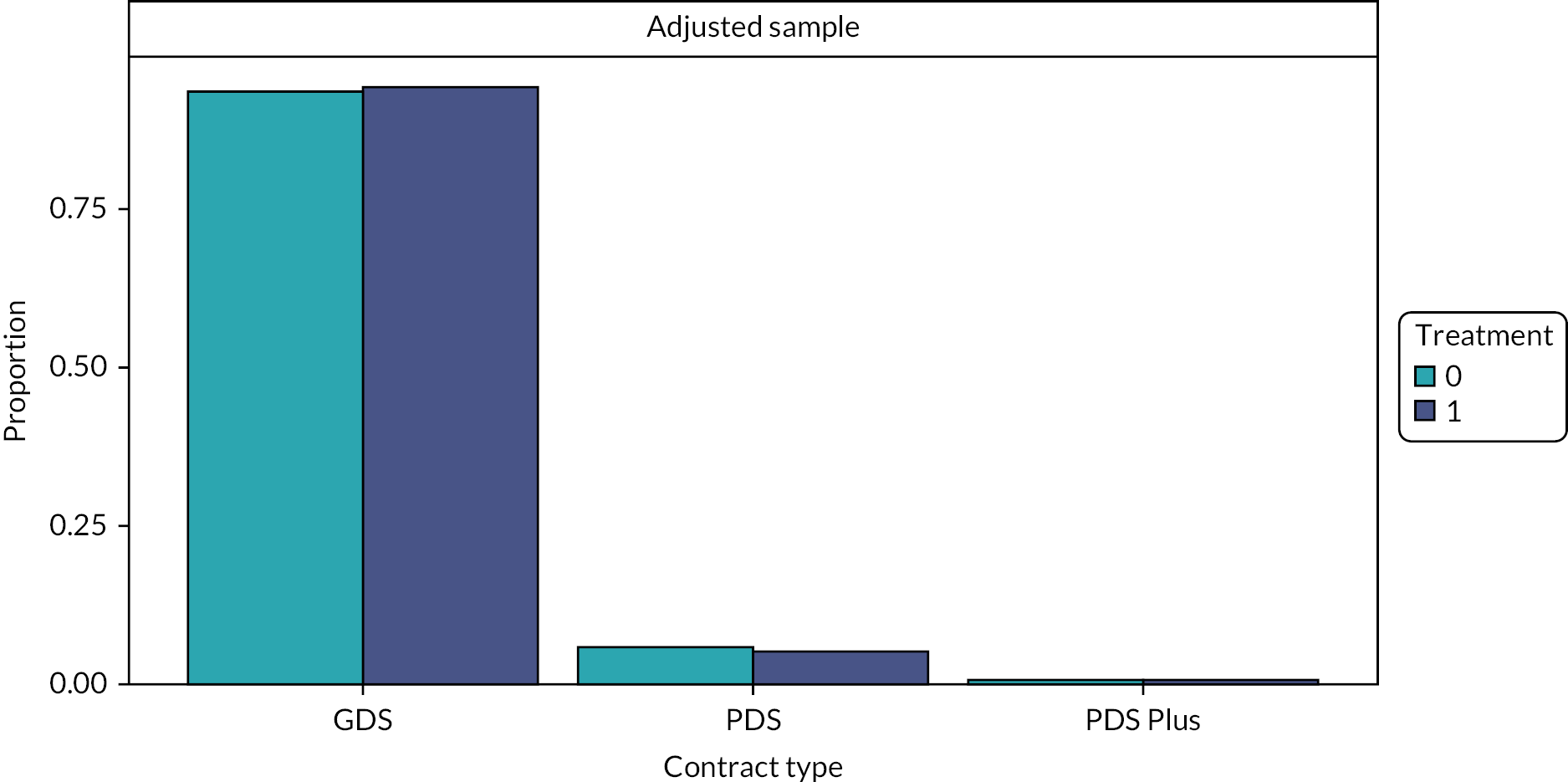
FIGURE 28.
Distributional balance for ethnicity category in the selected matched cohort (matching method 2).
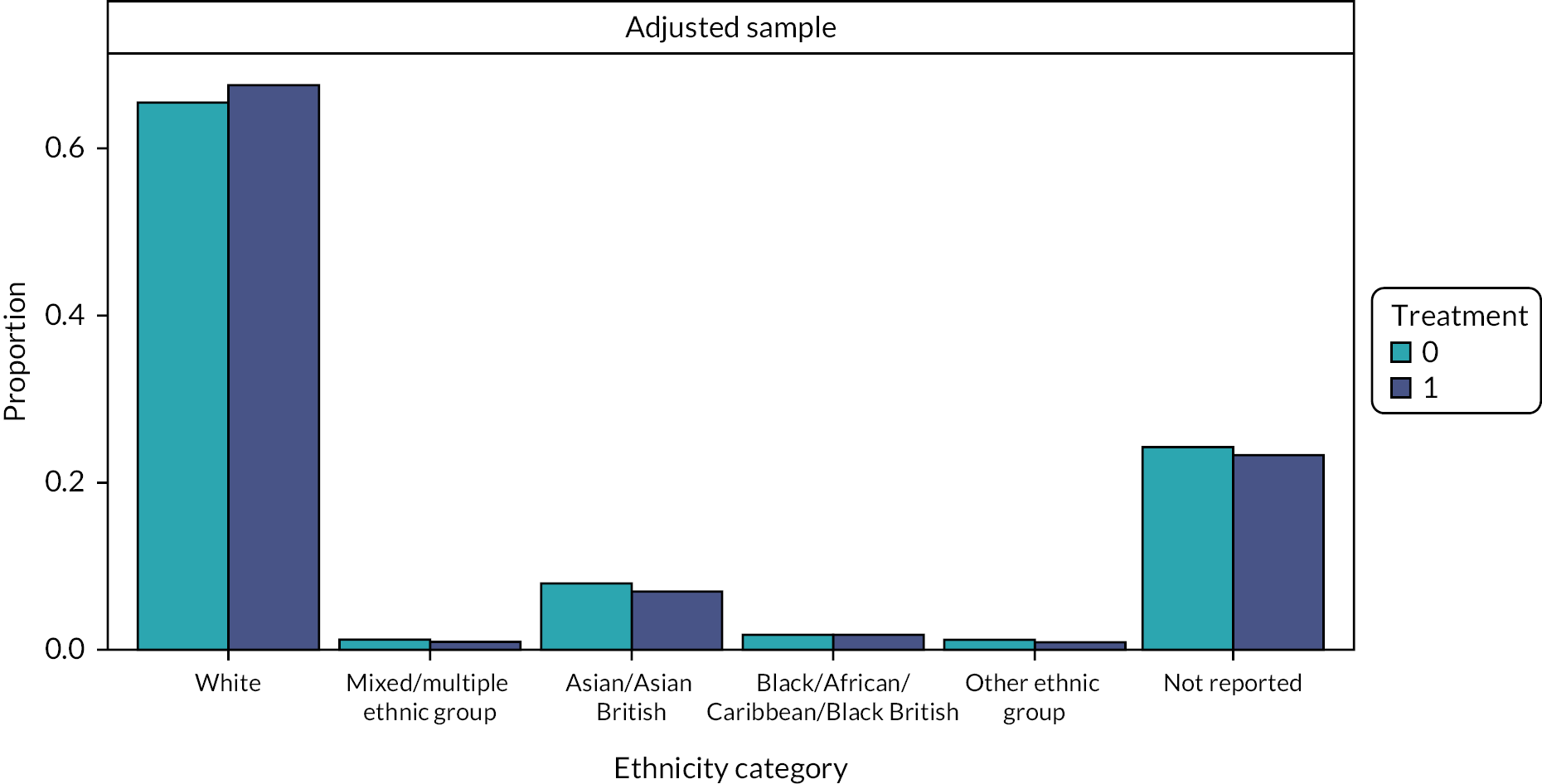
FIGURE 29.
