Notes
Article history
The research reported in this issue of the journal was funded by the HTA programme as project number 10/34/01. The contractual start date was in May 2012. The draft report began editorial review in May 2014 and was accepted for publication in September 2015. The authors have been wholly responsible for all data collection, analysis and interpretation, and for writing up their work. The HTA editors and publisher have tried to ensure the accuracy of the authors’ report and would like to thank the reviewers for their constructive comments on the draft document. However, they do not accept liability for damages or losses arising from material published in this report.
Declared competing interests of authors
Robert C Stein reports grants from the NIHR-UCLH Biomedical Research Centre during the conduct of the study; grants and personal fees from Celgene Ltd and grants from Amgen Ltd outside the submitted work. Christopher Poole reports personal fees from Genomic Health outside the submitted work. Andreas Makris reports personal fees from Genomic Health during the conduct of the study. John MS Bartlett reports the following, all outside the submitted work: personal fees and other (support in kind) from BioNTech AG; personal fees from GE Healthcare; other (support in kind) from NanoString Technologies Inc.; other (support in kind) from Genoptix Medical Laboratory; grants from the NIHR HTA programme; grants from Cancer Research UK; grants from Celgene Ltd; grants from Ontario Institute for Cancer Research (OICR) High Impact Clinical Trials (HICT) Programme; grants from The Breast Cancer Research Foundation; grants from Medical Research Council; grants from Pfizer (UK); grants from Breakthrough Breast Cancer; grants from European Union; grants from The Breast Cancer Institute (UK); grants from European Organisation for Research and Treatment of Cancer; grants from Roche Pharmaceuticals Limited; personal fees from Daiichi Sankyo Pharma Development; and personal fees from Rexahn Pharmaceuticals Inc.
Permissions
Copyright statement
© Queen’s Printer and Controller of HMSO 2016. This work was produced by Stein et al. under the terms of a commissioning contract issued by the Secretary of State for Health. This issue may be freely reproduced for the purposes of private research and study and extracts (or indeed, the full report) may be included in professional journals provided that suitable acknowledgement is made and the reproduction is not associated with any form of advertising. Applications for commercial reproduction should be addressed to: NIHR Journals Library, National Institute for Health Research, Evaluation, Trials and Studies Coordinating Centre, Alpha House, University of Southampton Science Park, Southampton SO16 7NS, UK.
Chapter 1 Background
The current treatment of breast cancer
Breast cancer is a major public health problem. It is the most commonly occurring cancer in the UK, with an annual incidence of 50,000, and it is the second most frequent cause of cancer death in women, with about 12,000 deaths in 2011. 1 Of the women who develop breast cancer, 80% are over 50 years old at diagnosis and most deaths occur in this age group.
The treatment of primary breast cancer, which is undertaken with curative intent, includes local (surgery and radiotherapy) and systemic [chemotherapy, anti-oestrogen and human epidermal growth factor receptor 2 (HER2)-targeted drugs] therapies. The goal of adjuvant systemic treatment is to eliminate occult microscopic metastatic disease and thus prevent incurable distant relapse. Decisions on adjuvant therapy are informed by an individual patient’s risk of developing future overt metastatic disease. This risk is a function of both tumour stage (size and number of involved axillary lymph nodes) and tumour biology. Relevant biological features include tumour grade, oestrogen receptor (ER) status and HER2 status. ER and HER2 status also predict sensitivity to anti-oestrogen drugs and anti-HER2-targeted therapy, respectively. Distant relapse, which affects a minority of patients, typically occurs after an interval of several years; later relapse is a feature of both ER-positive and lower-grade tumours. 2
Anti-oestrogen therapy with tamoxifen, and more recently aromatase inhibitors (AIs), is considered to be the mainstay of systemic adjuvant therapy for post-menopausal women with ER-positive disease, the most common presentation of breast cancer. AIs have been shown to be superior to tamoxifen in a number of large randomised clinical trials,3 and current National Institute for Health and Care Excellence (NICE) guidance recommends that these drugs should be offered to the majority of post-menopausal patients. 4
During the 2000s, there was a large expansion in the use of adjuvant chemotherapy, especially for post-menopausal women. Decisions on chemotherapy are based on perceived risk. In the UK, as in many other countries, it has become standard to offer chemotherapy with anthracyclines and/or taxanes to all women under 70–75 years of age with axillary node involvement. For those without nodal involvement, who are at inherently lower risk, chemotherapy is usually reserved for those with ER-negative and/or HER2-positive tumours or with high-grade tumours.
Although undoubtedly highly effective for some women, chemotherapy may be extremely unpleasant, with common side effects such as hair loss, fatigue, nausea, painful mouth ulcers, weight gain, muscle pain, diarrhoea or constipation, and loss of sensation in hands and feet. Rates of admission to hospital with serious complications have mostly been reported to be in the range 20% to 25%5–7 and there is a small risk of early death from treatment, reported to be in the range 0.2% to 0.3% in recent studies. 8,9 Patients are frequently unable to work both during treatment and for some time thereafter,10 which has considerable cost to society. Many are left with anxiety, fatigue and depression, which may severely affect their quality of life for months or even years afterwards. 11,12 There is also a small long-term risk of treatment-induced leukaemia and cardiomyopathy13 (although there is considerable uncertainty about the incidence of chemotherapy-induced cardiovascular complications). 8,14 It does not seem beyond the bounds of possibility that the overall mortality from early and late complications of chemotherapy lies in the range 1% to 2%.
Chemotherapy itself is expensive. The cost of delivering a course of fluorouracil, epirubicin, cyclophosphamide and docetaxel (FEC-T) or of fluorouracil, epirubicin and cyclophosphamide (FEC) alone, which are the two most commonly used adjuvant chemotherapy regimens in the NHS, is estimated at £4600 and £3800, respectively15 (updated to 2014 prices). This includes drug costs, outpatient visits and hospital admissions for the management of complications. Approximately 18,500 patients with breast cancer (41% of diagnoses) received chemotherapy in the UK in 2006. 16 As a result, adjuvant chemotherapy treatment for breast cancer imposes a financial burden of approximately £80M per year on the NHS.
Prognostic and predictive nomograms such as ‘Adjuvant! Online’ (Adjuvant! Inc., San Antonio, TX, USA; www.adjuvantonline.com)17 and PREDICT (www.predict.nhs.uk)18 have been increasingly used since the mid-2000s. These are derived largely from clinical trial-acquired follow-up data to inform decisions about the use of systemic adjuvant therapy. However, such tools provide information only on what will happen to groups of patients on average and are not reliable at predicting outcomes for individuals. Thus, within a population of women with ER-positive tumours, who may, as a group, benefit from a statistically significant and clinically meaningful reduction in the risk of relapse consequent to the addition of chemotherapy to adjuvant endocrine therapy, there will be individuals who gain nothing as well as those who gain everything.
The advent of new technologies that offer improved means to characterise the biology of individual tumours heralds an era of more personalised medicine with respect to their potential to inform rational treatment decisions on a patient-by-patient basis. The Optimal Personalised Treatment of early breast cancer usIng Multiparameter Analysis (OPTIMA) trial is designed as a prospective test of the success of these technologies in identifying that sizeable subgroup of women with breast cancer (among those who might be expected to be routinely offered adjuvant chemotherapy based on conventional criteria) whose tumours are intrinsically insensitive to chemotherapy. For these women, the benefit of receiving such treatment would not outweigh the harm from treatment, as they would experience only toxicity and delay in starting more effective adjuvant endocrine therapy and radiotherapy.
Redefining breast cancer
The traditional classification of breast cancer is based on morphology, most notably tumour grade, which when combined with stage (tumour size and extent of nodal spread) provides valuable prognostic information, as exemplified by the Nottingham Prognostic Index (NPI). 19 In recent years, multiple additional prognostic markers have been defined through studies of tumour protein and gene expression.
The best established of these are receptors for steroid hormones, specifically ER and progesterone receptor (PgR), and HER2 gene amplification. Positive ER expression confers a good prognosis and also predicts sensitivity to anti-oestrogen drugs. HER2 gene amplification with associated HER2 receptor overexpression is an adverse prognostic feature but predicts sensitivity to HER2-targeted drugs such as trastuzumab (Herceptin®, Roche). The value of Ki-67, a marker of proliferation which is not routinely measured, is more controversial20 and is subject to difficulties in assay standardisation. 21–24
Since 2000, with the emergence of gene expression profiling (GEP) using microarray technology, a new molecular classification of breast cancer has been developed. 25–27 This classification divides breast cancers into four main ‘intrinsic subtypes’: luminal A, luminal B, HER2 enriched and basal-like. These subtypes differ markedly in their clinical behaviour and response to therapy, as shown in the summary table (Table 1). This goes some way to explaining the highly heterogeneous clinical behaviour of the disease. Among the intrinsic subtypes, luminal A has a significantly better prognosis than the other subtypes. 28,29 Luminal A breast cancer is broadly recognised as having a low proliferation rate (typically grade 1 or 2), being strongly positive for steroid hormone receptor expression and expressing HER2 at normal levels, although some 25% of such cancers have a different molecular subtype. 30 Molecularly classified luminal A breast cancer makes up 50–55% of all breast cancers diagnosed in the developed world. 30
Characteristics | Intrinsic subtypes | |||
---|---|---|---|---|
Luminal A | Luminal B | HER2 enriched | Basal-like | |
Prognosis | Good | Moderate | Poor | Poor |
Proliferation | Low | Moderate | High | High |
Chemosensitivity | Low/nil | Moderate | High | High |
ER | Strong | Variable | Nil | Nil |
HER2 amplification | Uncommon | In subset | Usual | Nil |
The original research into intrinsic subtypes required complex microarray analysis of frozen tissue samples to analyse the simultaneous expression of thousands of genes within each breast cancer, with associated bioinformatics challenges. This GEP technology is widely regarded as too complex and variable to bring into the clinical setting. Recently, progress has been made in mapping the original microarray-based system onto immunohistochemical markers that can be used in routine pathology laboratories,2,31 although correlation with molecular subtyping is imperfect. 28 Several molecular assays which assign subtypes by measuring expression of a subset of the genes identified as significant in the original GEP studies are now marketed but are not in widespread clinical use. The four intrinsic subtypes do not provide a full description of breast cancer, and recent work suggests that there may be as many as 1032 but, until a more robust molecular classification is developed, the intrinsic classification provides an extremely useful framework for thinking about breast cancer.
Differential sensitivity of breast cancer subtypes to chemotherapy
The strongest evidence for the effectiveness of adjuvant chemotherapy comes from the meta-analyses of over 101,000 patients in 123 chemotherapy trials conducted around the world, known as the Oxford Overview. 9 For a post-menopausal woman with steroid hormone-sensitive breast cancer treated with tamoxifen, the Oxford Overview suggests that 10-year breast cancer mortality is reduced from about 32% to about 21% by anthracycline–taxane chemotherapy. Although this is clearly worthwhile, nine patients need to be treated for one life to be saved.
Most published adjuvant chemotherapy trials have made the assumption that chemotherapy benefits are similar for breast cancer, irrespective of tumour characteristics. Up to the mid-2000s, the majority have not restricted patient entry by tumour grade or receptor status. The underlying assumption in these trials that the proportional benefits of chemotherapy apply uniformly to all cancers irrespective of histological characteristics of the tumour is supported by the Oxford Overview analysis, although limited histological information is available from those older studies that included a no chemotherapy comparison. 9 The development of the intrinsic classification requires re-evaluation of all of the available evidence on adjuvant chemotherapy treatment. Now that different subtypes of breast cancer that behave in different ways are recognised, it is necessary to investigate the appropriate use of chemotherapy within the new classification.
It can be argued that the effectiveness of chemotherapy for luminal A breast cancer is low in comparison with the other subtypes, irrespective of tumour stage. 33 Retrospective ad-hoc subgroup analysis of a number of large trials of taxane versus non-taxane chemotherapy regimens have mostly suggested that there is no significant benefit for the newer and more toxic regimens for women with ER-positive HER2-negative cancers (e.g. see Hayes et al. 34), most of which would be luminal A tumours although not all such analyses confirm this, most notably the Oxford Overview. 9 Similar analyses of comparisons of anthracycline chemotherapy with older cyclophosphamide, methotrexate and fluorouracil (CMF) regimens suggest that the anthracycline benefit is limited to patients whose tumours expressed high-risk markers. 35 A recent analysis36 of two pivotal trials37,38 of CMF chemotherapy with or without endocrine therapy versus endocrine therapy alone in women node-negative cancers failed to demonstrate a clear chemotherapy benefit in those women whose tumours were demonstrated to be ER-positive using modern assays, in contrast to other subgroups. Furthermore, those trials of chemotherapy versus no chemotherapy that included endocrine therapy predated AI therapy, which is a more effective treatment than tamoxifen.
A second line of evidence that chemotherapy sensitivity is influenced by tumour biology comes from studies of presurgical (neoadjuvant) chemotherapy. Analysis of the outcome of treatment according to intrinsic subtype of individual tumours is particularly striking, with a ‘pathological’ complete response rate of 6% in luminal tumours compared with 45% in basal type. 39 A separate study showed that the chances of achieving a pathological complete response for patients with luminal B tumours was 2.4-fold greater than for patients with luminal A tumours. 28 Similar findings have been reported in the Investigation of Series Studies to Predict Your Therapeutic Response With Imagine and Molecular Analysis (I-SPY) 1 trial,40 which systematically evaluated chemotherapy response in relation to a number of biomarker profiles.
The third and particularly relevant line of evidence comes from the retrospective analysis of historical trials comparing chemotherapy plus tamoxifen with tamoxifen alone in ER-positive breast cancer according to the results of the Oncotype DX® (Genomic Health Inc., Redwood City, CA, USA) test performed on archival tumour tissue. The evidence that assays such as Oncotype DX are able to predict chemotherapy response is discussed below.
Multiparameter assays in breast cancer
The development of the intrinsic classification has transformed our understanding of breast cancer and is changing clinical management to a more individualised approach. In parallel, it is driving intensive research efforts to develop simpler molecular tools that classify breast cancers and, more importantly, quantitate the risk of relapse for individual patients following particular treatment. These new tests typically involve the simultaneous measurement of expression of a number of genes either at the protein or messenger ribonucleic acid (mRNA) level. Most of the better-validated assays are commercialised and are either available or in the process of being marketed for clinical use. Although the early multiparameter assays that relied on mRNA measurement required fresh tissue, all the commercialised assays now work on formalin-fixed paraffin-embedded (FFPE) tissue. One of the main drivers for the development of multiparameter assays is the clinical uncertainty surrounding the benefits of adjuvant chemotherapy for women with ER-positive HER2-negative breast cancer, so the majority of assays have focused on this group of patients. Most assays have been developed and validated in (largely) node-negative populations whose prognosis is not complicated by the effect of lymph node involvement.
The output of the available assays takes the form of either a simple numerical score that can be used against a calibration chart to estimate recurrence risk [e.g. Oncotype DX41 and Breast Cancer IndexSM (BCI) (bioTheranostics, San Diego, CA, USA)]42,43 and/or assignment to either a predefined risk category or subtype. The most common measure of relapse is the risk of developing metastatic disease by 10 years from diagnosis. Most commercially developed assays are strongly influenced by steroid hormone sensitivity, HER2 status and proliferation. A number of assays have been evaluated only in ER-positive populations.
All of the available assays have been retrospectively tested on archival material, mostly from historical trials; to date, no prospective evaluation of any multiparameter assay has been published. Although it has been argued that retrospective analysis of tumour samples can provide satisfactory validation when used with appropriate precautions,44 a recent consensus panel has argued strongly for prospective validation studies. 45 Four systematic reviews46–49 have examined the data supporting the validation of multiparameter assays, particularly Oncotype DX and MammaPrint® (Agendia, Irvine, CA, USA) in detail. Table 2 lists most of the multiparameter assays that have been described for ER-positive HER2-negative breast cancer. Several of these are described in detail below (see Individual multiparameter assays).
Assay (vendor) | Details of multiparametric assay | Output | Availability | Reference |
---|---|---|---|---|
Perou et al.25 and Sorlie et al.26,27 (non-proprietary) | The original description of the intrinsic classification using 495 genes (the most highly cited papers in breast cancer) | Subtype | – | Perou et al., 200025 Sorlie et al., 200326 Sorlie et al., 200127 |
Oncotype DX (Genomic Health Inc.) | A 21-gene qRT-PCR expression assay (using 16 cancer-related and five normalisation genes) | Risk score | Central laboratory (USA) | Paik et al., 200441 |
MammaPrint + BluePrint (Agendia, Irvine, CA, USA) | A 70 + 80 gene microarray-based expression signature | Risk category and subtype | Central laboratory (the Netherlands) | Glas et al., 200650 Mook et al., 201051 |
Rotterdam signature (non-proprietary) | A 76-gene microarray-based expression signature | Risk score | Not in clinical use | Foekens et al., 200652 |
Mammostrat (Clarient/GE Healthcare, Aliso Viejo, CA, USA) | A five-gene immunohistochemical assay | Risk score | Central laboratory (USA) | Ring et al., 200653 |
Prosigna (PAM50) (NanoString Technologies Inc., Seattle, WA, USA) | A 50-gene expression assay using the NanoString system | Subtype and risk score (± clinical data) | Regional laboratories | Geiss et al., 200854 Dowsett et al., 201355 |
BCI (bioTheranostics, San Diego, CA, USA) | A seven-gene qRT-PCR expression assay | Risk score | Central laboratory (USA) | Ma et al., 200842 |
IHC4 (AQUA and non-proprietary) | Quantitative immunohistochemical assay for ER, PgR, HER2, Ki67 | Risk score (± clinical data) | Academic | Cuzick et al., 201156 |
MapQuant (Genomic Grade Index, Bordet Institute, Brussels, Belgium) | A 97-gene microarray-based expression assay | Risk category | Not currently available | Sotiriou et al., 200657 |
EndoPredict (Sividon, Sividon Diagnostics, Cologne, Germany) | A seven-gene qRT-PCR expression assay | Risk score (± clinical data) | Regional laboratories | Filipits et al., 201158 |
NPI+ | A 10-gene immunohistochemical assay | Subtype and risk category (± clinical data) | In development | Rakha et al., 201459 |
MammaTyper (BioNTech Diagnostics GmbH, Mainz, Germany) | A five-gene qRT-PCR assay | Subtype | In development | Aigner et al., 201260 |
Individual multiparameter assays
Oncotype DX
This is a polymerase chain reaction (PCR)-based expression assay testing expression of 21 genes, 16 of which are cancer related and five are for normalisation. The assay is used in FFPE specimens. The initial study41 described the derivation of the 21-gene signature assay from expression array analysis of tamoxifen-treated cancers and its translation and validation into a multiplex PCR diagnostic assay. The test output is the continuously variable numerical ‘recurrence score’ (RS), which has a monotonic relation to the risk of distant recurrence at 10 years following tamoxifen treatment of ER-positive node-negative breast cancer. Individual patient risk can be estimated from the calibration provided with the results. Additionally, the patients are divided into three risk categories (low, intermediate and high), where intermediate is defined (from the initial validation study) as a 10–20% risk of developing distant metastases over 10 years. Genomic Health performs this test centrally in a single US laboratory; the UK list price for the assay is £2580.
Multiple additional studies,61–65 comprising the retrospective analysis of patients’ outcomes in Phase 3 trials within ER-positive breast cancer, have confirmed the value of Oncotype DX as a predictor of residual risk following endocrine therapy and have extended the original observations to patients with positive lymph nodes, and also to patients with ductal carcinoma in situ. 66 Oncotype DX has been demonstrated to reclassify risk defined by Adjuvant! Online,17 which is a risk predictor that uses conventional histopathology information. 64,67 Oncotype DX has also been shown to predict chemotherapy sensitivity in the neoadjuvant setting. 68,69
Analysis of individual patient Oncotype DX RSs has been performed retrospectively on a subset of patients included in two historical clinical trials of chemotherapy versus no chemotherapy. The analyses of the B-20 trial of the National Surgical Adjuvant Breast and Bowel Project (NSABP B-20) trial in women without axillary nodal involvement61 and in the Southwest Oncology Group (SWOG) trial 8814 (SWOG88-14) trial in women with node-positive disease62 have shown that there is no chemotherapy benefit for women with an RS in the low- or intermediate- risk groups. The analysis of the SWOG88-14 trial is particularly important as it shows that even in heavily (≥ 4) node-positive patients who have a poor prognosis by virtue of stage, there is no benefit to the addition of chemotherapy to adjuvant endocrine therapy, compared with endocrine therapy alone, if the RS is low. These data are widely interpreted to suggest that Oncotype DX is able to predict whether or not tumours are likely to be sensitive to chemotherapy. Incorporating clinical data (tumour stage, grade and age) for patients with node-negative disease into the test improves its performance as a prognostic test but crucially does not improve its ability to predict chemotherapy sensitivity. 64,70
A number of studies (e.g. Ademuyiwa et al. 71 and Albanell et al. 72), including two conducted within the UK,73,74 have shown that the use of Oncotype DX leads to changes in chemotherapy decisions in about one-third of patients, although these studies involve small sample sizes and often have poorly described protocols.
Limitations of Oncotype DX highlighted by the systematic reviews46–49 include the fact that there are relatively few data about the performance of the test in node-positive patients and that the data supporting the ability of the test to predict chemotherapy benefit are not robust. 49 Additionally, Oncotype DX, unlike some other tests, is only able to predict recurrence risk over the first 5 years after diagnosis. 75 No prospective studies reporting the impact of Oncotype DX on long-term outcomes, such as overall survival have been identified. In addition, the test has not been prospectively trialled against any alternatives. There is no evidence that the Oncotype DX assay is any more informative than other gene expression assays. 76
In 2007, an American Society of Clinical Oncology expert panel reviewed the evidence and recommended the use of Oncotype DX in routine care. 77 The test has proved to be popular with patients and clinicians, and is undoubtedly the global market leader in the field. Genomic Health reported that as of 31 December 2014, more than 500,000 tests have been preformed, the majority for invasive breast cancer, generating a total revenue of US$1.4B for the company over a 7-year period. 78
MammaPrint
The MammaPrint assay is based on 70 genes, identified by GEP, that were shown to predict outcome in a mixed population of young breast cancer patients with node-negative disease, none of whom was treated with adjuvant tamoxifen. 79 Following an initial validation study performed in a similar population,80 the test was commercialised. Initially it was performed using array technology on fresh-frozen tissue,50 but since 2012 it has been available for FFPE tissue samples. 81 Currently the test is marketed by Agendia as part of the SYMPHONY profile and is performed in central laboratories located in the Netherlands and in the USA; the current list price of SYMPHONY is €2675. The output from MammaPrint is essentially a simple binary division into low risk and high risk. The BluePrint® (Agendia, Irvine, CA, USA) assay provides subtyping information (luminal type, basal type and HER2 type); combining the MammaPrint and BluePrint results for patients with ER-positive tumours allows tumours to be divided into luminal A and luminal B subtypes.
Following the initial validation study,80 MammaPrint has been reported to provide valid prognostic information in a number of additional studies, and evidence has shown that it is able to reclassify risk against existing prognostic variables. 51,82–85 Several studies40,86,87 have shown that MammaPrint is able to predict response to neoadjuvant chemotherapy, including differentiating between high- and low-risk ER-positive disease. A study of patients pooled from several data sets has provided evidence that MammaPrint is able to predict chemotherapy benefit in patients with ER-positive disease and up to three involved lymph nodes. 88 There is evidence that MammaPrint results influence chemotherapy decisions made by European oncologists (predominantly Dutch) in clinical practice89,90 without any apparent increase in recurrence after 5 years. 91
Overall, the evidence supporting MammaPrint is broad-based and convincing but, in comparison with studies validating the use of Oncotype DX, it is less comprehensive, with individual studies tending to have a lower quality. This is also the conclusion of the four systematic reviews of the field. 46–49 The limitations of the evidence in support of Oncotype DX also apply to MammaPrint. Although one study92 has purported to show that MammaPrint is able to predict outcome beyond 5 years, it clearly does not predict late relapse.
MammaPrint is widely used in clinical practice in parts of Europe but has never achieved the global penetration of Oncotype DX. Its use in the UK is very limited, probably because the requirement for fresh tissue up to 2012 was widely considered to be an obstacle by clinicians and, as such, it was ‘second to market’.
IHC4
There is long-standing evidence that the conventional immunohistochemistry markers, ER, PgR, HER2 and Ki67 (a marker for cell proliferation),33,93 are able to identify patients at increased residual risk following endocrine therapy. The IHC4 test relies on quantitative immunohistochemistry for these four markers. The component scores have been integrated into a viable predictor of residual risk in post-menopausal women with ER-positive disease. The assay was developed using outcome data and stored tumour blocks from women who participated in the Arimidex, Tamoxifen, Alone or in Combination (ATAC) trial. 56 IHC4, using conventional manual colorimetric immunohistochemistry, has been developed in an entirely academic setting and, as there are no proprietary data, there is limited incentive for its commercial development. Genoptix, a US-based company, promotes the test using proprietary automated quantitative immunofluorescence (AQUA®). 94 The output from IHC4 is a numerical score, with a division into three risk groups using the same definitions as Oncotype DX.
The original IHC4 validation study was performed on a large patient cohort (n = 1125), and the report included a second validation performed on an independent patient cohort. 56 A completely independent study has been performed on around 4500 patients recruited from the Tamoxifen Exemestane Adjuvant Multinational (TEAM) study, using both conventional immunohistochemistry and quantitative immunofluorescence. 95 Both methods of detection provided significant prediction of residual risk following endocrine therapy with reasonable correlation, although the performance of quantitative immunofluorescence was superior on the basis of the c-index. One study96 has demonstrated that IHC4 is able to reclassify risk defined by Adjuvant! Online or the NPI. In addition to these studies, a detailed comparison has been performed between IHC4 and Oncotype DX in the transitional cohort of the ATAC trial (transATAC). 56 Correlation between the two was modest, but the two assays provide a similar amount of prognostic information. As both tests are strongly influenced by measures of proliferation, steroid hormone receptor signalling and HER2 status, and were developed with similar patient cohorts, this is unsurprising.
The validation of IHC4 as a predictor of residual recurrence risk involves far more patients than validation studies performed for any other multiparameter assay. Additionally, the similarity between IHC4 and Oncotype DX implies that, by extension, the ability of Oncotype DX to predict chemotherapy sensitivity is like to apply to ICH4. The low estimated cost of £150 for performing IHC4 using conventional immunohistochemistry49 and its portability are potential strong advantages for IHC4 over other multiparameter assays. However, its portability is also its principal weakness, as the reproducibility of manual quantitative immunohistochemistry, particularly for Ki67, is limited. 21–24 It remains to be demonstrated whether or not quantitative immunofluorescence established in local laboratories is more reproducible.
Prosigna® (PAM50)
PAM5028 is a quantitative reverse transcriptase-PCR expression assay developed in an academic setting using 50 genes selected from the original set identified by Perou et al. in their pioneering microarray studies of intrinsic subtype. 25–27 The assay provides subtyping information and additionally a numerical risk of recurrence (ROR) score; there are several variants of the ROR score incorporating varying amounts of clinical and conventional histological information.
The ProsignaTM test, which measures the expression of the PAM50 gene set using the NanoString platform,54 has been developed by NanoString Technologies (Seattle, WA, USA). 97 The assay can be performed in suitable local laboratories using hardware and reagents provided by the company. The analytical validity of the assay has been demonstrated in a distributed environment98 and the company was granted the necessary US Food and Drug Administration approval in September 2013 for its marketing as a prognostic assay in post-menopausal patients. Prosigna was independently trained and validated but Prosigna and PAM50 subtype predictions and ROR scores are highly concordant.
PAM50, and by inference Prosigna, can be used in all subtypes of breast cancer, but detailed validation studies have been performed on patients with ER-positive disease. 28,55,75,99–101 PAM50 has been validated as a predictor of residual risk in two studies28,99 and has been shown to reclassify risk defined by Adjuvant! Online using conventional pathology. Similarly, Prosigna has been demonstrated to predict residual risk and to reclassify risk in the large transATAC cohort and in approximately 1500 mostly node-negative patients treated with endocrine therapy alone from the Austrian Breast and Colorectal Cancer Study Group (ABCSG-8) study of endocrine therapy in post-menopausal women. 55,100 Prosigna, in contrast to Oncotype DX and IHC4, is able to predict late (beyond 5 years) recurrence in these two patient cohorts (transATAC and ABCSG-8). 75,101
Response rates to neoadjuvant chemotherapy have been shown to vary between both PAM50-28,40 and Prosigna102-defined intrinsic subtypes. Response rates have been reported to be lower for luminal A than for luminal B tumours and both luminal subtypes are less chemotherapy sensitive than non-luminal subtypes. Additionally, Prosigna ROR scores have been shown to be predictive of chemotherapy response. 102 Three studies have explored the ability of PAM50 to predict outcome in trials comparing two chemotherapy regimens, two of which were conducted in early breast cancer103,104 and one in advanced disease. 105 None of the three studies selected patients by receptor expression, so the numbers of patients with luminal disease analysed was comparatively small. None of the studies showed an overall statistically significant benefit for patients with luminal B versus luminal A disease. One of the adjuvant studies compared an anthracycline containing regimen with CMF with an overall positive result. 103 The second adjuvant study, which was also positive, showed that patients with luminal A breast cancers appeared to benefit from paclitaxel treatment, unlike those with higher-risk subtypes,104 which is a counterintuitive finding. In the study conducted in advanced disease,105 which showed no overall benefit for the addition of gemcitabine to docetaxel, there appeared to be a significant benefit for patients with luminal B versus luminal A disease, which was lost in multivariate analysis.
Mammostrat®
Derived following expression array analysis identifying markers of residual risk in early breast cancer, the Mammostrat® assay (Clarient/GE Healthcare, Aliso Viejo, CA, USA) relies on immunohistochemical analysis of five markers (p53, NDRG1, SLC7A5, CEACAM5 and HTF9C). The test classifies patients into three risk groups. First described in 2006,53 this assay was validated across multiple retrospective institutional and clinical trial cohorts, including the NSABP B-14 and NSABP B-20 trials, and the TEAM trial. 53,106,107 One health economic analysis suggests that the test is cost-effective in US health care. 108
Mammostrat was developed by Clarient and, following US Food and Drug Administration approval in 2007, the assay was commercialised in the USA as a marker of residual risk in early breast cancer. GE Healthcare acquired Clarient in 2010. Clarient/GE Healthcare discontinued development of the test in 2014. Although still commercially available in the USA, its future is uncertain.
MammaTyper®
This is a four-gene quantitative reverse transcriptase-PCR expression assay being developed commercially by BioNTech Diagnostics GmbH. The assay measures ER, PgR, HER2 and Ki67 mRNA. 60 These data are combined to allocate tumours to an intrinsic subtype rather than provide a risk score as IHC4. The definition of intrinsic subtype is based on an immunohistochemical definition, which does not map accurately onto PAM50/Prosigna defined subtypes. 99 Formal clinical validation studies of the assay are currently being conducted.
Comparative performance of multiparameter assays
Very limited information on direct comparisons between multiparameter assays is available. Comparisons have been performed between Oncotype DX, IHC4, Prosigna and BCI results from approximately 1000 post-menopausal patients treated with endocrine therapy from the transATAC cohort. 43,55,56,75 These have focused on high-level statistical analysis of the prognostic value of different tests within this population. Information on the proportion of patients testing ‘positive’ (i.e. high risk) for individual tests is more opaque and, in particular, data on results at the individual patient level are lacking. Nonetheless these comparisons have been of substantial value in allowing comparisons between tests, albeit limited to comparing their overall prognostic impact. Taken collectively, the results of these pivotal studies support the conclusion that all four assays provide similar prognostic information at the population level. They also provide evidence that Prosigna and BCI provide statistically more prognostic information than Oncotype DX and IHC4, and in contrast to Oncotype DX and IHC4, they provide information about the risk of late (beyond 5 years) relapse. No conventional parameters of performance (e.g. area under the receiver operator curve) have been presented at any time for any of the currently available assays, which makes it difficult to evaluate their performance in this regard. However, the statistical improvements in performance for Prosigna and BCI are modest, but important, leaving space for further improvement.
An alternative approach is to use published assay algorithms, or to ‘reverse engineer’ assays, and to apply these to gene expression data sets, with associated outcome data as an ‘in silico’ experiment. 109,110 Such comparisons have, like the direct comparisons, shown that the results of the various multiparameter assays are moderately correlated with respect to the prognostic information provided. The major limitations of this methodology are, first, that different array platforms lack probes that match to prognostic signatures, such that ‘missing genes’ may compromise analysis, and, second, that the algorithms used by the assays are calibrated according to the method used to measure gene expression and may not translate well into semiquantitative analysis of microarray data sets used for this analyses. Signatures compared, in a similar manner using arrays and reverse engineering, in the I-SPY 1 trial include MammaPrint and PAM50. 40 There was again a modest correlation between assay performance, and most provide predictive information about response to neoadjuvant chemotherapy and outcome. Once again, the analysis of I-SPY 1 provided no information on the comparative performance of assays at patient level.
Cost-effectiveness analyses of multiparameter testing
We performed a systematic review of literature on the cost-effectiveness of multiparameter testing; the methods are described in Appendix 1. Six studies assessed the impact of Oncotype DX (one in each of Canada,111 the USA,112 the UK,74 Germany,113 Australia114 and Japan115), and one assessed the impact of MammaPrint in the Netherlands. 116 No head-to-head comparisons of the alternative genomic tests have been conducted. Four studies74,112,113 either were funded by the test manufacturer or were carried out by researchers who worked for the manufacturer. 114 All studies compared the given genomic test with current conventional management, which consisted of current national guidelines and tools based on patient clinicopathological data (such as Adjuvant! Online,17 PREDICT18 and the NPI19).
The majority of studies considered mixed populations, including patients with both lymph node-negative and lymph node-positive disease. 74,113,115,116 Two studies112,113 assessed populations that included only patients with lymph node-positive disease, both of which evaluated Oncotype DX. Four studies reported specific details of patients’ level of lymph node involvement;112,113,115,116 most restricted their populations to patients with low levels (1–3) of lymph node involvement, with only one study111 including patients with higher (1–9) lymph node involvement.
The single MammaPrint study estimated that the test would lead to a 0.21 decrease in quality-adjusted life-years (QALYs) together with a US$2882 fall in costs, from a Netherlands societal perspective. 116
Of the six studies assessing Oncotype DX,74,111,115 two estimated that the test would dominate standard care strategies, with Oncotype DX found to produce a gain in QALYs and a reduction in costs. 112,113 Both of these studies were funded by the manufacturer. Vanderlaan et al. 112 found that, from a health-care payer perspective and using a lifetime horizon, Oncotype DX was estimated to produce a gain of 0.17 QALYS per patient and reduce costs by US$384 (based on 2009 costs). Eiermann et al. 113 found that, from a German health-care payer perspective and over 30 years, Oncotype DX was estimated to produce a gain of 0.06 QALYS and reduce costs by €561 per patient.
The remaining four studies74,111,114,115 found Oncotype DX to be more costly and more effective than standard care. The highest incremental cost-effectiveness ratio (ICER) value was estimated in the only study that included patients with high levels of lymph node involvement (1–9 positive nodes). Lamond et al. 111 found that, from a Canadian third-party payer perspective and over a lifetime horizon, Oncotype DX was estimated to produce a 0.06 QALY gain at an incremental cost of CA$14,844 (based on 2011 costs) [95% confidence interval (CI) CA$3616 to CA$25,646] per QALY. They found that there was almost a 100% probability of Oncotype DX-guided treatment being cost-effective, but that the results for the lymph node-positive group were more sensitive to one-way sensitivity analyses than the results in a lymph node-negative population. For the remaining three studies (which assessed mixed lymph node populations), the ICER results were £6232/QALY,74 JP¥568,533/QALY (equivalent to US$5685)115 and AUD$9986/QALY. 116
In addition to these studies, two studies15,49 have considered the likely cost-effectiveness of multiparameter assays for use in the NHS. Hall et al.,15 who performed a cost–utility analysis of Oncotype DX in a node-positive population by modelling outcome data from the SWOG88-14 Oncotype DX study62 to the NHS, concluded that Oncotype DX is unlikely to be cost-effective for this population. Ward et al. ,49 who undertook the external evidence review for the NICE DG10 guidance117 in a node-negative population, concluded that Oncotype DX could be cost-effective if offered to a higher-risk subset of such patients. They considered that MammaPrint and IHC4 may also be cost-effective, but the evidence was not sufficiently robust to draw a firm conclusion, while limited evidence suggested that Mammostrat is unlikely to be cost-effective.
The literature review also identified two budget impact analyses,118,119 both of which were funded by or affiliated with the manufacturer of the test. Ragaz et al. 118 assessed the impact of Oncotype DX on US and Canadian health-care costs in both lymph node-positive and lymph node-negative patients, and estimated that Oncotype DX could save US$330.8M in the USA and US$46.2M in Canada. Zarca et al. 119 assessed the impact of MammaPrint on health-care costs in France on node-positive (1–3) breast cancer patients, and estimated that MammaPrint could save €9043 per 100 patients per year compared with current practice.
Availability of multiparameter testing in the UK
Four multiparameter assays have been evaluated by NICE (Oncotype DX, MammaPrint, IHC4 and Mammostrat) for potential use in the NHS. The resulting guidance117 was published in September 2013. The guidance states that Oncotype DX ‘is recommended as an option for guiding adjuvant chemotherapy decisions for people with ER-positive, lymph node-negative and HER2-negative early breast cancer’. The recommendation was limited to patients at ‘intermediate risk’, defined as a NPI score of > 3.4, and relies on a discounted price for Oncotype DX. The guidance and resulting funding decisions apply only to England. The three other technologies evaluated were recommended for use only in research at this time. Five additional technologies, including PAM50, BCI and NPI+, were removed from the scope in November 2011, almost 2 years before the final guidance was published, on the grounds that at that time there was insufficient evidence to justify their inclusion in the economic analysis performed by the external assessment group. 120 The appraisal was also limited to patients with node-negative disease, as the evidence for the use of the tests was considered less robust in the node-positive population. The NICE committee recommendation for the use of Oncotype DX was made on the basis that the test provides superior prognostic information to conventional clinicopathological risk assessment. The committee, however, did not consider the evidence that Oncotype DX is able to predict the benefit of chemotherapy above and beyond providing prognostic information to be robust and recommended further research into this question for all four technologies.
The OPTIMA study
There are a number of important unanswered questions for clinicians and patients on the practical use of multiparameter assays in making decisions about chemotherapy. These include whether or not tests might be useful for patients with axillary node involvement, how best to use the tests, what threshold to use where this is not clearly defined and which of the available tests provides the most reliable results. Three ongoing international randomised controlled trials (RCTs) will generate prospective evidence for the validity of test-directed treatment assignment. 121–123
-
Trial Assigning IndividuaLized Options for Treatment (Rx) (TAILORx). 121 This US-based intergroup trial randomised patients to chemotherapy followed by endocrine therapy, or endocrine therapy alone based on an Oncotype DX test result. Eligible patients have ER-positive breast cancer without nodal involvement. All patients undergo Oncotype DX, and those with a RS in the range 11–25 are eligible for randomisation. Recruitment was suspended in October 2010, when 10,000 (out of a target of 11,000) patients had been enrolled. The primary analysis is expected in 2017. The majority of patients randomised in TAILORx would not currently be offered chemotherapy in the UK.
-
Microarray In Node-negative and 1- to 3-positive lymph node Disease may Avoid ChemoTherapy – European Organisation for Research and Treatment of Cancer Trial 1004 (MINDACT – EORTC 1004). 122 This pan-European trial is comparing adjuvant chemotherapy treatment decisions based on genetic profiling using the MammaPrint test run on fresh tissue, with decisions based on clinical parameters. Eligible patients can have up to three involved axillary lymph nodes. The MINDACT study has met its recruitment target of 6000 patients; the primary analysis is planned to take place in 2016.
-
Rx for Positive Node, Endocrine Responsive Breast Cancer (RxPONDER). 123 This is a US-based intergroup study that opened to recruitment in 2011. Eligible patients will have ER-positive, HER2-negative tumours with one to three involved axillary lymph nodes. All patients will undergo Oncotype DX testing prior to trial entry. Patients with a RS of ≤ 25 are eligible to be randomised between chemotherapy followed by endocrine therapy or endocrine therapy alone. The trial aims to test over 10,000 patients and to randomise 4500. The primary analysis is currently intended to take place in 2022.
The lack of comparative data with other multiparameter tests means that it is possible that other existing tests may allow more reliable identification of chemotherapy-sensitive disease than Oncotype DX or MammaPrint. Although NICE has recommended that Oncotype DX may be used to guide adjuvant chemotherapy decisions, it may not be the most cost-effective platform for making test-directed chemotherapy decisions in the NHS.
The OPTIMA trial seeks to advance the development of personalised treatment in breast cancer by establishing an appropriate and effective method of multiparameter analysis, to identify which women with ER-positive HER2-negative, lymph node-positive primary breast cancer are likely to benefit from chemotherapy, and which are not. It is axiomatic to the study that the benefit of chemotherapy is not evenly distributed among breast cancer subpopulations, contrary to the assumption made by NICE in DG10,117 and that the multiparameter assays are able to predict chemotherapy sensitivity. The potential ability of multiparameter assays to predict chemotherapy sensitivity was recommended as a topic for future research in the DG10 guidance. The study population is similar to that included in the NICE appraisal in respect of ER and HER2 status, but at higher risk, so would ordinarily be offered chemotherapy by virtue of either axillary lymph node involvement or because other adverse clinicopathological features; the majority of such patients would not be eligible for NHS-funded Oncotype DX testing. The OPTIMA study is an adaptive trial that allows more than one technology to be evaluated. It is planned to run in two phases with an initial feasibility study, now completed, to compare the performance of technologies in order to establish which of these will be included in the main trial, and to evaluate the acceptability of the approach to patients and its cost-effectiveness. The main study will use an end point of non-inferiority after 5 years because events often occur late in breast cancer and no intermediate end point that allows early prediction of recurrence has yet been identified.
OPTIMA prelim (the feasibility phase of OPTIMA) was funded by the Health Technology Assessment (HTA) programme of the National Institute for Health Research (NIHR) in 2012 (reference 10/34/01) and completed the primary phase recruitment on 3 June 2014. The main objectives of OPTIMA prelim were to demonstrate that recruitment to a large-scale randomised trial of test-directed chemotherapy is feasible in the UK and to select a multiparameter assay to use as the primary treatment discriminator in the main OPTIMA study. This report describes the methodology used in OPTIMA prelim and the results of the study.
Chapter 2 Methods: recruitment and study conduct
Trial design
The OPTIMA trial is a multicentre, partially blind randomised clinical trial with a non-inferiority end point and an adaptive design. The preliminary or feasibility phase of the study, which has the same structure as the main trial, is referred to as OPTIMA prelim (Figure 1).
FIGURE 1.
The OPTIMA prelim trial schema. FISH, fluorescence in situ hybridisation; non-amp, non-amplified; pT, pathological tumour size. Note that pN refers to the pathologically determined nodal status; pN1 represents 1–3 positive nodes; pN2 represents 4–9 positive lymph nodes.