Distributional balance for NHS exemption category in the selected matched cohort (matching method 2).
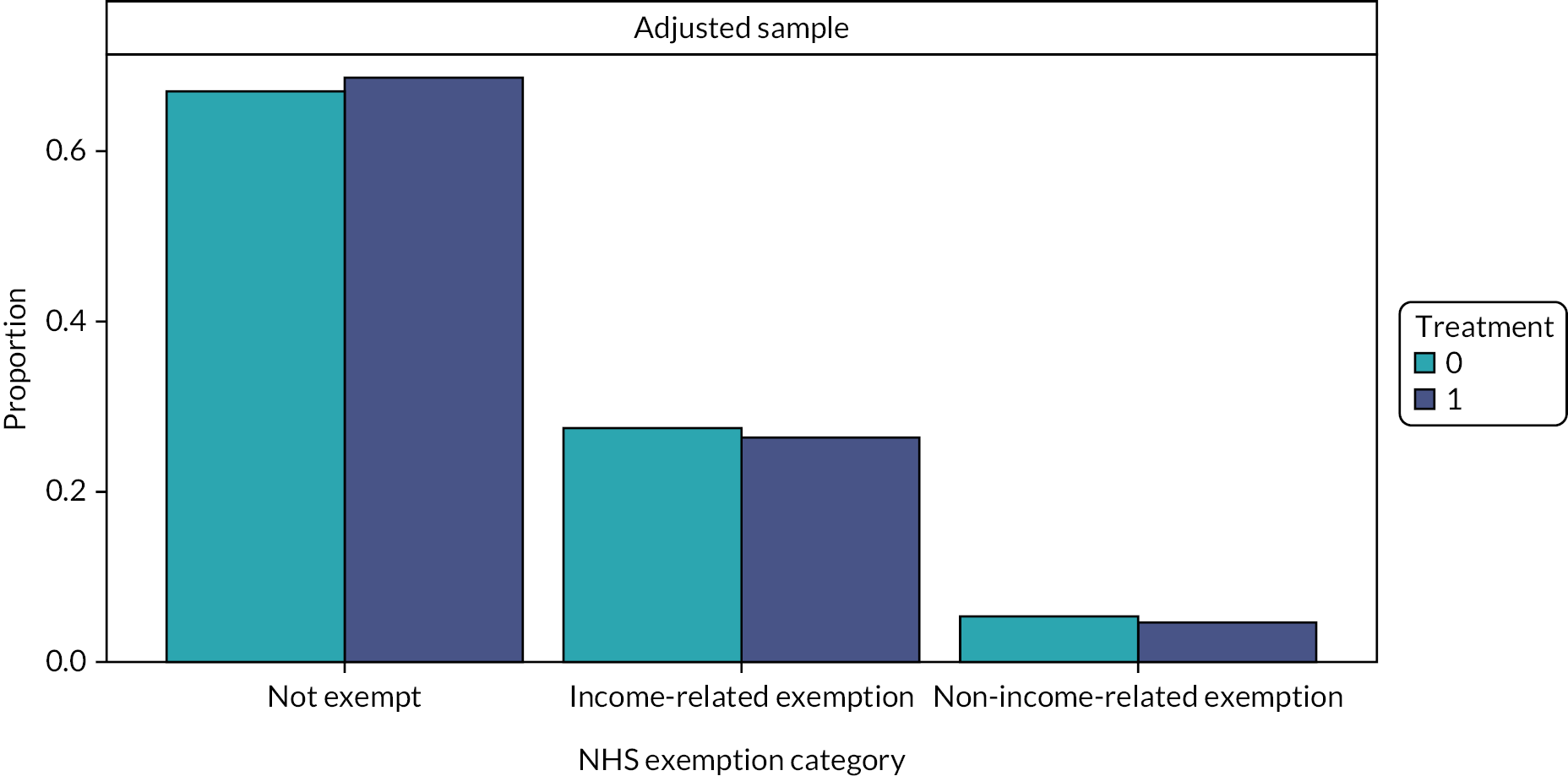
FIGURE 30.
Distributional balance for patient sex (gender) for the selected matched cohort (matching method 2).