OPTIMA prelim aimed to establish whether or not a large trial of multiparameter test-based treatment allocation (‘test-directed’ treatment) is acceptable to patients and clinicians, with 300 patients randomised in a 1 : 1 ratio. A 200-patient extension phase was built into the design of OPTIMA prelim to allow a smooth roll through to the main trial. A second key aim of the study was to compare the performance of alternate multiparameter tests to allow the selection of multiparameter tests to be evaluated in the main trial.
OPTIMA trial compares the standard treatment of chemotherapy followed by endocrine therapy with multiparameter test-directed treatment allocation to either chemotherapy followed by endocrine therapy or endocrine therapy alone. The randomisation of patients allocated to chemotherapy is concealed from treating centres. In the main trial, it is expected that approximately 1860 patients will be randomised to each arm in a two- or three-arm design (with either one or two test arms). Patients will be followed up for 10 years.
The OPTIMA trial is adaptive with respect to the technology used to make a chemotherapy treatment assignment. The technology used in the OPTIMA prelim was Oncotype DX, which was chosen as the best-validated test when the trial was designed. A RS cut-off point of > 25 versus ≤ 25 was selected as the threshold for allocation of patients to chemotherapy or to no chemotherapy. To avoid any potential confusion in the use of nomenclature, the two groups defined by this cut-off point are referred to either by RS value or as RS low or high score. The value of 25 was chosen (1) as it is the mid-point of the predefined intermediate-risk range (RS of 18–31), (2) because from the retrospective analyses of the NSABP B-20 and SWOG88-14 studies,61,62 this is roughly the level at which there appears to be a clinically meaningful benefit for adjuvant chemotherapy, and (3) because it is the upper limit at which randomisation is permitted in the ongoing TAILORx and RxPONDER trials. 123,124
Trial objectives
The OPTIMA prelim objectives were:
-
to evaluate the performance and health economics of alternative multiparameter tests to determine which will be evaluated in the main trial
-
to establish the acceptability to patients and clinicians of randomisation to test-directed treatment assignment
-
to establish efficient and timely sample collection and analysis, which is essential to the delivery of multiparameter test-driven treatment.
The OPTIMA main trial objectives are:
-
to evaluate whether or not test-directed chemotherapy treatment and endocrine therapy is non-inferior to chemotherapy followed by endocrine treatment for all patients in terms of invasive disease-free survival (IDFS).
-
to establish the cost-effectiveness of a test-guided treatment strategy compared with standard practice.
OPTIMA prelim success criteria
The prespecified criteria used to determine the success of OPTIMA prelim were recruitment of 300 patients no more than 2 years from the first centre opening to recruitment, and, for the final 150 patients:
-
patient acceptance rate should be at least 40%
-
recruitment should take no longer than 6 months
-
chemotherapy should start within 6 weeks of signing the OPTIMA prelim consent form for no less than 85% of chemotherapy-assigned patients.
Outcome measures
OPTIMA prelim
Patient acceptability
-
Time between consent and starting chemotherapy.
-
Recruitment time.
-
Agreement between tests.
The OPTIMA main trial
Primary outcomes
-
IDFS.
-
Costs and QALY.
Secondary outcomes
-
Quality of life as measured by the European Quality of Life 5-Dimesions (EQ-5D)125 and Functional Assessment of Cancer Therapy (FACT) questionnaire for patients with breast cancer. 126,127
-
Distant disease-free survival.
-
Comparative performance of multiparameter assays (if more than one adopted).
-
Patient compliance to long-term endocrine therapy.
-
Overall survival.
Eligibility criteria
Inclusion criteria
-
Female, aged ≥ 40 years.
-
Excised invasive breast cancer with local treatment either completed or planned according to trial guidelines.
-
ER-positive [Allred score of ≥ 3 or histoscore (H-score) of ≥ 10 or as otherwise established by the reporting pathologist] as determined by the referring centre and centrally confirmed.
-
HER2-negative (i.e. immunohistochemistry score of 0–1+) or fluorescence in situ hybridisation (FISH) or other in situ hybridisation non-amplified, as determined by the referring centre and centrally confirmed.
-
Axillary lymph node status: (1) 1–9 involved (macrometastases, i.e. > 2 mm OR micrometastases, i.e. > 0.2–2 mm) OR (2) node negative AND tumour size ≥ 30 mm. Nodes containing isolated tumour cell clusters only, that is ≤ 0.2 mm in diameter, were considered to be uninvolved.
-
Considered appropriate for adjuvant chemotherapy by treating physician.
-
Patient assessed as fit to receive chemotherapy and other trial-specified treatments with no concomitant medical, psychiatric or social problems that might interfere with informed consent, treatment compliance or follow-up.
-
Bilateral and multiple ipsilateral cancers were permitted provided at least one tumour fulfils the entry criteria and none meet any of the exclusion criteria. Patients with bilateral tumours where both tumours fulfil all eligibility criteria including size and nodal status were excluded. Note that for separate synchronous primary cancers, whether ipsilateral or bilateral, it was anticipated that laboratories would, as per standard good practice, assess ER and HER2 on the different lesions. Centres were requested to send a block for each separately reported tumour for central eligibility testing provided sufficient material was available. If there were multiple invasive foci deemed to derive from one main cancer (satellite foci), which had the same histological features including, for example tumour type and grade, it was not required that every focus has its receptor status reassessed.
-
Written informed consent for the study.
Exclusion criteria
-
Ten or more involved axillary nodes or involved internal mammary nodes.
-
ER negative OR HER2 positive/amplified tumour on central eligibility testing.
-
Metastatic disease. Note that formal staging according to local protocol was recommended for patients where there was a clinical suspicion of metastatic disease or for stage III disease (tumour > 50 mm with any nodal involvement OR any tumour size with four or more involved nodes).
-
Previous diagnosis of malignancy unless:
-
managed by surgical treatment only and disease free for 10 years
-
previous basal cell carcinoma of skin, cervical intraepithelial neoplasia or ductal carcinoma in situ of the breast treated with surgery only.
-
-
The use of oestrogen replacement therapy (HRT) at the time of surgery. Patients who were taking HRT at the time of diagnosis were eligible provided the HRT was stopped before surgery.
-
Presurgical chemotherapy, endocrine therapy or radiotherapy for breast cancer. Treatment with endocrine agents known to be active in breast cancer including ovarian suppression was permitted provided this was completed > 1 year prior to study entry.
-
Commencement of adjuvant treatment prior to trial entry. Short-term endocrine therapy initiated because of, for instance, prolonged recovery from surgery was permitted but had to be discontinued at trial entry.
-
Trial entry more than 8 weeks after completion of breast cancer surgery.
-
Planned further surgery for breast cancer, including axillary surgery, to take place after randomisation, except either re-excision or completion mastectomy for close or positive/involved margins, which could be undertaken following completion of chemotherapy.
-
Patients with more than two involved axillary nodes (as defined in the inclusion criteria) identified by sentinel node biopsy or by axillary sampling where further axillary surgery was not planned.
Study conduct
Ethical and regulatory approval
The South East Coast–Surrey Research Ethics Committee approved the trial on 22 June 2012. Local research and development department approval was obtained at each participating NHS trust prior to patients being enrolled into the trial. The trial was conducted in accordance with the principles and guidelines of the International Conference on Harmonisation, Good Clinical Practice, UK legislation, Warwick Clinical Trials Unit (CTU) standard operating procedures and the Research Ethics Committee approved protocol. The trial is registered as Current Controlled Trials ISRCTN42400492.
Management of the trial
The Trial Steering Committee (TSC) provided overall supervision of the trial, with an independent chairperson and majority of independent members. The Trial Management Group (TMG) comprised a multidisciplinary team of clinicians, statisticians, a translational scientist and a patient advocate, all with considerable expertise in all aspects of trial design, running, quality assurance and analysis. The Data Monitoring and Ethics Committee carried out the independent monitoring of the trial. Details of the membership of the TSC, TMG and Data Monitoring and Ethics Committee can be found in the Acknowledgements (see Acknowledgements and Contributors).
Trial centres
A total of 35 hospitals from 31 NHS trusts and health boards in England, Scotland and Wales participated in OPTIMA prelim. As the demonstration of recruitment feasibility was a major end point for OPTIMA prelim, it was important to ensure that trial centres were broadly representative of the UK. Rather than issue a general invitation to participate in the study, individual centres were approached in five main geographical clusters: Scotland, the West Midlands, East Anglia, North London and South West/South Wales. Both district general hospitals and cancer centres were included. A list of all participating centres can be found in the Acknowledgements (see Acknowledgements and Contributors). Prior to activating a centre to recruitment, a trial initiation meeting was held, in person or via teleconference, to provide study-specific training to all staff members working on the trial. Continued support was offered to existing and new staff at participating centres to ensure that they remained fully aware of trial procedures and requirements.
Patient information and informed consent
Patients who were potentially eligible for participation in the study according to the criteria (see Eligibility criteria) were identified at the multidisciplinary breast cancer meetings held at each of the centres. Patients were invited to participate in the OPTIMA prelim study during the initial consultation with their oncologist, where treatment options were discussed. Here the local principal investigator or their designee discussed the trial with the patient and provided the patient with a copy of the patient information sheet (PIS) (see Appendix 2). In many cases a research professional would be present in this consultation and/or meet with the patient afterwards to continue discussing the trial. Patients were given sufficient time to consider participation in the trial and subsequently the opportunity to ask any further questions and to be satisfied with the responses. Patients who were willing to participate were asked to provide written informed consent (see Appendix 2 for a copy of the consent form). Consent for the study included consent for use of the participant’s tissue samples, removed at the time of surgery, as part of the OPTIMA prelim. Participants were also asked to donate the remainder of their samples for future unspecified research projects; however, this was optional.
Randomisation procedure
Trial entry was defined as the time consent was given, which started the randomisation procedure. Once written informed consent had been given and chemotherapy selected from the regimens permitted in the protocol (Table 3), the researcher registered the patient with the CTU. During registration, eligibility was confirmed according to the results of local pathology testing and the patient was allocated a unique participant registration number.
Regimen | Drugs | Dose | Cycle schedule |
---|---|---|---|
FEC75–80 | Fluorouracil | 500–600 mg/m2 | i.v. q. 3 weeks × 6 cycles |
Epirubicin | 75–80 mg/m2 | ||
Cyclophosphamide | 500– 600 mg/m2 | ||
FEC90–100 | Fluorouracil | 500 mg/m2 | i.v. q. 3 weeks × 6 cycles |
Epirubicin | 90–100 mg/m2 | ||
Cyclophosphamide | 500 mg/m2 | ||
FEC-Pw | FEC100 (as above) | i.v. q. 3 weeks × 3–4 cycles | |
Followed by paclitaxel | 80–90 mg/m2 | i.v. q. 1 week × 9–12 cycles | |
TC | Docetaxel | 75 mg/m2 | i.v. q. 3 weeks × 4–6 cycles |
Cyclophosphamide | 600 mg/m2 | ||
FEC-T | FEC100 (as above) | i.v. q. 3 weeks × 3 cycles | |
Followed by docetaxel | 100 mg/m2 | i.v. q. 3 weeks × 3 cycles | |
E-CMF | Epirubicin | 100 mg/m2 | i.v. q. 3 weeks × 4 cycles |
Followed by cyclophosphamide | 600 mg/m2 OR 100 mg/m2 p.o. daily × 14 days | i.v. D1, 8 q. 4 weeks × 4 cycles | |
Methotrexate | 40 mg/m2 | ||
Fluorouracil | 600 mg/m2 |
Once the participant was registered, the centre sent relevant tumour block(s) from the surgical resection to the central laboratory for confirmatory testing of ER and HER2 status as soon as possible.
In order to avoid unnecessary delays in the randomisation procedure, on receipt of the tumour block, the central laboratory prepared samples for Genomic Health in parallel with undertaking eligibility testing. The central laboratory informed the CTU of the patient’s ER and HER2 status, and those eligible were randomised in a 1 : 1 ratio to standard treatment (control arm) or to test-directed treatment. Randomisation was performed centrally by a computer using a minimisation algorithm stratified to ensure balance across trial arms by chemotherapy regimen: anthracycline–non-taxane [FEC75–80, FEC90–100, epirubicin, cyclophosphamide, methotrexate and fluorouracil (E-CMF)] vs. taxane–non-anthracycline [docetaxel and cyclophosphamide (TC)] vs. combined anthracycline and taxane [FEC-T, fluorouracil, epirubicin, cyclophosphamide and paclitaxel (FEC-Pw)], number of involved nodes (none vs. positive sentinel node biopsy without axillary surgery vs. 1–3 vs. 4–9 nodes), menopausal status (pre-/perimenopausal vs. post-menopausal). Oncotype DX testing proceeded immediately for all participants [tumour blocks from participants randomised to the control arm underwent Oncotype DX testing as part of the pathology study (see Chapter 3, Pathology research in the preliminary studies)]. Genomic Health reported the test result to the CTU, which subsequently informed the research centre, by fax and e-mail, whether or not the participant was to receive chemotherapy according to randomisation and RS for those randomised to test-directed treatment. It was anticipated that the randomisation procedure, from date of consent/registration to treatment assignment, would take between 3 and 4 weeks.
Blinding
OPTIMA prelim was a partially blinded trial. For participants who were allocated to receive endocrine therapy alone, it was not possible to blind either the participant or the clinicians at the centre. However, for participants allocated to receive chemotherapy followed by endocrine therapy, the participant and the centres were blind to the participant’s randomisation. In order to maintain blinding, for participants randomised to the standard arm, the CTU delayed informing the treating centre of the treatment allocation by a time period equivalent to that taken to perform the Oncotype DX test for those randomised to test-directed treatment. In practice this was on receipt of the Oncotype Dx test result as this was performed for all participants in OPTIMA prelim irrespective of randomisation. To assess the success of the OPTIMA prelim and perform a comparison of multiparameter tests, the randomised treatment allocation remained blinded to the analyst, as it was not needed in the analysis of the OPTIMA prelim.
Trial treatment
With the exception of mandating whether or not a participant received chemotherapy, treatment pathways for patients joining the OPTIMA prelim study were designed to be as similar as possible to those for equivalent patients who were either ineligible or chose not to join the study. The process of consent and subsequent Oncotype DX testing was expected to delay the start of systemic treatment (with either chemotherapy or endocrine therapy) by about 3 weeks compared with non-trial-treated patients. This delay is not expected to be clinically deleterious. 128 Although the protocol contained recommendations for the management of all aspects of patient treatment based on national/international guidelines (where available) and consensus views on best practice, the expectation was that participating centres already follow these and, therefore, that treatment would follow the local implementation of best practice and national guidelines.
Chemotherapy
Chemotherapy was chosen at the time of registration from the list of protocol-permitted regimens (see Table 3). The regimens included in the list were selected from those with good evidence for efficacy and which are widely used in the NHS.
Endocrine therapy
The OPTIMA prelim protocol specified that endocrine therapy should be started no later than 2 weeks after treatment allocation in participants assigned to receive no chemotherapy and 4 weeks after the final dose of chemotherapy for all other participants. Concomitant endocrine therapy and chemotherapy was not permitted in the OPTIMA prelim. It was also not permitted for endocrine therapy to be delayed until after radiotherapy. Permitted endocrine therapy for participants in the trial was:
-
post-menopausal at trial entry: AI (anastrozole, letrozole, exemestane permitted)
-
premenopausal at trial entry: tamoxifen for 5 years and ovarian suppression with either
-
a gonadotropin-releasing hormone agonist for a minimum of 3 years or
-
bilateral surgical oophorectomy (radiation menopause was not permitted).
-
Within the OPTIMA prelim study ovarian suppression was recommended for all premenopausal women (1) to ensure that the patients within both arms received equally balanced endocrine treatment and (2) to eliminate the risk of confounding from different rates of chemotherapy induced menopause between the arms.
Surgery
All participants received appropriate surgery, performed according to local guidelines. Primary surgery consisted of a wide local excision or mastectomy. If breast conservation surgery was undertaken, then the acceptable circumferential and deep/superficial margin widths were determined by local protocol. If required, re-excision for clear margins was permitted to take place before or after chemotherapy.
All participants underwent preoperative axillary staging with an ultrasound scan and needle biopsy or fine-needle aspiration of any suspicious or indeterminate nodes. Participants with preoperative pathologically proven axillary lymph node involvement underwent axillary clearance. Participants with involved axillary lymph nodes identified at sentinel node biopsy (including macrometastases, micrometastases, and isolated tumour cell clusters) received further management according to local protocol. Centres could choose to avoid axillary clearance following a positive sentinel node biopsy for participants who had undergone breast-conserving surgery and who fulfilled the following criteria:
-
no palpable nodes
-
no more than two involved nodes
-
clinical tumour size T1–T2 (≤ 5 cm).
All planned axillary surgery was completed prior to randomisation to allow for stratification by the extent of axillary involvement.
Radiotherapy
Radiotherapy was given in accordance with local guidelines. The protocol stated the following recommended best clinical care as a reference for centres.
-
Breast radiotherapy was required for all patients who had undergone breast-conserving surgery. Whole breast, including the primary tumour bed, was the target volume. Centres could give a tumour bed boost in conjunction with whole-breast radiotherapy as per local guidelines. Partial breast radiotherapy was permitted, but only for patients who had a negative sentinel node biopsy or a full axillary clearance.
-
Participants who had undergone a mastectomy were required to receive chest wall radiotherapy if they had four or more positive axillary nodes or T3 tumours with any node positivity. Chest wall radiotherapy was recommended for tumours with a positive deep margin. Centres could also consider chest wall radiotherapy for patients with 1–3 positive axillary nodes or high-risk node-negative disease. The chest wall was the target volume.
-
Treatment of the supraclavicular fossa was required when four or more axillary lymph nodes were involved and could be given according to local guidelines for patients with 1–3 involved axillary nodes. Axillary radiotherapy, in addition to breast radiotherapy, was permitted using a four-field technique, when patients with up to two involved sentinel nodes did not undergo clearance. The axilla was not otherwise routinely irradiated. Internal mammary nodes were not routinely irradiated.
Recommended schedules after breast-conserving surgery or mastectomy were:
-
40 Gy in 15 fractions, five fractions per week
-
50 Gy in 25 fractions, five fractions per week
-
45 Gy in 20 fractions, five fractions per week.
Dose fractionation for tumour bed boost and regional lymph nodes was given according to local protocol.
Screening information
In order to assess patient acceptability, each participating centre maintained a screening log to document all patients considered for the trial but subsequently excluded. Where possible, the reason for non-entry to the trial was documented. Screening logs were requested by the CTU on a monthly basis. No patient-identifiable data were recorded on the screening log.
Data collection
Clinical and resource use data generated by all centres were collected on study case report forms (CRFs), which were monitored and entered into the trial database by the CTU. The participant’s details were entered onto the local centre’s patient identification log; at no time was this log forwarded to the CTU. To preserve participants’ anonymity, only their allocated trial number and initials were used on the CRFs and any correspondence. With participants’ permission, their name, date of birth, address and NHS/Community Health Index number were also provided to the CTU on the registration CRF to allow flagging with the Office for National Statistics. Participants’ confidentiality was respected at all times.
Case report form completion guidelines were provided to all centres to aid consistency of completion. Data management and monitoring were conducted in accordance with the CTU standard operating procedures, which are designed to ensure that trial data are as complete and accurate as possible, and comply with the Data Protection Act 1998. 129 Data management practice included verification, database validation and formal data checking following data entry. All missing and ambiguous data were pursued until resolved or confirmed as unavailable. Monthly reminders were sent to participating centres in order to flag any forthcoming or outstanding clinical visits or questionnaires.
Quality of life and health resource use assessment
The patient questionnaire was designed to assess quality of life and health resource use. The baseline questionnaire was provided by the centre and completed by participants after they had given written informed consent but before randomisation. Further questionnaires were administered at 3, 6, 12 and 24 months from date of consent. These further questionnaires were either given to participants at a clinic appointment or sent by post. The OPTIMA prelim study used two instruments to gather information on quality of life as the basis for evaluating both effectiveness and cost-effectiveness: EQ-5D124 and the FACT questionnaire comprising FACT – General126 and FACT – Breast cancer. 127
Follow-up
Participants are followed up annually for 10 years from trial entry. Annual follow-up appointments could be conducted in the clinic or via telephone for participants who had been discharged from clinical review. The method of follow-up is recorded on the CRF.
Withdrawals
Participants had the right to withdraw from the trial at any time and for any reason. Those participants who were deemed ineligible by central review were not randomised and did not count towards the sample size. Participants who were registered but not randomised were not classified as withdrawals. Owing to the cost, the multiparameter assay was cancelled for those who withdrew consent post randomisation but prior to treatment allocation. For the purposes of the preliminary study, randomised participants who had not yet been allocated treatment and for whom an Oncotype DX result was not obtainable were not followed up. Participants who declined to comply with their trial-allocated treatment remained on-study and are followed up in accordance with the protocol.
Analysis of the OPTIMA prelim success criteria
The acceptability of the trial was assessed as the proportion of eligible patients consenting to participate in the OPTIMA prelim. The characteristics of the patients for whom the trial and the concept of test-driven therapy were acceptable were provided. The time from signing the OPTIMA prelim consent form to starting chemotherapy was calculated and the proportion of chemotherapy-assigned patients starting treatment within 6 weeks was determined. Compliance with the test-directed treatment decision was assessed through the proportion of patients choosing not to follow the result of the randomisation.
Decision to continue to the main trial
There were no formal stopping rules within OPTIMA prelim, as the ability to continue to the main trial was dependent on a combination of patient acceptability, recruitment, number of alternative tests to consider and cost-effectiveness. The decision on whether or not the main trial is feasible was dependent on meeting the predefined success criteria for the study (see OPTIMA prelim success criteria).
Involving patients in developing a study
Recruitment into the OPTIMA trial was anticipated to be challenging because women who would normally be offered immediate chemotherapy after surgery were to be asked to consider the possibility of not receiving chemotherapy on the basis of a test. As reported elsewhere, trials with control, placebo or ‘no’/’yes’ treatment arms can be inherently difficult to recruit. 130–133 Although the OPTIMA study is slightly different, in that a personal genomic test will dictate the need for chemotherapy, it is not known how this may influence perceptions of the study and whether, or how much, this will impact recruitment. Patient and public involvement (PPI) is, therefore, integral to the design of the OPTIMA study in order to understand the possible opportunities and problems that this might present from the perspective of people with cancer. It is planned that data and insight from PPI will allow for ongoing protocol amendments, and advice and training to be disseminated to recruiting staff so that appropriate information can be given to potential participants. This will prevent recruitment being compromised by misunderstanding or inappropriate concerns from either staff or patients.
Three complementary stages of PPI have taken place during OPTIMA prelim, consisting of:
-
engagement as a co-investigator and consequent contribution to the study design, and information by a member of a patient advocacy group, Independent Cancer Patients’ Voice (ICPV)
-
representation of the same patient advocate on the TMG with full participation in the review of trial conduct and progress
-
focus groups with people who have or have had cancer about their perspectives on being offered participation in the OPTIMA trial or a similar study potentially offering less treatment than expected.
Focus group design
Three focus groups were held for people who had or were living after cancer. These were held in Coventry in August 2012, in Cambridge in February 2013 and Guildford in April 2013. At least two members of the OPTIMA trial team were in attendance to offer factual advice about the trial and to give administrative support,134 but their contribution was kept to a minimum to allow free discussion at each focus group. The focus groups were facilitated by trained facilitators and discussions were moderated independently by members of the charity ICPV, of whom one or two attended each group. Participants were people who lived locally and had been invited via a support group they attended or through ICPV. Twelve self-selecting participants attended each focus group. The majority were women with primary breast cancer, but the group in Guildford was made up of six women living after breast cancer and six people with other cancers, including four men. Each focus group was audio-recorded to ensure that data were as complete and reliable as possible, and a member of the study team took notes of the proceedings and main areas of discussion.
Potential focus group participants were given a précis of the trial protocol, copies of information given to potential OPTIMA prelim trial participants (the PIS) and details of the focus group aims. Additionally, an information leaflet about taking part in the focus group was provided for participants to read before each meeting, and to advise them that they would be asked for consent to take part in the study and have their contribution recorded if they attended. Each focus group meeting started with introductions and a presentation of the trial with an opportunity to ask questions. Following this, the intended structure of the meeting was explained. Ground rules were suggested by the study team members and agreed at each meeting by attendees (Table 4). Participants were also asked for written consent to take part in the focus group and for the focus group to be recorded; all participants gave consent. It was made clear that documentation would not contain anything that would make individuals identifiable and that names should not be used during the focus group to keep data reporting as confidential as possible.
Rule number | Rules of engagement |
---|---|
1 | Everyone has the right to say what they want to say without interruption |
2 | Everyone’s opinion and point of view will be respected |
3 | No identifiable information will leave the room (except recorded data and only personal identification by the speaker will be permitted) |
Each group then proceeded with an invitation for each member to give a brief introduction of why they had attended the focus group and any other information that they felt was pertinent to the group. The majority of people also gave details about their diagnosis and treatment at this point, if applicable.
Research aim
The research aim for the focus groups was to seek an understanding from people with personal experience of cancer of the possible difficulties and/or opportunities involved in making a decision to take part in the OPTIMA study. Owing to the complexity of the study, each focus group session started with a brief 5-minute introduction to the trial and written material was handed out in a pack to each participant including the flow diagram.
Each group discussion was loosely structured to allow participants to focus on the difficulties and opportunities that were important to them. Each was opened with the question, ‘Do you think you would take part in the OPTIMA study?’. This was clarified by questions from the interview schedule detailed in Table 5.
Key question | Related questions |
---|---|
What information would you need to make a decision to consent to the OPTIMA trial? |
|
Should an independent organisation provide information to potential participants? |
|
What do you think might motivate people who have refused the main study to take part in a qualitative study? |
|
Analysis
The analysis of focus group data has been criticised as being subjective, with methods often not explained fully in publications. 134–137 It is usually based on a ‘constant comparison’ approach, as initially used in grounded theory research,138 and involves coding and categorising data to identify themes.
We followed a previously described approach to analysis,139 which incorporates eight key stages of interpretation (Table 6).
Stage | What is interpreted | What to consider |
---|---|---|
1 | Words | The actual words used and their meaning (e.g. metaphorical use) |
2 | Context | The context in which things are said (by both participants and facilitators) |
3 | Internal consistency | Any changes in opinion by the participants |
4 | Frequency | How often a view is expressed (but also need to consider contrary but relevant views) |
5 | Intensity of comments | The ‘depth of feeling’ in which views are expressed |
6 | Specificity of responses | Personal experience vs. hypothetical situations |
7 | Extensiveness | The number of participants who express a view |
8 | Big picture | Significant themes or concepts that emerge from an accumulation of data and intersect various discussions (e.g. in all groups) |
The three focus groups were analysed separately and then combined to create a composite. Analysis was iterative and did not follow a linear course. It involved listening to tapes, transcribing them and reading the transcripts with the notes taken during the group. The transcripts were loaded into NVivo V.9 (QSR International, Warrington, UK), which supports qualitative methods by allowing the organisation and analysis of unstructured spoken data. While scrutinising the data and highlighting content of interest, we began to code the data line by line. During this stage, developing categories and themes began to emerge. After this had been done separately for the three groups, they were amalgamated and coding and data categorisation continued until there was consensus about the themes.
Qualitative recruitment study methods
As there were anticipated challenges to recruitment, in addition to PPI, the OPTIMA prelim incorporated an integrated qualitative recruitment study (QRS). This used a refinement of the methods developed for the ProtecT (Prostate testing for cancer and Treatment) study’s complex recruitment intervention. 140 The QRS in OPTIMA prelim aimed to work with the chief investigator (CfI), TMG and OPTIMA staff to identify and address barriers to recruitment, in three phases:
-
Phase 1 sought to identify and understand recruitment difficulties through use of multiple qualitative methods.
-
Phase 2 involved sharing phase 1 findings with the TMG and CfI, and working collaboratively to design and deliver interventions to optimise recruitment and informed consent. Phase 2 interventions included individual confidential and group feedback sessions to dissemination of tips and guidance for recruiters. All forms of intervention were shaped on the basis of the findings from phase 1 and consideration of how the OPTIMA trials specific set-up could accommodate the intended changes.
-
Phase 3 was an evaluative process, consisting of careful mapping out of QRS interventions and assessment of potential impact on recruitment figures and recruiters’ practices.
The methods outlined below follow the consolidated criteria for reporting qualitative research (COREQ) checklist. 141 A copy of the COREQ checklist can be found in Appendix 3.
Phase 1: understanding recruitment issues
The first phase of the integrated QRS sought to understand barriers to recruitment, with the intention of developing interventions to support recruiters, improving the quality of information provision where needed and optimising recruitment rates. The approaches used to identify recruitment difficulties followed principles of ethnography, with a flexible approach to data collection and analysis of interviews, observations and documentary data including PISs and screening logs.
Sampling and recruitment
The sampling frame consisted of all TMG members and OPTIMA prelim staff members from centres participating in the QRS at the start of the study (9 out of the 25 centres open to recruitment). ‘QRS centres’ comprised those which agreed to take part in this ‘optional’ element of OPTIMA prelim. Additional centres that opened in later stages of the trial were also approached to take part in the QRS (contributing audio-recordings only).
Interviews with OPTIMA staff
The TMG and staff members at QRS centres were purposefully selected for interview based on their involvement in recruiting patients or trial oversight, and with the aim of selecting individuals occupying a range of professional roles relevant to the recruitment process (e.g. oncologists, research nurses). Some informants were purposefully selected on the basis of emerging analytical insights that warranted further investigation. Informants were invited via e-mail to take part in a semistructured research interview with LR (the qualitative researcher working on OPTIMA) at a mutually convenient time/date. E-mail reminders were sent 2 weeks after dispatch of the original invitation. There was also scope to approach further individuals for interview as new centres opened throughout the course of OPTIMA prelim.
Following semistructured interviews, all research nurses at participating QRS centres were invited (via e-mail) to take part in a short, structured telephone interview to answer a series of questions about eligibility processes and patient pathways at their centre. The processes of organising a convenient time/date and dealing with non-respondents were similar to those described above.
Audio-recorded consultations
All staff members involved in recruiting patients were asked to routinely record consultations at which the OPTIMA prelim was discussed with patients. A research nurse or the principal investigator (all of whom were oncologists) for each centre distributed staff information sheets about the recording process and obtained one-off written consent that covered all subsequent recordings made throughout the study period. Patients were ‘recruited’ through a two-step consent process, developed to ensure that they were fully informed. Staff obtained initial verbal consent to record the patient’s first consultation, followed by written consent in a follow-up consultation. An information sheet explaining the recording process was handed to patients in the first consultation and patients were given sufficient time to consider their participation (at least 24 hours). If the patient declined participation in their follow-up consultation, any recording(s) obtained to date were destroyed and no further recordings made. If consent was provided, the second and any subsequent consultations were also recorded.
Data collection
Interviews
Semistructured interviews with staff were conducted face to face on hospital trust premises or via telephone if meeting in person was not feasible. A topic guide was used to ensure broad topics were consistently covered, although questions were adapted according to the role of the informant and sufficient scope provided for exploration of new topics that emerged through discussion. Topic guide areas covered: informants’ summaries of the RCT design and protocol; views on whether or not the trial conception/design were appropriate; thoughts on whether or not practices deviated from protocols; equipoise in relation to the RCT arms, and experiences of recruitment successes and difficulties (final topic guides shown in Appendix 4 and the original versions are shown in Appendix 5). All interviews were conducted by LR and JD (Jenny Donovan, the QRS lead) between January and June 2013, and lasted between 40 minutes and 1.5 hours. Sampling of potential interview participants ceased once the QRS team were satisfied that data saturation had been achieved, defined as the point at which no new themes emerged from two consecutive interviews.
A series of structured, short telephone interviews (lasting up to 10 minutes) were conducted with research nurses and notes were taken in response to the structured fact-based questions about eligibility processes and patient pathways.
Audio-recorded consultations
Audio-recordings were collected throughout the duration of the QRS (April 2013–April 2014). Centres were provided with audio-recording devices and instructions for recording, uploading and storing recordings on a secure computer on trust premises. Staff were encouraged to routinely record all interactions with patients (following receipt of written consent) and received monthly updates from LR. Transfer of recordings was periodically arranged through postage of encrypted data storage devices between the research nurses and LR. Consultations were deleted from trust computers once LR confirmed safe receipt of each (set of) recording(s).
In addition to these data collection processes, LR joined in on scheduled ‘core TMG’ and wider TMG meetings, occurring approximately biweekly and once every few months, respectively, to facilitate understanding of recruitment issues and trial processes. The ‘core TMG’ consisted of select members of the TMG with the greatest responsibility for trial oversight (e.g. the CfI, trial manager and other individuals with leadership responsibilities).
Data analysis methods
Interview analysis
Interviews were audio-recorded, transcribed in full and analysed thematically using the constant comparison method derived from grounded theory methodology. 138 This involved line-by-line coding of transcripts, categorising codes into themes, and developing codes and themes as transcripts were reread in light of newly collected data. Analysis was primarily conducted by LR and supported through use of the software package NVivo (version 9). A sample (10%) of transcripts from interviews were independently analysed by a trained qualitative researcher working in trials methodology (SP) in the initial stages of data collection. A basic coding frame was agreed, which evolved as data collection proceeded. The QRS research team regularly discussed extracts of raw data in face-to-face meetings. A descriptive account of interview findings was written towards the end stages of data collection and discussed by the research team to fine tune the ‘key themes’. This document evolved as analysis progressed and discussions among the research team developed over time. Major themes from interviews were presented in matrices, with individual accounts arranged by centre and participant role to encapsulate patterns of meaning within each set of accounts. There was an attempt to search for ‘negative cases’ in relation to particular themes or theories; where present, these were fully reported in the evolving descriptive account. A final series of Microsoft Word (Microsoft Corporation, Redmond, WA, USA) documents, each devoted to a particular theme, were compiled towards the end of the analytical process to facilitate reporting of findings.
Screening log analysis
There was an intention to map the patient eligibility and recruitment pathways through analysis of screening log data, with a view to producing detailed accounts of the numbers and percentages of patients at each stage of the process. It emerged that screening logs did not provide the necessary data to do this, as these only recorded information from the point at which the patient was approached about trial participation; details of initial screening and eligibility were not captured in the logs. In light of this, interview data were used to map out a broad outline of eligibility processes and recruitment pathways for each site. Screening logs were referred to regularly to track recruitment rates, which, in turn, partially informed targeting of centres that could benefit from intervention. Screening logs also provided useful details pertaining to recruiters’ reported reasons for patients declining the trial (see Chapter 4, Screening and acceptability). These insights were considered alongside data emerging from interviews and audio-recorded consultations.
Audio-recorded consultation analysis
A systematic and multifaceted approach was adopted for analysis of audio-recorded consultations. Consultation transcripts were initially analysed thematically using the constant comparison methods described above. Areas of interest were coded to a greater number of levels than sections that were not directly relevant to the OPTIMA prelim (e.g. rapport-building conversation, family medical histories). Key themes arising from interviews were used as a starting point for in-depth analysis. Recruiters’ explanations and use of terminology in the lead up to points of ‘confusion’ were scrutinised and compared with explanations of the same concepts in other consultations – particularly those that appeared to be easily understood and accepted by patients. Approaches to analysis inspired by conversation analysis were used in a targeted, practical manner, where there was an intention to interpret patients’ reactions to trial explanations (e.g. intonation of words/noises and the meanings of silences/pauses in interaction). 142,143
The audio-recordings were initially listened to by LR. She noted points of interest and preliminary thoughts, and followed this with targeted transcription of consultations. Sections of speech that were not directly relevant to the OPTIMA prelim were summarised by LR in brackets (with timings). This included non-clinical discussions about patients’ life contexts and details of medical history. Speech that was directly or loosely related to the OPTIMA prelim’s rationale, aims and processes was transcribed using standard notation. Points of confusion or contention or ‘troubled’ speech were targeted for verbatim transcription (i.e. including pauses, repetition of words and ‘ums’, ‘ahs’). The length of time before introducing the OPTIMA prelim study was timed for all consultations. This was considered alongside patients’ engagement and comprehension of trial-specific processes.
Analytic synthesis
Themes for the interview and audio-recording data sets were compared and considered alongside screening log data describing patients’ reasons for declining the trial. Findings from the multiple data sources were presented to the CfI and TMG as key findings for phase 1 of the QRS.
Phase 2: development and implementation of recruitment strategies
The findings from the QRS were developed into plans for feedback and training with the CfI and TMG members. All materials used for guiding and supporting recruiters were based on findings grounded in data collected in phase 1. Recruitment difficulties recurring across centres formed the basis of ‘generic’ interventions disseminated across all centres through ‘tips and guidance’ documents and regional feedback meetings attended by multiple centres. Clinician-to-clinician meetings between clinical members of the TMG and clinicians at recruiting centres were suggested where differences in clinical opinion had implications for recruitment. Single-centre feedback sessions were also developed for those who requested this or were unable to attend regional meetings. Difficulties experienced by individual recruiters were tackled through development of individual feedback plans that were discussed in confidence with the relevant individuals.
Phase 3: impact of qualitative recruitment study interventions
The final phase of the QRS drew on multiple approaches to evaluating the impact of the QRS on recruitment. The time points at which QRS interventions were delivered were mapped out on graphs that charted recruitment figures over time. Changes in the direction (or rate of increase/decrease) in recruitment figures were assessed relative to the QRS interventions. Other approaches to analysis considered recruitment rates in centres that received certain forms of intervention, compared with centres that did not. Further qualitative analysis also featured in this phase, where recruiters’ practices were compared before and after points of intervention. The varied approaches to evaluating the QRS were considered collectively with a view to forming tentative conclusions about the QRS’s success in optimising recruitment within OPTIMA prelim.
Chapter 3 Methods: pathology and health economic study
Pathology research in the preliminary study
Additional research testing of tumour tissue was planned for all confirmed eligible patients, irrespective of randomisation, to enable the performance of alternative multiparameter assays to be evaluated. This research is integral to the preliminary study, and all patients who joined the study were required to consent to this research. Genomic Health performed Oncotype DX testing on samples from participants randomised to the standard arm as part of this programme at the time of eligibility confirmation; these results were not used to determine the participant’s treatment. Following Oncotype DX testing, residual tumour tissues were stored in the OPTIMA tissue bank at the University of Edinburgh. This is a good laboratory practice facility managed by Professor John Bartlett [University of Edinburgh and Ontario Institute for Cancer Research (OICR) Toronto].
Patients were also asked to give additional optional consent for future (unspecified) research to be performed on their tissue samples, which could include genetic testing. If such permission was given, tumour samples were retained in the OPTIMA tissue bank beyond the completion of the study. Should the treating centre require a sample for diagnostic use in the future, the stored tumour sample will be returned.
Multiparameter assays performed
We set out to evaluate as many tests as possible through positive engagement with multiple partners. Discussions with several vendors of tests, which owing to their characteristics might be candidates for inclusion in the OPTIMA main study, were undertaken during the design phase of OPTIMA prelim to secure collaboration. Strenuous efforts were made to secure collaboration with additional test vendors during the recruitment phase. We recognised that for commercially available tests it was important that evidence of quality of test performance was ensured. The possibility of centralising tests to a single laboratory was considered but rejected because the performance of most tests was dependent on commercial laboratories (e.g. Oncotype DX, MammaPrint) or expert readers (e.g. Mammostrat).
The vendors of the following tests were approached for support:
-
BCI
-
Endopredict (Sividon Diagnostics, Cologne, Germany)
-
Genomic Grade Index (Bordet Institute, Brussels, Belgium)
-
MammaPrint/BluePrint/TargetPrint
-
Mammostrat
-
MammaTyper
-
Molecular Grade IndexSM (bioTheranostics/Qiagen, Limburg, the Netherlands)
-
NexCourse Breast by AQUA
-
Prosigna (PAM50).
Five tests were not taken forward for analysis in the OPTIMA prelim cohort following discussions with the vendors/licensees: BCI, Endopredict, Genomic Grade Index, Molecular Grade Index and Mammostrat. The reasons for rejecting these tests included OPTIMA prelim population not appropriate for the test in question (n = 3); test licensing under negotiation/transfer (n = 1); and test withdrawn from study (n = 1). The decision not to offer these tests for the OPTIMA population reflected commercial concerns about prematurely transposing tests into novel applications. Tests developed by two vendors who were supportive during the design phase were not included because of changed commercial development plans.
It is noteworthy that all companies participating in these discussions were supportive of the collaborative effort being undertaken and the OPTIMA trial in particular, and sought to ensure the broadest comparison between testing approaches given commercial constraints.
The Prosigna assay was performed at Professor Bartlett’s research laboratory at the OICR, following staff training and accreditation by NanoString Technologies. MammaPrint and BluePrint assays were performed by Agendia technologies using sections provided by the University of Edinburgh. MammaTyper quantitative PCR assays were performed by Stratifyer GmBH at laboratories in Mainz, Germany, using residual ribonucleic acid (RNA) from the Prosigna assay extracted using the Roche mRNA extraction kit provided by Prosigna. The NexCourse Breast IHC4 assay using AQUA (IHC4 AQUA) was performed by Genoptix medical laboratories on replicate tissue microarrays (TMAs) provided by the University of Edinburgh.
In addition to the commercial assays, IHC4 was also performed by conventional pathology techniques [HER2 testing by in situ hybridisation at the UCL (University College London) Advanced Diagnostics Laboratory and ER, PgR and Ki67 on TMAs by quantitative image analysis (Ariol version 4.0 SL50, Leica Microsystems, Wetzlar, Germany) using standard immunohistochemistry at OICR as previously described]. 144 Quantitation of ER, PgR and Ki67 to the degree required to calculate an IHC4 is not standard practice in NHS pathology laboratories and, indeed, Ki67 is not a standardised marker for routine testing in breast cancer pathology assessment.
Results were calculated to established procedures for each assay or, in the case of IHC4, using the published algorithm. 56 Tumours were categorised into risk groups based on the predefined cut-off points for the continuous Prosigna ROR scores, IHC4 and IHC4 AQUA (see Appendix 6 for details). These risk groups were then compared with each other including those defined by Oncotype DX.
Some guidelines, including the internationally recognised St Gallen guideline,145 recommend the use of breast cancer subtyping as a means of informing treatment options. Breast cancers can be subtyped into four main molecularly defined intrinsic subtypes (see Parker et al. 28 and references therein25–27), namely luminal A, luminal B, HER2 enriched and basal-like.
Three tests (BluePrint, Prosigna and MammaTyper) provide information on molecular subtypes. BluePrint, which is performed together with MammaPrint, and Prosigna additionally provide information about recurrence risk, which is binary in the case of MammaPrint and takes the form of a categorised risk score for Prosigna, while MammaTyper is specifically focused on providing subtype information including a stratification of ‘luminal B’ cases into low and high risk based on the Ki67 result (see Appendix 6 for details).
Analytical methods
For the purpose of analysis, patients were divided by randomisation and by Oncotype Dx score (≤ 25 vs. > 25). Patients#x02019; individual predicted predicted risks of both recurrence and death were summarised using median and ranges. The kappa coefficient and associated 95% CI was used to assess agreement between tests. All statistical analyses were performed using the SAS statistical package (version 9.3; SAS Institute Inc., Cary, NC, USA).
Sample size
OPTIMA prelim required 300 patients to be recruited over the first 2 years (a 6-month set-up phase and an 18-month recruitment phase). Oncotype DX was used prospectively to decide on patient chemotherapy treatment in the research arm (using a RS cut-off point of ≤ 25 vs. > 25), while alternative tests were applied retrospectively. The true efficacy of the tests will not be known until all patients have been followed up for 5 years and IDFS is compared. Therefore, the study was powered to compare risk groups from the multiparameter tests with Oncotype DX as well as with each other.
To assess agreement between tests, the sample size calculations assumed that 70% of patients randomised to test-directed treatment would be assigned to no chemotherapy as a result of the Oncotype DX test and the true kappa value was 0.8. A sample size of 300 patients would provide stability around the kappa estimate and provide a lower 95% CI of 0.73. These numbers were also sufficient to be able to detect agreement between tests, taking into account the expected type of patients entered into the study, if the assumed proportion of patients randomised to test-directed treatment would be assigned to no chemotherapy varied from 55% to 80% (Table 7). The lower confidence limit for kappa varied from 0.74 to 0.72, when the proportion of patients assigned to having no chemotherapy varied from 55% to 80%.
Sample size | Proportion of patients assigned to no chemotherapy | Lower 95% CI if the true kappa is 0.8 |
---|---|---|
300 | 0.55 | 0.735266 |
300 | 0.60 | 0.7341705 |
300 | 0.65 | 0.7322176 |
300 | 0.70 | 0.7291755 |
300 | 0.75 | 0.7246098 |
300 | 0.80 | 0.7176715 |
Economic evaluation
Introduction to the economic analysis
There is currently interest in using decision modelling earlier in the research and development process for new health-care technology. 146 This is, in part, a response to the high costs and high failure rates of pre-regulatory Phase III trials. A decision model can be used to analyse uncertainties in the current effectiveness and cost-effectiveness evidence. The estimate can then be used to tailor a Phase 3 research design to address uncertainties that pose the most risk for clinical, regulatory and reimbursement decision-makers.
The objective of the economic analysis for OPTIMA prelim was to establish the value of conducting further research into the cost-effectiveness of Oncotype DX or alternative test-directed therapy in the UK in the setting of a main OPTIMA trial. OPTIMA prelim also assesses the feasibility of resource use measurement and the within-trial identification of appropriate health-care costs and health-related quality-of-life information to inform the calculation of QALYs.
The economic model and methods developed for this analysis are described in this section. Details on the interpretation of the results of the economic analysis are given in Chapter 5 (see Economic analysis).
Test selection for inclusion in the main economic (base-case) analysis
The factors that were considered when assessing alternative tests for possible inclusion in the main study included analytical validity or reproducibility, biological plausibility and cost-effectiveness. Evidence must exist that an assay produces reproducible results when it is run more than once on the same sample and for distributed tests, that the results are reproducible between laboratories undertaking the assay. The assignment of patients to chemotherapy or not by a test must also be plausible. For instance, if a HER2 test was used as the primary discriminator within the OPTIMA trial, no patient would be assigned chemotherapy, which may make this test appear highly cost-effective. However, although HER2 is a binary test that identifies a high-risk patient population, HER2-positive patients are excluded from the OPTIMA trial, so the test is not plausible.
A base-case model was specified as the main analysis and from which sensitivity analyses were performed. Tests were selected for inclusion in the base-case analysis if they met all three of the following criteria:
-
sufficient evidence of analytical validity in support of an achievable rollout into routine care in the NHS
-
peer-reviewed evidence published in the public domain for clinical validity in a relevant clinical setting
-
existence of either (a) a global or UK list price in the public domain for the test or (b) a credible way of estimating the cost of performing the test including a list price for all test components.
Tests meeting these criteria were Oncotype DX, MammaPrint and Prosigna. IHC4 was not included owing to concerns about the analytical validity when used as a locally implemented test in NHS laboratories. Further details about this rationale are outlined above (see Chapter 1, Individual multiparameter assays) and elaborated on in the discussion. IHC4 AQUA and MammaTyper were excluded owing to a lack of peer-reviewed published evidence for clinical validity in a relevant clinical setting. MammaTyper was also excluded, as the manufacturer put no price forward. An additional sensitivity analysis (see Sensitivity analysis number 3, all tests included) was conducted that included all tests. Where a price was not available from the manufacturer (as with MammaTyper) this was estimated by the analyst.
Clinical risk prediction
The outcome of the OPTIMA prelim patients will be unknown for 5 years and, therefore, it was necessary to forecast long-term survival outcome for the OPTIMA trial population as an assessment of the population under study and to enable the calculation of lifetime expected QALYs for the economic model. The population included in the OPTIMA prelim study are ER-positive, HER2-negative early-stage breast cancer patients. The clinical risk prediction nomograms NPI,19 Adjuvant! Online (version 8)17 and PREDICT18 were applied to each patient. Both Adjuvant! Online and PREDICT give an estimate of treatment benefit for both endocrine therapy and chemotherapy over a 10-year period. PREDICT, but not Adjuvant! Online, takes HER2 status into account, while only Adjuvant! Online provides an estimate of recurrence-free survival (RFS). Chemotherapy benefits estimated with the nomograms used the intended regimen for that patient, where FEC75–80, FEC90–100, E-CMF and TC are considered to be ‘second-generation’, and FEC-T and FEC-Pw are ‘third-generation’ regimens.
Endocrine therapy was specified as AI for post-menopausal and tamoxifen for premenopausal patients in Adjuvant! Online. Comorbidities for Adjuvant! Online were entered as ‘average for age’. No attempt was made to apply a correction for the HER2-negative status of the OPTIMA population using the inbuilt prognostic tool. Ki67 was entered as ‘unknown’ in PREDICT. Five-year RFS probability was also estimated using both a ‘constant benefit’ model (which assumes that the benefit of chemotherapy is constant across all risk scores and independent of tumour biology as determined in the Early Breast Cancer Trialists’ Collaborative Group meta-analysis)9 and a ‘variable benefit’ model (which assumes that the benefit of chemotherapy varies according to the test risk score, as determined in the SWOG88-14 trial). 62 These two models are described in more detail in sections Model parameters and Sensitivity analysis. The additional benefit that might be gained from having chemotherapy was calculated per patient as the difference in the survival estimates obtained if they were to have both endocrine therapy and chemotherapy, and that for if they only had endocrine therapy. The median and ranges of the estimates from each nomogram for all patients within the RS low group and high groups separately were obtained.
The economic model
A model-based economic evaluation of Oncotype DX was conducted during the design period of the OPTIMA prelim. This served a dual purpose: first, it allowed the model to inform the design of OPTIMA prelim, ensuring that the design and data collection was pertinent for UK NHS decision-making; and, second, it prepared a suitable model that could form the basis of an economic analysis at the end of the feasibility period to aid in the design and selection of appropriate tests for inclusion in the main trial. The original model is described in a separate publication. 15
For the OPTIMA prelim analysis, model parameters have been updated by a systematic literature search and costs have been replaced by contemporary costs, where possible from 2014, adjusted to a common base year of 2012–13 (with conversions for the list prices of tests into GBP made using www.xe.com). An additional health state representing acute myeloid leukaemia secondary to chemotherapy has also been added.
The economic model comprises a time-dependent discrete-state transition model (modified Markov model). A detailed explanation of these methods is provided by Sonnenberg and Beck. 147 The model was used to estimate mean differences in clinical effects, including life-years, QALYs and costs for a hypothetical cohort of women with ER-positive, lymph node-positive breast cancer.
Overview and model structure
The methods for the economic model followed the NICE guidance. 148 The structure of the model was developed by consensus among clinical experts, health economists and medical statisticians. The structure was developed from a previously published model. 149 The first part of the model structure is an instantaneous decision tree that allocates a patient cohort either to standard care, where all patients receive chemotherapy, or to a test that is used to allocate patients to high- or low-recurrence risk groups (Figure 2). Patients in the high-risk group, but not those in the low-risk group, are allocated chemotherapy. The second part is a modified Markov model, with annual cycles, which is used to calculate mean life-years, QALYs and costs per patient in each group (Figure 3). All patients were assumed to receive identical non-chemotherapeutic adjuvant therapies.
FIGURE 2.
Decision tree.

FIGURE 3.
State-transition model.

Patients entered the model at the initiation of adjuvant therapy and were assumed to be disease free. This time point is synchronous with randomisation into OPTIMA prelim. The model assumes that patients then move into a disease-free (follow-up) state; develop a local recurrence (which includes locoregional recurrence) or a distant recurrence; develop congestive cardiac failure, acute myeloid leukaemia; or die of unrelated causes. It was assumed that patients remained disease free until they developed a breast cancer recurrence, cardiac failure, acute myeloid leukaemia or died.
The model assumes that patients who develop a local recurrence can be treated curatively and move into a separate ‘disease-free after recurrence’ state, which carries distinct risks of further distant relapse. For simplicity, the model assumes that patients can develop only one local recurrence. Patients remain in the distant recurrence state until death from breast cancer or death from other causes.
Model parameters
Test allocation
Estimates of the proportion of patients assigned to high- and low-risk groups and recurrence estimates for each group were initially taken from the SWOG88-14 Oncotype DX analysis and used in a validation model. 62 Proportional allocation was available for alternative Oncotype DX RS cut-off points of 18 and 25. Patient allocation into risk groups identified by all Oncotype DX cut-off points and patient allocation by alternative tests were subsequently replaced by data from OPTIMA prelim. In the SWOG88-14 trial, 40% [standard error (SE) 0.026] of patients were allocated to a group with a RS of < 18 and 59% (SE 0.026) were allocated to a group with a RS of ≤ 25. These proportions were represented by a beta distribution in the probabilistic model. Test allocation was subsequently estimated from the OPTIMA prelim data for use in the base-case analysis and sensitivity analyses.
Cancer recurrence rates and chemotherapy effect sizes
Estimation of cancer recurrence rate is central to the model. A major challenge of using decision-analytic modelling before the main OPTIMA trial is the uncertainty about recurrence rates and chemotherapy effects given the scarcity and limited transferability of available published evidence. In parallel with the OPTIMA prelim statistical analysis, five separate model specifications were proposed, each relying on alternative assumptions about chemotherapy effect and population characteristics.
Validation model: update of published model based on the SWOG88-14 trial (Oncotype DX)
In the original model, recurrence rates were taken from the SWOG88-14 trial for all patients treated with or without anthracycline-containing chemotherapy. 62 SWOG88-14 provides survival estimates over 10 years. It should be noted that the main limitation of the validation model is that the SWOG patient population may not be representative of a UK OPTIMA population. For example, in the intervening years since the SWOG88-14 trial, the NHS breast screening programme has been put in place; recruitment into the OPTIMA trial is commencing nearly 20 years later than into SWOG88-14, the OPTIMA trial has different eligibility criteria than SWOG88-14 (with HER2-positive disease excluded) and, therefore, there is likely to be different distribution of test scores. In addition, because RFS was not available for this study, IDFS was used as a proxy for RFS, justified by the low number of deaths during the first 5 years of follow-up. Recurrence rates were available for groups selected by Oncotype DX based on RS cut-off points of 18 or 25. In this updated analysis, RFS for each group was represented by an exponential survival distribution (constant hazard), specified separately for years 1–5 years and 6–10 years. This uses 5- and 10-year IDFS proportions with SEs taken directly from the SWOG88-14 trial data, which was kindly provided by the investigators (Table 8). The annual hazard was calculated from the event rate over each 5-year period represented by a beta distribution, the parameters of which were calculated from the moments, to allow Monte Carlo simulation in the probabilistic analysis. An important additional parameter is the transition probability from disease recurrence to death, referred to as post-recurrence survival; this is a major component defining the relationship between RFS and overall survival. There is very little evidence to inform this parameter in the relevant patient populations.
Patient group | RS | 5-year IDFS | SE | 10-year IDFS | SE |
---|---|---|---|---|---|
All patients treated with chemotherapy | All | 0.79 | 0.028 | 0.61 | 0.038 |
Tamoxifen alone | RS of ≤ 18 | 0.91 | 0.039 | 0.60 | 0.092 |
RS of ≤ 25 | 0.85 | 0.038 | 0.57 | 0.067 | |
Tamoxifen and chemotherapy | RS of > 18 | 0.74 | 0.039 | 0.58 | 0.049 |
RS of > 25 | 0.71 | 0.048 | 0.56 | 0.059 |
The OPTIMA prelim base-case model
Clinical outcomes from the OPTIMA prelim trial were estimated as described earlier (see Clinical risk predication) using Adjuvant! Online. The economic model requires an estimate of RFS, and the analysis is therefore restricted by the availability of this. Forecast RFS, assuming no chemotherapy, was derived for the OPTIMA prelim patient population using baseline clinical parameters entered into Adjuvant! Online. 17
The only evidence of a predictive (variable chemotherapy benefit) effect at the time of writing is based on retrospective Oncotype DX testing of patients in the SWOG88-14 trial (Table 9).
Oncotype DX RS | Hazard ratio | Upper 95% CI | Lower 95% CI |
---|---|---|---|
10 | 1.24 | 0.62 | 2.48 |
18 | 1.03 | 0.58 | 1.81 |
25 | 0.87 | 0.53 | 1.42 |
31 | 0.75 | 0.48 | 1.18 |
40 | 0.61 | 0.39 | 0.96 |
This was represented in the economic model by a linear regression model,
where logHR = the log-hazard ratio for RFS, α= 0.4541 (SE 0.03749), β= –0.0238 (SE 0.00418), RS = recurrence score and the correlation between α and β is assumed to be –0.5.
The resulting hazard ratio was applied to the no chemotherapy recurrence rates for all individual patients in the ‘chemotherapy for all’ standard care arm of the model over the first 5 years. In the test-directed arm of the model, the same chemotherapy effect was applied only to patients in the high-risk groups. Where there were no data providing evidence for the predictive ability of alternative tests, extra uncertainty was introduced into the model for these alternative tests depending on the degree of discordance between the test and Oncotype DX. For example, a completely concordant test will have identical predictive ability and therefore no extra uncertainty is introduced. To achieve adequate representation of extra uncertainty, a suitable prior for the chemotherapy effect of the alternative test was required. In the absence of any informative information, this prior treatment effect was represented by a hazard ratio of mean 1 with a very large standard deviation (SD), assumed to be log-normally distributed. The choice of prior was subject to sensitivity analysis. In the base-case analysis, post-recurrence survival was assumed to be independent of pretreatment with adjuvant chemotherapy; the annual post-recurrence probability of death was assumed to be constant across groups with a mean of 0.30 (SD 0.22).
Chemotherapy treatment and toxicity
The chemotherapy regimens and toxicity rates were used to estimate treatment-related costs. The proportions of patients treated with anthracycline plus taxane, anthracycline alone, or taxane alone were estimated from the OPTIMA prelim data. Chemotherapy toxicity rates150 were estimated from landmark chemotherapy clinical trials (Table 10). 151–153 Toxicity rates for FEC-Pw were assumed to be equivalent to FEC-T, and toxicity rates for epirubicin were assumed to be equivalent to FEC. Toxicity rates for FEC75 were assumed to be equivalent to two-thirds the rates for FEC100.
Toxicity parameter | Probability | Distribution |
---|---|---|
TC (USO-9735 trial, Jones et al. 2009,151 n = 506) | ||
Febrile neutropenia | 0.046 | Beta(23,483) |
Anaemia | 0.010 | Beta(5501) |
Thrombocytopenia | 0.005 | Beta(2504) |
Stomatitis | 0.008 | Beta(4502) |
Diarrhoea | 0.025 | Beta(12,494) |
Nausea and vomiting | 0.030 | Beta(15,491) |
FEC100 (PACS-01 trial, Roché et al. 2006,152 n = 995) | ||
Febrile neutropenia | 0.084 | Beta(84,911) |
Anaemia | 0.014 | Beta(14,981) |
Thrombocytopenia | 0.003 | Beta(3,992) |
Stomatitis | 0.04 | Beta(40,955) |
Diarrhoea | 0 | Beta(1,996) |
Nausea and vomiting | 0.205 | Beta(204,791) |
FEC-T (PACS-01 trial, Roché et al. 2006,152 n = 1001) | ||
Febrile neutropenia | 0.112 | Beta(112,889) |
Anaemia | 0.007 | Beta(7994) |
Thrombocytopenia | 0.004 | Beta(4997) |
Stomatitis | 0.059 | Beta(59,942) |
Diarrhoea | 0 | Beta(1,1002) |
Nausea and vomiting | 0.112 | Beta(112,889) |
E-CMF (TACT2 trial, Cameron et al. 2010,153 n = 1029) | ||
Febrile neutropenia | 0.13 | Beta(137,892) |
Anaemia | 0.03 | Beta(31,998) |
Thrombocytopenia | 0.01 | Beta(10,1019) |
Stomatitis | 0.00 | Beta(1,1030) |
Diarrhoea | 0.04 | Beta(46,983) |
Nausea and vomiting | 0.02 | Beta(24,1005) |
Late effects
Parameters are included in Table 11. The population age- and sex-specific incidence of congestive heart failure was taken from Office for National Statistics published data as presented by the British Heart Foundation. 154 The lifetime relative risk of congestive cardiac failure after chemotherapy was based on data from the Oxford Overviews and applied to the population incidence, to provide an estimate of excess congestive heart failure because of chemotherapy. 9 Mortality after onset of congestive cardiac failure was taken from a UK population study. 155
Parameter | Mean | SE | Distribution | Notes | Source |
---|---|---|---|---|---|
Proportion locoregional vs. distant recurrence | 0.31 | 0.015 | Beta | Proportion of recurrences that are local to the original breast cancer primary | Baum et al., 2003156 |
Death after distant recurrence | 0.30 | 0.025 | Beta | Annual probability of death after a distant recurrence (ER-positive) | Walkington et al., 2012157 |
Background mortality | Age specific | Fixed | Life tables | Office for National Statistics, 2009158 | |
Chemo-associated excess mortality (first year) | 0.0024 | 0.0019 | Beta | 2.4% aged 55–69 years | Peto et al., 20129 |
Background rate CHF | Age specific | Fixed | Annual age-specific female incidence of CHF | Townsend et al., 2012154 | |
Relative risk of CHF with anthracycline treatment | 1.61 | 0.31 | Log-normal | Applied as a constant lifetime risk | Peto et al., 20129 |
Death after CHF | 0.6 | 0.033 | Beta | Hillingdon study | Cowie et al., 2000155 |
Background annual rate AML (female, aged 60–79 years) | 0.00296 | Fixed | Fixed | Bhayat et al., 2009159 | |
Relative risk of AML after chemotherapy | 2 | 4 | Log-normal | Uncertain estimate | Azim et al., 2011160 Peto et al., 20129 Praga et al., 2005161 |
Relative 5-year survival for AML (female, aged ≥ 65 years) | 0.0383 | 0.0048 | Beta | Assumes constant relative survival, applied to background mortality rate | Oliver et al., 2013162 |
The age- and sex-specific incidence of acute myeloid leukaemia was taken from a large UK primary care-derived population database. 159 There is evidence for an increased relative risk of acute myeloid leukaemia in patients treated with chemotherapy from a number of published pooled trial-based analyses, but this was difficult to estimate reliably owing to the low absolute numbers of observed events. 8,160,161 The relative risk of acute myeloid leukaemia was estimated at 7.6 (SE 7.1). Survival after a diagnosis of acute myeloid leukaemia was based on UK Cancer Registry statistics, as provided in a report by the Northern and Yorkshire Cancer Registration and Information Service. 162
Other transition probabilities and proportions
The mean time from metastatic recurrence to death was estimated from a UK patient-level analysis of 1000 consecutive breast cancer patients in a single NHS trust with a minimum of 10 years’ follow-up. 157 An estimate of the proportion of recurrences that are locoregional was taken from the ATAC trial, which included a large number of UK patients with similar characteristics to patients enrolled into OPTIMA prelim. 156 Background age-specific non-breast cancer mortality was estimated by subtracting age-specific breast cancer-specific mortality from the age- and sex-specific background mortality, obtained from the UK Office for National Statistics. 158
Costs
Costs were adjusted to a base year of 2012–13 using the Hospital and Community Health Service pay and prices index published by the Personal Social Services Research Unit. 163 In the probabilistic analysis, costs were assumed to have a log-normal distribution. The additional expected health-care costs in patients receiving chemotherapy were derived by combining the expected costs of chemotherapy procurement and delivery with those expected from follow-up. Assumptions were made about routine practice in the UK NHS based on advice from oncologists in London, Edinburgh and Leeds:
-
Chemotherapy regimens represented in the economic model are FEC100, FEC-T, FEC-Pw, E-CMF and TC (see Table 3).
-
Average body surface area 1.7 m2.
-
Relative dose intensity 92% (all planned cycles completed).
-
No vial sharing permitted (remaining drugs in vials assumed to be wasted).
-
Standard supportive medication, procurement, laboratory, pharmacy and administration costs are based on electronic Market Information Tool,164 British National Formulary165 and NHS reference costs. 166
-
Two medical oncology clinic visits per regimen and one specialist nurse review per cycle.
-
Granulocyte colony-stimulating factor (five doses of filgrastim 300 µg) for 50% of FEC, 100% of FEC-T, and 0% of TC and E-CMF cycles.
-
Aprepitant (Emend®, Merck Sharpe & Dohme Ltd) is used as a prophylactic anti-emetic in 20% of FEC cycles.
-
Grades 3 and 4 acute toxicity rates as per the PACS-01, US Oncology 9735 (USO-9735) and the trial of accelerated adjuvant chemotherapy with capecitabine in early breast cancer (TACT2) trials [including febrile neutropenia rates for TC (4.6%), FEC100 (8.4%), FEC-T (11.2%) and E-CMF (13%)].
-
Fifty per cent of toxicity-related hospital admissions were assumed to be of more than 2 days’ duration (long stay) and 50% were assumed to be of less than 2 days’ duration (short stay).
-
Fifteen per cent of patients have a central line inserted (but associated complications of this are not costed).
-
Community health-care costs, out-of-pocket patient and carer costs and costs attributed to future lost productivity are not included.
-
Value-added tax (VAT) is not included on drug costs.
Unit costs for on-chemotherapy grade 3 and 4 toxicity were taken from the UK NHS reference costs. 166 A full breakdown of chemotherapy costs is given in Tables 12–16.
Regimen | Drug | Dose mg/m2 | Dose (mg/patient) | Vial size (ml) | Vial strength (mg/ml) | Mg per vial | Price per vial (£) | Number of vials/cycle | Drug cost/cycle (£) |
---|---|---|---|---|---|---|---|---|---|
FEC100 | Fluorouracila | 500 | 782 | 10 | 25 | 250 | 3.20 | 4 | 12.80 |
Epirubicinb | 100 | 156.4 | 100 | 2 | 200 | 27.87 | 1 | 27.87 | |
Cyclophosphamidea | 500 | 782 | – | – | 1000 | 17.06 | 1 | 17.06 | |
TC | Docetaxelb | 75 | 117.3 | 7 | 20 | 140 | 35.74 | 1 | 35.74 |
Cyclophosphamidea | 600 | 938.4 | – | – | 1000 | 17.06 | 1 | 17.06 | |
FEC-T | Fluorouracila | 500 | 782 | 10 | 25 | 250 | 3.20 | 4 | 12.80 |
Epirubicinb | 100 | 156.4 | 100 | 2 | 200 | 27.87 | 1 | 27.87 | |
Cyclophosphamidea | 500 | 782 | – | – | 1000 | 17.06 | 1 | 17.06 | |
FEC-T | Docetaxelb | 100 | 156.4 | 8 | 20 | 160 | 44.55 | 1 | 44.55 |
E-CMF | Epirubicinb | 100 | 156.4 | 100 | 2 | 200 | 27.87 | 1 | 27.87 |
Cyclophosphamidea | 600 | 938.4 | – | – | 1000 | 17.06 | 2 | 34.12 | |
Methotrexatea | 40 | 62.56 | 2 | 25 | 50 | 2.62 | 4 | 10.48 | |
Fluorouracila | 600 | 938.4 | 10 | 25 | 250 | 3.20 | 8 | 25.60 | |
FEC75 | Fluorouracila | 500 | 782 | 10 | 25 | 250 | 3.20 | 4 | 12.80 |
Epirubicinb | 75 | 117.3 | 100 | 2 | 200 | 27.87 | 1 | 27.87 | |
Cyclophosphamidea | 500 | 782 | – | – | 1000 | 17.06 | 1 | 17.06 | |
FEC-Pw | Fluorouracila | 500 | 782 | 10 | 25 | 250 | 3.20 | 4 | 12.80 |
Epirubicinb | 100 | 156.4 | 100 | 2 | 200 | 27.88 | 1 | 27.88 | |
Cyclophosphamidea | 500 | 782 | – | – | 1000 | 17.06 | 1 | 17.06 | |
Paclitaxelb | 80 | 136 | 25 | 6 | 150 | 11.26 | 3 | 33.78 |
Supportive medications | Unit dose | Unit cost (£) | Units per cycle | % use | Per cycle (£) |
---|---|---|---|---|---|
Dexamethasone (oral)a | 2 mg | 0.03 | 20 | 1 | 0.60 |
Dexamethasone (i.v.)a | 3.3 mg | 0.40 | 8 | 1 | 3.20 |
Ondansetrona | 8 mg | 0.15 | 1 | 1 | 0.15 |
Aprepitant (premade pack)b | 1 pack | 47.42 | 1 | 0.2 | 9.48 |
Metoclopramidea | 10 mg | 0.01 | 7 | 0.5 | 0.03 |
Filgrastim (Zarzior®, Sando) (GCSF)b,c,d | 300 μg | 52.70 | 5 | 1d | 263.50 |
Total cost of supportive medications per cycle (£) | |||||
FEC100 + 50% GCSF | 145.21 | ||||
FEC100 + GCSF | 276.96 | ||||
T + GCSF | 267.48 | ||||
CMF | 0.36 | ||||
TC | 3.98 | ||||
P | 0.08 | ||||
E | 0.03 |
Parameter | Mean (£) | HRG code |
---|---|---|
Toxicity (grades 3 and 4) HRG – short stay | ||
Anaemia | 644 | PA48B |
Febrile neutropenia | 877 | PA45Z |
Allergic reaction | 491 | WA11C |
Nausea | 340 | PA28B |
Diarrhoea | 356 | PA26B |
Thrombocytopenia | 540 | SA12K |
Stomatitis | 387 | CZ23Y |
Toxicity (grades 3 and 4) HRG – long stay | ||
Anaemia | 1099 | PA48B |
Febrile neutropenia | 3485 | PA45Z |
Allergic reaction | 1808 | WA11C |
Nausea | 856 | PA28B |
Diarrhoea | 1107 | PA26B |
Heart failure | 2290 | EB03E |
Thrombocytopenia | 1311 | SA12K |
Stomatitis | 1551 | CZ23Y |
Drug regimen (number of cycles) | Central line costs (£) | Drug costs (£) | Delivery costs (£) | Supportive medication | Medical oncology costs (£) | Specialist nurse review (£) | Blood tests (£) | Toxicity costsa (£) | Total cost (£) |
---|---|---|---|---|---|---|---|---|---|
FEC100 (6) | 18.17 | 346.38 | 1284.58 | 871.27 | 310.81 | 613.10 | 62.32 | 359.53 | 3866.17 |
FEC-T (3 + 3) | 18.17 | 306.84 | 1284.58 | 1238.07 | 450.03 | 613.10 | 62.32 | 378.18 | 4351.30 |
TC (4) | 18.17 | 211.20 | 856.39 | 15.91 | 310.81 | 408.74 | 41.55 | 158.16 | 2020.93 |
FEC75 (6) | 18.17 | 346.38 | 1284.58 | 80.77 | 310.81 | 613.10 | 62.32 | 239.69 | 2955.82 |
E-CMF (4 + 4) | 18.17 | 392.28 | 2569.16 | 1.54 | 450.03 | 817.47 | 124.64 | 360.10 | 4733.39 |
FEC-Pw (3 + 3) | 18.17 | 274.53 | 2569.16 | 435.89 | 450.03 | 613.10 | 124.64 | 378.18 | 4863.70 |
Parameter | Mean (£) | SE |
---|---|---|
Costs | ||
Disease free (annual cost excluding chemotherapy-related costs) | 1000.31 | 3.83 |
Disease free after local recurrence (annual, year 2 on) | 1354.17 | 151 |
Local recurrence (first year) | 6126.35 | 517 |
Distant recurrence (annual) | 1681.53 | 32 |
The mean annual costs of disease-free and cancer recurrence health states were estimated from hospital income recorded research157,167 (see Table 16). These costs are based on the national payment by results tariff produced by the UK Department of Health specific to each year in which they were incurred, adjusted for inflation to the base year for the analysis. 168 Patient data were censored at last follow-up contact and the Kaplan–Meier sample average cost method was used to adjust for censoring. 169 The annual cost of the disease-free state was based on year 2 onwards costs under the assumption that this represents the costs of follow-up minus chemotherapy costs.
Test costs
Test costs were calculated on a per-sample basis using current list prices and data from manufacturers. Where a list price was not available in the public domain, the manufacturers were asked for an expected UK price. Any anticipated NHS discounts were not considered. Any assumptions used in the cost calculations were based on expert opinion. Costs were converted to 2013 pounds sterling using the following exchange rates: GBP to euros = €0.825, and USD to GBP = £0.60.
All tests were assumed to be exempt from VAT. For tests conducted within the NHS it was assumed that all NHS purchasing was operated under a managed service contract (which excludes VAT); similarly tests conducted by commercial institutions for the NHS are exempt from VAT. 170 For tests conducted within NHS laboratories, labour costs were calculated based on estimates of the overall time to run assay samples, and did not include sectioning time, pathologist time to mark areas for extraction and for reporting. A summary of the per-sample test costs of all tests is provided in Table 17.
Test | Cost per test (£) |
---|---|
Oncotype DX | 2580.00 |
MammaPrint/BluePrint | 2207.00 |
Prosigna | 1672.00a |
MammaTyper | 1277.00a,b |
IHC4 AQUA | 720.00 |
IHC4 | 152.00a |
A breakdown of the cost calculations for each of the tests is given below.
Oncotype DX: for Oncotype DX, tests are sent to the manufacturer (Genomic Health) to complete and return, with no additional costs to the NHS. The cost for Oncotype DX is based on the manufacturer list price at the time of analysis (£2580).
MammaPrint/BluePrint: tests are sent to the manufacturer to complete and return, with no additional costs to the NHS. The advertised list price, confirmed by manufacturer at the time of analysis, was €2675 excluding VAT, equivalent to £2207.
Prosigna: testing requires the purchase or lease of a NanoString instrument in addition to individual assay kits in order to process samples within the NHS. The cost per test, therefore, depends on the machine capital costs (purchase and services), the assay cost, RNA extraction/preparation and the labour costs. These are summarised below.
-
Capital costs: machine purchase, lifetime and service costs were communicated by the manufacturer at the time of the analysis:
-
machine purchase – US$285,000 = £171,000
-
machine expected lifetime = 5 years
-
service cost – US$15,000/year = US$75,000/5 years = £45,000/5 years
-
total capital cost per site = £216,000 per 5 years.
-
The expected number of tests required is 4376 per year (Cancer Registry data171). Assuming five sites within the NHS, 875 tests are required per year, per site. Capital cost per test (five sites) is, therefore, £216,000/5 years/875 tests = £49.37. There is uncertainty around the number of instruments that would be purchased across England. Assuming three sites there is a lower (25th) quartile value of £29.61 and assuming 10 sites there is an upper (75th) quartile value of £98.72.
-
Assay cost:
-
Prosigna assay – £1277 (manufacturer quoted UK cost)
-
cartridge sizes – four or 10.
-
Each cartridge pack includes one quality assurance sample, and an entire cartridge must be used at once; any unused cartridges from the pack are wasted. Thus, a pack of four can run a maximum of three samples plus one quality assurance test, and a pack of 10 can run up to nine samples.
Assuming a 5-day working week, 52 weeks per year, and excluding Christmas and New Year (3 days), there are ≈257 [(52 × 5) – 3] working days in 1 year. Assuming that there are five sites, to complete 875 tests per year requires completing (on average) 3.4 tests per day per site, or 17 per week per site. It is assumed that assays are batched on a weekly basis; therefore, running 17 tests per week requires two cartridges of size 10, with wastage of one sample. We therefore assume that, on average, cartridge sizes of size 10 are used with one sample wasted.
The expected assay cost is, therefore, £1596.25 per test (£1277 × 10/8).
There is uncertainty around the number of samples that would be wasted per cartridge. This is represented in the model using a log-normal distribution with a lower bound of £1418.89 per test (assuming maximum number of nine samples run using cartridge size 10, i.e. £1277 × 10/9) and an upper bound of £5108 per test (assuming minimum number of one samples run using cartridge size 4, i.e. £1277 × 4/1), assuming these bounds are 95% CIs.
-
Labour cost: biomedical scientist time – assuming ‘batching’ of tests in groups of 17 (plus two controls) it is estimated that 11 hours of hands-on time is required for the macrodissection, RNA extraction and assay set-up. This equates to 39 minutes of biomedical scientist time per test. Valued at an Agenda for Change grade 7 technician hourly rate of £22.98, this equates to £14.94 labour cost.
-
RNA extraction/preparation materials:
-
US$500 for 25 isolations using the Roche kit (F. Hoffmann-La Roche Ltd, Basel, Switzerland) (US$20/sample = £12 per sample; communication with manufacturer).
-
Total cost per Prosigna test: capital cost per test (£49.37) plus assay cost (£1596.25) plus RNA extraction cost (£12) plus labour cost (£14.94) = £1672.56.
-
Incorporating uncertainties around the capital cost, assay cost and labour costs gives a log-normal distribution, with mean £1672.50 and SD £50.94 (µ = 7.422, σ = 0.030).
-
MammaTyper: testing requires the purchase or lease of a Roche LightCycler real-time PCR platform (F. Hoffmann-La Roche Ltd, Basel, Switzerland). The cost per test therefore depends on the machine capital costs (purchase and servicing), the assay cost, RNA extraction, preparation and the labour costs. These are summarised below.
-
Capital costs: capital costs include a Roche Diagnostics LightCycler real-time PCR machine. Purchase and service costs of the LightCycler machine were communicated by Roche at the time of the analysis:
-
LightCycler LC/Z480 (96-well) machine purchase (F. Hoffmann-La Roche Ltd, Basel, Switzerland) – £23,500
-
machine expected lifetime – 10 years
-
service cost – 10% of purchase cost from year 2 = £21,150 (to 10 years)
-
total capital cost per site = £44,650 over 10 years.
-
The expected number of tests required is 4376 per year (bespoke analysis by Public Health England Cancer Registry171). Assuming five sites within the NHS, then 875 tests are required per year, per site. Capital cost per test (five sites) is therefore (£44,650 × 5)/(4376 × 10) = £5.102. There is uncertainty around the number of instruments that would be purchased across England. Assuming three sites there is a lower (25th) quartile value of £3.061 and assuming 10 sites there is an upper (75th) quartile value of £10.203.
-
Assay cost:
-
MammaTyper assay – £1277 (interquartile range £400–1400) (analyst estimated UK price)
-
MammaTyper tests are purchased in batches of 10 (eight tests + two controls).
-
Two controls need to be included with each real-time PCR run. Controls can be reused/thawed up to three times.
Assuming a 5-day working week, 52 weeks per year, and excluding Christmas and New Year (3 days), there are ≈257 [(52 × 5)–3] working days in a year. Assuming five sites, to complete 875 tests per year requires completing (on average) 3.4 tests per day per site, or 17 per week per site. It is assumed that assays are batched on a weekly basis. Therefore, running 17 tests per week in a single batch with two controls.
The expected assay cost is, therefore, £1596 per test (price × 10/8) [interquantile range (IQR) £500–1750].
-
Labour cost: biomedical scientist time – assuming ‘batching’ of tests in groups of 17 (plus two controls) it is estimated that 11 hours of hands-on time are required for the macrodissection, RNA extraction and assay set-up. MammaTyper preparation, set-up of master mixes, distribution of master mixes and set-up of real-time instrument is estimated at 1 hour. This equates to 42 minutes of biomedical scientist time per test. Valued at an Agenda for Change grade 7 technician hourly rate of £22.98, this equates to £16.09 labour cost.
-
RNA extraction/preparation materials:
-
£283 for 50 isolations using the Roche kit (£12 per sample).
-
Total cost per MammaTyper test: capital cost per test (£5.102) plus assay cost (£1596) plus RNA extraction cost (£12) plus labour cost (£16.09) = £1629.19 (IQR £531.15–1788.29).
Incorporating uncertainties around the capital cost, assay cost and labour costs gives a log-normal distribution, with mean £1629.19 and SD £1905.11 (µ = 6.991, σ = 0.899).
IHC4 AQUA: price provided by the manufacturer in 2014 for in-house/central commercial testing was US$1200 (£720).
IHC4: for IHC4 performed using routine staining methods, costs of staining are relatively simple to estimate; however, owing to the quantitative nature of the IHC4 score, additional pathological assessment is required to accurately estimate ER histoscores, and PgR and Ki67 percentage-positive cells within the narrow bands (30 units or 10%) required.
Within the OPTIMA prelim study, IHC4 was performed in a central laboratory, using TMAs and image analysis, which does not reflect routine diagnostic practice (where whole slides are assessed) and precludes accurate assessment of test costs as they would be in a NHS setting. No formal measurement of the time required by individual pathologists to perform the additional quantification required for this test is available to provide a cost estimate was undertaken within OPTIMA prelim. For the purposes of the economic model, the time taken for IHC4 was estimated by consultation with NHS pathologists and laboratory managers.
It was assumed that all IHC4 testing was conducted at local hospitals and laboratories, using currently available technology. Block selection and retrieval was not costed, as these are already routinely conducted within the NHS. The cost of consultant time was assumed to be £157 per hour based on Personal Social Services Research Unit costs. 163 The calculation of the IHC4 cost per test was as follows:
-
ER – £26 (10 minutes) extra pathologist time
-
PR – £15 consumables/laboratory costs plus £26 (10 minutes) pathologist time
-
Ki67 – £20 consumables/laboratory costs plus £52 (20 minutes) extra pathologist time
-
HER2 – no extra cost
-
generation of IHC4 report via algorithm – £13 (5 minutes of pathologist time)
-
total cost per test = £152.
As there remains uncertainty about this estimate, which is based on expert opinion, it will be represented as an uncertain parameter in the OPTIMA model, with mean £152 and IQR £116–207 (SD £69) (implying that there is a 50% chance that the true cost lies within this range). This is represented in the model by a log-normal distribution with parameters µ= 4.93 and σ= 0.429.
Utilities
A literature review was carried out to update the relevant health utility values for the OPTIMA model. 172 Full details of the literature review and data extraction for utility values can be found in Appendices 1 and 7. The utility parameters used in the model are shown in Table 18.
Health state | Mean | SE | Distribution | Source |
---|---|---|---|---|
Starting utility | Age group specific
|
Fixed | NA | Kind et al., 1998173 |
Disease free (no chemotherapy)a | –0.003 | 0.03 | Log-normal | Campbell et al., 2011174 |
Disease free (on chemotherapy)a | –0.099 | 0.033 | Log-normal | Campbell et al., 2011174 |
Local recurrencea | –0.108 | 0.04 | Log-normal | Campbell et al., 2011174 |
Distant recurrencea | –0.303 | 0.16 | Log-normal | Campbell et al., 2011174 |
Congestive heart failure | 0.528 | 0.047 | Beta | Kirsch and McGuire, 2000175 |
Sensitivity analyses
Sensitivity analysis number 1: variable survival after recurrence
It is likely that survival duration after a recurrence will be different in patients who receive adjuvant chemotherapy and those who do not. In the base case, the annual post-recurrence probability of death was assumed to be 0.30 (SD 0.22). In sensitivity analysis number 1, the annual probability of death after recurrence varied depending on whether or not previous adjuvant chemotherapy had been given. Given that overall survival is available for the SWOG88-14 trial, the post-recurrence survival parameter was derived by calibrating the validation model against this outcome measure. The resulting annual probability of death following recurrence is, therefore, 0.40 (SD 0.17) for patients treated with adjuvant chemotherapy and 0.14 (SD 0.17) for patients who did not receive adjuvant chemotherapy and who had a low Oncotype DX RS.
Sensitivity analysis number 2: constant chemotherapy effect
In this sensitivity analysis we assumed that multiparameter tests have only prognostic ability, with no ability to predict the magnitude of benefit from chemotherapy. For this analysis the effect of chemotherapy was taken from the Oxford Overview meta-analysis and was applied to the predicted RFS over a 5-year period for patients receiving chemotherapy in the model. The hazard ratio for RFS for anthracycline chemotherapy was taken to be 0.69 (SE 0.04) over the first 5 years with an additional benefit from the addition of a taxane of 0.84 (95% CI 0.78 to 0.91). 9 A limitation of this approach is that it allows only incorporation of uncertainty around the forecast where full information is available about the prognostic model; such information is not available for Adjuvant!. Therefore, in an attempt to represent probable uncertainty, the sample SD of the Adjuvant! Online RFS estimates was taken as a proxy for the SE of individual estimates. In this model, specification of annual post-recurrence probability of death was assumed to be constant across groups with a mean of 0.30 (SD 0.22).
Sensitivity analysis number 3: all tests included
An additional analysis that included all tests was performed, ignoring barriers to implementation and irrespective of the clinical evidence for the tests. Where list prices were unavailable, these were sought from the manufacturers or estimated by the analyst.
Analysis and the handling of uncertainty
The cost-effectiveness analysis was conducted in accordance with the specifications of the NICE reference case. 148 Outcomes for effects are measured in life-years, which represent the mean number of years of life per patient and are measured by the area under the survival curve. Life-years were weighted by estimates of health-related quality of life, which are represented by utility values (such that death is represented by 0 and 1 is the best possible health), to produce QALYs. Cost outcomes were measured as the mean cost per patient. The final cost-effectiveness outcome measure – the ICER – is the difference in expected costs for each cohort divided by the difference in expected effects (cost per QALY). According to NICE, the threshold ICER for an intervention to be considered cost-effective in the UK ranges from £20,000 to £30,000. 148 The analytic perspective was that of the UK NHS; thus, only direct costs to the NHS were considered. The base year for costs was 2013. In the initial model based on the SWOG88-14 trial, the starting age of the patient cohort was 60 years, which is consistent with the average age of patients in the majority of trials in ER-positive, early-stage breast cancer. This was modified in accordance with the findings of OPTIMA prelim. The time horizon was the lifetime of the patient cohort, assuming that all patients had died by age 100 years. Both costs and benefits were discounted at 3.5% as recommended by the NICE reference case. Mathematical programming was implemented using the R statistical programming language (The R Foundation for Statistical computing, Vienna, Austria). The University of Leeds Advanced Research Computing Cluster, which is part of the Yorkshire White Rose High-Performance Computing Grid, was used for the analysis.
Probabilistic sensitivity analysis
To characterise overall uncertainty in the output measures, a probabilistic sensitivity analysis was conducted using Monte Carlo simulation. 176 This method comprises, for repeated model simulations, a random draw from statistical distributions of all model inputs to produce a distribution of model outputs. This distribution allows characterisation of uncertainty in the ICER, which, owing to the mathematical properties of the ICER, is expressed as a probability of cost-effectiveness rather than as CIs. The distributions from which this random draw was made were fitted to each input parameter using reported means and variance according to methods recommended by the NICE reference case. For example, the beta distribution was used for binomial proportions and utility weights, the Dirichlet distribution was used for multinomial proportions, and the log-normal distribution was used for relative risks, hazard ratios and costs. A further explanation of probabilistic sensitivity analysis and the simulation techniques used can be found in Briggs et al. 177 Analyses were an average of 10,000 simulations unless otherwise specified.
Value-of-information analysis
Value-of-information analysis provides a useful framework for setting priorities for further research into cost-effectiveness. This approach relies on the fact that resources that are spent on a new intervention will not be available to spend on alternative interventions. If we invest in a new intervention that is not the most cost-effective option, we could lose health (or money) that could have been gained by patients if we had invested in a more cost-effective intervention. Research (i.e. the gain of more information), therefore, has value if it reduces the risk of adopting an intervention that is not cost-effective. This value can be quantified as either lost health (measured in QALYs) or lost health-care resources (measured in monetary units). An overall maximum value can be calculated (the expected value of perfect information), which represents the burden of uncertainty on the decision-maker for a defined decision. Alternatively, a maximum value can be attributed to specific components of the evidence base [the expected value of perfect parameter information (EVPPI)].
The expected value of perfect information can be thought of as the opportunity cost (lost benefit) associated with the risk of making an incorrect decision. The value of information is related to both the estimated cost-effectiveness and the current level of uncertainty about the cost-effectiveness estimate. It is the value to society of reducing all uncertainty about a decision to zero and, therefore, represents a ceiling on the value of further research. It is useful for determining if further research, including clinical trials and test optimisation research, is worthwhile and, if so, the type of research that should be undertaken. The value of a defined research proposal can also be estimated by calculating the expected value of sample information (EVSI). EVSI calculation remains experimental and computationally expensive, which may limit its ability to inform a research design question in a timely manner. For this reason, EVPPI will form the main component of the analysis, supplemented, where possible, by EVSI.
Calculation of value of information in this study used the non-parametric methods that are well described by Briggs et al. 178 The results presented in this report were generated using a meta-modelling approach implemented by the Sheffield Accelerated Value of Information tool (http://savi.shef.ac.uk/SAVI/). 179 An annual discount rate of 3.5% is applied over a 10-year time period, assuming that this is the time over which the decision is relevant. Value is expressed as ‘net monetary benefit’ or ‘net health benefit’. This is a composite scale that allows financial costs and health benefits to be expressed in common units and relies on the decision rule for cost-effectiveness,
where ΔE and ΔC are the incremental effects and costs and λ is the willingness-to-pay threshold. This is rearranged, for example, to give:
Chapter 4 Results: recruitment and study conduct
Recruitment
The first centre opened to recruitment into the OPTIMA prelim study on 5 September 2012. There were 35 centres open to recruitment and, of these, 34 centres recruited participants. Between 16 October 2012 and 3 June 2014, when the database was locked, 350 participants were registered, of whom 313 were subsequently randomised into the study (Figure 4). The final 150 patients were recruited within 6 months at a rate of 28 patients consented (registered) or 25 patients randomised per month. The average recruitment rate was 0.8 patients consented per open site per month in the final 6 months of recruitment (0.7 over the whole recruitment period). The average recruitment rate for randomising patients was 0.7 patients randomised per open site per month in the final 6 months of recruitment (0.6 over the whole recruitment period) (Figure 5). Recruitment rates for recruiting centres ranged from 0.1 to 2.2 patients consenting (registered) per month open.
FIGURE 4.
Recruitment graph.