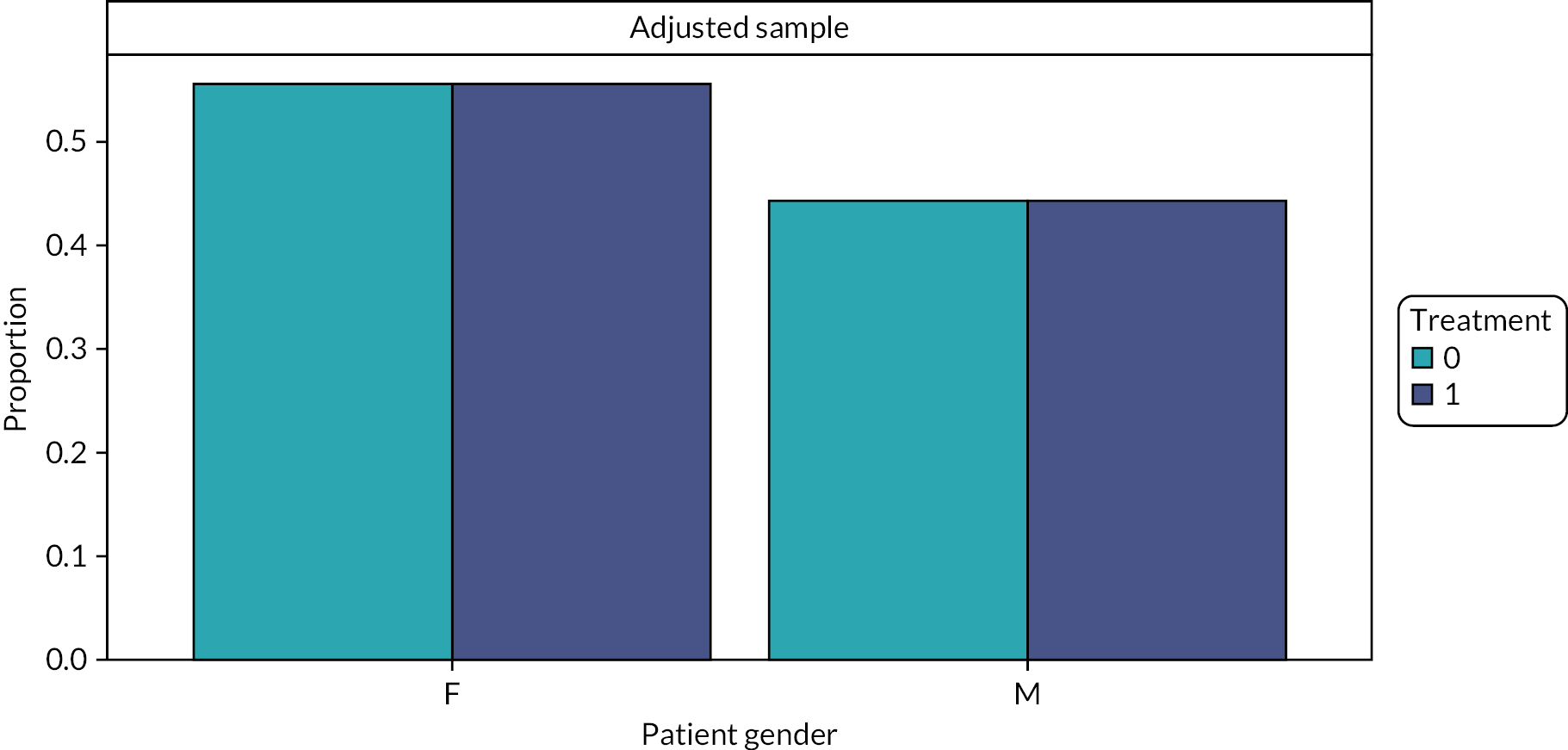
FIGURE 31.
Distributional balance for patient deprivation (IMD 2015) in the selected matched cohort (matching method 2).
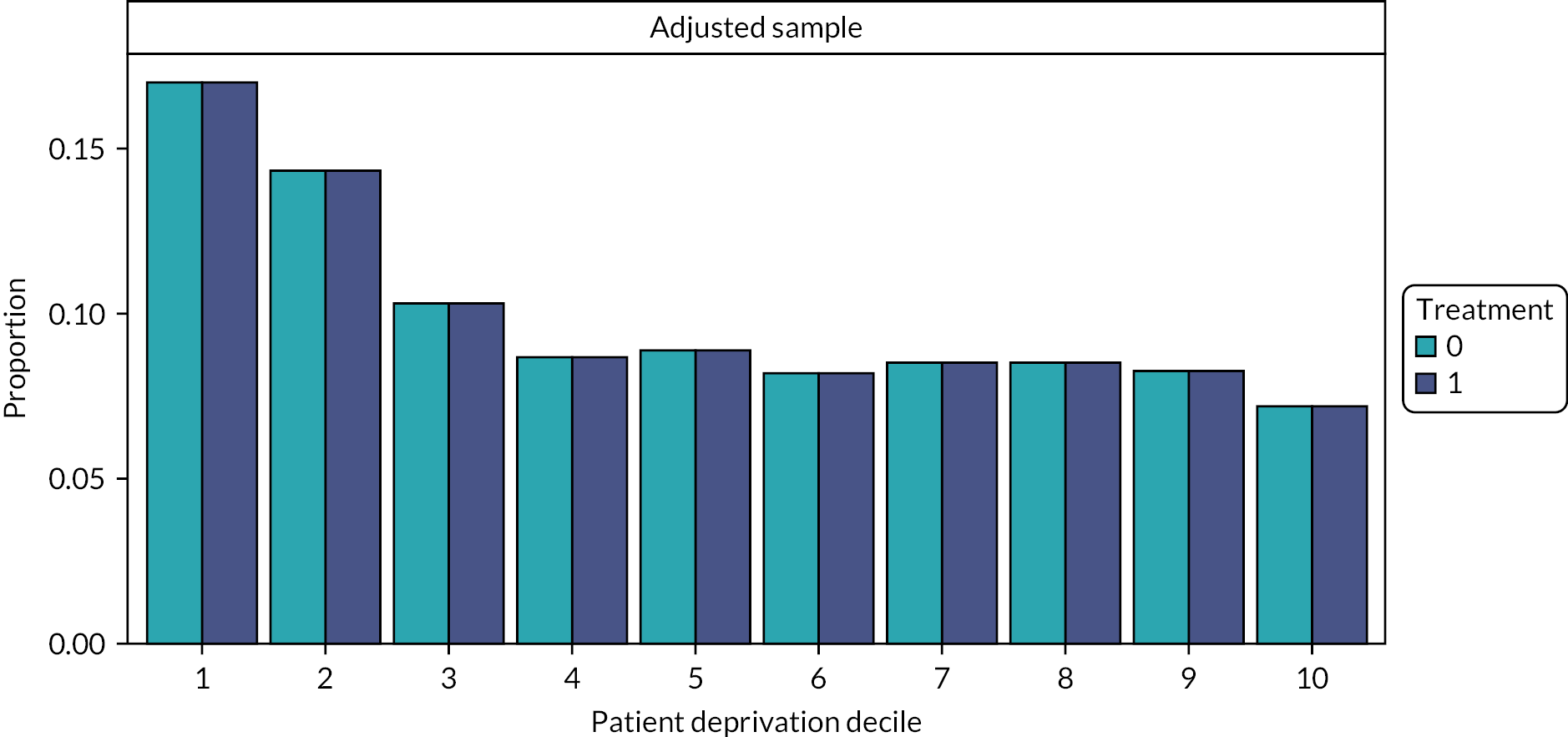
FIGURE 32.