FIGURE 5.
Recruitment rate per open centres.

Screening and acceptability
A total of 968 patients were reported on the screening logs, of whom 795 were deemed eligible according to local information; however, 45 of these were excluded by clinicians (Figure 6). Of the 750 patients approached by their clinicians about participating in OPTIMA prelim, 350 consented to join the study. This equates to a 47% patient acceptance rate, which was above the target 40%. The acceptance rate ranged from 0% to 100% across the 35 open centres, with 19 (54%) centres having an acceptance rate above the target 40%.
FIGURE 6.
Screening of potential participants.
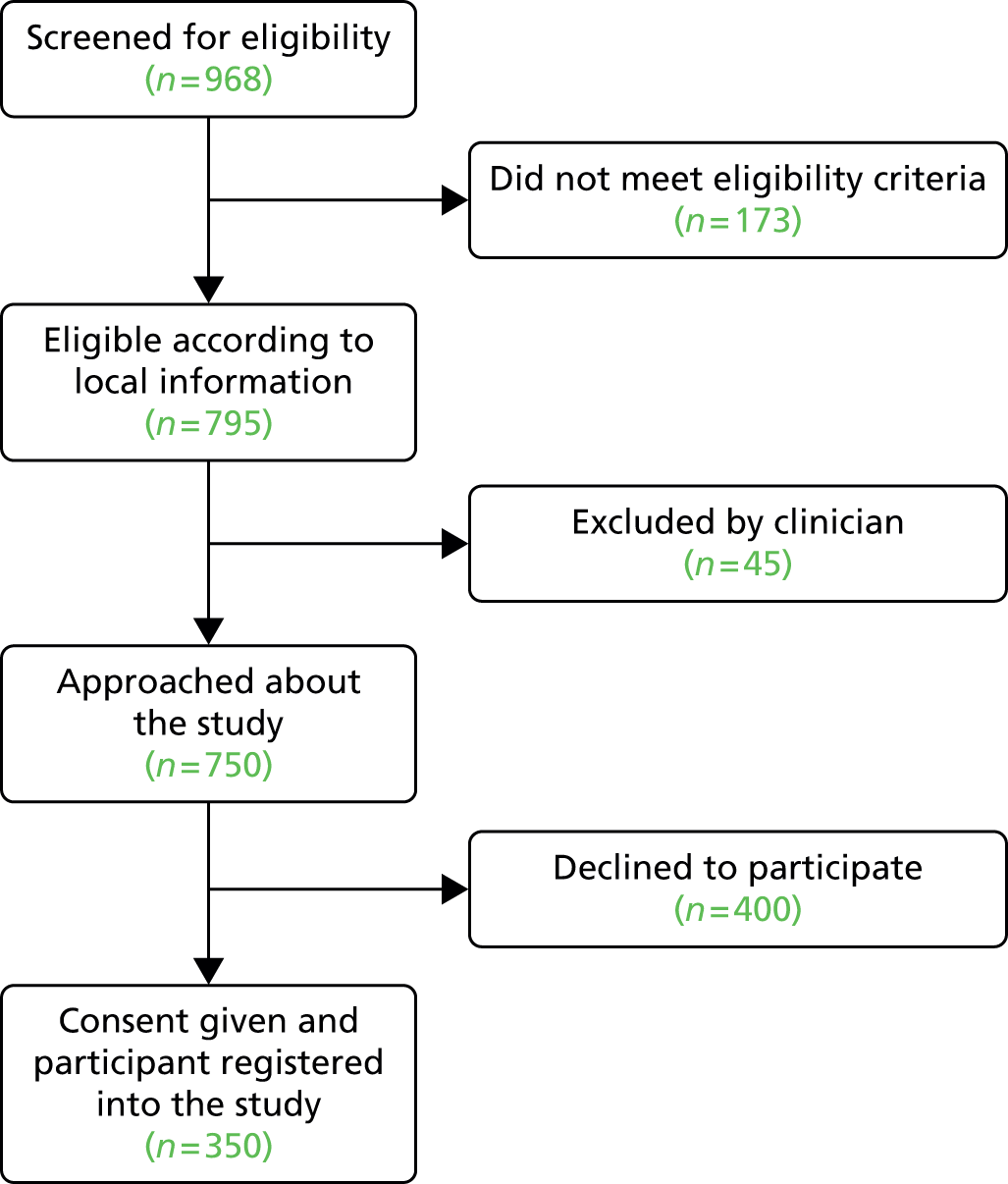
Patients did not have to give a reason for declining to take part but when a reason was given it was recorded on the screening log. Recorded reasons are summarised in Table 19. Of those who declined to enter the study, 51% did so because they had a strong treatment preference (33% wanted chemotherapy and 18% did not want to have chemotherapy). Some patients who declined to enter the study (8%) chose to pay privately for the Oncotype DX test.
Patient-reported reason | Number of patients reporting this reason | Percentage of those declining (%) |
---|---|---|
Wanted to receive chemotherapy | 133 | 33 |
No reason given | 98 | 25 |
Did not want to receive chemotherapy | 70 | 18 |
Decided to pay for Oncotype DX test privately | 30 | 8 |
Did not want to participate in a clinical trial | 30 | 8 |
Did not want to delay treatment start | 14 | 4 |
Declined any further treatment | 4 | 1 |
PIS sounded too risky | 5 | 1 |
Did not want to travel to the hospital participating in OPTIMA prelim for treatment | 5 | 1 |
Unable to make decision | 3 | 1 |
Uncertain of the Oncotype DX test | 2 | 1 |
Patient did not understand the PIS owing to poor understanding of English | 2 | 1 |
Able to have Oncotype DX test on NHS | 1 | 0 |
Uncertain if want chemotherapy or not | 1 | 0 |
PIS too complicated | 2 | 1 |
Registered patients who were not randomised
Of the 350 patients who consented to participate in the OPTIMA prelim, 313 had been randomised prior to 3 June 2014 and 15 were undergoing central eligibility confirmation on that date (Figure 7).
FIGURE 7.
The CONSORT diagram.

A total of 22 (6%) registered patients were not randomised (see Figure 7) for the following reasons:
-
referring site realised that patient did not meet eligibility criteria (n = 2)
-
HRT was being taken at the time of breast cancer surgery (n = 1)
-
repeat local receptor testing showed tumour to be ER negative (n = 1)
-
-
insufficient invasive tissue to perform Oncotype DX test and therefore ineligible (n = 1)
-
withdrawal of patient by their clinician (n = 2)
-
underlying condition that made patient unsuitable for the trial (n = 1)
-
axillary surgery had been delayed and needed to begin chemotherapy immediately (n = 1)
-
-
patient withdrew consent (n = 5)
-
sample submitted for central review did not contain sufficient invasive tumour for testing and patient did not want to wait for another sample to be sent and tested (n = 3)
-
decided to pay for an Oncotype DX test privately (n = 1)
-
decided did not want to receive chemotherapy (n = 1)
-
-
deemed ineligible on central review of receptor status (n = 12).
The discrepancy between local and central determination of receptor status was low at 3.7% (95% CI 1.7% to 5.8%), with only 12 of the 325 registered patients for whom central review of receptors was performed deemed ineligible (Table 20).
Case | Central ER results | Central HER2 results | Outcome | |||||
---|---|---|---|---|---|---|---|---|
Status | Quick score | % positivity | HER2 gene status | HER2 gene ratio | Average CEP17 copy number per cell | Average HER2 copy number per cell | ||
A | Negative | 0 | 0 | Non-amplified (≤ 1.79) | 1.19 | 1.8 | 2.15 | Heterogeneous tumour. Part of tumour strongly ER-positive (which local receptor status was determined on) and part of the tumour ER-negative |
B | Negative | 0 | 0 | Non-amplified (≤ 1.79) | 1 | 2.35 | 2.35 | Local ER-positive result owing to faulty staining of slide. ER repeated on core biopsy using different antibody clone, which showed negative staining for ER |
C | Negative | 0 | 0 | Non-amplified (≤ 1.79) | 1.21 | 2.15 | 2.6 | Local ER-positive result was because of local error, the core biopsy was retested and ER-negative |
D | Negative | 0 | 0 | Amplified (> 2.20) | 3.59 | 1.1 | 3.95 | Local difficulties in retesting core biopsy. However, decision taken to treat patient as HER2-positive (according to central test) and ER-positive (according to original biopsy) |
E | Negative | 0 | 0 | Non-amplified (≤ 1.79) | – | – | – | Heterogeneous tumour. Tumour shows two different cell populations. One completely negative for ER and a second one (probably lobular carcinoma) strongly positive in all the cells |
F | Positive | 8 | 98 | Borderline amplified (2.00–2.20) | 2 | 2.78 | 5.55 | FISH repeated locally; remained HER2-negative |
Ga | Positive | 8 | 98 | Borderline amplified (2.00–2.20) | 2.14 | 1.65 | 3.53 | Local decision to treat according to HER2 status from UCL-AD as this was performed on the excision specimen and the local HER2 was performed on the core biopsy |
Ga | Positive | 8 | 98 | Borderline amplified (2.00–2.20) | 2.06 | 1.69 | 3.47 | |
H | Negative | 0 | 0 | Not done | – | – | – | Local ER repeated on core and surgical resection. Assessed these as low positive; Allred 5 on core biopsy and Allred 3 on resection. Decision taken to treat with chemotherapy and endocrine therapy as per local results |
I | Positive | 7 | 95 | Borderline amplified (2.00–2.20) | 2.2 | 1.43 | 3.14 | Unknown |
J | Positive | 8 | 95 | Amplified (> 2.20) | 2.7 | 2 | 5.4 | Unknown |
K | Positive | 8 | 95 | Amplified (> 2.20) | 2.39 | 1.55 | 3.7 | Unknown |
L | Positive | 8 | 98 | Amplified (> 2.20) | 2.81 | 1.35 | 3.8 | Unknown |
Randomised participants
The characteristics of the 313 randomised patients are shown in Table 21. Patients were allocated to the partially blinded trial arms (157 on arm A and 156 on arm B) and their characteristics were well balanced across trial arms.
Characteristic | Arm A,a n (%) | Arm B,a n (%) | Total, n (%) |
---|---|---|---|
Number of patients | 157 (50) | 156 (50) | 313 |
Age in years, median (range) | 57 (40–78) | 58 (40–78) | 58 (40–78) |
Stratification variables | |||
Menopausal status of participant | |||
Pre-menopausal | 52 (33) | 50 (32) | 102 (33) |
Post-menopausal | 105 (67) | 106 (68) | 211 (67) |
Number of involved nodes | |||
None | 31 (20) | 30 (19) | 61 (19) |
1–3 | 99 (63) | 99 (63) | 198 (63) |
4–9 | 22 (14) | 21 (14) | 43 (14) |
Positive sentinel node biopsy without clearance surgeryb | 5 (3) | 6 (4) | 11 (4) |
Intended chemotherapy regimen | |||
Anthracycline–non-taxane | 62 (40) | 61 (39) | 116 (39) |
FEC75–80 | 42 (27) | 50 (32) | 92 (29) |
FEC90–100 | 9 (6) | 7 (4) | 16 (5) |
E-CMF | 11 (7) | 4 (3) | 15 (5) |
Taxane–non-anthracycline (TC) | 17 (11) | 18 (12) | 35 (11) |
Anthracycline–taxane | 78 (49) | 77 (49) | 155 (50) |
FEC-T | 78 (49) | 74 (47) | 152 (49) |
FEC-Pw | 0 (0) | 3 (2) | 3 (1) |
Tumour characteristics | |||
Histological grade | |||
1 | 9 (6) | 11 (7) | 20 (6) |
2 | 105 (67) | 105 (67) | 210 (67) |
3 | 43 (27) | 40 (26) | 83 (27) |
Tumour size in mm, median (range) | 28 (2–150) | 27 (7–170) | 28 (2–170) |
≤ 30 | 91 (58) | 90 (58) | 181 (58) |
> 30 | 66 (42) | 66 (42) | 132 (42) |
Lymphovascular invasion reported | |||
No | 84 (54) | 90 (58) | 174 (56) |
Yes | 66 (42) | 62 (40) | 128 (40) |
Not known | 7 (4) | 4 (2) | 11 (4) |
Tumour type | |||
Ductal | 110 (70) | 113 (72) | 223 (71) |
Lobular | 31 (20) | 36 (23) | 67 (21) |
Tubular/cribriform | 2 (1) | 0 (0) | 2 (1) |
Mucinous | 3 (2) | 1 (1) | 4 (1) |
Micropapillary | 1 (1) | 0 (0) | 1 (1) |
Mixed | 10 (6) | 6 (4) | 16 (5) |
Withdrawal post randomisation
Four participants withdrew consent for the trial following randomisation but before receiving their treatment allocation (see Figure 7). Of these, two patients withdrew consent because of the delay in treatment allocation. One patient was withdrawn by her clinician because she had received preoperative letrozole and was therefore ineligible. One patient was withdrawn because, prior to treatment allocation, it was found that there was insufficient invasive tumour to obtain an Oncotype DX test and no additional sample was available.
A further three participants withdrew following treatment allocation. One patient was withdrawn by her clinician as a subsequent bone scan revealed metastatic disease and two patients withdrew consent as they chose not to follow the results of the randomisation; one patient decided to pay for Oncotype DX off-study and one did not accept her trial-allocated treatment (chemotherapy).
These seven patients are included in the baseline data, but no further information is available.
Sample handling logistics
The median time between patients signing the OPTIMA prelim consent form to treatment being allocated was 20 days (IQR 16–23 days, range 10–41 days). Treatment allocation took longer than 4 weeks from date of consent for 25 patients (8%). The main reasons for these delays were staffing problems and issues within the central laboratory (10 patients) and obtaining a suitable sample from centres for testing (11 patients). Furthermore, central eligibility testing took substantially longer than the target timeline for two patients and Oncotype DX testing took substantially longer for one patient.
The median time between patients signing the OPTIMA prelim consent form and starting chemotherapy for those allocated to chemotherapy was 4.6 weeks (IQR 4.1–5.3 weeks, range 2.6–11.3 weeks). The proportion of chemotherapy-assigned patients starting treatment within 6 weeks was 91% over the whole recruitment period, but 92% within the last 6 months of recruitment. The delays with allocating chemotherapy because of problems with the tissue samples led to the withdrawal of four registered patients and two randomised patients prior to treatment allocation.
Recruitment conclusions
OPTIMA prelim had three pre-defined success criteria for establishing feasibility, namely that 300 patients would be recruited in no more than 2 years from the first centre opening, and, for the final 150 patients, (1) patient acceptance rate should be at least 40%; (2) recruitment should take no longer than 6 months; and (3) chemotherapy should start within 6 weeks of signing the OPTIMA prelim consent form for no less than 85% of chemotherapy-assigned patients. All of these criteria have been met demonstrating that a large-scale randomised trial to demonstrate the effectiveness of multiparameter test-directed chemotherapy allocation in a high-risk population of patients with ER-positive HER2-negative chemotherapy is feasible in the UK.
Involving patients in developing the study
Three patient focus groups met to discuss the OPTIMA trial; two of these were held shortly before and after the study opened (in August 2012 and February 2013) and the third discussion, which involved a mixed patient group, took place in a non-participating centre in April 2013. Members of ICPV facilitated all three groups. Although the conversations took different directions, it is possible to extrapolate certain themes that recurred throughout and within the groups. These illustrated the ‘big picture’ and explained some of the possible difficulties and opportunities that these groups felt would be involved in making a decision to take part in the OPTIMA study. These are described below.
Personalised chemotherapy
Perceptions of chemotherapy and the move towards more test-guided treatment, such as that evaluated by the OPTIMA trial, were discussed at length in all the groups. Although a small number of participants saw the benefits in receiving no or less chemotherapy, they felt most patients would want to treat breast cancer as thoroughly as possible, which meant having chemotherapy. Focus group participants acknowledged that test-guided treatment was becoming more commonplace now (e.g. those mentioned by group members included HER2 and ER status testing and tests required for treatment of haematological malignancy). However, they felt that there was not always a clear understanding if and when chemotherapy was needed, and that some patients ignored potential side effects in their enthusiasm for treatment.
Overall, it was felt that there was a need for contemporary information, both generally in the media and more specifically, such as in the OPTIMA prelim participant information leaflet, so that the expectation of chemotherapy, and the view that more chemotherapy is always better and that less is somehow deficient, will be challenged by both patients and within society. One group felt that this was a view perpetuated by health professionals and should be confronted through education. They felt that endocrine therapy, such as tamoxifen, was not, as one participant described, ‘painted in the same light’ by health professionals and was therefore seen to be less important and less effective. Participants stated that evidence-based information must be made widely available to make people aware that every person is different; that there is a multiplicity of breast cancer diagnoses and thus every treatment is different and ‘personalised’. They felt this would manage expectations more appropriately and prevent inappropriate comparisons with other women. They also felt that it needed to be explained that best practice changes over time.
Focus group participants felt that there should be more information made available in the news media and magazines about personalised, test-guided treatments to make the public aware and change perceptions generally about cancer always requiring chemotherapy.
It was agreed that the best way to introduce the study to potential OPTIMA participants would be to explain that breast cancer treatment is always tailored to the individual woman and it should be stressed that the OPTIMA trial involves personalised treatment, rather than less treatment, in any written information about the study.
There was some concern from focus group participants that people would think that less treatment, as prescribed in the OPTIMA trial, was simply a cost-saving measure by the NHS. They felt it should be made explicit to potential study participants that this was not the case.
Deciding to take part in research and the OPTIMA study
There was a consensus that the OPTIMA study seemed to be an appropriate and helpful study from a PPI perspective and focus group participants said they would take part in principle (one woman had actually been offered and consented to OPTIMA prelim). The reasons why they would take part were both general to research and specific to the OPTIMA study. For example, being invited to take part in research made one feel valued and important, and it was seen to make a difference to treatment and potentially help others. It also made individuals feel ‘looked after’ and the additional follow-up and ‘active monitoring’ that was involved, even within the control group, was highly valued. Participants felt that this was specifically the case in the OPTIMA study, in which the regular and active monitoring involved was seen as positive and information given about individual risk was seen as constructive.
The hypothetical question of making an informed choice to take part in the OPTIMA study was appreciated to be very difficult; focus group participants in all three groups wished this to be noted. Describing their own experience of being invited to take part in RCTs, some participants had felt under enormous pressure to make a quick decision. A minority felt that deciding to take part would have caused too much personal anxiety and so they would have preferred to leave the decision to, as described by one participant, ‘those that were qualified’. Another minority felt that they would be deterred from taking part because of the wait to get results and consequent delay in starting treatment. There was a common misconception among focus group participants that chemotherapy had to be instigated very quickly to have the most beneficial effect but they were reassured that, in breast cancer, adjuvant chemotherapy could be delayed for up to 12 weeks. 180 Participants felt that this should be made very clear to potential OPTIMA study participants. Knowing this, they also felt that the complexity of the OPTIMA trial and the possibility of potentially receiving less treatment would mean that the information and consent process would be likely to take longer and research staff should be made aware and of this and trained, if appropriate.
The information provided for the OPTIMA study
Focus group participants from all three groups stressed the importance of an expert multidisciplinary team to manage the OPTIMA study involvement and treatment in which potential study participants could have ready access. In order for the OPTIMA study participants to have confidence in their treatment allocation and in their decision to consent to the study, they agreed they would need confidence in the information they were given and the person giving it.
All focus groups also agreed that an audio-recording of the initial consultation would be positive for potential OPTIMA study participants to take away with them as discussions and information given in consultations often, as one participant described, ‘went in one ear and out of the other’. It was also felt that it was important for the OPTIMA study information to be made available so that it could be discussed with family members and friends away from the hospital environment. Multiple formats, which would allow patient choice and be appropriate for different people, were preferable and formats described included digital versatile discs, leaflets, websites and podcasts. Independent information was also valued and it was felt that this could be hosted by a neutral organisation such as ICPV or a cancer charity. The provision of full information was felt to be important and would encourage rather than discourage recruitment.
There was also some concern about the partial blinding in the OPTIMA study and the inherent lack of information. For example, would trial participants allocated to no chemotherapy worry that they actually had a borderline score? Focus group participants were reassured that borderline scores of the genomic test had been lowered specifically for the purposes of the trial to ensure safety. Focus group participants felt that if potential OPTIMA participants were told this, they might be more likely to consent knowing that there was less risk of a false-negative score. They also felt strongly that potential study participants should be made aware that chemotherapy could be offered at a later date if necessary, as many had been under the impression that the test would predict chemotherapy use indefinitely, which is not the case.
The additional burdens of chemotherapy
Interestingly, focus group participants felt that the important additional burdens of treatment were often ignored by health professionals and were under-reported and therefore not recognised. These were often related to employment and financial issues. As such, the health economics questionnaire that is included in the OPTIMA study was seen to be particularly useful and important. The additional burdens that were discussed in the groups are reproduced in Box 1. The quality-of-life questionnaires that are included as part of the trial were also perceived as highly relevant and valuable, although some individuals felt that certain important and impactful information would not be gathered by the measures proposed, for example the impact of tamoxifen and AIs on sexuality and sexual function.
Wigs, scarves and hats.
Complementary therapy, for example counselling, reflexology.
Gym membership for exercise and physiotherapy.
Child care.
New clothes because of weight change/‘hot flushes’.
Extra washing/heating costs (owing to vasomotor symptoms).
Increased cost of food (more preprepared food, more fresh food, more wastage).
New shoes (to accommodate fallen arches).
Increased cost of skin care, for example moisturisers.
Increased cost of holidays (need for better accommodation, costly travel insurance).
Patient and public involvement conclusion
Data from the three focus groups and the dissemination of these results have generated invaluable in-depth information about the potential impact of taking part in the OPTIMA study from people who have a direct experience of living with cancer. It has shown us that the study is supported and, in theory, people would be keen to be involved and take part. However, it has highlighted the difficulties involved in accepting randomisation to potentially less treatment than expected. The data that have been generated by the focus group participants have been incorporated into the study design and, although they cannot be truly quantified, have probably contributed to the success of the study.
Qualitative recruitment study results
The integrated QRS undertaken within the OPTIMA prelim study was based on a refinement of the methods developed for the ProtecT study complex recruitment intervention. 140 This section reports on the details of the data collected and analysed, and presents the findings from each of the three phases of the QRS. Detailed results from the analysis of phase 1 (including the recruitment difficulties identified through interviews with the OPTIMA staff members and audio-recordings of recruitment consultations) are reported in full in Appendix 8.
A summary of those findings is presented in this section, followed by the full findings from phase 2 (describing the delivery of interventions to address the barriers identified from phase 1) and phase 3 (considering the impact of the QRS interventions on recruitment during OPTIMA prelim).
Qualitative recruitment study data set
In total, 18 of the 32 staff contacted for interview responded: 14 agreed to participate (no withdrawals), two declined outright and two declined the interview at the time, with the offer of being contacted at a later more convenient date. The 14 semistructured interviews were conducted with participants across six centres and included eight oncologists (three of whom were also members of the TMG), four research nurses, one surgeon and a non-recruiting member of the TMG. Six interviews were conducted face to face and eight were conducted via telephone. Interviews were conducted with staff from ‘mid-range’ centres by chance rather than by design. One of the centres included in the QRS had failed to recruit any patients; the remaining centres’ recruitment rates ranged from 0.32 to 1.85 patients per month compared with an overall range of 0 to 2.20 in the study. At least one site from each of the OPTIMA prelim’s geographical clusters participated in interviews.
Of the eight research nurses invited to take part in structured telephone interviews about patient pathways, all agreed to participate and none withdrew.
A total of 36 audio-recordings were obtained from 29 patients’ consultations. First and second oncology consultations were recorded for 7 of the 29 patients. First oncology consultations were available for 21 patients and the second oncology consultation was available for one patient. Twelve different recruiters (10 oncologists and two registrars) led the consultations and audio-recordings were obtained from all geographical clusters.
Phase 1: understanding recruitment issues
Detailed findings are reported in Appendix 8. In summary, the recruitment issues identified through the QRS fell under three overarching themes:
-
eligibility processes and patient pathways
-
missed opportunities for discussing the trial
-
opportunities for improving communication and information provision.
The sources of data outlined earlier (see Qualitative recruitment study data set) were considered in tandem to identify key recruitment challenges in the OPTIMA prelim. Although there was some overlap in identified recruitment challenges, which emerged through different methods of data collection, there was also a tendency for certain methods to be better suited for uncovering certain challenges to recruitment. For instance, challenges relating to communication were largely uncovered through audio-recorded consultations, while issues relating to patient pathways were largely based on information derived from interviews. Some themes, such as recruiters’ levels of equipoise, were supported through both interview data and audio-recorded consultations.
Eligibility processes and patient pathways
‘Eligibility processes’ relate to clinicians’ and triallists’ decision-making about patients’ eligibility for the OPTIMA prelim study, while ‘patient pathways’ refers to the series of OPTIMA prelim-related events patients experienced in the lead up to entering (or declining) the trial. These processes had potential to hinder recruitment and so provided an initial focus for phase 1. Obtaining an overview of these processes from OPTIMA prelim staff also provided a useful framework for placing specific barriers to recruitment into context. A summary of these processes is presented in the paragraphs below, with more detailed descriptions shown in Appendix 8.
Although trial eligibility processes appeared straightforward, systems were not always in place to map eligibility from the first point at which patients were considered for the study [i.e. within multidisciplinary team (MDT) meetings]. At the commencement of the trial, screening logs recorded patients from only the point of registration and randomisation, thus making it difficult to elucidate the full patient pathway. This missing information pertaining to the screening process probably led to potentially eligible patients not being offered the opportunity to consider the study.
Patient pathways were clear, simple and appeared successfully integrated into research nurses’ services. However, patients sometimes gave decisions about participation via telephone conversations with research nurses rather than in face-to-face consultations. Consequently, some patients may not have discussed their thoughts or concerns about the OPTIMA prelim study with an oncologist. This had implications for clinicians’ opportunities to resolve patient misunderstanding and/or concerns (if appropriate) and limited scope for the QRS to capture discussions for or against trial participation (i.e. through audio-recordings).
Missed opportunities for discussing the OPTIMA prelim with patients
Interviews and early TMG meetings showed recurring concern among staff that fewer than anticipated patients were being approached about OPTIMA prelim. Regular telephone conversations with research nurses frequently suggested that no eligible patients had been identified. Six interview informants from five different centres talked about the limited flow of eligible patients they had encountered at the time of interview. This was the most frequently reported issue raised by informants when asked about their views on the main barriers to recruitment:
I don’t know why we’re not actually identifying (any); it’s not that we’re deciding that we’re . . . no, it’s not that we’re approaching patients and they’re saying ‘No’.
Research nurse (RN) 1
With the starting pool of potential eligible patients unknown, little could be deduced with regards to the numbers of patients deemed ineligible or the associated underlying reasons for ineligibility. This led to TMG members producing broad, non-specific explanations of why the number of patients approached for OPTIMA prelim was lower than expected when the study was conceived:
[Dr A] will probably tell you but at the meeting yesterday [they] said how surprised [they were] about how small the pool of eligible patients is. It’s much smaller than (they) thought it would be [. . .]; that’s like a change of tune, really, from before we started recruiting (they) were very positive about how big the pool was [. . .]. So I think (their) experiences in trying to identify eligible patients have shown that perhaps the expectation of how many eligible patients there would be, were a bit, perhaps slightly over-enthusiastic.
TMG member
Further evidence supporting the theory that the full pool of eligible patients was not being offered participation in the study came in the form of recruitment figures and acceptance rates. Actual recruitment figures trailed behind target figures throughout the recruitment period, while ‘acceptance’ rates consistently exceeded targets. Interviews with recruiters gave insight into potential attitudinal factors that may have played a role in restricting the pool of eligible patients. In summary, these factors related to the following.
Discomfort surrounding eligibility criteria
Recruiters’ unease surrounding various aspects of the eligibility criteria was widespread, reported by staff members from every centre participating in interviews. OPTIMA prelim staff varied in their readiness to accept increasing risk (in terms of disease status). Increasing lymph node involvement, tumour size and grade caused discomfort surrounding the upper thresholds of the eligibility criteria stated in the protocol.
Concern about patients’ ability to handle information
Some clinical informants gave general or specific examples of refraining from offering the trial to patients on the basis of their clinical judgement. Failure to approach patients at a clinician’s discretion would not breach the study protocol; the eligibility criteria clearly stated:
Patient must be fit to receive chemotherapy and other trial-specified treatments with no concomitant medical, psychiatric or social problems that might interfere with informed consent, treatment compliance or follow up.
However, an issue that arises is whether or not the decision not to inform patients about the opportunity to take part in the OPTIMA prelim study was always appropriate or well founded. Such judgements, if applied differently across different centres, could have had implications for the numbers of patients being approached for the OPTIMA prelim.
Priority of the OPTIMA study at multidisciplinary team meetings and among surgeons
Although research nurses were well versed in the inclusion and exclusion criteria, some felt that they did not always have the necessary expertise or voice to flag a patient as an OPTIMA prelim candidate at a MDT meeting. This suggested that potentially eligible patients were at risk of falling through the net at the stage of initial eligibility screening.
Summary of missed opportunities to discuss the OPTIMA prelim study
To summarise, findings from QRS interviews and informal discussion/observation in meetings suggested that there had been missed opportunities to approach eligible patients about the OPTIMA prelim study. This was a key finding from the QRS and suggested that there would be clear opportunities to increase levels of recruitment. These findings were supported through the observation that, although numbers of eligible participants were falling short of targets, the study seemed very acceptable to patients who were informed about it, as the percentage of eligible patients agreeing to randomisation consistently exceeded targets (see Screening and acceptability).
Opportunities for improving communication and information provision
Communication and information provision were largely explored through audio-recorded consultations, although data from staff interviews sometimes provided a useful backdrop for exploring particular challenges. Recruitment consultations varied in length and structure across and between centres, with some formats more conducive to explaining the study than others. Difficulties emerged in relation to how trial-specific processes were explained to patients, and staff attitudes also played a role in influencing the direction consultations took – particularly when it came to exploring patients’ views and perspectives on OPTIMA prelim participation.
Explaining randomised controlled trial processes and concepts of uncertainty
A fundamental challenge to recruitment identified by interview informants was the perceived difficulty in explaining the OPTIMA prelim trial design. Having an arm that split into two further arms, combining random and test-directed treatment allocations, and the partially blinded design were all thought of as potentially confusing to patients. Some interview informants had directly experienced patients dismissing the trial on this basis:
This particular lady just didn’t go into any detail at all she said, I just didn’t know what it was talking about, and I said, and I tried to explain bits of it to her, but no, she said, I just, I don’t, I just want to, I don’t understand it, she just said locked her mind off completely to it.
RN4
In the light of the above, analysis of audio-recorded consultations focused on recruiters’ explanations of trial processes and scrutinised information exchange that led up to evidence of patient misconceptions or confusion. Particular practices were identified as being potentially detrimental to patient understanding. These included absent or incomplete explanations of ‘randomisation’, ‘blinding’ and unclear descriptions of the treatment(s) provided in each trial arm. Explanations of the trial arms also revealed examples of loaded terminology, including use of the words ‘standard’ and ‘experimental’ (see Appendix 8).
The issue of uncertainty was an important theme to arise from audio-recorded consultations, particularly in relation to the discussions about chemotherapy benefits and the accuracy of the test. There were differences in how recruiters framed chemotherapy provision at the start of the consultation, which in turn had implications for patient understanding. Recruiters’ explanations ranged from presenting chemotherapy as a treatment with definite benefits, to framing it as an uncertainty that required careful balancing of benefits and side effects/complications. There were occasional examples in which patients still believed that they would definitely benefit from and/or receive chemotherapy even after the trial had been explained. Uncertainty surrounding use of the Oncotype DX test in treatment decision-making was another difficult concept to communicate according to recruiters’ interview accounts (i.e. oncologists and research nurses). Overselling the benefits of the test could prompt patients to pay for the test privately, whereas an overly cautious approach ran the risk of obscuring any incentives for participating in OPTIMA prelim. These considerations raised the dilemma of how best to reassure patients while conveying the inherent risk of participating in a study in which treatment allocation may be determined by a test that requires further research:
The trouble is if in our initial interview we sort of really down play the test as not being useful, then nobody would want to go in the trial in the first place. And if we play it too much they think ‘well it’s such a great test, I don’t want to risk not having it in the trial’ and go and find out the information. So again, it’s that fine balance.
Oncologist (Onc) 4
The TMG meetings revealed that a particular point of concern was a line in the PIS that highlighted the uncertainty surrounding the test’s accuracy. Based on TMG members’ experiences (not audio-recorded), patients had reportedly refused the trial once they had read the following statement:
The test might not work so well for patients with larger tumours or involved lymph glands. We do not believe this to be the case. However if it was, then in the future we might realise that we should have given you chemotherapy.
It was noted that this issue reportedly materialised after (rather than within) the first oncology consultation (i.e. once the patients had read the information sheets). This prompted us to consider how the Oncotype DX test was described in consultations, with a focus on how uncertainty was communicated and responded to (see Appendix 8 for details). Overall, recruiters varied in how they conveyed uncertainty, which in turn had implications for patients’ understandings of the trial aims and design.
Tailoring consultations to the OPTIMA prelim study
Recruiters varied in the extent to which they presented OPTIMA prelim as the primary focus of the discussion. This was influenced by the extent to which they covered diagnostic information and non-OPTIMA prelim-related treatments within the first oncology consultation. Some consultations were particularly long (up to 52 minutes), covering non-trial-related information in some detail prior to introducing the OPTIMA prelim study. In some ways, it could be considered that many appointments were not ‘recruitment consultations’ at all. Although there was the opportunity to present the OPTIMA prelim study, this opportunity was not always fully utilised in some consultations. In other cases, the study was presented as a ‘next step’ in a series of ‘information blocks’, rather than the heart of the consultation. Some recruiters successfully tailored information provision around explanations of the trial, whereas other struggled to do this, resulting in the aims and key details of the trial becoming somewhat lost.
Patient preferences
Screening logs and interview informants’ accounts suggested that patients’ preference either for or against chemotherapy was the most common reason for declining the trial (50% of reasons for decline in final screening logs: 33% for and 17% against):
We have a very good functioning wider unit [. . .]. There’s nothing that’s blocking us from doing it other than patient preference.
Onc3
There were few (if any) examples in consultations where patients expressed strong preferences, but there were some examples where patients appeared to be influenced by other clinicians that they had seen prior to the oncologist (e.g. surgeons). There were also examples of patients showing misunderstandings about trial participation while explaining their decision to refuse the trial; however, the recruiters did not always address these misconceptions, instead accepting the patients’ decisions without further discussion. A common thread that ran throughout recruiters’ interviews was that breast cancer patients in particular were thought likely to have set preferences given the influence of the media, peers and the well-publicised nature of the disease. As such, some recruiters completely disassociated themselves from patients’ decisions about trial participation and many indicated that they would accept patients’ preferences with no further exploration. Recruiters’ discomfort in exploring patients’ decisions and/or preferences stemmed from concern that raising these issues would jeopardise their relationships with patients or leave them susceptible to accusations of coercion.
Delay
Patients’ concern about the delay attributed to waiting for the Oncotype DX test result was a dominant theme to emerge from audio-recorded consultations. Almost every consultation analysed supported this, with most patients asking about how long the testing process would take. Although some recruiters reassured patients that the delays associated with OPTIMA prelim had no implications for treatment safety, this was not consistently done across consultations. This, therefore, also represented a missed opportunity to address patients’ concerns and provide reassurance.
Summary of opportunities for improving communication and information provision
Analysis of audio-recorded consultations revealed opportunities to clarify and refine recruiters’ explanations of trial-specific processes to patients, reconsider use of terminology and frame explanations of treatment options around the OPTIMA prelim trial design. Both interview data and audio-recorded consultations revealed recruiters’ discomfort and reluctance to explore patients’ decisions to decline the trial. These attitudes stemmed from concerns of being seen to coerce and the belief that patients’ views are fixed at the outset of consultations. We found no evidence of clear ‘fixed’ patient preferences in the consultations we listened to, but there were examples of patient expectations for chemotherapy/no chemotherapy that had stemmed from previous interactions with clinicians (e.g. surgeons) prior to the first oncology consultation.
Summary of findings from QRS phase 1 and opportunities for intervention
Phase 1 of the QRS identified a number of challenges to recruitment from the analyses of interviews and recruitment consultations, including opportunities for improvement to discuss with the CfI and TMG with a view to developing a plan of action to be implemented in phase 2. The potential opportunities included:
-
Considering introducing screening logs at the stage of eligibility assessment. Screening logs could then record the recruitment process in greater detail, from the first point of identifying a potentially eligible patient, through to the patient’s decision to accept or decline the trial. This would reveal if as many potentially eligible patients as possible were being approached and informed about OPTIMA prelim, and help to identify centre-specific barriers to recruitment.
-
Discussing ‘on the ground’ perceptions of equipoise – including discomforts around particular eligibility criteria – to reach a consensus about the need to change the criteria or to discuss these issues more widely.
-
Considering whether or not the basis of decisions not to approach patients could be standardised or measured and whether or not they were appropriate, with a view to increasing opportunities for potentially eligible patients to decide for themselves whether or not to participate.
-
Considering how to raise the profile of OPTIMA prelim at MDT meetings and among surgeons.
-
Providing recruiters with support to explain the study design, justification and details more clearly; promoting early mention of OPTIMA prelim in consultations and encouraging recruiters to frame their explanations of treatment options around the OPTIMA prelim study design.
-
Exploring with recruiters how best to conceptualise issues of uncertainty and discuss those with patients in the context of the OPTIMA prelim trial.
-
Raising issues about the use of terminology that might not help recruitment, including the use of the words ‘standard’ and ‘experimental’, which suggest the trial arms are not equivalent.
-
Exploring with recruiters how best to describe advantages and disadvantages of chemotherapy and the Oncotype DX test so that patients could understand the key issues.
-
Exploring with recruiters how best to present issues of delays with Oncotype DX.
-
Exploring with recruiters opportunities to discuss the origin of patient preferences for or against chemotherapy and, in appropriate cases, to provide additional information that might alleviate inappropriate concerns – all with the aim of ensuring more informed decision-making about participation in the study.
Phase 2: developing interventions to address recruitment challenges
Identification of potential barriers to recruitment was an iterative process that spanned the entire recruitment period. As themes emerged from data collection, interventions were designed in collaboration with the TMG and delivered to centres as recruitment proceeded. This section will outline the timing and nature of these interventions, and discuss their function relative to the recruitment challenges identified in the previous section. A summary of the interventions delivered is shown below in Table 22.
Date | QRS intervention |
---|---|
17 June 2013 | Updated TMG about eligibility criteria discomfort (emerging findings from interviews); suggested clinician-to-clinician discussions |
22 July 2013 | Raw preliminary interview data on eligibility issues sent to CfI |
9 September 2013 | Reminded TMG about eligibility criteria concerns; raised importance of detailed records of entire eligibility assessment process; advised on the benefits of exploring patients’ treatment preferences |
4 October 2013 | Tips/guidance document circulated to all centres |
16 October 2013 | Edited PIS distributed to all centres |
18 October 2013 | Descriptive summary of interview findings sent to CfI |
8 November 2013 | Group feedback presented to Scottish centres |
14 January 2014 | Group feedback presented to South West England and Welsh centres |
31 January 2014 | Recruitment tips presented to newly opening centre |
10 February 2014 | Individual feedback provided for Onc8 |
6 March 2014 | Individual feedback provided for Onc9 |
13 March 2013 | Group feedback presented to centre 5 |
14 March 2013 | Individual feedback presented to Onc4 |
27 March 2013 | Individual feedback presented to Onc1 |
27 March 2013 | Group feedback provided for centre 3 |
Addressing issues of discomfort surrounding eligibility: Trial Management Group discussion, circulation of interview findings and clinician-to-clinician centre visits
The QRS interviews provided strong evidence of discomfort surrounding the OPTIMA prelim eligibility criteria, suggesting that the full pool of eligible patients was not being approached. The knowledge acquired from interviews was fed back to the CfI and ‘core TMG’ over a prolonged period, initially through comments on ‘preliminary’ findings from interviews and finally through more formalised written reports of interview findings produced for the CfI (18 October 2013). Findings were also shared generically in wider TMG meetings attended by oncologists from centres around the UK. TMG members’ responses to these findings suggested familiarity with the issues raised and opened up discussions about how clinicians’ discomfort surrounding higher-risk status patients could be addressed. One early suggestion generated by the core TMG was to reassure individual oncologists about high-risk cases by publicising their peers’ readiness to include these patients in the study. This suggestion was put into practice on the premise that awareness of what oncologists around the country were doing might alleviate individual oncologists’ concerns – particularly those in ‘isolated’ centres. Following this, the QRS team revisited recruiters’ discomfort surrounding eligibility criteria at a wider TMG meeting, suggesting that open discussion with non-recruiting centres might be beneficial (17 June 2013). In particular, the team felt it was important that these took the form of peer-to-peer discussions among clinical experts who fully understood the realities of embedded clinical practice. Clinical members of the core TMG made a series of centre visits following this, targeting non-recruiting centres. The function of these meetings was to openly address recruitment difficulties in a supportive and confidential environment. Centre visits continued throughout the duration of the recruitment period.
Amendments to the patient information sheet
Members of the TMG expressed concern that the PIS section outlining the ‘disadvantages’ of OPTIMA prelim participation prompted patients to decline the trial. This prompted the QRS team to review the PIS. This opportunity was used to review the PIS in its entirety, with a view to considering how patients could interpret the information. Elements of the study arm descriptions were clarified, but most amendments were concentrated on the ‘advantages’ and ‘disadvantages’ sections of the document. These sections needed to provide an honest and clear description of the trial-specific advantages and disadvantages of participation, in as balanced a way as possible. Visually, the disadvantages section of the document far outweighed the advantages section, yet it became apparent that some of the details stated as ‘disadvantages’ were repetitions of trial processes described elsewhere. Through discussion with the TMG, it was agreed that some of this information was not a specific disadvantage of trial participation and it was removed.
The particular line in the PIS that had created most concern among recruiters informed patients that the Oncotype DX test might not work and that they might find out that they ‘should have received chemotherapy’. Audio-recordings of recruiters’ descriptions of uncertainty surrounding the test were used to inform how this disadvantage could be conveyed. It was evident that some recruiters successfully communicated the same idea stated in the PIS, but preceded this with information about the current use of the test (i.e. in other countries and in different subgroups of breast cancer patients). Audio-recordings also showed examples of recruiters reassuring patients that the test had not been ‘plucked out of the air’. It was on this basis that information surrounding the test’s current use, and intended extended use, were added to the PIS. Similarly, we found evidence of recruiters tentatively stating that they believed the test would work, balancing this with the assertion that concrete evidence was not yet available. Similar information was added to the ‘disadvantages’ section of the PIS. Patient representatives and the Research Ethics Committee approved these amendments made to the PIS and the updated version was circulated in October 2013.
Tips and guidance for recruiters
The challenges associated with structuring consultations and explaining trial-specific processes were addressed through circulation of a tips and guidance sheet formulated by the QRS team (see Appendix 9). This document provided recommendations on how recruiters might explain difficult concepts such as randomisation, blinding and the events that occur in each treatment arm. Other details pertinent to the study rationale were also included to help recruiters structure their consultations.
All information stated within the tips and guidance document was grounded in the data collected from audio-recordings and based on the potential opportunities listed above. Suggestions about how to explain concepts were based on clear, effective examples derived from the collection of recorded consultations. The CfI contributed some key clinical concepts and the document as a whole was reviewed by the CfI prior to finalising. A letter from the CfI accompanied the document, with the option for recruiters to contact the QRS team if they had any questions. Importantly, the letter framed the tips and guidance as suggestions that recruiters may wish to incorporate into their current practices. The document was circulated to all open OPTIMA prelim centres in October 2013.
Group feedback sessions
A series of group feedback sessions was delivered to a range of QRS and non-QRS participating centres around the UK. The purpose of these feedback sessions was to spark discussion to generate acceptable solutions to recruitment challenges, using findings from the interviews and audio-recordings as prompts. The QRS team guided this process by showing extracts from consultations deemed more or less effective, with recruiters encouraged to comment on and evaluate the extracts themselves. Group feedback sessions were particularly targeted to addressing broad topics relevant to incorporating the OPTIMA prelim study into clinical practice rather than specific technical challenges (e.g. explaining difficult concepts, which were better addressed by the tips and guidance sheet). Topics addressed included discomfort surrounding eligibility criteria, approaches to structuring consultations and how to respond to patient preferences. Presentations were adapted to each feedback session, using updated recordings or focusing in on topics that were problematic for the centre in question. TMG members, particularly the CfI, assisted in the formulation and delivery of presentations; for instance, the CfI contributed a number of vignettes designed to generate discussion about eligibility and took the lead for this part of the presentation at one of the meetings.
Exploring patient preferences was covered in all feedback presentations. The widespread discomfort surrounding this was still apparent in meetings, but the QRS team attempted to show the merits of exploring preferences (through actual consultation data) and offered suggested ‘lead-ins’ to how recruiters might respond to a patient preference.
An attempt was made to deliver group feedback to as many regional clusters as possible by organising meetings that could be attended by representatives from multiple centres. Secondary to this option was to target centres that requested feedback and centres that appeared to be struggling with recruitment.
Four group feedback sessions were conducted in total: two attended by multiple centres and two directed at single centres. Meetings attended by multiple centres were conducted in Scotland and the south-west of England. Both regional meetings had at least one representative from local centres present. Centre-specific feedback meetings were conducted in a centre that requested feedback and a centre that had been struggling with recruitment.
Individual feedback sessions
In addition to group feedback sessions, recruiters who provided at least three audio-recorded consultations were offered confidential individual feedback, which was conducted over the telephone or in person. A summary sheet of key points discussed was provided to recruiters following this session. Individual feedback sessions were useful for discussing specific challenges individual recruiters faced, which might not have been appropriate to discuss in a public context (e.g. concerns of coercion). Furthermore, these sessions were an ideal opportunity to discuss challenges that were specific to the individual, rather than ‘recurring’ challenges addressed in group meetings. All guidance provided by the QRS team was offered in the form of suggestions that were discussed with the recruiters, making it clear that the individual should proceed as they feel best. In all cases, it was emphasised that recruiters needed to remain comfortable and confident while speaking with patients about OPTIMA prelim. In total, individual feedback was provided to four oncologists. These were delivered in the later stages of recruitment, given the need to base feedback on a sufficient number of recordings and the relatively low numbers of recordings that were made.
Phase 3: impact of qualitative recruitment study interventions
There were a number of approaches taken to evaluate the impact of the QRS, although no single method could definitively show the influence interventions had on recruitment. As a consequence, the discussions that follow should be viewed as speculative, although viewing the problem from multiple angles could contribute to a clearer picture than relying on one method alone. In the light of this, three approaches were used to explore the potential impact of the QRS interventions: analysis of how recruitment figures changed relative to interventions; analysis of recruitment figures for centres receiving group feedback versus those that did not; and a qualitative analysis of changes in practice for individual recruiters.
Changes in recruitment figures with respect to qualitative recruitment study interventions
Figures 4 and 5 show overall recruitment with time and monthly recruitment rate adjusted for the number of open centres. Recruitment rate fluctuated substantially from month to month but there was an overall upwards trend. The month of early October to early November 2013 coincide with a series of general QRS interventions that could have impacted all centres or large clusters of centres. It is apparent that the recruitment figures immediately following these interventions changed the trajectory, as rates of increase sped up and the gap between ‘target’ and ‘actual’ figures began to close. This trajectory continues for the remainder of the recruitment period, while the difference between ‘target’ and ‘actual’ recruitment figures continued to narrow. One would not expect recruitment rates to increase at the exact point at which QRS interventions were disseminated. A lag is to be expected, to account for the time needed for knowledge/advice to diffuse and for eligible patients to emerge.
The observations described above are far from conclusive evidence that the QRS had a positive impact on recruitment. Causality cannot be established based on these data, owing to the countless confounding factors that could have influenced recruitment and/or uptake of the interventions. For instance, we cannot prove definitively that recruiters took note of the interventions, and could speculate that the slight increase in recruitment could be attributed to any number of reasons. It should be noted, however, that this slight boost in recruitment could be considered unusual given the time of the year (December/Christmas shut-down).
Comparison of recruitment figures for centres with and without group feedback
Comparing centres that did/did not receive non-generic interventions was problematic, given that feedback sessions occurred at various points in time within each centre’s ‘recruiting trajectory’. Centres had been open to recruitment for different lengths of time, resulting in different levels of experience and (possibly) confidence among clinical staff. Comparing ‘feedback’ versus ‘no feedback’ centres was also problematic as there were difficulties in grouping ‘feedback centres’ in a single category: some centres received both individual and group feedback, while others received one or the other; some had their full clinical team present at feedback meetings, while others had one or two individuals present; some centres were visited by the QRS team or TMG, whereas others sent representatives to regional meetings; and, finally, the professional roles and numbers of those attending feedback meetings varied by centre (sometimes oncologists were present, sometimes research nurses, and sometimes both). Comparing recruitment rates for centres with/without feedback was further complicated by extraneous factors that could influence recruitment rates (e.g. centres size, location), including more elusive factors that were harder to measure (e.g. ‘commitment’ to the trial). None of these factors could be controlled or accounted for. The distribution of ‘generic guidance’ to all centres also raised the question of whether or not any ‘no feedback’ centres truly existed, as generic guidance often overlapped with the advice and recommendations offered in feedback meetings.
Despite these difficulties, there were some examples where QRS interventions or ‘clinician-to-clinician’ discussions had clear impact. For example, two centres that had not yet recruited, despite being open for numerous months, consistently recruited immediately after ‘clinician-to-clinician’ visits took place. Looking at changes in recruitment before and after group feedback sessions, it was noted that recruitment rates (patients per month) were almost always higher after these meetings took place (9 out of 11 centres). Once again, though, it is difficult to distinguish the impact of the QRS from the effects of ‘natural increasing momentum’; crude comparisons of change in recruitment rates could have been made with ‘no feedback’ centres if there was a single point of QRS intervention, but as mentioned above, variable timings of feedback delivery meant this was not possible.
Qualitative analysis of audio-recordings: do recruiters’ practices change?
One relatively straightforward approach to assessing the impact of QRS interventions was to look at whether or not suggestions in the tips and guidance sheet and feedback presentations were adopted by recruiters. Three recommendations were consistently covered in ‘generic’ QRS interventions (i.e. widespread advice to all centres), and were, therefore, ideal points to evaluate across centres before and after intervention. These were:
-
advice to draw out the arms of the trial and explain each route separately (e.g. ‘option 1′ and ‘option 2′)
-
recommendations to specify that randomisation means there is no control over allocation
-
advice to reassure patients that treatment delays associated with OPTIMA prelim were safe.
These points were also deemed the most important advice generated by the QRS team, having considered factors that most frequently appeared to lead to patient confusion or concern. Table 23 sets out whether or not each of these suggestions were detected in consultations occurring before (no shading) and after (green shading) the date of intervention. Examples occurring before intervention indicate that the recruiter was already doing this. The date of intervention was defined as the first point at which these recommendations were distributed (October 2013 – the ‘tips and guidance’ document). All feedback meetings also covered these points, all of which took place after October 2013. The data summarised in the table are based on targeted analysis of consultations (arranged by date) to focus specifically on the topics of interest. The word ‘Yes’ showed that the recruiter spontaneously demonstrated the behaviour/recommendation, while ‘Yesa/Yesb’ indicate that the behaviour was demonstrated in response to patient confusion (a) or questioning (b).
Centre | Consultation identifier | Draws diagram of trial arms? | Specifies randomisation = no control over allocation | Reassures about delay? |
---|---|---|---|---|
Centre 1 | OP8 | No | No | No |
OP9 | No | No | Yes | |
Centre 2 | OP1 | No | No | No |
OP2 | No | No | No | |
OP10 | No | No | No | |
OP12 | No | No | No | |
OP13 | No | No | No | |
OP14 | Yesa | No | Yes | |
OP17 | No | No | No | |
OP20 | Yes | Yes | Yes | |
OP21 | Yes | Yes | No | |
OP22 | Yes | Yes | Yes | |
OP26 | Yes | No | Yes | |
Centre 3 | OP1 | No | No | No |
OP2 | Yesa | No | No | |
OP4 | No | No | No | |
OP13 | No | No | No | |
OP14 | No | No | NA | |
Centre 4 | OP4 | No | No | Yes |
OP6 | No | No | No | |
OP8 | Yes | Yes | Yes | |
OP9 | Yes | No | Yes | |
Centre 5 | OP26 | No | No | NA |
OP27 | No | No | NA | |
OP28 | No | No | NA | |
OP30 | No | No | NA | |
Centre 6 | OP1 | Yes | Yesb | Yes |
OP1 | Yes | Yes | Yes |
There is a general pattern suggesting change in centres’ practices before and after the QRS intervention, although there are a few exceptions where practices were present before intervention and not adopted after intervention. Evidence to support real changes in practice was particularly strong where recruiters very clearly altered their approaches to communicating concepts. This was most apparent in recruiters’ descriptions of the study design before and after QRS intervention (specifically, their tendencies to draw out arms and describe each separately as two ‘options’). This is demonstrated through extracts from the following oncologist’s pre- and post-intervention explanations (Box 2). The changes apparent were similar for other recruiters who adopted the technique of drawing out the study arms.
What OPTIMA does, essentially, we do the Oncotype DX test on the tumour and then you get told whether you’re going to have chemotherapy or not [. . .]. Optima is a randomised study, so 50% of the women at random in this study will get standard treatment with chemotherapy, and the other 50% in this study will have the treatment decision made according to the results of the Oncotype tests.
Onc1
Intervention
November 2013 (post QRS intervention)
So we feed details about you into a computer, and it tells us whether you get the standard treatment, which is option 1, or the Oncotype test, which is option 2. So if you look at the diagram, the way it works is that if you are allocated to option 1, you get the chemotherapy that I’ve described, followed by hormone tablets for 5 years or 10. And you get your radiotherapy whilst you’re having your hormone tablets.
Onc1
OK.
Patient
You have option 2, um we send the tumour for an Oncotype test, and if it comes back as a high score then you get exactly the same as you would in option 1. But if it comes back as a low score, it says that chemotherapy is not likely to benefit you, and we’ll manage with oestrogen tablets alone.
Onc1
Not all elements of the QRS guidance and advice could be evaluated through qualitative analysis. Recruiters’ readiness to explore patient decisions and preferences was a clear opportunity for change, but actual practices could not be assessed owing to the limited numbers of audio-recorded consultations where patients voiced their decisions. On a more general level, there were limited opportunities to discuss pre-/post-intervention changes in practices for individual recruiters, as few (if any) had contributed enough recordings that could be compared before and after intervention.
Qualitative recruitment study conclusions
Phase 1 of the QRS provided considerable information about the process of recruitment, as it was enacted in clinical centres participating in OPTIMA prelim. Interviews, audio-recordings and screening logs all provided insights that enabled a detailed and nuanced understanding of the challenges faced by recruiters in explaining the complex issues inherent in the OPTIMA prelim study and its design. These understandings were transmitted to the CfI, TMG and recruiters in a range of ways, including direct discussion of eligibility and information issues with the CfI and TMG, and through individual feedback, group discussions, tips and guidance documents and a revised PIS.
The methods used in this QRS were developed in a number of other RCTs. 140,181,182 Several issues identified as important barriers to recruitment in other trials were also found in OPTIMA prelim, such as recruiters’ discomfort in exploring patient preferences, and the use of problematic terminology in recruitment consultations and trial documentation. 182,183 However, there were also some original barriers to recruitment that were specific to the OPTIMA prelim trial, including recruiters’ discomfort in eligibility criteria and patients’ difficulties understanding the trial design.
The QRS was facilitated by a supportive CfI and TMG, but was hampered by clinical centres being given the opportunity to opt out of the QRS, and by recruiters’ unwillingness to audio-record consultations. These issues have also been found previously. 184 If OPTIMA prelim proceeds to a main trial, clearer support for the QRS as a fully integrated and study-wide recruitment support initiative should be considered and attention given to encouraging (or perhaps expecting) greater commitment to recording consultations. In particular, it was noted that most of the centres participating in the QRS in the current study were mid-range recruiters. Future work would benefit from targeting centres for participation in the QRS on the basis of purposeful sampling methods, where screening logs are used to select centres deemed as ‘high’ and ‘low’ recruiters. This approach depends on clear, complete screening logs that record the entire recruitment process, from initial screening to trial participation. A more detailed discussion of the limitations of the QRS methods, including ideas for optimising future integration of the QRS in the OPTIMA main study, is presented in the Chapter 6, Qualitative recruitment study and Appendix 10.
Chapter 5 Results: pathology and health economic study
Pathology analysis
The objective of the OPTIMA prelim pathology analysis, in conjunction with the health economic analyses, was to identify which test(s) should be taken forward into the main OPTIMA trial. This chapter addresses this question by first discussing the data from several different multiparameter tests conducted on the same samples and then looking at how those data and the predicted patient outcomes identify test(s) that would be strong candidates for further research within the main OPTIMA trial.
Of the 313 patients randomised between 10 October 2012 and 3 June 2014, 302 tumour samples were available for analysis at the central laboratory; four patients had withdrawn prior to Oncotype DX testing, one patient was ineligible and a further six patients had insufficient sample for additional multiparameter testing, although an Oncotype DX score was obtained. These 302 patients are the focus of this section of the report.
Characteristics of patients included in the pathology analysis by Oncotype DX recurrence score groups
Of the 302 patients, 247 (82%) had a RS of ≤ 25, 54 (18%) had a RS of > 25 and one patient had insufficient tumour available for Oncotype DX testing, although the sample was suitable for alternative testing. The characteristics of the 301 patients split by high and low RS are given in Table 24. Histological grade (1 or 2 vs. 3) was the only characteristic that was significantly associated with a RS of ≤ 25 or > 25 (odds ratio 12.3, 95% CI 6.3 to 24.4; p < 0.0001).
Characteristic | RS ≤ 25, n (%) | RS > 25, n (%) | Total, n (%) | |||
---|---|---|---|---|---|---|
Number of patients | 247 (82) | 54 (18) | 301 | |||
Oncotype DX RSa | ||||||
< 18 | 163 (66) | 0 (0) | 163 (54) | |||
18–25 | 84 (34) | 0 (0) | 84 (28) | |||
26–31 | 0 (0) | 20 (37) | 20 (7) | |||
> 31 | 0 (0) | 34 (63) | 34 (11) | |||
Age in years, median (range) | 58 (40–78) | 57 (42–76) | 58 (40–78) | |||
Menopausal status of participant | ||||||
Pre-/perimenopausal | 82 (33) | 14 (26) | 96 (32) | |||
Post-menopausal | 165 (67) | 40 (74) | 205 (68) | |||
Number of involved nodes | ||||||
None | 46 (19) | 11 (20) | 57 (19) | |||
1–3 | 158 (64) | 34 (63) | 192 (64) | |||
4–9 | 32 (13) | 9 (17) | 41 (13) | |||
Positive sentinel node biopsy without clearance surgery | 11 (4) | 0 (0) | 11 (4) | |||
Intended chemotherapy regimen | ||||||
Anthracycline – non-taxane | 94 (38) | 22 (41) | 116 (39) | |||
Taxane – non-anthracycline | 30 (12) | 4 (7) | 34 (11) | |||
Anthracycline – taxane | 123 (50) | 28 (52) | 151 (50) | |||
Tumour grade | ||||||
1 | 19 (8) | 0 (0) | 19 (6) | |||
2 | 185 (75) | 15 (28) | 200 (67) | |||
3 | 43 (17) | 39 (72) | 82 (27) | |||
Largest tumour size in mm, median (range) | 26 (2–170) | 31 (8–70) | 28 (2–170) | |||
Lymphovascular invasion reported | ||||||
No | 147 (60) | 22 (41) | 169 (56) | |||
Yes | 90 (36) | 31 (57) | 121 (40) | |||
Not known | 10 (4) | 1 (2) | 11 (4) | |||
Number of active comorbidities, median (range) | 0 (0–4) | 0 (0–7) | 0 (0–7) | |||
ER score – Allred | ||||||
≤ 6 | 6 (2) | 5 (9) | 11 (4) | |||
7–8 | 241 (98) | 49 (91) | 290 (96) |
Comparison of Oncotype DX with ‘conventional’ prognostic risk assessment tools
The actual outcomes for the patients recruited into OPTIMA prelim will not be known for several years and were therefore estimated using a variety of nomograms and models as described above (see Chapter 3, Clinical risk prediction. Table 25 provides the median (range) estimates of overall survival and RFS by RS for the 301 patients with available data, predicted using the nomograms, PREDICT18 (www.predict.nhs.uk), Adjuvant! Online17 (www.adjuvantonline.com) and NPI,19 and also 5-year RFS from a constant benefit model and a variable benefit model.
Risk and treatment groups | RS ≤ 25 | RS > 25 | Total |
---|---|---|---|
Number of patients, n (%) | 247 (82) | 54 (18) | 301 |
NPI | |||
Median (range) | 4.5 (2.8 to 8.2) | 5.4 (3.6 to 6.8) | 4.6 (2.8 to 8.2) |
≤ 3.4, n (%) | 12 (5) | 0 | 12 (4) |
> 3.4 to ≤ 5.4, n (%) | 196 (79) | 29 (54) | 225 (75) |
> 5.4, n (%) | 39 (16) | 25 (46) | 64 (21) |
PREDICT 10-year overall survival, median (range) | |||
ET only | 78.4 (31.4 to 94.6) | 69.4 (25.1 to 86.0) | 77.0 (25.1 to 94.6) |
CT and ET | 83.8 (39.8 to 95.9) | 76.1 (41.6 to 91.2) | 82.6 (39.8 to 95.9) |
Additional benefit of CT | 4.9 (1.2 to 25.8) | 8.0 (2.3 to 22.6) | 5.5 (1.2 to 25.8) |
Adjuvant! Online 10-year overall survival, median (range) | |||
ET only | 69.4 (25.4 to 90.4) | 63.1 (38.8 to 78.6) | 68.6 (25.4 to 90.4) |
CT and ET | 77.3 (31.0 to 93.6) | 73.4 (43.6 to 86.9) | 76.4 (31.0 to 93.6) |
Additional benefit of CT | 6.3 (1.2 to 25.8) | 8.8 (2.1 to 18.3) | 6.8 (1.2 to 25.8) |
Adjuvant! Online 10-year RFS, median (range) | |||
ET only | 61.7 (22.0 to 82.1) | 55.2 (35.4 to 72.9) | 60.5 (22.0 to 82.1) |
CT and ET | 73.1 (29.1 to 89.4) | 70 (41.4 to 80.9) | 72.9 (29.1 to 89.4) |
Additional benefit of CT | 10 (2.7 to 31.0) | 12.5 (3.6 to 33.3) | 10.5 (2.7 to 33.3) |
Constant benefit model for 5-year RFS, median (range) | |||
ET | 78.5 (46.9 to 90.6) | 74.3 (59.5 to 85.4) | 77.8 (46.9 to 90.6) |
CT and ET | 85.8 (61.8 to 93.9) | 82.8 (71.9 to 90.5) | 85.2 (61.8 to 93.9) |
Additional benefit of CT | 7.2 (3.3 to 14.9) | 8.5 (5.1 to 12.4) | 7.5 (3.3 to 14.9) |
Variable benefit model for 5 year RFS, median (range) | |||
ET | 78.5 (46.9 to 90.6) | 74.3 (59.5 to 85.4) | 77.8 (46.9 to 90.6) |
CT and ET | 76.4 (46.2 to 88.9) | 82.2 (66.0 to 89.8) | 77.2 (46.2 to 89.8) |
Additional benefit of CT | –1.9 (–11.0 to 4.0) | 6.2 (2.6 to 21.7) | –1.0 (–11.0 to 21.7) |
As expected, both the median predicted overall survival and median RFS rates were poorer for the Oncotype DX high-score group than for the low-score group for all nomograms, although the ranges overlapped between the two groups (Table 25). The median predicted additional benefit of chemotherapy compared with that predicted from endocrine therapy alone was consistently greater for the Oncotype DX high-score group for all nomograms although, again, the ranges overlapped with the low-score group. The variable benefit model, which assumes that the benefit of chemotherapy varies with Oncotype DX scores shows, as expected, the most difference in the benefit of chemotherapy between the categories.
Risk categorisation by different molecular tests
One of the key end points for OPTIMA prelim is the selection of one (or more) multiparameter assay(s) to be used as the primary discriminator for chemotherapy allocation in the main study. Tumour specimens from the 302 patients were therefore analysed using additional tests to help inform this decision. Two groups of multiparameter assays were used. The first group of tests discussed in the section, Oncotype DX, MammaPrint, Prosigna ‘risk of recurrence’, which is weighed for proliferation score and tumour size (ROR_PT; NanoString Inc.) IHC4 and IHC4 AQUA, measure aspects of tumour biology and, based on previous clinical data sets, provide numerical scores for individual patients that reflect recurrence risk. The tests also provide predefined risk categories that divide the population into three to four groups based on the numerical risk score that is part of the test output; MammaPrint provides only a binary risk category but no numerical score. The Prosigna assay alone among the tests incorporates some tumour stage information, namely tumour size (but not nodal status). For the purposes of this analysis, the Oncotype DX scores were divided into two groups (rather than the manufacturer’s three predefined risk groups) with the same RS cut-off point of ≤ 25 versus > 25 used for chemotherapy allocation in the OPTIMA prelim. It must be emphasised that predefined risk categories provided by the tests are specific to that test. Although some have been defined using similar boundaries, the tests have been developed in different patient populations and therefore the actual definitions of the risk category boundaries may not translate between assays.
The second group of assays, BluePrint, Prosigna and MammaTyper, divide tumours into the intrinsic subtypes (see Parket et al. 28 and references therein25–27). MammaTyper further divides luminal B cancers into ‘low’ and ‘high’ risk based on proliferation. The subtype of breast cancer identified by these tests reflects the tumour biology, which in turn influences recurrence risk. These tests do not directly ‘measure’ risk but classify tumours into subtypes, which are known to have different drug sensitivities and natural histories. There is extensive literature that shows that luminal A breast cancer is associated with a significantly better prognosis than the other three intrinsic subtypes. Tumours have, therefore, mostly been divided into luminal A and non-luminal A subtype in this analysis. In practice, the great majority of non-luminal A tumours are categorised as luminal B. Again it must be emphasised that the precise definition of subtype is specific to the relevant assay. MammaTyper classified far fewer OPTIMA prelim patients as luminal A than Prosigna and BluePrint; therefore, on the grounds of clinical applicability (plausibility) we chose to combine the MammaTyper luminal A and low-risk luminal B groups [referred to hereafter as MammaTyper Int. (intermediate)].
The proportion of low-risk tumours predicted by Oncotype DX was higher (82%, 95% CI 78% to 86%) than the proportion predicted as either low or intermediate risk using Prosigna ROR_PT (66%, 95% CI 61% to 71%) or IHC4 (72%, 95% CI 67% to 77%). MammaPrint and IHC4 AQUA identified the fewest low-risk tumours, 61% (95% CI 55% to 67%) and 62% (95% CI 56% to 68%), respectively (Table 26). Prosigna ROR_PT identified 36% (95% CI 31% to 41%) as just low risk, compared with 24% (95% CI 19% to 29%) for IHC4 (see Table 26).
Risk group | Oncotype DX RS ≤ 25 vs. > 25 | MammaPrint | Prosigna ROR_PT | IHC4 | IHC4 AQUA |
---|---|---|---|---|---|
n | 301 (99.7%) | 298 (99%) | 299 (99%) | 257 (85%) | 271 (90%) |
Low risk | 247 (82%) | 183 (61%) | 108 (36%) | 62 (24%) | 87 (32%) |
Intermediate (low–mid) risk | – | – | 88 (30%) | 123 (48%) | 80 (30%) |
Mid risk | – | – | – | – | 55 (20%) |
High risk | 54 (18%) | 115 (39%) | 103 (34%) | 72 (28%) | 49 (18%) |
A comparison of kappa statistics between tests with risk categorisation (i.e. Oncotype DX, MammaPrint, ROR_PT, IHC4 and IHC4 AQUA) showed the highest agreement between IHC4 and IHC4 AQUA grouping low/intermediate (κ =0.60; 95% CI 0.50 to 0.70), but this is only slightly higher than the agreement between the classifications using MammaPrint and ROR_PT (low/intermediate, κ = 0.53; 95% CI 0.43 to 0.63) and Oncotype DX and IHC4 (low/intermediate, κ = 0.53; 95% CI 0.41 to 0.65) (Table 27).
Kappa statistic (95% CI) | MammaPrint (low) | ROR_PT (low/intermediate) | IHC4 (low/intermediate) | IHC4 AQUA (low/low–mid) |
---|---|---|---|---|
Oncotype DX (RS of ≤ 25) | 0.40 (0.30 to 0.49) | 0.44 (0.33 to 0.54) | 0.53 (0.41 to 0.65) | 0.40 (0.30 to 0.51) |
MammaPrint | – | 0.53 (0.43 to 0.63) | 0.33 (0.21 to 0.44) | 0.42 (0.30 to 0.53) |
ROR_PT (low/intermediate) | – | – | 0.39 (0.27 to 0.50) | 0.43 (0.31 to 0.54) |
IHC4 (low/intermediate) | – | – | – | 0.60 (0.50 to 0.70) |
What is clear from the data is that discordance between different tests in assigning individual tumours to risk categories is common. For the five tests [Oncotype DX, MammaPrint, Prosigna ROR_PT (low/intermediate), IHC4 (low/intermediate) and IHC4 AQUA (low/low–mid)], only 93 (31%) tumours were classified as low/intermediate risk by all five tests and only 26 (8%) tumours high risk by all five tests. Discordant results between the tests were seen in 183 (61%) tumours although there was agreement between four tests for 94 (31%) tumours. The rates of agreement with other tests were similar for all tests (Table 28). This is consistent with what is known about both (1) the similar prognostic information provided by different tests and (2) differences in which each test measures residual risk (see Chapter 6).
Number of other tests agreed with test | Oncotype DX | ROR_PT | MammaPrint | IHC4 | IHC4 AQUA |
---|---|---|---|---|---|
4 | 119 (39%) | 119 (39%) | 119 (39%) | 119 (39%) | 119 (39%) |
3 | 84 (28%) | 77 (26%) | 73 (24%) | 67 (22%) | 75 (25%) |
2 | 54 (18%) | 52 (17%) | 47 (16%) | 36 (12%) | 33 (11%) |
1 | 31 (10%) | 33 (11%) | 34 (11%) | 25 (8%) | 27 (9%) |
0 | 13 (4%) | 18 (6%) | 25 (9%) | 10 (4%) | 17 (6%) |
Missing | 1 (1%) | 3 (1%) | 4 (1%) | 45 (15%) | 31 (10%) |
Intrinsic subtypes
While the above tests simply measure biological parameters in order to predict outcomes, the growing recognition that breast cancers can be categorised into discrete biological subgroups (or subtypes) has led to the development of multiparameter prognostication based on these subtypes. Table 29 shows the division of tumours into luminal A or non-luminal A for those multiparameter assays that provide intrinsic subtype information. For illustration, the results for both MammaTyper luminal A and MammaTyper Int., which extends the luminal A group to a combination luminal A and low-risk luminal B, are included. All further analysis involving MammaTyper were performed using MammaTyper Int.
Subtype group | BluePrint (n = 298) | Prosigna (n = 299) | MammaTyper Int.a (n = 298) | MammaTyper (n = 298) |
---|---|---|---|---|
Luminal A | 181 (61%) | 178 (60%) | 186 (62%) | 53 (18%) |
Non-luminal A | 117 (39%) | 121 (40%) | 112 (38%) | 245 (82%) |
Interestingly, despite all the patients in this study being screened by central testing as ER-positive, HER2-negative, a small proportion of tumours (15 in total) were classified by molecular subtyping as either ‘HER2 enriched’ or ‘basal-like’ by different testing methods. This phenomenon is well recognised,28 although its significance is unclear. Two tumours were classified as basal-like tumour by Prosigna; one of these was also identified as basal by BluePrint and as triple negative by MammaTyper. The six tumours classified as HER2 enriched by Prosigna did not include the seven categorised as HER2 type by either MammaTyper only (n = 4), BluePrint only (n = 2) or both (n = 1). The subtype tests (BluePrint, Prosigna and MammaTyper Int.) identified approximately 60% of tumours as luminal A [BluePrint: 61% (95% CI 55% to 67%), Prosigna 60% (95% CI 54% to 66%) and MammaTyper Int. 62% (95% CI 56% to 68%)].
Despite the close similarity in the proportions of tumours identified as luminal A, only 121 (40%) tumours were classified as luminal A subtype by all three tests and 58 tumours (19%) as all other subtypes. Discordant results across these three tests for subtype classification were seen in 123 (41%) tumours.
Given the data outlined above on subtyping across different test platforms, it is unsurprising that, as predicted, moderate kappa statistics between different tests were observed (Table 30). In a comparison between subtype assignment by BluePrint, Prosigna and MammaTyper Int., kappa statistics ranged from 0.39 (95% CI 0.29 to 0.50) for the comparison of BluePrint and MammaTyper Int. to 0.55 (95% CI 0.45 to 0.64) for the comparison of BluePrint and Prosigna.
Kappa statistic (95% CI) | Prosigna subtype | MammaTyper Int.a |
---|---|---|
BluePrint subtype | 0.55 (0.45 to 0.64) | 0.39 (0.29 to 0.50) |
Prosigna subtype | – | 0.44 (0.34 to 0.54) |
Assessing the relationship between the continuous Prosigna subtyping and Prosigna risk of recurrence risk score
Prosigna is unique among the multiparameter assays evaluated in providing both a subtype (luminal A, luminal B, HER2 enriched and basal-like) and a continuous risk score (ROR_PT) with predefined risk categories. It is interesting to compare the information provided by these two measures that are derived from measurement of the expression of an identical set of genes.
All 178 tumours classified as luminal A by Prosigna had a ROR_PT score less than the predefined high-risk cut-off point (see Table 31); 108 (61%) of these lay in the low-risk group and the remainder, 70 (39%), were in the intermediate-risk group. None of the 113 luminal B tumours was classified as low risk; 97 (86%) of these lay in the high-risk group (ROR_PT ≥ 61) (see Table 31), with the remainder in the intermediate-risk group. Interestingly, eight tumours, all of which were centrally confirmed as ER-positive/HER2-negative, were grouped into either the basal-like (n = 2) or HER2-like (n = 6) subtypes by Prosigna, and were intermediate or high risk, respectively, according to ROR_PT score (Table 31).
Test result | Prosigna subtypes | |||
---|---|---|---|---|
Luminal A, n (%) | Luminal B, n (%) | Basal-like, n (%) | HER2 enriched, n (%) | |
Number of patients | 178 (59) | 113 (38) | 2 (1) | 6 (2) |
ROR_PT median (interquartile range) | 37 (28–44) | 70 (63–78) | 53 (47–58) | 76 (72–78) |
Range | 5–59 | 43–96 | 47–58 | 64–84 |
ROR_PT | ||||
Low risk (0–40) | 108 (61) | 0 | 0 | 0 |
Intermediate risk (41–60) | 70 (39) | 16 (14) | 2 (100) | 0 |
High risk (61–100) | 0 | 97 (86) | 0 | 6 (100) |
Assessment of patients’ tests results according to Oncotype DX recurrence score group
Tumours were classified as low score or high score according to Oncotype DX for the purposes of treatment allocation. Table 32 shows how tumours were categorised by the other multiparameter assays according to this division. Of the 247 tumours with an Oncotype DX RS of ≤ 25, 177 (72%) were classified as low risk by MammaPrint, 175 (71%) were classified as luminal A by BluePrint subtype 172 (70%) tumours were categorised as luminal A by Prosigna and 168 (68%) were classified as luminal A by MammaTyper Int. (see Table 32). Conversely, of the 54 tumours with a high-risk RS, 44 (88%) were classified both as high risk by MammaPrint and as non-luminal A BluePrint subtype and 36 (66%) were classified as high-risk luminal B by MammaTyper Int.
Test result | Oncotype DX RS ≤ 25, n (%) | Oncotype DX RS > 25, n (%) | Total, n (%) |
---|---|---|---|
Number of patients tested by Oncotype DX | 247 (82) | 54 (18) | 30 (100) |
Number of patients tested by MammaPrint/BluePrint | 247 (83) | 50 (17) | 297 (100) |
MammaPrint | |||
Low risk | 177 (72) | 6 (12) | 183 (62) |
High risk | 70 (28) | 44 (88) | 114 (38) |
BluePrint subtype | |||
Luminal A | 175 (71) | 6 (12) | 181 (60) |
Luminal B | 70 (28) | 43 (86) | 113 (38) |
Basal | 0 (0) | 1 (2) | 1 (1) |
HER2 type | 2 (1) | 0 (0) | 2 (1) |
Number of patients tested by Prosigna | 246 (83) | 52 (17) | 298 (100) |
Prosigna subtype | |||
Luminal A | 172 (70) | 5 (10) | 178 (59) |
Luminal B | 74 (30) | 39 (75) | 113 (38) |
Basal-like | 0 (0) | 2 (4) | 2 (1) |
HER2 enriched | 0 (0) | 6 (11) | 6 (2) |
ROR_PT, median (range) | 43 (5 to 91) | 77 (32 to 96) | 47 (5 to 96) |
ROR_PT | |||
Low risk | 106 (43) | 2 (4) | 108 (36) |
Intermediate | 81 (33) | 6 (11) | 87 (29) |
High risk | 59 (24) | 44 (85) | 103 (35) |
Number tested by MammaTyper | 243 (82) | 54 (18) | 298 (100) |
MammaTyper Int. subtypea | |||
Luminal A | 168 (69) | 18 (33) | 180 (62) |
Luminal B | 72 (30) | 34 (63) | 106 (36) |
HER2 positive | 3 (1) | 1 (2) | 4 (1) |
Triple-negative breast cancer | 0 (0) | 1 (2) | 1 (1) |
Number tested by IHC4 | 207 (80) | 50 (20) | 257 (100) |
Median IHC4 score (range) | –2.6 (–90.0 to 110.0) | 54.0 (–37.8 to 143.7) | 7.5 (–90.0 to 143.7) |
IHC4 | |||
Low risk | 61 (29) | 1 (2) | 62 (24) |
Intermediate risk | 113 (55) | 10 (20) | 123 (48) |
High risk | 33 (16) | 39 (78) | 72 (28) |
Number tested by IHC4 AQUA | 223 (82) | 47 (18) | 270 (100) |
Median IHC4 AQUA score (range) | –23.3 (–141.9 to 121.6) | 28.7 (–40.3 to 133.7) | –16.6 (–141.9 to 133.7) |
IHC4 AQUA | |||
Low | 86 (38) | 1 (2) | 87 (32) |
Low–mid | 75 (34) | 5 (11) | 80 (30) |
Mid | 42 (19) | 12 (25) | 54 (20) |
High | 20 (9) | 29 (62) | 49 (18) |
Economic analysis
In order to calculate the health economic aspects of the various tests, given that we do not know the actual outcomes of the enrolled patients, these have to be estimated. As discussed in Chapter 3, Clinical risk prediction, there are different views on whether or not the benefit of the chemotherapy is dependent on the test score, and this section will present the health economics analysis using different assumptions within alternative sensitivity analyses.
The tests meeting eligibility for inclusion in the base-case analysis were Oncotype DX, MammaPrint, Prosigna Subtype and Prosigna ROR_PT. The base-case analysis excluded IHC4 and IHC4 AQUA because of concern about their analytical validity in the context of a possible national NHS roll-out as outlined in Chapter 1, Individual multiparameter assays. Although MammaTyper has demonstrated analytical validity, there were no published clinical validation data and no cost information was available. No clinical validation data have been published for IHC4 AQUA, which was also excluded on these grounds.
Validation model: Oncotype DX versus standard care based on the SWOG88-14 trial
The validation model represents an update of the model, which was originally published in 2012, and which compared Oncotype DX with chemotherapy for all. 15 Results of the validation analysis comparing standard care (chemotherapy for all patients or ‘chemo-for-all’) to Oncotype DX are shown in Table 33. Two potential RS cut-off points for Oncotype DX have been considered: ≤ 25 and cut-off score of < 18, hereafter referred to as Oncotype DX_25 and Oncotype DX_18. The results indicate that Oncotype DX increases QALYs regardless of which cut-off point is used; however, Oncotype DX_25 proves more cost-effective than Oncotype DX_18, as Oncotype DX_25 produces additional QALYs at a lower cost per QALY rate than using a cut-off point of 18. That is, Oncotype DX_25 has a lower ICER than Oncotype DX_18 and, therefore, Oncotype DX_25 is preferred to Oncotype DX_18, thus supporting the cut-off point of 25 used in the OPTIMA prelim trial on health economic grounds.
Strategy | Mean cost (£) | Mean QALYs | Incremental cost (£) | Incremental QALYs | ICER (£/QALY) |
---|---|---|---|---|---|
Chemotherapy for all | 13,565 | 7.76 | – | – | – |
Oncotype DX_18 | 15,364 | 8.19 | 1799 | 0.43 | 4184a |
Oncotype DX_25 | 15,061 | 8.45 | –304 | 0.27 | Dominate |
Results of the analysis including only Oncotype DX_25 versus chemotherapy for all are shown in Table 34. Compared with chemotherapy for all, Oncotype DX_25 is associated with a cost per additional QALY of £2166.
Strategy | Mean cost (£) | Mean QALY | Incremental cost (£) | Incremental QALY | ICER (£/QALY) |
---|---|---|---|---|---|
Chemotherapy for all | 13,565 | 7.76 | – | – | – |
Oncotype DX_25 | 15,061 | 8.45 | 1495 | 0.69 | 2166 |
Main economic analysis (base case)
The base-case comparison includes four tests (Oncotype DX, MammaPrint, Prosigna Subtype and Prosigna ROR_PT) and assumes that the relative benefit of chemotherapy is dependent on risk groups and test score. For the analysis we used cut-points dividing tumours into high- or lower-risk groups as follows: Oncotype Dx, RS > 25 vs. ≤ 25; MammaPrint, vendor pre-defined; Prosigna subtype, non-luminal A vs. luminal A; Prosigna ROR_PT, vendor pre-defined score of ≥ 60 vs. < 60. Post-recurrence survival is assumed to be constant regardless of pretreatment with adjuvant chemotherapy and the benefit from chemotherapy is assumed to depend on the test scores. The costs, QALYs and net health benefit of all tests are shown in Table 35 along with the probability of producing more QALYs or costing less than chemotherapy for all.
Treatment strategy | Mean cost (£) | Mean QALYs | Net health benefit (QALYs) | Probability more effective than chemotherapy for all | Probability cost-saving vs. chemotherapy for all |
---|---|---|---|---|---|
Chemotherapy for all | 13,961 | 7.69 | 6.99 | – | – |
Oncotype DXa | 13,853 | 7.89 | 7.20 | 0.73 | 0.53 |
MammaPrinta | 14,156 | 7.87 | 7.16 | 0.73 | 0.39 |
Prosigna subtypea | 13,680 | 7.87 | 7.19 | 0.74 | 0.62 |
Prosigna ROR_PTa | 13,487 | 7.88 | 7.20 | 0.73 | 0.68 |
Considered in isolation, all tests are more cost-effective than standard care alone, since they all lead to an increase in QALYs and all except MammaPrint result in an overall reduction in costs (see Table 35). The net health benefit from all tests is higher than chemotherapy for all, although is fairly similar between tests.
Uncertainty in the cost-effectiveness is represented graphically for each test in comparison with chemotherapy for all in the scatterplot on the incremental cost-effectiveness plane (Figure 8) and the cost-effectiveness acceptability curves in Figure 9.
FIGURE 8.
Scatterplot on the incremental cost-effectiveness plane, comparing each test included in the base-case analysis with chemotherapy for all.
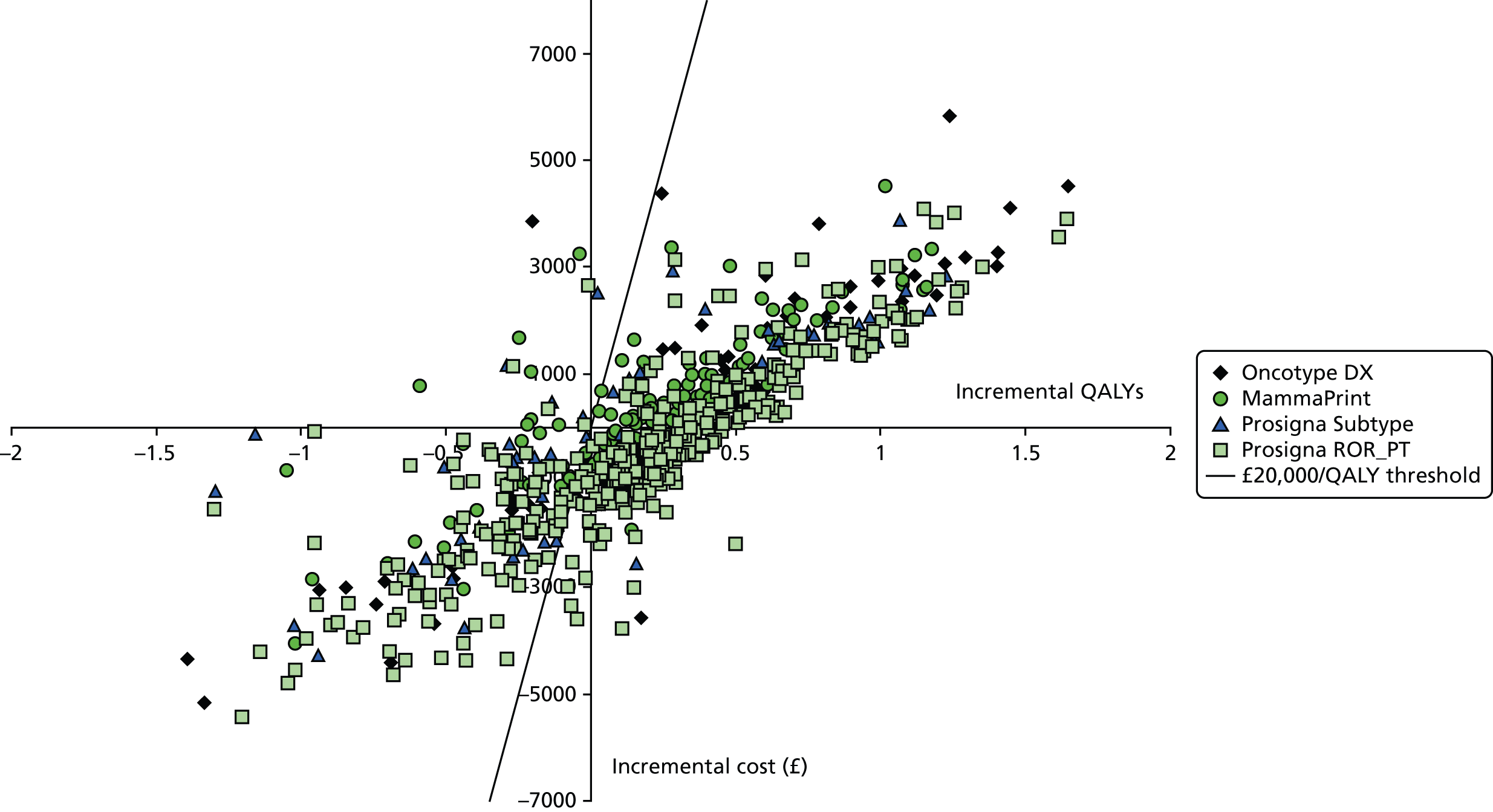
FIGURE 9.
Cost-effectiveness acceptability curves: tests compared with chemotherapy for all.