Distributional balance for dental practice deprivation (IMD 2015) in the selected matched cohort (matching method 2).
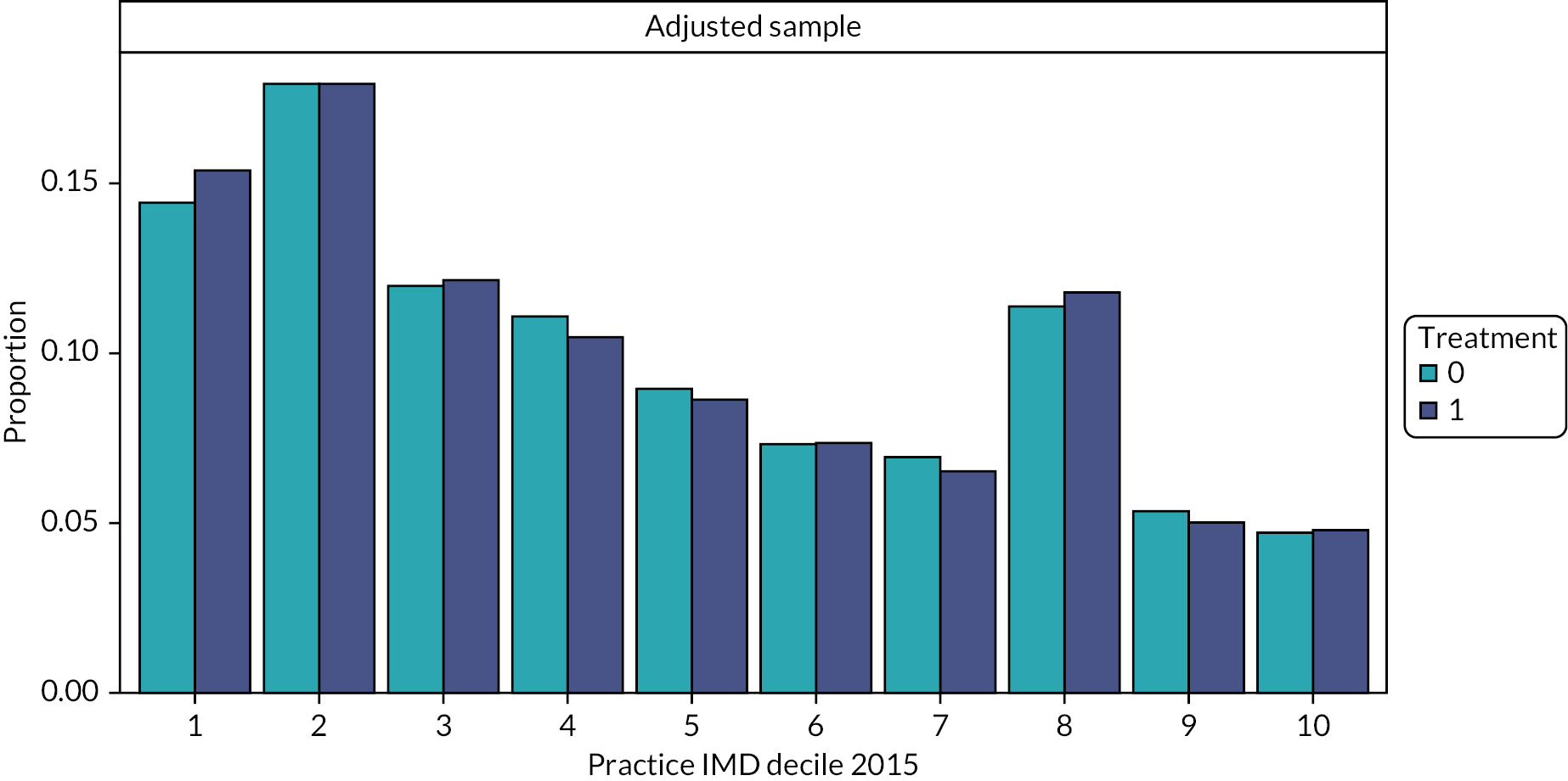
FIGURE 33.
Distributional balance for urban–rural classification of patients, for the selected matched cohort (matching method 2).
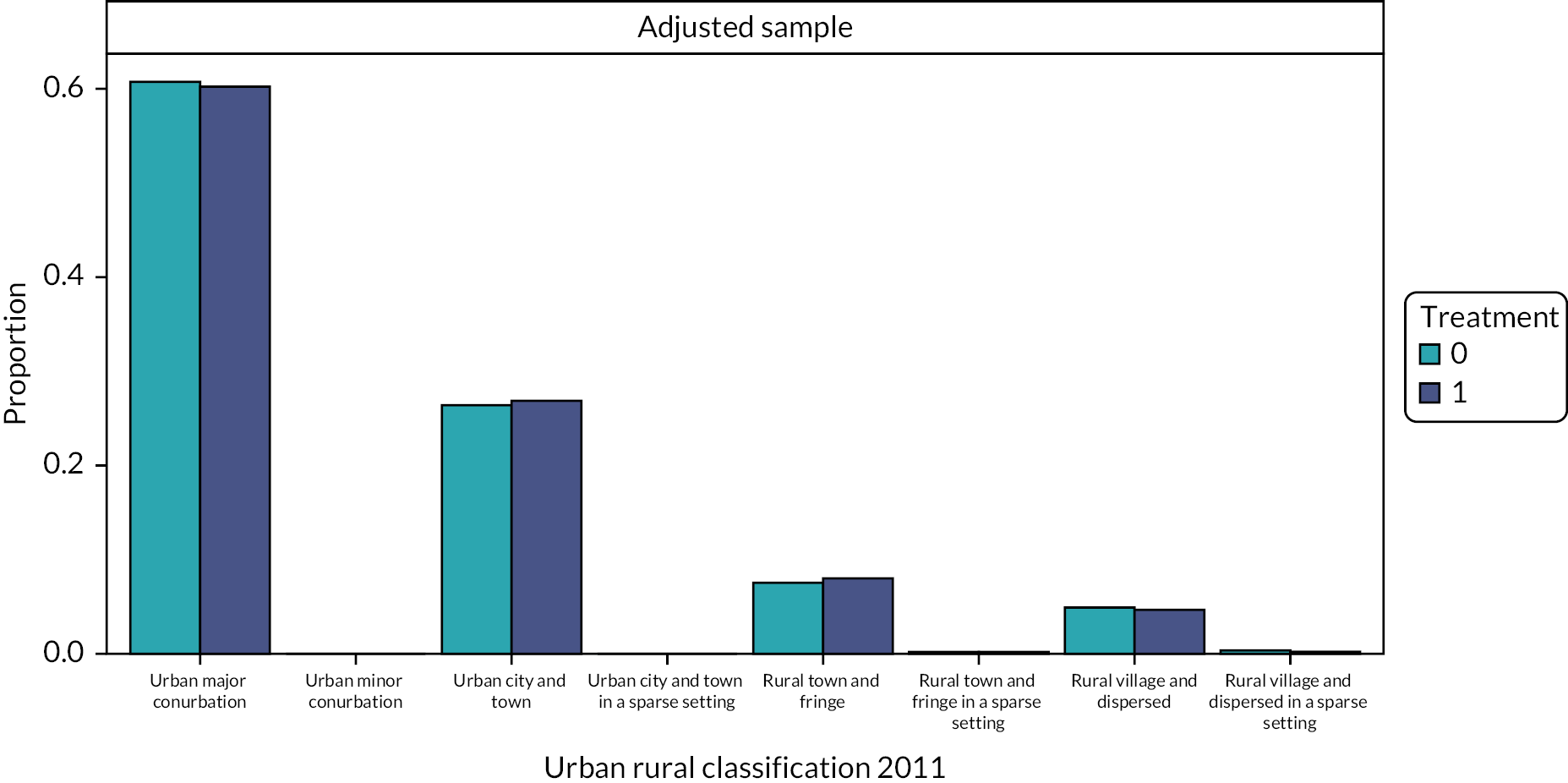
Appendix 6 Clinical results – effect estimates from the regression models including the main and interaction terms and 95% confidence intervals
Negative binomial regression | Number of obs. = 6,370,280 (SE adjusted for 1,592,570 clusters in subclass) |
|||
---|---|---|---|---|
IRR | Robust SE | 95% CI | ||
Fluoridation status | ||||
Fluoridated | 0.9763139 | 0.0040392 | 0.9684292 | 0.9842627 |
Patient deprivation decile | ||||
IMD decile 1 | 1.268732 | 0.0039805 | 1.260954 | 1.276558 |
IMD decile 2 | 1.214809 | 0.0038662 | 1.207255 | 1.222411 |
IMD decile 3 | 1.154561 | 0.003797 | 1.147143 | 1.62027 |
IMD decile 4 | 1.116263 | 0.0036138 | 1.109202 | 1.123368 |
IMD decile 5 | 1.095029 | 0.0035953 | 1.088005 | 1.102099 |
IMD decile 6 | 1.074532 | 0.0034287 | 1.067833 | 1.081273 |
IMD decile 7 | 1.060437 | 0.0034295 | 1.053737 | 1.06718 |
IMD decile 8 | 1.034779 | 0.0033514 | 1.028231 | 1.041369 |
IMD decile 9 | 1.014367 | 0.0033101 | 1.0079 | 0.020875 |
Interaction | ||||
Fluoridated IMD decile 1 | 0.9697637 | 0.0048761 | 0.9602538 | 0.9793679 |
Fluoridated IMD decile 2 | 0.978867 | 0.0050292 | 0.9690594 | 0.987738 |
Fluoridated IMD decile 3 | 1.009967 | 0.005449 | 0.9993433 | 1.020704 |
Fluoridated IMD decile 4 | 1.004074 | 0.0055378 | 0.9932781 | 1.014986 |
Fluoridated IMD decile 5 | 0.9902695 | 0.0051756 | 0.9795954 | 1.00106 |
Fluoridated IMD decile 6 | 0.9887894 | 0.0055011 | 0.9780661 | 0.9996303 |
Fluoridated IMD decile 7 | 1.00266 | 0.005549 | 0.9918426 | 1.013595 |
Fluoridated IMD decile 8 | 1.011994 | 0.0056206 | 1.001038 | 1.023071 |
Fluoridated IMD decile 9 | 1.013065 | 0.0056888 | 1.001976 | 1.024276 |
Constant | 4.985121 | 0.0009466 | 4.961301 | 5.009056 |
/lnalpha | −0.0191232 | 0.0009466 | −0.0209875 | −0.017268 |
alpha | 0.9810584 | 0.0009287 | 0.97924 | 0.9828803 |
Negative binomial regression | Number of obs. = 5,517,106 (SE adjusted for 1,419,879 clusters in subclass) |
|||
---|---|---|---|---|
Mean DMFT | IRR | Robust SE | 95% CI | |
Fluoridation status | ||||
Fluoridated | 0.968103 | 0.0022525 | 0.9636982 | 0.9725279 |
Patient deprivation decile | ||||
IMD decile 1 | 0.9437338 | 0.0018396 | 0.9401351 | 0.9473463 |
IMD decile 2 | 0.9596556 | 0.0018917 | 0.9559551 | 0.9633705 |
IMD decile 3 | 0.9567034 | 0.0019619 | 0.9528659 | 0.9605563 |
IMD decile 4 | 0.9562818 | 0.0019319 | 0.9525027 | 0.9600758 |
IMD decile 5 | 0.9670694 | 0.0019536 | 0.9632479 | 0.9709061 |
IMD decile 6 | 0.9869707 | 0.0019141 | 0.9832263 | 0.9907295 |
IMD decile 7 | 0.9904629 | 0.001919 | 0.9867089 | 0.9942312 |
IMD decile 8 | 0.9861983 | 0.0019028 | 0.9824759 | 0.9899348 |
IMD decile 9 | 0.9889758 | 0.0019103 | 0.9852387 | 0.992727 |
Interaction | ||||
Fluoridated IMD decile 1 | 0.9546576 | 0.0028915 | 0.9490072 | 0.9603417 |
Fluoridated IMD decile 2 | 0.9997162 | 0.0030568 | 0.9937428 | 1.005725 |
Fluoridated IMD decile 3 | 1.038682 | 0.003326 | 1.032184 | 1.045222 |
Fluoridated IMD decile 4 | 1.043307 | 0.0034161 | 1.036633 | 1.050024 |
Fluoridated IMD decile 5 | 1.046466 | 0.0033716 | 1.039878 | 1.053095 |
Fluoridated IMD decile 6 | 1.033708 | 0.0033195 | 1.027223 | 1.040235 |
Fluoridated IMD decile 7 | 1.038095 | 0.0033038 | 1.03164 | 1.044591 |
Fluoridated IMD decile 8 | 1.04654 | 0.0033055 | 1.040082 | 1.053039 |
Fluoridated IMD decile 9 | 1.033247 | 0.0032875 | 1.026824 | 1.03971 |
Constant | 13.7855 | 0.0194522 | 13.74743 | 13.82368 |
/lnalpha | −0.4855158 | 0.0011996 | −0.4878671 | −0.4831646 |
alpha | 0.6153797 | 0.0007382 | 0.6139345 | 0.6168283 |
Negative binomial regression | Number of obs. = 5,517,106 (SE adjusted for 1,419,879 clusters in subclass) |
|||
---|---|---|---|---|
Mean number of missing teeth | IRR | Robust SE | 95% CI | |
Fluoridation status | ||||
Fluoridated | 0.9840689 | 0.0038353 | 0.9765805 | 0.9916146 |
Patient deprivation decile | ||||
IMD decile 1 | 1.217372 | 0.0038389 | 1.209871 | 1.22492 |
IMD decile 2 | 1.210712 | 0.0038827 | 1.203126 | 1.218346 |
IMD decile 3 | 1.15646 | 0.0038863 | 1.148868 | 1.164102 |
IMD decile 4 | 1.111036 | 0.0037152 | 1.103778 | 1.118342 |
IMD decile 5 | 1.098831 | 0.0036829 | 1.091636 | 1.106073 |
IMD decile 6 | 1.095001 | 0.0035309 | 1.088103 | 1.101944 |
IMD decile 7 | 1.073625 | 0.0034467 | 1.066891 | 1.080402 |
IMD decile 8 | 1.04828 | 0.0033703 | 1.041696 | 1.054907 |
IMD decile 9 | 1.029923 | 0.0033161 | 1.023444 | 1.036443 |
Interaction | ||||
Fluoridated IMD decile 1 | 0.9490276 | 0.0046723 | 0.9399142 | 0.9582294 |
Fluoridated IMD decile 2 | 0.9915517 | 0.0049602 | 0.9818773 | 1.001321 |
Fluoridated IMD decile 3 | 1.036801 | 0.0054666 | 1.026142 | 1.047571 |
Fluoridated IMD decile 4 | 1.055798 | 0.0057172 | 1.044651 | 1.067063 |
Fluoridated IMD decile 5 | 1.048944 | 0.0056233 | 1.03798 | 1.060024 |
Fluoridated IMD decile 6 | 1.039546 | 0.0055781 | 1.028671 | 1.050537 |
Fluoridated IMD decile 7 | 1.045617 | 0.0055491 | 1.034798 | 1.05655 |
Fluoridated IMD decile 8 | 1.069425 | 0.0056606 | 1.058388 | 1.080578 |
Fluoridated IMD decile 9 | 1.043565 | 0.0055575 | 1.032729 | 1.054514 |
Constant | 5.914064 | 0.01395 | 5.886786 | 5.941469 |
/lnalpha | 0.0957889 | 0.0009871 | 0.0938542 | 0.0977235 |
alpha | 1.100527 | 0.0010863 | 1.0984 | 1.102658 |
Appendix 7 Clinical results – ancillary analyses of the primary outcome taking into account the number of courses of treatment received by each patient
Invasive dental treatments
For the primary outcome, an ancillary analysis including an offset term taking into account the number of courses of treatment per patient was carried out. This was to reflect the fact the number of invasive dental treatments a patient can receive is proportional to the number courses of treatment, and that the number of courses of treatments, that is, the opportunity for an invasive treatment to occur, differed from observation to observation.
The IRR for the effect of water fluoridation from this model was 1.013 (95% CI 1.011 to 1.016), indicating that the rate of invasive dental treatments per course of treatment in the fluoridated group was 1% higher than that of the sub/non-optimally fluoridated group. The predicted mean number of invasive treatments from this model was 5.940 (SE 0.004) for the sub/non-optimally fluoridated group and 6.020 (SE 0.005) for the fluoridated group.