The results of an incremental analysis, in which tests included in the base case compete with each other, rather than just competing with chemotherapy for all, is presented in the cost-effectiveness acceptability curves in Figure 10. At a willingness-to-pay threshold of £20,000 per QALY Prosigna ROR_PT has the highest probability of being cost-effective at 39%.
FIGURE 10.
Cost-effectiveness acceptability curve for the base-case analysis: tests compared with each other.
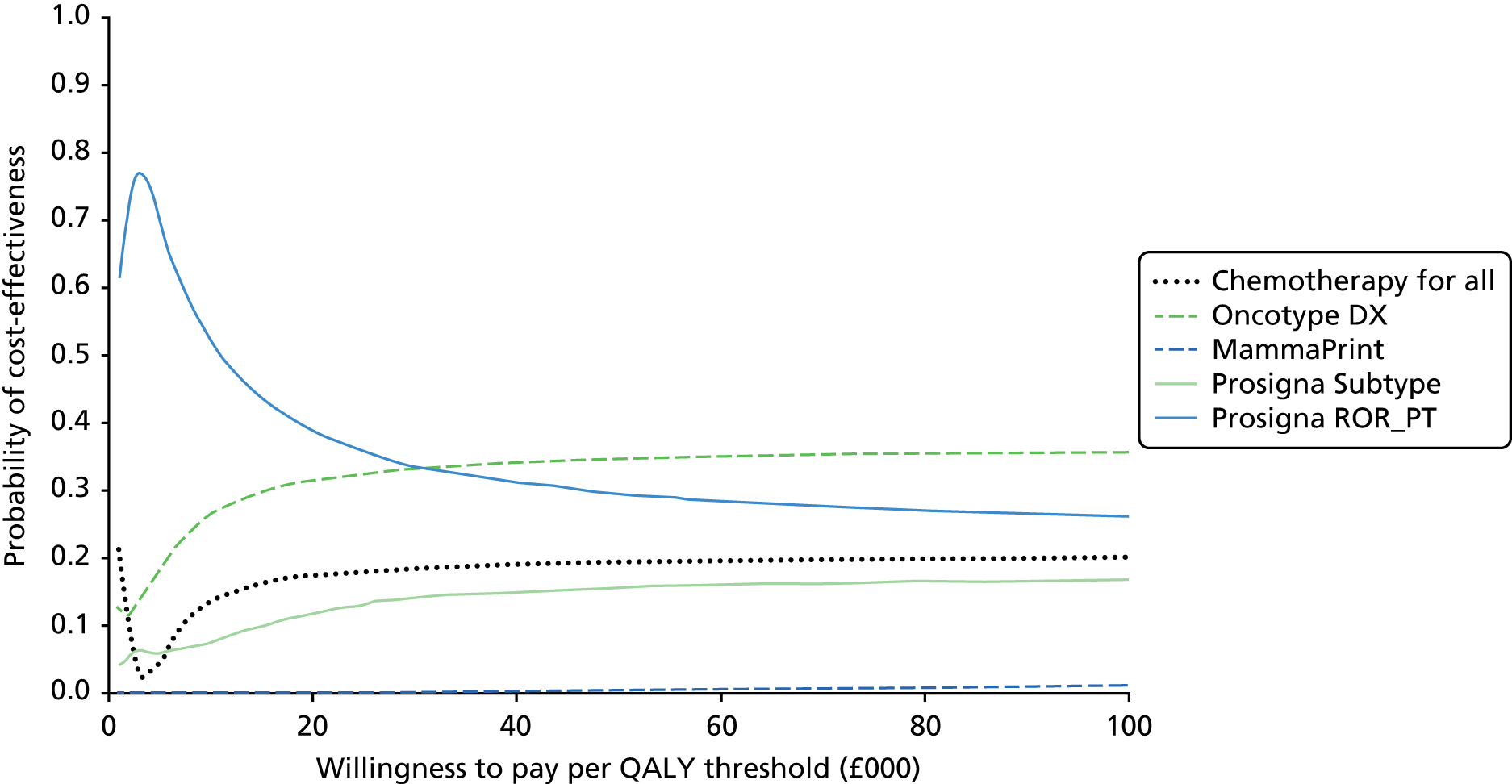
Sensitivity analysis number 1
In sensitivity analysis number 1, it is assumed that post-recurrence survival is dependent on previous treatment with adjuvant chemotherapy. Results of sensitivity analysis number 1, including only tests included within the base-case analysis, are shown in Table 36 and in the accompanying cost-effectiveness acceptability curve (Figure 11). It should be noted that this analysis favours Oncotype DX over the other tests on the basis of expected cost-effectiveness, with Prosigna ROR_PT falling into second place in terms of both expected net health benefit and the probability of cost-effectiveness.
Treatment strategy | Mean cost (£) | Mean QALYs | Net health benefit (QALYs) |
---|---|---|---|
Chemotherapy for all | 13,079 | 7.47 | 6.81 |
Oncotype DX | 15,320 | 8.28 | 7.52 |
MammaPrint | 15,008 | 8.10 | 7.35 |
Prosigna Subtype | 14,437 | 8.07 | 7.35 |
Prosigna ROR_PT | 14,437 | 8.13 | 7.41 |
FIGURE 11.
Cost-effectiveness acceptability curve for sensitivity analysis number 1.

Sensitivity analysis number 2
In sensitivity analysis number 2, it is assumed that the effect of treatment (i.e. hazard ratio for RFS with chemotherapy compared with no chemotherapy) is constant across test-defined risk groups. It is also assumed that post-recurrence survival is constant across all patients, regardless of pretreatment, as in the base-case analysis. The mean costs, QALYs and net health benefit are presented in Table 37. Chemotherapy for all is more cost-effective than any of the testing options, although there is some uncertainty about this as demonstrated in the cost-effectiveness acceptability curve (Figure 12).
Treatment strategy | Mean cost (£) | Mean QALYs | Net health benefit (QALYs) | Probability more effective than chemotherapy for all | Probability cost-saving vs. chemotherapy for all |
---|---|---|---|---|---|
Chemotherapy for all | 13,917 | 7.99 | 7.30 | – | – |
Oncotype DX | 13,826 | 7.89 | 7.21 | 0.34 | 0.58 |
MammaPrint | 14,139 | 7.92 | 7.22 | 0.34 | 0.41 |
Prosigna Subtype | 13,665 | 7.93 | 7.25 | 0.35 | 0.66 |
Prosigna ROR_PT | 13,472 | 7.92 | 7.25 | 0.34 | 0.71 |
FIGURE 12.
Cost-effectiveness acceptability curve for sensitivity analysis number 2.

Value-of-information analysis for the base-case analysis and sensitivity analyses numbers 1 and 2
The expected value of perfect parameter information is calculated for the 5-year RFS parameters and other parameters that would be informed by the proposed OPTIMA trial (including benefit from chemotherapy and the proportion allocated to high risk). This represents a ceiling on the value of research into these parameters. Each EVPPI calculation is the EVPPI for a comparison between chemotherapy for all and chemotherapy directed by a single alternative test. Tests with higher EVPPI are, therefore, a higher priority for inclusion in further randomised research which has 5-year RFS as an outcome. The EVPPI is presented in Figure 13 and is high for all tests included in the base-case analysis, suggesting high value in further research into test-directed chemotherapy. The expected net benefit, probability of cost-effectiveness and the EVPPI all favour Prosigna (ROR_PT) as the preferred test for both current adoption and inclusion in further research. The EVPPI for MammaPrint is higher than for Oncotype DX, suggesting that this may be the second priority, but that it should not be prioritised over Prosigna. The ranking of tests is similar in sensitivity analysis number 1. For sensitivity analysis number 2 (constant benefit from chemotherapy, irrespective of test score) the EVPPI is much lower for all tests except MammaPrint (see Figure 13).
FIGURE 13.
Value-of-information analysis for the base-case comparison and sensitivity analyses numbers 1 and 2. SA, sensitivity analysis.
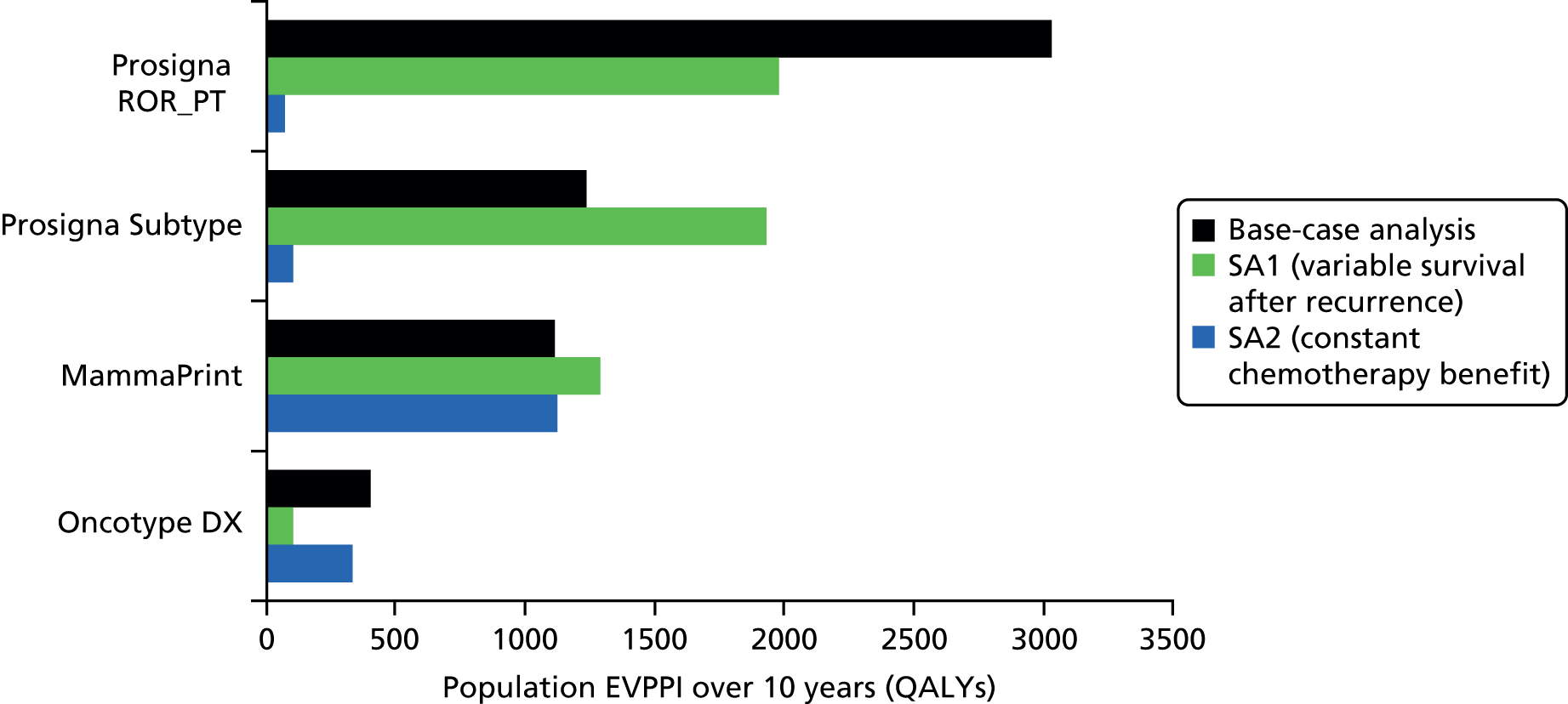
Sensitivity analysis number 3
Sensitivity analysis number 3 includes all tests, irrespective of eligibility for inclusion in the base-case analysis. The costs, QALYs and net health benefit are shown in Table 38. The probabilistic results are shown in Figure 14. The results of the probabilistic analysis are presented as a cost-effectiveness acceptability curve compared with chemotherapy for all in Figure 15 and with each other in Figure 16. It can be seen that all three additional tests have a net health benefit higher than that of the tests included in the base-case analysis, suggesting that they would be more cost-effective if adopted at the present time. The results of a value-of-information analysis, which includes all tests as comparators, presented in Figure 17, confirms the base-case conclusion that Prosigna ROR_PT remains the priority for further research, although it is now closely followed by IHC4.
Treatment strategy | Mean cost (£) | Mean QALYs | Net health benefit (QALYs) | Probability more effective than chemotherapy for all | Probability cost-saving vs. chemotherapy for all |
---|---|---|---|---|---|
Chemotherapy for all | 13,961 | 7.69 | 6.99 | – | – |
Oncotype DXa | 13,853 | 7.89 | 7.20 | 0.73 | 0.53 |
MammaPrinta | 14,156 | 7.87 | 7.16 | 0.73 | 0.39 |
Prosigna subtypea | 13,680 | 7.87 | 7.19 | 0.74 | 0.62 |
Prosigna ROR_PTa | 13,487 | 7.88 | 7.20 | 0.73 | 0.68 |
MammaTyper | 13,017 | 7.88 | 7.22 | 0.73 | 0.80 |
IHC4 | 12,846 | 7.87 | 7.22 | 0.73 | 0.90 |
IHC4 AQUA | 12,069 | 7.87 | 7.27 | 0.73 | 0.84 |
FIGURE 14.
Scatterplot on the incremental cost-effectiveness plane, comparing each test with chemotherapy for all.

FIGURE 15.
Cost-effectiveness acceptability curves for sensitivity analysis number 3: all tests included and compared with chemotherapy for all.

FIGURE 16.
Cost-effectiveness acceptability curves for sensitivity analysis number 3: all tests included and compared with each other.

FIGURE 17.
Sensitivity analysis number 3: value-of-information analysis, all tests included.

Relationship between Adjuvant! Online survival prediction and test scores
The relationship of the Oncotype DX scores and ROR_PT scores with the Adjuvant! Online 10-year overall survival for endocrine therapy is displayed in Figures 18 and 19. These data are presented because of the reliance on outcomes forecast by Adjuvant! Online in the cost-effectiveness model. There is a slightly higher negative correlation between the Adjuvant! Online 10-year overall survival for endocrine therapy and ROR_PT scores (r = –0.39) compared with Oncotype DX RS (r = –0.19), which may be contributing to the higher QALYs in the Prosigna analysis.
FIGURE 18.
Scatterplot of the Adjuvant! Online 10-year overall survival for endocrine therapy only against risk scores: Oncotype DX.

FIGURE 19.
Scatterplot of the Adjuvant! Online 10-year overall survival for endocrine therapy only against risk scores: ROR_PT.