We modelled the effects of water fluoridation and area-level deprivation on the number of invasive dental treatments using a negative binomial regression model with main and interaction effects, cluster robust SEs and accounting for weighting. We included an offset term for the total number of courses of treatment. The number of observations for this outcome was 6,370,280.
The conditional effect estimate for the rate of invasive dental treatments per course of treatment according to water fluoridation status from the model with the interaction term was IRR 0.973 (95% CI 0.966 to 0.979). The predicted marginal mean values for the number of invasive dental treatments were 5.983 (SE 0.005) in the fluoridated group and 5.898 (SE 0.004) in the sub/non-optimally fluoridated group. The effect estimates from the regression model for the main and interaction terms along with their CIs are reported in Table 39.
Negative binomial regression | Number of obs. = 6,370,280 (SE adjusted for 1,592,570 clusters in subclass) |
|||
---|---|---|---|---|
IRR | Robust SE | 95% CI | ||
Fluoridation status | ||||
Fluoridated | 0.9727213 | 0.0035996 | 0.9656918 | 0.9798019 |
Patient deprivation decile | ||||
IMD decile 1 | 1.582798 | 0.0044045 | 1.574189 | 1.591454 |
IMD decile 2 | 1.504887 | 0.0042218 | 1.496635 | 1.513184 |
IMD decile 3 | 1.425992 | 0.0041142 | 1.417951 | 1.434078 |
IMD decile 4 | 1.348468 | 0.0038299 | 1.340982 | 1.355995 |
IMD decile 5 | 1.282928 | 0.0037152 | 1.275667 | 1.290231 |
IMD decile 6 | 1.19961 | 0.0033565 | 1.193049 | 1.206207 |
IMD decile 7 | 1.152356 | 0.0032593 | 1.145985 | 1.158762 |
IMD decile 8 | 1.103324 | 0.0031206 | 1.097224 | 1.109457 |
IMD decile 9 | 1.064858 | 0.0030316 | 1.058933 | 1.070816 |
Interaction | ||||
Fluoridated IMD decile 1 | 1.114613 | 0.0050558 | 1.104747 | 1.124566 |
Fluoridated IMD decile 2 | 1.087393 | 0.0050568 | 1.077527 | 1.097349 |
Fluoridated IMD decile 3 | 1.045675 | 0.0051364 | 1.035656 | 1.05579 |
Fluoridated IMD decile 4 | 1.024506 | 0.0051467 | 1.014468 | 1.034643 |
Fluoridated IMD decile 5 | 0.9975503 | 0.0050105 | 0.9877781 | 1.007419 |
Fluoridated IMD decile 6 | 1.001706 | 0.0050375 | 0.9918817 | 1.011629 |
Fluoridated IMD decile 7 | 1.002839 | 0.0050291 | 0.99303 | 1.012744 |
Fluoridated IMD decile 8 | 1.002222 | 0.0050172 | 0.9924361 | 1.012103 |
Fluoridated IMD decile 9 | 0.9974461 | 0.0050275 | 0.9876408 | 1.007349 |
Constant | 0.4495232 | 0.00095 | 0.4476652 | 0.451389 |
Offset number of courses of treatment received (ln) | 1 (exposure) |
Figure 33 illustrates the mean predicted number of invasive dental treatments for the fluoridated and sub/non-optimally fluoridated groups at each deprivation decile based on the regression model for the rate of invasive dental treatments per course of treatment. The discrete changes in the predicted number of invasive treatments (the difference in the predicted values of the mean number of invasive treatments in the fluoridated group and the mean number in the sub/non-optimally fluoridated group) at each deprivation decile are also shown in accompanying Table 42.
Deprivation decile (IMD) | Difference in the predicted mean number of missing teeth (95% CI) (negative values favour water fluoridation) | p > |z| |
---|---|---|
1 | 0.604 (0.565 to 0.642) | 0.000 |
2 | 0.393 (0.355 to 0.432) | 0.000 |
3 | 0.111 (0.069 to 0.152) | 0.000 |
4 | −0.021 (−0.062 to 0.02) | 0.310 |
5 | −0.172 (−0.210 to −0.134) | 0.000 |
6 | −0.139 (−0.175 to −0.104) | 0.000 |
7 | −0.128 (−0.162 to −0.094) | 0.000 |
8 | −0.126 (−0.158 to −0.093) | 0.000 |
9 | −0.144 (−0.175 to −0.112) | 0.000 |
10 | −0.124 (−0.156 to −0.091) | 0.000 |
The mean predicted number of invasive dental treatments for the matched sample overall is highest for the most deprived decile, decreasing steadily to the least deprived decile. Within the fluoridation group, the predicted number of invasive dental treatments is higher for the more deprived deciles (deprivation decile 1 most deprived, mean 7.773, SE 0.015) than the least deprived deciles (deprivation decile 10 least deprived, mean 4.406, SE 0.014). In the most deprived deciles from IMD 1–3, the mean predicted number of invasive treatments is higher in the fluoridated group than the sub/non-optimally fluoridated group. In these deciles, the rate per course of treatment is higher in the fluoridated group than in the sub/non-optimally fluoridated group. From decile 4 onwards the predicted number of invasive treatments is higher in the sub/non-optimally fluoridated group than the fluoridated group. In all deciles the difference in the mean predicted number of invasive treatments is less than one invasive dental treatment.
Probability of receiving any invasive dental treatments taking number of courses of treatment into account
A logistic regression was used to model the probability of receiving an invasive dental treatment according to water fluoridation status, with the number of courses of treatment as an additional covariate. The resulting conditional effect estimate was an increase in the odds of receiving an invasive dental treatment in the fluoridated compared to the sub/non-optimally fluoridated group (OR 1.013, 95% CI 1.007 to 1.018). The predicted values for the probability of receiving at least one invasive dental treatment was 0.847 (SE 0.0003) for the fluoridated group and 0.845 (SE 0.0002) for the sub/non-optimally fluoridated group.
FIGURE 34.
Predicted number of invasive dental treatments with 95% CIs by water fluoridation status and deprivation decile from negative binomial regression model with offset number of courses of treatment.
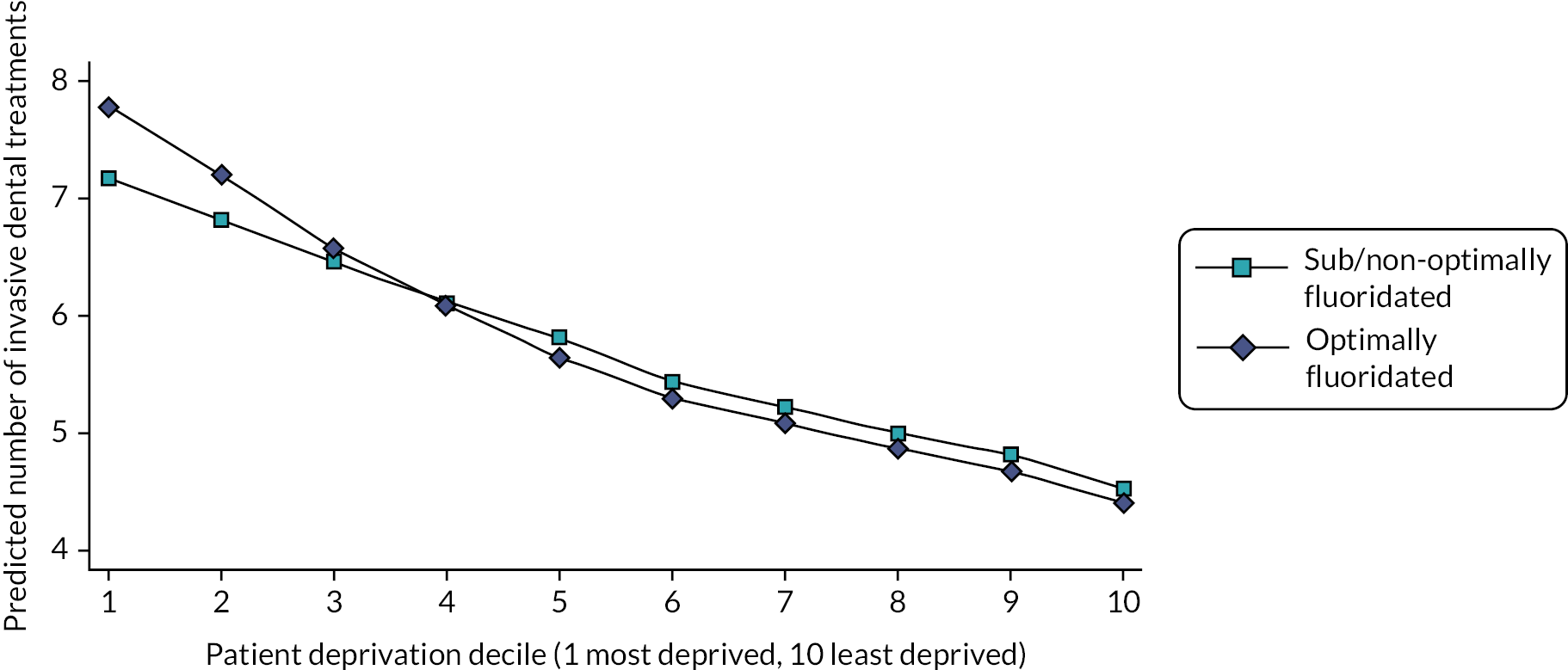
Glossary
Some of the text in this glossary has been reproduced from Goldthorpe J, Walsh T, Tickle M, Birch S, Hill H, Sanders C, et al. An evaluation of a referral management and triage system for oral surgery referrals from primary care dentists: a mixed-methods study. Health Soc Care Deliv Res 2018;6(8):1–126 under the CC BY 4.0 license, which permits others to distribute, remix, adapt and build upon this work provided the original work is properly cited. The text below includes additions, rewording and formatting changes to some of the original text.
Caries Tooth decay, also known as dental caries or cavities, is the breakdown of dental hard tissues due to acids made by bacteria present in dental plaque as a result of their metabolisation of dietary carbohydrates.
Decayed, missing and filled surfaces (adult) A cumulative count of the total number of permanent (adult) tooth surfaces ever affected by decay for an individual. Counts the number of tooth surfaces that are Decayed, Missing (extracted) or Filled. Each tooth has four or five surfaces that can be affected by decay. Mean decayed, missing and filled surfaces are used as a measure of population-level oral health.
Decayed, missing and filled surfaces (baby) A cumulative count of the total number of primary (baby) tooth surfaces ever affected by decay for an individual. Counts the number of tooth surfaces that are Decayed, Missing (extracted) or Filled. Each tooth has four or five surfaces that can be affected by decay. Mean decayed, missing and filled surfaces are used as a measure of population-level oral health.
Decayed, missing and filled teeth (adult) A cumulative count of the total number of permanent (adult) teeth ever affected by decay for an individual. Includes the number of teeth that are Decayed, Missing (extracted) or Filled. Mean decayed, missing and filled teeth are used as a measure of population-level oral health.
Decayed, missing and filled teeth (baby) A cumulative count of the total number of primary (baby) teeth ever affected by decay for an individual. Includes the number of teeth that are Decayed, Missing (extracted) or Filled. Mean decayed, missing and filled teeth are used as a measure of population-level oral health.
Fluorosis A cosmetic condition that affects the teeth. It is caused by overexposure to fluoride during the first 8 years of life. This is the time when most permanent teeth are being formed. After the teeth erupt, the teeth of those affected by fluorosis may appear mildly discoloured, with mottled areas or lines.
FP17 To claim payment for National Health Service activity under general dental service and personal dental service contracts, providers submit FP17 forms detailing dental activity data. The data recorded on the FP17 show the patient charge collected, the number of units of activity performed and treatment banding information.
General dental services The most widespread of the two main contract types for primary care dentistry. General dental service contracts are usually not time-limited, and contract holders are required to provide the full range of services described as ‘mandatory’. Patient charge revenue is collected, and units of dental activities are the currency of the contract.
Lower super output area A geographic area which is used to describe the health and social care characteristics of the population living there. Each lower super output area contains an average of 1500 people and/or 600 households.
National Health Service Business Services Authority Dental Services The National Health Service Business Services Authority Dental Services remunerates dentists based on FP17 claims submitted and provides dental statistics and key information to national, regional and local National Health Service organisations.
Ofwat The economic regulator of the water sector in England and Wales.
Patient charge revenue Patient charge revenue generated by the fees charged for dental treatment at bands 1, 2 and 3. This is a copayment scheme, and certain individuals are exempted from paying based on their age or benefit status.
Postcode A coding system made up of a combination of letters and numbers which is used to sort mail in the United Kingdom.
Unit of dental activity The contract currency for general dental service and personal dental service contracts in England. Each dental procedure has been classified into a banding structure, which determines what patients pay in National Health Service dental charges and the number of units of dental activity a dentist receives. Band 1 attracts one unit of dental activity, whereas bands 2 and 3 attract 3 and 12 units of dental activity, respectively. The Annual Contract Value is the annual target of units of dental activity that a dental contact must provide. The national average price for a unit of dental activity is approximately £27, but the unit of dental activity value is determined individually for each contract, therefore dental practices in the same locality, serving similar populations, are likely to have different units of dental activity values.
Unit of orthodontic activity The contract currency for general dental service or personal dental service contracts in England that have been commissioned specifically to provide orthodontic care.
Retrospective cohort study A type of research study where the health/disease status of the participants (the ‘cohort’) is already recorded and researchers look backwards into their past for factors that could explain their health status.
List of abbreviations
- AMSTAR 2
- A MeaSurement Tool to Assess Systematic Reviews
- ANSAOH
- Australian National Survey of Adult Oral Health
- ATT
- average treatment effect in the treated
- BSA
- Business Service Authority
- CADTH
- Canadian Agency for Drugs and Technologies in Health
- CATFISH
- Cumbrian Assessment of Teeth a Fluoride Intervention Study for Health
- COMET
- core outcome measures in effectiveness trials
- DAG
- directed acyclic graph
- DMFS
- decayed, missing and filled surfaces
- DMFT
- decayed, missing and filled teeth
- DWI
- Drinking Water Inspectorate
- EPR
- electronic patient record
- eQQ
- empirical quantile–quantile
- GDP
- general dental practitioner
- GDS
- general dental service
- GIS
- geographic information system
- GLM
- generalised linear model
- HES
- Hospital Episode Statistics
- ICER
- incremental cost-effectiveness ratio
- ICHOM
- International Consortium for Health Outcomes Measurement
- IMD
- Index of Multiple Deprivation
- IRR
- incidence rate ratio
- KPI
- key performance indicator
- LSOA
- lower super output area
- MRC
- Medical Research Council
- NHMRC
- National Health and Medical Research Council
- NICE
- National Institute for Health and Care Excellence
- NIHR
- National Institute for Health and Care Research
- OMG
- Operational Management Group
- ONS
- Office for National Statistics
- PDS
- personal dental service
- PDS+
- personal dental service plus
- PHE
- Public Health England
- PPI
- patient and public involvement
- PRIMER
- Primary Care Research in Manchester Engagement Resource
- PWC
- population-weighted-centroids
- QALY
- quality-adjusted life-year
- QoL
- quality of life
- ROI
- return on investment
- SMD
- standardised mean difference
- SSC
- Study Steering Committee
- UDA
- unit of dental activity
- UOA
- unit of orthodontic activity
- VR
- variance ratio
- WSZ
- water supply zone