Chapter 6 Discussion
Recruitment and study conduct
Recruitment and study design
OPTIMA prelim opened to recruitment in late September 2012. The first patient consented to join the study in October 2012. By 3 June 2014, 350 patients had been registered, of whom 313 had been randomised from 35 recruiting centres. The OPTIMA TMG, and the NIHR HTA programme as study funder, always considered that patient recruitment might prove to be difficult. Previous experience of clinical trials involving an experimental arm in which less treatment is given than is standard clinical practice, such as the ProTect trial,140 have experienced difficulties in recruitment. 185,186 Prespecified success targets were designed to demonstrate that recruitment into the proposed main trial was feasible once the number of recruiting centres was scaled up. Most of these applied to the final 6 months of recruitment to allow time to open the requisite number of sites and for research staff to learn how to recruit potential participants. Site selection for the OPTIMA prelim was on the basis of individual invitation rather than an open call. Five main geographical clusters were established, namely North London, East Anglia, the South West, the West Midlands and Scotland. Sites within each cluster included a mixture of cancer centres and district general hospitals. This policy was designed to ensure that feasibility could be demonstrated in a representative selection of UK centres rather than in those that are best described as enthusiasts.
All predefined feasibility conditions have been met, demonstrating that a large-scale effectiveness trial of the use of multiparameter assays as a decision tool for patients with primary ER-positive HER2-negative breast cancer in the UK is indeed achievable. This is despite approximately 80% of patients having lymph node involvement, which places them at comparatively high recurrence risk.
Two of the three other international trials of test-directed chemotherapy decisions have met their recruitment targets. 121,124 The MINDACT study estimated patient risk using information from a clinical risk prediction nomogram, Adjuvant! Online, and a biological test, MammaPrint. 122,186 Patients with discordant risk assessments were randomised to a decision based on one of the two. Eligible patients could have up to three involved axillary lymph nodes but there was no restriction on receptor status. Patients with high-risk disease, determined on the basis of test concordance or by randomisation, were treated with chemotherapy and offered an optional further randomisation between one of two chemotherapy regimens. Similarly, those who had ER-positive disease, whether or not they received chemotherapy, were eligible for optional randomisation between two endocrine treatment regimens. A formal feasibility analysis was published after 800 patients (including a small number from the UK) had been registered, at which time 25% of registered patients had discordant test results. 187 The MINDACT design is very complex, which inevitably created difficulties in explaining the study to patients. There is no published information on the acceptability of the study to patients, although the use of a risk score provided by Adjuvant! Online is likely to have been reassuring. The study recruited approximately 6600 patients. The first analysis is expected to be published in 2016.
The TAILORx trial was conducted in the USA. Eligible patients had ER-positive, HER2-negative tumours no larger than 5 cm in diameter and without lymph node involvement. Registered patients underwent Oncotype DX testing. 121,124 Those with a RS in the range 11–25 were eligible for randomisation between chemotherapy followed by endocrine therapy versus endocrine therapy alone. Non-randomised patients with low or high scores were followed up in registry arms. The study, which has a non-inferiority end point, aimed to enrol 11,248 patients and to randomise approximately 4500; no information is available on the number of eligible patients who accepted randomisation. Most of the patients randomised in the study would be considered at too low a risk to be offered chemotherapy in routine UK clinical practice. The primary analysis is planned to take place in December 2017.
The RxPONDER study is similar in design to TAILORx but includes patients with one to three involved lymph nodes; those with an Oncotype DX RS of ≤ 25 are eligible for randomisation. 123,188 In potential study participants, tumour testing may be performed either through trial registration or independently. An estimated 9400 patients will need to be screened to achieve the randomisation target of 4000. Cost-effectiveness research is an integral component of RxPONDER, which is largely funded by the USA private health insurers. The primary analysis of the study, which began recruitment in 2011, is expected in 2022. No information has been published on the acceptability of the study to patients.
The OPTIMA study has significant design differences to these three studies. Patients with up to nine involved axillary nodes are eligible for randomisation, which by virtue of tumour stage is a higher-risk group than the other studies have allowed. The study is partially blinded to minimise the likelihood of bias in clinician behaviour and to reduce the risk of non-acceptance of treatment allocation; thus, participants allocated to chemotherapy are not informed if they have been randomised to the control arm or have a high-score Oncotype DX test result. Although patients allocated to no chemotherapy are aware that they have a low-score Oncotype DX result, the actual RS is not disclosed to the site or patient. Patients who are premenopausal at diagnosis are routinely treated with ovarian suppression regardless of whether or not they receive chemotherapy, as otherwise a chemotherapy-induced menopause, which is difficult to identify reliably, would be a potential source of inequality between the treatment arms. All of these features have been identified in screening logs as reasons for potential participants choosing not to join the study. Despite this, overall patient acceptance identified from the screening log was 47%, which exceeds the protocol-defined target of 40%. Of those patients who went on to be randomised, 20% of patients had node-negative disease while 15% had four or more involved nodes. A total of 31% of participants were reported to be pre- or perimenopausal at the time of randomisation. Thus, the OPTIMA prelim has demonstrated that it is possible to conduct a study with design features that will maximise the chances that the study will deliver an unbiased result despite their difficulties for potential participants.
The reasons for the choice of an Oncotype DX RS cut-off point of ≤ 25 vs. > 25 for chemotherapy have been explained in Chapter 2, Methods. To put this into context, a RS of 25 equates to a 16% risk of developing metastatic disease over 10 years in a node-negative breast cancer population treated with tamoxifen for 5 years but not chemotherapy,41 often termed residual risk. Any estimation of chemotherapy benefit at this level of risk, however, must take into account that approximately one-third of this risk relates to chemotherapy-insensitive late (beyond 5 years) relapse. 9
Clinical decisions on chemotherapy use are influenced by residual risk and the predicted likelihood of chemotherapy benefit, meaning the prevention of distant recurrence and death, which is related to residual risk. There is no universally accepted level of predicted benefit that informs practice. Perception of clinical risk and what constitutes a meaningful benefit from medical treatment is very much a matter of individual judgement for both patients and clinicians. In the UK, most oncologists will discuss breast cancer chemotherapy with patients who have a 3–5% predicted chance of benefit and recommend its use for those with > 5% chance of benefit. At this level, although there is a significant population-wide treatment benefit, the probability that an individual patient will benefit is modest.
Risk of recurrence is influenced by clinical stage as well as by tumour biology, and adding stage information to multiparameter assay output improves the prediction of residual risk (e.g. see Tang et al. 64,70). Should the hypothesis underlying the OPTIMA study prove correct, however, then patients with tumours with a low multiparameter risk score are unlikely to benefit to a meaningful degree from chemotherapy despite a potentially high risk resulting from adverse stage, particularly lymph node involvement. This is the conclusion from the retrospective analysis of the NSABP B-20 trial. 64,70 It is difficult to make a direct estimate of the likely level of chemotherapy benefit in the largely node-positive OPTIMA population at the RS threshold of 25. Estimates based on NSABP B-2061 and SWOG88-1462 studies performed in node-negative and node-positive populations, respectively, suggest a reduction in 10-year breast cancer mortality risk of in the order 5%. If a RS of 25 is indeed close to the score at which the Oncotype DX predicts significant chemotherapy sensitivity, then nodal status and other stage information are unlikely to have much influence on this estimate. In contrast, for patients at risk by virtue of adverse stage and whose tumours have higher scores predicting chemotherapy sensitivity in addition to risk, chemotherapy is likely to offer a very substantial benefit. Similar considerations should apply to other multiparameter assays.
Patient focus groups
Three patient focus groups were held to assess the acceptability and decision-making for women who may have been offered randomisation into the OPTIMA prelim. These groups also reviewed the patient information, leaflets and consent form. The study was acceptable to the majority of participants. Some had clear preference for chemotherapy as that is what they had been offered and some discussed how they felt not being offered chemotherapy. The trial design using a ‘test’ to decide treatment was acceptable and most felt that a personalised approach using these multiparameter tests would be a preferred option. The results of the focus groups provided some triangulation on the QRS for the TMG.
Qualitative recruitment study
Key findings from the qualitative recruitment study
The QRS was designed as an integral part of the OPTIMA prelim and identified a number of key challenges to recruitment. It devised and delivered interventions to address these difficulties in collaboration with the TMG and CfI. The most prominent identified themes that recurred across interviews and audio-recorded consultations related to difficulties in eligibility processes and issues of clinician–patient communication. These challenges had the potential to limit the number of patients approached about the trial, and/or ran the risk of affecting the trial’s acceptability to patients.
In terms of difficulties in eligibility processes, some clinicians showed discomfort with the OPTIMA prelim’s eligibility criteria, which deviated from embedded clinical practice. For instance, some clinicians felt patients with the extensive lymph node involvement or higher tumour grade required chemotherapy, leading to reluctance to enter these individuals into a trial where they may not receive this treatment. Equipoise issues in some cases therefore had implications for whether or not certain high-risk patients were offered the trial at all. Discomfort surrounding eligibility criteria also had potential to influence how clinicians communicated with patients. For example, audio-recordings revealed that the prospect of chemotherapy was sometimes (possibly inadvertently) presented as the preferred choice by clinicians. Recordings of consultations also revealed examples of patients holding treatment expectations based on advice they had received from other clinicians they had encountered in their care pathway. Clinicians’ discretion played a role in determining whether or not patients would be approached about the trial; these judgments were sometimes based on subjective criteria, such as clinician assessments of whether or not patients could cope with additional information. As patient advocates, clinicians clearly felt they were protecting patients’ interests. However, these reported practices implied that patients themselves did not always make decisions about trial participation.
A number of recruitment challenges uncovered by phase 1 of the QRS related to matters of communication, such as the quality and clarity of explanations provided to patients. Recruiters experienced difficulties in explaining the OPTIMA prelim trial design – particularly the unconventional way in which the ‘test-directed treatment’ arm split into two further arms. Audio-recordings of consultations showed occasional use of problematic terminology to describe arms, and challenges in explaining trial-specific processes such as ‘randomisation’ and ‘blinding’. Concepts such as ‘uncertainty’ and ‘risk’ were also communicated in a variety of ways, to different levels of detail. Interviews uncovered recruiter discomfort in exploring patients’ decisions to decline the trial for fear of jeopardising relationships with patients, or being accused of coercive behaviour. While these scenarios should be handled sensitively, recruiters found it easier to avoid exploring decisions about trial participation altogether. However, analysis of audio-recordings provided some evidence to suggest that this avoidance could lead to missed opportunities to address patients’ misconceptions and concerns.
Identification of the above challenges led to a series of QRS interventions, designed and delivered in collaboration with the TMG and CfI. Key interventions included promotion of clinician-to-clinician discussions to address concerns about eligibility criteria; circulation of generic ‘tips and guidance’ sheets to help recruiters explain trial-specific processes within the context of the OPTIMA prelim; organisation of group feedback meetings to share QRS findings and address concerns collaboratively; and delivery of individual feedback, to provide tailored advice and consider solutions to challenges experienced by individual recruiters.
Recruitment into OPTIMA prelim continued to improve as the study progressed. However, it is difficult to assess the impact of the QRS, as claims of causality are problematic considering the multiple variables that could have influenced recruitment at any given time. Nonetheless, we found some qualitative evidence to suggest QRS interventions had an impact on some clinicians’ practices, although these findings were based on a small sample of audio-recorded consultations.
Strengths and limitations
There were a number of limitations to the QRS methods. In terms of sampling, the full range of the OPTIMA prelim centres were not available owing to some centres’ decisions to decline participation in the optional QRS. The QRS consisted of mainly mid-range recruiting centres, not those with the highest and lowest recruitment rates. Inclusion of centres with more extreme recruitment figures would have been beneficial for comparison purposes, and may have contributed to further advice and recommendations to optimise information provision and recruitment. Furthermore, questions should be raised about possible differences between centres that opted for and against QRS participation (e.g. differences in commitment to the trial, differences in resource availability).
Despite encouragement from the QRS team and TMG, recording of consultations did not occur as a matter of routine, and the full range of interactions with patients was not captured. This is particularly true for second oncology consultations (where patients sometimes gave their decisions about participation). Incomplete recordings of each patient’s pathway made it difficult to track events and made deciphering patients’ reasons for declining the trial problematic. In addition, limited numbers of audio-recordings for each individual recruiter restricted opportunities to assess the impact of QRS interventions through ‘before/after’ comparisons. Reluctance to routinely record consultations may have been an indirect consequence of recruiters perceiving the QRS as an optional additional task. The main trial, if funded, will need to frame the QRS as a fully integrated component of the trial.
Although not a limitation of the qualitative methodology, questions remain with regards to the impact of QRS interventions on recruitment rates and recruiters’ practices. We saw some evidence of recruiters changing their practices and can be certain that recruitment improved over time. However, we cannot make claims of causality between QRS interventions and recruitment rates. Further research by the QRS team will consider this in more detail by exploring innovative ways in which this type of work can be evaluated in the context of RCTs.
The above limitations are being addressed in future applications of integrated qualitative work by the QRS team, and will be in the main trial, if funded. However, the practical (rather than theoretical) applications of the QRS to the OPTIMA study need to be considered foremost; it is this applied nature of the QRS that distinguishes it from traditional qualitative studies.
A clear strength of the QRS was its mixed-method, flexible approach to investigation. This enabled the team to understand recruitment difficulties during enrolment in the trial, leading to identification of challenges that were specific to the OPTIMA prelim (e.g. eligibility concerns), as well as difficulties that have been previously reported in most trials (e.g. difficulties explaining trial-specific processes). The opportunity to feedback findings quickly to change participants’ practices was a key strength of this research, highlighting its direct practical applications. This process of sharing findings with participants also allowed for further exploration of ‘challenges’, thus reinforcing the iterative nature of the work undertaken and providing an informal form of participant validation.
The QRS work undertaken was a collaborative process that was designed to build on the support and expertise of the TMG to help design and deliver interventions. This element of the QRS is an important strength, as interventions were refined through input from a truly multidisciplinary team.
Lessons learnt from the qualitative research study: implications for a main trial
In the light of the above challenges and interventions in the OPTIMA prelim, there are a number of key lessons that could be carried forward to the main trial. As new centres are likely to open, issues of equipoise and discomfort surrounding eligibility criteria are likely to present again. The TMG could pre-empt these difficulties by providing time for clinicians to discuss eligibility criteria at site initiation meetings. One option may be to present clinical vignettes to prompt discussion about eligibility concerns – a technique adopted in some of the group feedback meetings in the OPTIMA prelim.
A clear lesson from the QRS is the need for continued effort to use qualitative methods to optimise the quality of information provision and address recruitment challenges in the main study. Experiences of the QRS within OPTIMA prelim will help inform ways in which the QRS can be better integrated to ensure it is used to its maximum potential. First, a more purposeful approach to sampling centres will be used, with a view to including a maximum range of centres that vary by size, geographical location and recruitment rates (based on screening log analysis). As suggested above, this process will work best if the QRS is presented as an integrated component of the main study. Recruiters will be encouraged to record routinely all interactions with patients who have given consent; this may call for the QRS team to offer further support with the recording process (e.g. by making site visits where needed) and for recruiters to prioritise face-to-face (rather than telephone) consultations whenever possible. Even if telephone consultations are a necessity, additional equipment can be provided to ensure these discussions are also captured.
Finally, a key consideration of the QRS within a main trial will be to compare recruiters’ practices before and after feedback. This will help in the development of strategies to evaluate the impact of QRS interventions. Promotion of routine audio-recording of consultations will be a cornerstone of this work.
Pathology and health economics
Characteristics of the OPTIMA prelim population
An important consideration is whether or not the population recruited represents those to whom the research question is relevant. Although the prevalence of high-grade tumours in the OPTIMA-eligible population is unknown, there seems to be an excess of histological grade 2 lesions compared with grade 3 tumours in the OPTIMA prelim; 6%, 67% and 27% of lesions were reported to be grade 1, 2 and 3, respectively. For reference, in an historic and completely unselected series of patients presenting with symptomatic early breast cancer the ratio of grade 1, 2 and 3 lesions was reported to be 2 : 3 : 5. 189 In mixed screening and symptomatic series of ER-positive tumours, lower proportions of high-grade tumours (e.g. 3 : 4 : 3190) have been described. It is likely that the proportion of high-grade tumours in the OPTIMA-eligible population lies between these two proportions. Certainly the 18% of tumours with an Oncotype DX score of ≥ 25 was lower than the predicted 30% in our population. There appears, however, to be little difference from that expected in tumour type distribution, with 70% of cancers of no special type/ductal in large series, compared190 with 71% in the OPTIMA prelim. One possible explanation for the apparently lower than expected recruitment of patients with more aggressive tumours is an element of patient selection by recruiting clinicians. It is important to ensure that the study is not skewed towards patients with very low-biological-risk tumours if it is to be relevant to the entire population. Recruiting clinicians therefore need to be reassured that multiparameter assays reliably classify tumour risk irrespective of histological grade; going forwards to the main trial, this will require education.
Central review of receptor status
We undertook routine central retesting of receptor status in the OPTIMA prelim because of concern about a potential for bias if patients with either ER-negative or HER2-positive disease based on local histopathology testing were included in the study. There are many possible causes for this scenario, including a well-recognised low incidence of HER2 gene amplification among tumours that are considered HER2-negative by immunohistochemistry in first step of the standard two-stage HER2 testing procedure recommended in UK guidelines. 191 Any participants with ER-negative or HER2-positive disease would be disadvantaged if allocated to endocrine therapy only, and such imbalance in treatment allocation would be a potential source of confounding. To minimise this risk, central review to confirm the referring pathology laboratory results for ER positivity and HER2 negativity was undertaken with immunohistochemistry for ER, and with FISH for HER2 status. The former was scored using the Allred system (% staining and average intensity; range 0–8) with a cut-off point of ≥ 3 defined as positive. HER2 positivity with FISH was defined as a ratio of HER2 to chromosome 17 centromeric probe copy numbers of > 2.0 (2.0–2.2 defined as borderline but amplified). This confirmed the eligibility of all but 12 of 325 patients (96.3%) tested on central review of receptor status.
Reassuringly, in the OPTIMA prelim, only 1.2% (n = 4) of patients who locally were thought to have ER-positive disease were found centrally to have ER-negative tumours. A total of 2.2% (n = 7) had tumours with at least borderline amplification HER2 (three borderline but amplified, four amplified). Of these, one woman had a tumour that was both ER negative and HER2 positive centrally. Two additional tumours were heterogeneous, with discrete subpopulations of ER-positive and ER-negative cells and were, for this reason, deemed ineligible (< 1%).
This level of discrepancy is lower than reported in the majority of clinical trials of early breast cancer, although many of these have documented central review of HER2-positive (rather than HER2-negative) disease. For example, among cases regarded locally as ER-positive, 4% and 16%, respectively, were centrally redefined as ER-negative in two reviewing centres in Italy and the USA in the BIG 2-06/NCCTG N063D trial. 192 Similarly, in a centrally reviewed consecutive series of node-negative breast cancer from the Netherlands, 4% and 5% disagreement was seen for ER and HER2, respectively, between local and central tests (n = 694). 193 The low level of disagreement in receptor status between local and central laboratories that we report in this study reflects improvements in standardisation of receptor testing and the high levels of quality assurance in the UK including, for example, mandatory participation in National External Quality Assessment Service for Immunohistochemistry. 194
The overall 3.7% (95% CI 1.7% to 5.8%) incidence of discrepancy between local and central laboratories is reassuringly low. Although none of these cases underwent Oncotype DX testing, it is likely that such cases would generate high-risk RS and consequently be allocated chemotherapy. Of the seven (2.2%) patients who were found to have tumours that were HER2 amplified, three were classified as borderline while four were clearly amplified. Such patients would ordinarily be treated with adjuvant trastuzumab but received this treatment only by virtue of agreeing to join the OPTIMA prelim. The evidence of benefit for adjuvant trastuzumab treatment for cases with equivocal HER2 amplification195 is controversial.
Therefore, based on the experience in 325 tumours centrally tested for ER and HER2, given the discordant rates of ≤ 2.2% for each receptor separately, our experience with central review of ER and HER2 status suggests that this is unnecessary in a large Phase 3 trial.
Multiparameter assays
Oncotype DX was chosen as the primary discriminator for decisions on treatment allocation for OPTIMA prelim as this was judged most likely among the available tests to be acceptable to patients and clinicians alike. The evidence supporting the use of Oncotype DX was and remains significantly stronger than for potential alternatives. There is widespread familiarity with the test as the result of its successful marketing in the UK private health-care sector. This has been reinforced by the NICE DG10 guidance,117 which provides it with a stamp of authority that its competitors lack. Nevertheless there remain significant uncertainties in relation to the use of Oncotype DX and other multiparameter assays that justify the OPTIMA study.
One particular issue in relation to Oncotype DX is the choice of a cut-off point above which patients should be advised to have chemotherapy treatment. The test output is calibrated as risk of distant recurrence at 10 years in patients with ER-positive disease treated with tamoxifen alone. The Oxford Overview has clearly shown that there is no evidence that the recurrence risk in breast cancer patients who remain disease free 5 years after diagnosis is any different between those who did or did not receive adjuvant chemotherapy. This is despite the observation that one-third of the recurrences during the 10 years after diagnosis occur in the second half of this period. 9 Therefore, estimation of likelihood of chemotherapy benefit in relation to test output is inherently difficult. In the absence of robust data on the effectiveness of chemotherapy in relation to Oncotype DX RS, the choice of a cut-off point is always going to be somewhat arbitrary. The rationale for selection of a cut-off point of 25 is described above (see Chapter 2, Methods).
As there is no established method for the comparison between multiparameter assay platforms being attempted in the OPTIMA prelim, the analysis has required the development of a methodology. There is much uncertainty inherent in this, which is discussed in Chapter 6, Pathology study results. A particular issue is that the assays have mostly developed to indicate residual risk of relapse after 10 years of endocrine therapy and their predefined risk groupings reflect this. The limitations of this definition in relation to decisions on chemotherapy use are described in the previous paragraph. As there is no standardised definition of risk of relapse, the equivalent cut-off points for the available assays differ. This means that the proportions of cases assigned by the assays to low-risk (or not high risk where there are multiple divisions) and high-risk groups differ, which for the purposes of OPTIMA means that proportions of patients advised chemotherapy differ. Consequently, the assumptions for treatment benefit made in the health economics analysis are uncertain. As a result of this, any conclusions that can be drawn from this analysis are, at best, an estimate and require formal testing in a prospective study.
Pathology study results
This study within the OPTIMA prelim represents, to our knowledge, one of the few and certainly the most comprehensive ‘head-to-head’ comparisons of multiple tests designed to utilise tumour biology to predict patient residual risk. Rarely has there been the opportunity to compare results of multiple tests on a patient-by-patient basis to explore differences in risk categorisation. However, it should be remembered in the interpretation of these data that this study was designed with limited and focused goals. We are aware of the intense interest around this study and wish to ensure that results are interpreted in the appropriate context.
The second objective of this study, after demonstrating feasibility, was to aid the selection of candidate biological risk stratification tools for inclusion in the main OPTIMA trial. The OPTIMA trial aims to test whether or not, for ER-positive HER2-negative patients deemed to be clinically high risk, an additional biological predictor of risk can inform treatment. Specifically, for the clinically high-risk population is it appropriate to assign biologically low-risk patients to endocrine therapy without chemotherapy while retaining chemotherapy for clinically and biologically high-risk patients?
A key challenge for the OPTIMA triallists remains: which of the several validated diagnostic assays that assess biological risk should be used to address the trial question?
In addressing this second goal, we recognise a number of important factors, which both inform and limit the interpretation of results from the OPTIMA prelim pathology analysis.
-
OPTIMA prelim was neither designed nor powered to evaluate or compare the prognostic value of the tests included in the study. The tests that have been evaluated in the study have been extensively validated prior to inclusion in this study for their ability to inform risk, mostly following endocrine treatment. In addition, a number of elegant studies from the transATAC study group have shown that each test provides broadly similar risk discrimination in luminal breast cancers. 43,55,56 However, all tests, while of significant value in informing patient choice, are only modestly predictive of the risk of relapse at the individual patient level. Within the validation studies for each test there are patients assigned to ‘low-risk’ groups who relapse and die of their disease, and in all cases the majority of patients who are ‘high-risk’ do not in fact relapse and die from their disease. These tests therefore represent a significant step towards personalised medicine but are not yet perfect. This is important since it implies that discordance in risk estimates between tests that measure risk in different ways may not be unexpected.
-
The OPTIMA prelim study sought to evaluate diagnostic tests in a high-risk patient group as defined by tumour stage; for some tests this means that results from the OPTIMA prelim are using risk scores designed for node-negative disease in a predominantly node-positive population. This is in line with current thinking that ‘biological’ risk measures are informative across, and to a degree independent of, stage of disease. This thinking is supported by the fact that only grade, in the current study, is significantly associated with biological risk defined by multiparameter assay. This association may be explained by the fact that both grade and most biological risk tools assess tumour proliferation.
The key end point required for an appropriate choice between tests for the OPTIMA trial, which was also a key objective of the current OPTIMA prelim analysis, was to identify the proportion of cases assigned as high risk (or low risk) by each test, accepting that for each test this was a ‘true result’. As regards biological risk, since we cannot assess outcome, each test must function as its own gold standard.
Each test for which data are available provides an individual patient risk assessment. If all, or indeed any, of the candidate tests were 100% accurate in predicting risk (recurrence) it would be appropriate to use concordance estimates or kappa statistics to compare tests. However, in the absence of such a ‘gold standard’, kappa comparisons between tests simply reflect agreement between multiple modestly accurate tests. The low concordance between tests reflect the fact that the tests are measuring different genes, using different technology and highlight the problems of predicting recurrence risk based on the biology and management of the tumour.
We have presented comparisons between two different types of test results: (1) molecular subtype comparisons that group tests into luminal A, luminal B, etc. and (2) risk assessments where tests have included a risk prediction (either categorical or continuous). For tests generating continuous risk scores we have grouped patients into ‘low risk’ (patients who might safely avoid chemotherapy in the OPTIMA study) or ‘high risk’ (patients for whom their biological risk would suggest that chemotherapy should be given). For example, Oncotype DX provides both a continuous risk score and assigns patients to ‘low’, ‘intermediate’ or ‘high’ relapse risk. For the purposes of the OPTIMA trial we have combined the OPTIMA prelim cases classified by Oncotype DX as ‘low‘ (54% of cases) and ‘intermediate’ risk (28% of cases) into a single category of ‘low’ risk of relapse/low probability of chemotherapy benefit (called low score for convenience). For other tests, patients were categorised on the basis of pre-established cut-off points or, in the cases of tests providing subtype information (e.g. BluePrint), on the basis of subtype (luminal A vs. all others).
Comparison of risk categorisation and subtype prediction between tests
Of the five tests that categorise risk, Oncotype DX, Prosigna and IHC4 predicted similar rates of ‘low-risk’ cases, while MammaPrint identified fewer biologically low-risk cases in the OPTIMA prelim population (see Table 26). It should, however, be recognised that with the small sample size available (302 patients) we are not able to state clearly that, for example, Oncotype DX identifies more ‘low-risk’ patients than ‘Prosigna’, or vice versa. However, at a simple level the proportion of patients who, on the basis of these five tests, might safely avoid chemotherapy, is comparable and can be used to inform cost-effectiveness analyses (see Discussion of the health economic methodology and results). Differences in proportions of cases identified between tests will impact on the cost-effectiveness of different tests in the context of the main OPTIMA trial.
Three tests (BluePrint, Prosigna and MammaTyper) provide information on molecularly defined intrinsic subtypes. BluePrint and Prosigna provide this information alongside molecular profiling for residual risk, while MammaTyper is specifically focused on providing subtype information including a stratification of ‘luminal B’ cases into low and high risk.
As with risk scores, we show a broad similarity in the proportion of OPTIMA prelim cases, all of which are centrally confirmed as ER-positive and HER-negative, assigned by each test to luminal A or to luminal B/HER2 enriched/basal-like. BluePrint assigned 61%, MammaTyper assigned 62% and Prosigna assigned 60% of cases to the luminal A category and 39%, 38% and 40%, respectively, to non-luminal A subtypes. Although interesting, given that the study was recruiting only patients with luminal breast cancer, the identification of small numbers of ‘HER2-enriched’ or ‘basal-like’ ER-positive/HER2-negative cancers, particularly with complex multiparameter tests like Prosigna, is unsurprising and has been frequently reported in other studies (e.g. Parker et al. 28).
What is also clear from these data is that different tests commonly disagree about individual tumours. Only 93 (31%) of patients were classified as low/intermediate risk by all five tests (IHC4, IHC4 AQUA, Oncotype DX, MammaPrint and Prosigna) and only 26 (8%) patients were classified as high risk by all five tests. Over 60% of patients were classified as high risk by at least one test and low risk by at least one test. A similar finding has been reported in a comparison between PAM50 and Oncotype DX. 55 Similar, but perhaps more surprising, disagreement between the three tests that predict subtype was observed; only 59% of cases were consistently classed as either luminal A or ‘non-luminal A’ by all three subtyping tests. Kappa values give a measure of this disagreement where test thresholds are similar; for example, in the comparisons between Oncotype DX and IHC4 risk categorisation, and in the subtype predictions made by BluePrint and Prosigna, the kappa values were 0.53 and 0.55, respectively, indicating modest agreement.
The discrepancies between the tests are likely to reflect the differences in both the specific genes and the number of genes assessed by the individual tests. Test training may also contribute to discrepancies, although most of the tests were developed in ER-positive patient populations treated with endocrine therapy but not chemotherapy. This aspect of the OPTIMA prelim study will be of interest as we seek to understand the factors that underlie the different risk and subtype classifications.
It is not possible for us to draw any conclusions about the relative clinical performance of the tests in the absence of outcome data, and, in any case, the sample size is too small to allow an adequately powered comparison to be made. Our conclusion from this analysis is that these tests, each of which is independently validated as a tool to assess residual risk of relapse (although as yet there is no formal publication of validation studies for IHC4 AQUA and MammaTyper), are all modestly accurate meaning that differences between risk categorisation highlight the potential for improvements in assessment of residual risk without, at this stage, providing a means of discriminating between tests currently available.
Summary
In one of the few detailed comparisons of risk signatures at a patient level we have demonstrated that marked differences between diagnostic tests exist at an individual patient level. This is as predicted from the relatively modest performance of all current residual risk tests. Other studies performed in the field, although with fewer comparisons, suggest that all existing tests are broadly similar in the overall ‘quantity’ of risk information they provide to patients. 43,55,56 The analysis from the OPTIMA prelim study, although we lack clinical outcome, is consistent with this conclusion. Therefore, although interesting and informative, this test-to-test comparison is unable to direct choice of tests for the OPTIMA main trial beyond triaging towards those tests most likely to be cost-effective in the economic analyses described in Discussion of the health economic methodology and results. Some tests, clearly, may be less ideal as candidates for the main OPTIMA trial for technical reasons, but others cannot be easily discarded at this stage, and indeed may be selected solely on the basis of potential cost-effectiveness between these biologically similar test approaches.
What this test-to-test comparison does reveal, illustrated by those cases where discrepancies between tests exist, is that collectively there is significant room for improvement of the predictive impact of tests of residual risks, which at present remains unrealised.
Discussion of the health economic methodology and results
The economic analysis excluded IHC4 because of lack of cost information and because of concern about its analytical validity. Although MammaTyper has demonstrated analytical validity, there are no published clinical validation data and no cost information available. There are no published data on either the analytical or the clinical validity of IHC4 AQUA.
The results of the economic analysis require careful interpretation. The differences in the expected value between the alternative tests are relatively small, and the uncertainty in the evidence for all tests is substantial. Even the test with the best current clinical evidence base, Oncotype DX, is less (rather than more) likely to be a good use of limited NHS resources when considered in comparison with alternative tests, according to NICE’s standard value criteria. 148,196
MammaPrint is expected to be dominated by, that is produce fewer health benefits and cost more than, other options in all of the scenarios, and the probability of MammaPrint being cost-effective never exceeds single figures. On this basis, it seems reasonable to exclude MammaPrint from consideration for current adoption, as such a low proportion of simulations indicate that choosing MammaPrint would be a suboptional decision. One can speculate why this is the case, but it may be that because this is a dichotomous test, developed across the whole spectrum of breast cancer subtypes, it may not have been optimised for the patient cohort of those with a luminal breast cancer, which is the target population for the OPTIMA study. Despite its apparent low expected cost-effectiveness, MammaPrint is associated with considerable uncertainty and this manifests as research value in the value-of-information analysis.
The other strong conclusion is that test-directed chemotherapy appears to be cost-effective, regardless of the choice of tests. This supports the further development of an evidence base sufficient for robust adoptions decision-making.
An additional question is whether or not there is value in further research on some of these tests. The estimates of the value of further research for Oncotype, MammaPrint and Prosigna tests are sufficient to justify UK NHS investment in studies capable of reducing the test-specific uncertainty. The range of analyses presented here show that there is substantial uncertainty about the performance of each of the tests and there is also substantial uncertainty about the appropriate characterisation of the long-term outcome, as demonstrated by the variation in the results of the analyses across the different scenarios. Addressing the latter source of uncertainty within the trial may require a trial design with long-term detailed follow-up. It may be efficient to reduce the uncertainty on these factors through analysis of existing cancer registry data, in addition to within a clinical trial, as information on cancer recurrence and post-recurrence treatments starts to be captured in national data sets.
It is important to note that differing conclusions are drawn regarding the optimal use of Prosigna (ROR_PT or Subtype) depending on which sensitivity analysis is correct. This kind of model-dependent structural uncertainty is difficult to characterise in the value-of-information analysis. Given the lack of consensus between models it is difficult to recommend an optimal use of Prosigna based on purely economic grounds. Ideally, different ROR_PT cut-off points should be explored in further prospective research. If a single cut-off point needs to be chosen, as appears to be the case in light of the manufacturer’s marketing strategy, then it may be reasonable to base its choice using clinical or mechanistic justification.
The final notable observation from these results is that the value of undertaking further research around Oncotype DX, when the comparator is ‘chemotherapy for all’, is considerably lower than for the comparator technologies in all scenarios. This difference is likely to be driven by the higher uncertainties around the longer-term clinical outcomes, which is amplified in the model by discordance with Oncotype DX. The price differential will also be contributing, with Oncotype DX being more expensive per test than Prosigna. Again, further sensitivity analysis is required to fully understand what is driving the value of information and a more granular EVPPI analysis will enhance understanding.
The economic analysis conducted at the end of the OPTIMA prelim has presented a number of challenges. The use of value-of-information analysis to inform a design decision between a feasibility study and a fully powered RCT is a new application for this method, much advocated by methodologists in the UK and worldwide. 146 The need for influential assumptions within the model prior to the availability of evidence from a definitive trial necessitates extensive scenario analysis to test these assumptions. This has resulted in the need for three different model specifications and multiple scenarios, making the results complex to understand. The recommendation of Prosigna as most valuable for further research has withstood testing of the main assumptions, suggesting that it is a robust recommendation.
A particular additional challenge in the use of value-of-information analysis within a short analysis period is the computational expense. Each EVPPI calculation in this analysis, which uses 5000 Monte Carlo simulations in both the inner and outer loops, required in excess of 7 months of processing time using a fast processor. The OPTIMA prelim analysis was therefore only possible using a high-performance computing facility (White Rose Grid: www.whiterose.ac.uk/projectswhite-rose-grid/) to implement analyses in parallel.
A desirable further sensitivity analysis is exploration of the influence of Adjuvant! Online as a forecasting tool in the main economic analysis. It is possible that the reliance on this tool has biased the results in favour of tests for which the risk score correlates most highly with it. This is exemplified in Figures 18 and 19, which show that Prosigna ROR_PT is more highly correlated with Adjuvant! Online predictions than is Oncotype DX. The lack of CIs around Adjuvant! Online estimates also means that uncertainty will be underestimated in the model. The validation model is protected from this bias and further work exploring alternative tests using this model would be warranted. It is disappointing that validated alternatives to Adjuvant! Online reporting RFS are not available.
Overall conclusions
OPTIMA prelim has succeeded in all of its aims. The study was designed to establish whether or not a large-scale RCT of test-directed chemotherapy in patients with early breast cancer who are at significant future recurrence risk by virtue of axillary lymph-node involvement is feasible in the UK. The underlying hypothesis is that multiparameter assays are predictive of chemotherapy efficacy, which is a recommendation for future research in the NICE DG10 guidelines. 117
Prespecified targets were built into the protocol and the study was opened in a broadly representative set of UK centres. All targets were met, indicating feasibility of a study designed to demonstrate non-inferiority of chemotherapy allocation based on the outcome of a multiparameter assay performed on individual patients’ tumour samples.
Recruitment posed significant challenges. PPI in the study design and in the early phases of recruitment, and an integrated QRS provided valuable insights into barriers to recruitment, and resulted in changes to the PIS and to advice given to participating centres. These insights will be important for the main study.
Oncotype DX was used as the primary discriminator for chemotherapy decisions in the OPTIMA prelim. Additional multiparameter assays were performed on participant tumour samples and health-economic modelling was performed using the data. A substantial degree of discordance was demonstrated in the risk assignments/biological subtype of individual tumours although the overall amount of information provided by each assay in the overall population appears to be similar. Effectiveness studies are required to demonstrate whether or not the differences between assays are clinically significant. The health economics analysis suggests that, of the assays that were evaluated, there is substantial value in further research involving all tests, although Prosigna can currently be considered the highest priority from the perspective of the UK NHS. The level of uncertainty in health economics analyses undertaken, however, is substantial. A prospective study is therefore required to validate both the clinical and economic hypotheses that underlay the OPTIMA prelim.
Recommendations for further research
OPTIMA prelim has succeeded in its aims of demonstrating the feasibility, within the UK, of a large-scale clinical trial to establish a method of selecting patients with moderate- or high-risk hormone-sensitive primary breast cancer who are likely to benefit or not benefit from post-operative chemotherapy and to establish the cost-effectiveness of alternative test-guided treatment strategies. Existing data that support the use of multiparameter assays as an aid to making chemotherapy decisions are based on retrospective analysis of historical data. Very few data are available on the clinical validity of multiparameter assays in patients with lymph node involvement, which is the population in whom the cost-effectiveness of test-guided treatment decisions is likely to be greatest. The NICE DG10 guidance,117 which applies only to women with lymph node-negative breast cancer, recommends that further research on the ability of multiparameter assays to predict chemotherapy benefit be performed. A prospective clinical trial designed to answer these questions would be of benefit to the NHS and should be undertaken. It should be noted that, from the perspective of the NHS, the value of information for further research into Prosigna is higher than for the other two assays that were evaluated in the base-case analysis. The discrepancies demonstrated between the tests that were evaluated in this study at an individual patient/sample level show that there is substantial scope for refinement of exiting multiparameter assays.
Implications for practice
OPTIMA prelim was not designed and is not expected to generate any information that will result in a change in clinical practice. The finding of significant disagreement between multiparameter assays at an individual patient level should give clinicians currently using these technologies in their clinical practice pause for thought. As there is no established ‘best test’, not only are there limitations to the evidence base supporting multiparameter assay use but there are also limitations to the technology that is currently available. Nevertheless, the data generated by this study do not in any way undermine existing data supporting multiparameter assays use to guide chemotherapy decisions.
Acknowledgements
We would especially like to acknowledge the invaluable contribution of the 350 women who courageously volunteered to participate in the study. We would like to thank all research clinicians and staff in each trial centre for all their hard work in the conduct of this study. We are grateful to the members of the TSC and Independent Data Monitoring Committee for their support and guidance. Thanks are also given to members of the NIHR HTA programme who have been supportive and encouraging throughout this study.
Contributions of authors
All named authors are members of the OPTIMA TMG. All have contributed to the writing of this report. Their additional roles in the study and contribution to this report are listed under the TMG.
Trial Management Group
Robert C Stein (Consultant Medial Oncologist and Honorary Senior Lecturer, University College London Hospitals, London, UK) is CfI and lead of clinical aspects of the trial. He made substantial contributions to the concept and design of the study, its day-to-day management and overall conduct, and to data analysis and the writing of this report.
Janet A Dunn (Professor of Clinical Trials, University of Warwick, UK) was the CTU lead and senior statistician for the study. She substantially contributed to the trial design and conduct, including day-to-day management and monitoring as well as the statistical analysis plan. She contributed to the preparation of the methods and results section of the report. She was responsible for the patient focus groups.
John MS Bartlett (Programme Director and Honorary Professor, Ontario Institute of Cancer Research, Canada) was the translational research lead for the trial. He contributed to study design and managed tissue banking, the establishment of commercial relationships for undertaking multiparameter assays, the performance of laboratory assays and data analysis. He contributed to the relevant sections of the report.
Amy F Campbell (Trial Manager, Warwick CTU University of Warwick, UK) was responsible for the day-to-day management of the trial, monitored data collection, sample collection and analysis. She contributed to the writing of the methods and results sections and collated the report.
Andrea Marshall* (Principal Research Fellow in Medical Statistics, University of Warwick, UK) is the trial statistician. She contributed to the statistical analysis plan and conducted the statistical analysis of the data, and wrote the statistical methods and results sections of this report.
Peter Hall* (NIHR Clinical Lecturer Medical Oncology and Health Economics, University of Leeds, UK) contributed to the health economics aspects of study design, produced the health economics analysis plan, undertook the health economics analysis and contributed to the writing of the health economics aspects of the methods, results and discussion sections of this report.
Leila Rooshenas* (Research Associate, University of Bristol, UK) was the researcher for the qualitative research substudy. She conducted all interviews with professionals, analysed audio-recordings and provided feedback to centres. She wrote the QRS methods and results sections of this report.
Adrienne Morgan (Patient Advocate and Chairperson of ICPV Trustees) contributed to the design and overall conduct of the study, and to the preparation of the sections of the report discussing patient focus groups.
Christopher Poole (Professor of Medical Oncology, University of Warwick, UK) contributed to the design of the trial and its overall conduct, and to the preparation of the background and discussion sections of this report.
Sarah E Pinder (Professor of Breast Pathology, King’s College London, UK) contributed to the trial design, advised on pathology aspects of trial conduct and prepared important intellectual content for the discussion section of the report.
David A Cameron (Professor of Oncology and Head of Cancer Services, University of Edinburgh, UK) contributed to the study design and its overall conduct, and advised on the health economics and clinical aspects of the trial.
Nigel Stallard (Professor of Medical Statistics, University of Warwick, UK) advised on statistical analysis of diagnostic testing for the study design, and contributed to the trials design and statistical sections of this report.
Jenny L Donovan (Professor of Social Medicine, University of Bristol, UK) designed the QRS and revised the QRS methods and results sections of this report for important intellectual content.
Christopher McCabe (Professor of Health Economics, University of Alberta, Canada) contributed to the health economics aspects of study design. He advised on the health economic analysis plan and revised the health economic sections of this report for important intellectual content.
Luke Hughes-Davies (Consultant Oncologist, Addenbrooke’s Hospital, Cambridge, UK) is co-CfI. He contributed to the concept and design of the study, its day-to-day management and overall conduct, and to the preparation of the background and discussion sections of this report.
Andreas Makris (Consultant Clinical Oncologist, Mount Vernon Hospital, Northwood, UK) is co-CfI. He contributed to the concept and design of the study, its day-to-day management and overall conduct and contributed to the preparation of the background and discussion sections of this report.
*These authors made equal contributions to the analysis of data and their presentation in this report.
Other members of the Trial Management Group
Peter Canney (Consultant Oncologist, retired) contributed to the study concept and design.
Helena Earl (Reader in Clinical Cancer Medicine, University of Cambridge, UK) contributed to the study design and its overall conduct.
Mary Falzon (Consultant Histopathologist, UCL Hospitals, London, UK) was responsible for central eligibility testing and advised on pathology aspects of trial conduct.
Adele Francis (Consultant Breast Surgeon, University Hospital Birmingham, UK) advised on surgical aspects of study design and contributed to its overall conduct.
Victoria Harmer (Breast Care Nurse, Imperial College NHS Healthcare Trust, London, UK) advised on nursing aspects of the study.
Helen Higgins (Senior Project Manager Warwick CTU, University of Warwick, UK) managed the study opening and contributed its day-to-day management.
Claire Hulme (Health Economist, Leeds Institute of Health Sciences, Leeds, UK) advised on patient questionnaires and health-care utilisation analysis.
Iain Macpherson (Clinical Senior Lecturer in Medical Oncology, Beatson West of Scotland Cancer Centre, Glasgow, UK) contributed to the overall conduct of the study.
Daniel Rea (Senior Lecturer in Medical Oncology, University of Birmingham, UK) contributed to the study design and its overall conduct.
Funding
The OPTIMA study was funded by the NIHR HTA programme grant 10/34/01. This publication presents independent research commissioned by NIHR; the views and opinions expressed by authors in this publication are those of the authors and do not necessarily reflect those of the NHS, the NIHR, MRC, CCF, NETSCC, the HTA programme or the Department of Health.
Funding for OICR is provided by the Government of Ontario. Agendia Inc., NanoString Technologies, Stratifyer/BioNTech Diagnostics, and Genoptix Medical Laboratories supported testing by provision of reagents and test results (as appropriate) at no financial cost to the study.
Participating centres
The following centres and principal investigators contributed patients to the trial:
Addenbrooke’s Hospital: Dr Luke Hughes-Davies.
Alexandra Hospital, Redditch: Dr Denise Hrouda.
Ayr Hospital: Dr Graeme Lumsden.
Barnet Hospital: Dr Rob Stein.
Beatson West of Scotland Cancer Centre: Dr Iain Macpherson.
Bedford Hospital (Primrose Oncology Unit): Dr Sarah Smith.
Bristol Haematology and Oncology Centre: Dr Jeremey Braybrooke.
City Hospital, Birmingham: Dr Daniel Rea.
Dumfries and Galloway Royal Infirmary: Dr Tamsin Evans
Forth Valley Royal Hospital: Dr Judith Fraser.
Hairmyres Hospital, Lanarkshire: Dr Grainne Dunn.
Inverclyde Royal Hospital: Dr Abdulla Alhasso.
Luton and Dunstable University Hospital: Dr Mei-Lin Ah-See.
Mount Vernon Hospital: Dr Andreas Makris.
Musgrove Park Hospital: Dr John Graham.
Norfolk and Norwich University Hospital: Dr Adrian Hartnett.
Northwick Park Hospital: Dr Andreas Makris.
Peterborough City Hospital: Dr Karen McAdam.
Queen Elizabeth Hospital, Birmingham: Dr Daniel Rea.
Queen Elizabeth Hospital, King’s Lynn: Dr Margaret Daly.
Royal Alexandra Hospital: Dr Abdulla Alhasso.
Royal Devon and Exeter Hospital: Dr David Hwang.
Royal Glamorgan Hospital: Dr Jacinta Abraham.
Royal United Hospital Bath: Dr Mark Beresford.
St Bartholomew’s Hospital: Dr Rebecca Roylance.
The Christie: Dr Anne Armstrong.
The Woodlands Centre, Hinchingbrooke: Dr Cheryl Palmer.
Torbay Hospital: Dr Andrew Goodman.
University Hospital Coventry: Professor Christopher Poole.
University Hospital Crosshouse: Dr Graeme Lumsden.
Velindre Cancer Centre: Dr Annabel Borley.
Western General Hospital, Edinburgh: Dr Angela Bowman.
Wishaw General Hospital, Lanarkshire: Dr Jonathan Hicks.
Yeovil District Hospital: Dr Urmila Barthakur.
York District Hospital: Dr Andrew Proctor.
Independent members of the Trial Steering Committee
Professor Tim Maughan, Professor of Clinical Oncology, University of Oxford (chairperson); Professor Jon Deeks, Professor of Biostatistics, University of Birmingham; Professor Robert Leonard, Professor of Oncology, Imperial College, London; Dr Colin Purdie, Consultant Pathologist, Ninewells Hospital and Medical School, Dundee; Ms Julia Simister, Research Network Manager, Surrey West Sussex and Hampshire Cancer Research Network; and Professor Ian Tomlinson, Professor of Molecular and Population Genetics, Nuffield Department of Clinical Medicine, University of Oxford.
Independent Data Monitoring Committee members
Professor Lajos Pusztai, Chief of Breast Medical Oncology, Yale Cancer Centre, Yale School of Medicine (Chairperson); Professor Doug Altman, Director, Centre for Statistics in Medicine, University of Oxford; and Professor Giuseppe Viale, Director, Division of Pathology, European Institute of Oncology, University of Milan.
Other contributors
Warwick Clinical Trials Unit
Henry Adjei (Programmer, University of Warwick); Claire Balmer (OPTIMA Patient Focus Group Facilitator, University of Warwick); Rishi Bhandari (Randomisation Officer, University of Warwick); Scott Booth (Randomisation Officer, University of Warwick); Claire Daffern (Quality Assurance Manager, University of Warwick); Anchael Hyare, (Data Clerk, University of Warwick, UK); Sarah Lowe (Trial Administrator, University of Warwick); Chockalingam Muthiah (Programmer, University of Warwick); Kerry Raynes (Data Clerk, University of Warwick); Adrian Willis (Senior Programmer, University of Warwick); and Kate Packard (Trial Administrator, University of Warwick).
The OPTIMA Tissue Bank
Monika Sobol (Laboratory Technician, University of Edinburgh); Tammy Piper (Clinical Trials Laboratory Manager, University of Edinburgh); and Carrie Cunningham (Acting Clinical Trials Laboratory Manager and Laboratory Technician, University of Edinburgh).
Ontario Institute for Cancer Research
Jane Bayani (Scientific Associate, OICR); Mary Anne-Quintayo (Research Associate, OICR) and Fu-Yan (Research Technician, OICR).
Qualitative recruitment study
Sangeetha Paramasivan (Research Associate, University of Bristol).
Health Economists
Alison Smith and Armando Vargas-Palacios (Health Economists, University of Leeds); and Judy Wright (Information Specialist, University of Leeds).
Publications
Bartlett JMS, Stein RC, Bayani J, Marshall A, Dunn JA, Campbell AF, et al. on behalf of the OPTIMA Trial Management Group. Comparison of multiparameter tests in the UK OPTIMA-Prelim trial. 2014 San Antonio Breast Cancer Symposium. Cancer Res 2015;75(Suppl.):P4-11-07.
Hall PS, Smith AF, Vargas-Palacios A, Stein RC, Bartlett J, Bayani J, et al. UK OPTIMA-prelim study demonstrates economic value in more clinical evaluation of multi-parameter prognostic tests in early breast cancer. 2014 San Antonio Breast Cancer Symposium. Cancer Res 2015;75(Suppl.):P6-08-11.
Wason J, Marshall A, Dunn J, Stein RC, Stallard N. Adaptive designs for clinical trials assessing biomarker-guided treatment strategies. Br J Cancer 2014;110:1950–7.
Stein RC, Bartlett JMS, Peter Hall P, Marshall A, Rooshenas L, Campbell A, et al. Results of the OPTIMA (Optimal Personalised Treatment of early breast cancer usIng Multi-parameter Analysis) preliminary study. 2014 NCRI Cancer Conference, 2–5 November 2014, Liverpool, UK, abstract no. A128.
Rooshenas L, Donovan J, Paramasivan S, Bartlett JMS, Hall P, et al. Using genetic tests to determine chemotherapy provision in breast cancer: is recruiting into a trial of chemotherapy versus ‘test-directed’ chemotherapy possible? Addressing recruitment challenges in the OPTIMA trial. 2014 NCRI Cancer Conference, 2014 NCRI Cancer Conference, 2–5 November 2014, Liverpool, UK, abstract no. B126.
Wilson C, Rooshenas L, Birtle A, Stein R, Hall E, Donovan, J. The Uncertain Case for Adjuvant Chemotherapy: Lessons for Recruitment from Two Pragmatic Cancer Trials (Optima HTA10/34/01, POUT CRUK/11/027). 2014 NCRI Cancer Conference, 2014 NCRI Cancer Conference, 2–5 November 2014, Liverpool, UK, abstract no. B108.
Bartlett J, Canney P, Campbell A, Cameron D, Donovan J, Dunn J, et al. Selecting breast cancer patients for chemotherapy: the opening of the UK OPTIMA trial. Clinic Oncol 2013;25:109–16.
Dunn J, Marshall A, Campbell A, Stallard N, Hulme C, Higgins H, et al. Using adaptive designs for decision making within the optima trial: optimal personalised treatment of early breast cancer using multiparameter tests. Trials 2013;14(Suppl. 1):O12.
Hall P, McCabe C, Hulme C, Edlin R, Dunn J, Cameron D, et al. Efficient design of a phase 3 trial of competing tests for personalised cancer treatment in the absence of gold standard outcome data: challenges and potential solutions. Trials 2013;14(Suppl. 1):5.
Morgan A, Stein R, Makris A, Hughes-Davies L, Balmer C, Campbell, et al. Patient engagement. OPTIMA: Optimal Personalised Treatment of early breast cancer usIng Multiparameter Analysis (A63). National Cancer Research Institute, 2013 Cancer Conference, Liverpool, UK, 3–6 November, 2013.
Stein R, Makris A, Hughes-Davies L, Campbell A, Marshall A, Bartlett J, et al. OPTIMA prelim: optimal personalised treatment of early breast cancer using multiparameter tests. American Society of Clinical Oncology Annual Meeting Abstracts. J Clinic Oncol 2013;31:TPS656.
Stein R, Makris A, Hughes-Davies L, Campbell A, Marshall A, Bartlett J, et al. OPTIMA prelim: optimal personalised treatment of early breast cancer using multiparameter tests – study progress (A37). National Cancer Research Institute, 2013 Cancer Conference, Liverpool, UK, 3–6 November 2013.
Wason J, Stallard N, Dunn J, Stein R. An adaptive biomarker strategy clinical trial design. Trials 2013;14(Suppl. 1):103.
Stein R, Makris, A, Hughes-Davies L, Dunn J, Bartlett J, Donovan J, et al. OPTIMA prelim: Optimal personalised treatment of early breast cancer using multiparameter analysis: preliminary study. American Society of Clinical Oncology Annual Meeting Abstracts. J Clinic Oncol 2012;30:TPS665.
Data sharing statement
All available data related to this study, subject to clauses related to commercial confidentiality in the agreements with the vendors of multiparameter assays evaluated as part of this study, can be obtained from the corresponding author.
Disclaimers
This report presents independent research funded by the National Institute for Health Research (NIHR). The views and opinions expressed by authors in this publication are those of the authors and do not necessarily reflect those of the NHS, the NIHR, NETSCC, the HTA programme or the Department of Health. If there are verbatim quotations included in this publication the views and opinions expressed by the interviewees are those of the interviewees and do not necessarily reflect those of the authors, those of the NHS, the NIHR, NETSCC, the HTA programme or the Department of Health.
References
- Cancer Research UK . Breast Cancer Statistics – Key Facts n.d. http://info.cancerresearchuk.org/cancerstats/types/breast/ (accessed 14 February 2014).
- Cheang MC, Voduc D, Bajdik C, Leung S, McKinney S, Chia SK, et al. Basal-like breast cancer defined by five biomarkers has superior prognostic value than triple-negative phenotype. Clin Cancer Res 2008;14:368-76. http://dx.doi.org/10.1158/1078-0432.CCR-07-1658.
- Early Breast Cancer Trialists’ Collaborative Group . Aromatase inhibitors versus tamoxifen in early breast cancer: patient-level meta-analysis of the randomised trials. Lancet 2015;386:1341-52. http://dx.doi.org/10.1016/S0140-6736(15)61074-1.
- Early and Locally Advanced Breast Cancer: Diagnosis and Treatment (CG80). London: NICE; 2009.
- Day FL, Bull JM, Lombard JM, Stewart JF. Changes in medical oncology admissions for the management of breast cancer complications: an Australian institution’s experience. Asia Pac J Clin Oncol 2011;7:46-53. http://dx.doi.org/10.1111/j.1743-7563.2011.01391.x.
- Oladipo O, Coyle V, McAleer JJ, McKenna S. Achieving optimal dose intensity with adjuvant chemotherapy in elderly breast cancer patients: a 10-year retrospective study in a UK institution. Breast J 2012;18:6-22. http://dx.doi.org/10.1111/j.1524-4741.2011.01177.x.
- Carroll JP, Protani MM, Nguyen L, Cheng ME, Fay M, Saleem M, et al. Toxicity and tolerability of adjuvant breast cancer chemotherapy in obese women. Med Oncol 2014;31. http://dx.doi.org/10.1007/s12032-014-0881-z.
- Petrelli F, Borgonovo K, Cabiddu M, Lonati V, Barni S. Mortality, leukemic risk, and cardiovascular toxicity of adjuvant anthracycline and taxane chemotherapy in breast cancer: a meta-analysis. Breast Cancer Res Treat 2012;135:35-46. http://dx.doi.org/10.1007/s10549-012-2121-6.
- Peto R, Davies C, Godwin J, Grey R, Pan HC, . Early Breast Cancer Triallists’ Collaborative Group . Comparisons between different polychemotherapy regimens for early breast cancer: meta-analyses of long-term outcome among 100,000 women in 123 randomised trials. Lancet 2012;379:32-44.
- Jagsi R, Pottow JA, Griffith KA, Bradley C, Hamilton AS, Graff J, et al. Long-term financial burden of breast cancer: experiences of a diverse cohort of survivors identified through population-based registries. J Clin Oncol 2014;32:269-76. http://dx.doi.org/10.1200/JCO.2013.53.0956.
- Elklit A, Blum A. Psychological adjustment one year after the diagnosis of breast cancer: a prototype study of delayed post-traumatic stress disorder. Br J Clin Psychol 2011;50:350-63. http://dx.doi.org/10.1348/014466510X527676.
- O’Connor M, Christensen S, Jensen AB, Moller S, Zachariae R. How traumatic is breast cancer? Post-traumatic stress symptoms (PTSS) and risk factors for severe PTSS at 3 and 15 months after surgery in a nationwide cohort of Danish women treated for primary breast cancer. Br J Cancer 2011;104:19-26. http://dx.doi.org/10.1038/sj.bjc.6606073.
- Wolff AC, Blackford AL, Visvanathan K, Rugo HS, Moy B, Goldstein LJ, et al. Risk of marrow neoplasms after adjuvant breast cancer therapy: the National Comprehensive Cancer Network experience. J Clin Oncol 2015;33:40-8. http://dx.doi.org/10.1200/JCO.2013.54.6119.
- Pinder MC, Duan Z, Goodwin JS, Hortobagyi GN, Giordano SH. Congestive heart failure in older women treated with adjuvant anthracycline chemotherapy for breast cancer. J Clin Oncol 2007;25:3808-15. http://dx.doi.org/10.1200/JCO.2006.10.4976.
- Hall PS, McCabe C, Stein RC, Cameron D. Economic evaluation of genomic test-directed chemotherapy for early-stage lymph node-positive breast cancer. J Natl Cancer Inst 2012;104:56-6. http://dx.doi.org/10.1093/jnci/djr484.
- Cheung S, Greenway N, Lagord C, Williams L, Keirins O, Lawrence G. All Breast Cancer Report: A UK Analysis of All Symptomatic and Screen-Detected Breast Cancers Diagnosed in 2006. London: West Midlands Cancer Intelligence Unit, Public Health England; 2009.
- Ravdin PM, Siminoff LA, Davis GJ, Mercer MB, Hewlett J, Gerson N, et al. Computer program to assist in making decisions about adjuvant therapy for women with early breast cancer. J Clin Oncol 2001;19:980-91.
- Wishart GC, Bajdik CD, Dicks E, Provenzano E, Schmidt MK, Sherman M, et al. PREDICT plus: development and validation of a prognostic model for early breast cancer that includes HER2. Br J Cancer 2012;107:800-7. http://dx.doi.org/10.1038/bjc.2012.338.
- Blamey RW, Ellis IO, Pinder SE, Lee AH, Macmillan RD, Morgan DA, et al. Survival of invasive breast cancer according to the Nottingham Prognostic Index in cases diagnosed in 1990–1999. Eur J Cancer 2007;43:1548-55. http://dx.doi.org/10.1016/j.ejca.2007.01.016.
- Yerushalmi R, Woods R, Ravdin PM, Hayes MM, Gelmon KA. Ki67 in breast cancer: prognostic and predictive potential. Lancet Oncol 2010;11:174-83. http://dx.doi.org/10.1016/S1470-2045(09)70262-1.
- Polley MY, Leung SC, McShane LM, Gao D, Hugh JC, Mastropasqua MG, et al. An international Ki67 reproducibility study. J Natl Cancer Inst 2013;105:1897-906. http://dx.doi.org/10.1093/jnci/djt306.
- Dowsett M, Nielsen TO, A’Hern R, Bartlett J, Coombes RC, Cuzick J, et al. Assessment of Ki67 in breast cancer: recommendations from the International Ki67 in Breast Cancer Working Group. J Natl Cancer Inst 2011;103:1656-64. http://dx.doi.org/10.1093/jnci/djr393.
- Varga Z, Diebold J, Dommann-Scherrer C, Frick H, Kaup D, Noske A, et al. How reliable is Ki-67 immunohistochemistry in grade 2 breast carcinomas? A QA study of the Swiss Working Group of Breast- and Gynecopathologists. PLOS ONE 2012;7. http://dx.doi.org/10.1371/journal.pone.0037379.
- Polley MY, Leung SC, Gao D, Mastropasqua MG, Zabaglo LA, Bartlett JM, et al. An international study to increase concordance in Ki67 scoring. Mod Pathol 2015;28:778-86. http://dx.doi.org/10.1038/modpathol.2015.38.
- Perou CM, Sorlie T, Eisen MB, van de Rijn M, Jeffrey SS, Rees CA, et al. Molecular portraits of human breast tumours. Nature 2000;406:747-52. http://dx.doi.org/10.1038/35021093.
- Sorlie T, Tibshirani R, Parker J, Hastie T, Marron JS, Nobel A, et al. Repeated observation of breast tumor subtypes in independent gene expression data sets. Proc Natl Acad Sci USA 2003;100:8418-23. http://dx.doi.org/10.1073/pnas.0932692100.
- Sorlie T, Perou CM, Tibshirani R, Aas T, Geisler S, Johnsen H, et al. Gene expression patterns of breast carcinomas distinguish tumor subclasses with clinical implications. Proc Natl Acad Sci USA 2001;98:10869-74. http://dx.doi.org/10.1073/pnas.191367098.
- Parker JS, Mullins M, Cheang MC, Leung S, Voduc D, Vickery T, et al. Supervised risk predictor of breast cancer based on intrinsic subtypes. J Clin Oncol 2009;27:1160-7. http://dx.doi.org/10.1200/JCO.2008.18.1370.
- Caan BJ, Sweeney C, Habel LA, Kwan ML, Kroenke CH, Weltzien EK, et al. Intrinsic subtypes from the PAM50 gene expression assay in a population-based breast cancer survivor cohort: prognostication of short- and long-term outcomes. Cancer Epidemiol Biomark Prev 2014;23:725-34. http://dx.doi.org/10.1158/1055-9965.EPI-13-1017.
- Sweeney C, Bernard PS, Factor RE, Kwan ML, Habel LA, Quesenberry CP, et al. Intrinsic subtypes from PAM50 gene expression assay in a population-based breast cancer cohort: differences by age, race, and tumour characteristics. Cancer Epidemiol Biomark Prev 2014;23:714-24. http://dx.doi.org/10.1158/1055-9965.EPI-13-1023.
- Cheang MC, Chia SK, Voduc D, Gao D, Leung S, Snider J, et al. Ki67 index, HER2 status, and prognosis of patients with luminal B breast cancer. J Natl Cancer Inst 2009;101:736-50. http://dx.doi.org/10.1093/jnci/djp082.
- Curtis C, Shah SP, Chin SF, Turashvili G, Rueda OM, Dunning MJ, et al. The genomic and transcriptomic architecture of 2,000 breast tumours reveals novel subgroups. Nature 2012;486:346-52. http://dx.doi.org/10.1038/nature10983.
- Coates AS, Colleoni M, Goldhirsch A. Is adjuvant chemotherapy useful for women with luminal a breast cancer?. J Clin Oncol 2012;30:1260-3. http://dx.doi.org/10.1200/JCO.2011.37.7879.
- Hayes DF, Thor AD, Dressler LG, Weaver D, Edgerton S, Cowan D, et al. HER2 and response to paclitaxel in node-positive breast cancer. N Engl J Med 2007;357:1496-506. http://dx.doi.org/10.1056/NEJMoa071167.
- Gennari A, Sormani MP, Pronzato P, Puntoni M, Colozza M, Pfeffer U, et al. HER2 status and efficacy of adjuvant anthracyclines in early breast cancer: a pooled analysis of randomised trials. J Natl Cancer Inst 2008;100:14-20. http://dx.doi.org/10.1093/jnci/djm252.
- Colleoni M, Cole BF, Viale G, Regan MM, Price KN, Maiorano E, et al. Classical cyclophosphamide, methotrexate, and fluorouracil chemotherapy is more effective in triple-negative, node-negative breast cancer: results from two randomised trials of adjuvant chemoendocrine therapy for node-negative breast cancer. J Clin Oncol 2010;28:2966-73. http://dx.doi.org/10.1200/JCO.2009.25.9549.
- International Breast Cancer Study Group . Endocrine responsiveness and tailoring adjuvant therapy for postmenopausal lymph node- negative breast cancer: a randomized trial. J Natl Cancer Inst 2002;94:1054-65. http://dx.doi.org/10.1093/jnci/94.14.1054.
- Castiglione-Gertsch M, O’Neill A, Price KN, Goldhirsch A, Coastes AS, . International Breast Cancer Study Group . Adjuvant chemotherapy followed by goserelin versus either modality alone for premenopausal lymph node-negative breast cancer: a randomized trial. J Natl Cancer Inst 2003;95:1833-46. http://dx.doi.org/10.1093/jnci/djg119.
- Rouzier R, Perou CM, Symmans WF, Ibrahim N, Cristofanilli M, Anderson K, et al. Breast cancer molecular subtypes respond differently to preoperative chemotherapy. Clin Cancer Res 2005;11:5678-85. http://dx.doi.org/10.1158/1078-0432.CCR-04-2421.
- Esserman LJ, Berry DA, Cheang MC, Yau C, Perou CM, Carey L, et al. Chemotherapy response and recurrence-free survival in neoadjuvant breast cancer depends on biomarker profiles: results from the I-SPY 1 Trial (CALGB 150007/150012; ACRIN 6657). Breast Canc Res Treat 2012;132:1049-62. http://dx.doi.org/10.1007/s10549-011-1895-2.
- Paik S, Shak S, Tang G, Kim C, Baker J, Cronin M, et al. A multigene assay to predict recurrence of tamoxifen-treated, node-negative breast cancer. N Engl J Med 2004;351:2817-26. http://dx.doi.org/10.1056/NEJMoa041588.
- Ma XJ, Salunga R, Dahiya S, Wang W, Carney E, Durbecq V, et al. A five-gene molecular grade index and HOXB13:IL17BR are complementary prognostic factors in early stage breast cancer. Clin Cancer Res 2008;14:2601-8. http://dx.doi.org/10.1158/1078-0432.CCR-07-5026.
- Sgroi DC, Sestak I, Cuzick J, Zhang Y, Schnabel CA, Schroeder B, et al. Prediction of late distant recurrence in patients with oestrogen-receptor-positive breast cancer: a prospective comparison of the breast-cancer index (BCI) assay, 21-gene recurrence score, and IHC4 in the TransATAC study population. Lancet Oncol 2013;14:1067-76. http://dx.doi.org/10.1016/S1470-2045(13)70387-5.
- Simon RM, Paik S, Hayes DF. Use of archived specimens in evaluation of prognostic and predictive biomarkers. J Natl Cancer Inst 2009;101:1446-52. http://dx.doi.org/10.1093/jnci/djp335.
- Azim HA, Michiels S, Bedard PL, Singhal SK, Criscitiello C, Ignatiadis M, et al. Elucidating prognosis and biology of breast cancer arising in young women using gene expression profiling. Clin Cancer Res 2012;18:1341-51. http://dx.doi.org/10.1158/1078-0432.CCR-11-2599.
- Marchionni L, Wilson RF, Marinopoulos SS, Wolff AC, Parmigiani G, Bass EB, et al. Impact of Gene Expression Profiling Tests on Breast Cancer Outcomes. Rockville, MD: Agency for Healthcare Research and Quality; 2007.
- Smartt P. A Comparison of Gene Expression Profiling Tests for Breast Cancer. Christchurch: University of Canterbury, Health Services Assessment Collaboration; 2010.
- Hornberger J, Alvarado MD, Rebecca C, Gutierrez HR, Yu TM, Gradishar WJ. Clinical validity/utility, change in practice patterns, and economic implications of risk stratifiers to predict outcomes for early-stage breast cancer: a systematic review. J Natl Cancer Inst 2012;104:1068-79. http://dx.doi.org/10.1093/jnci/djs261.
- Ward S, Scope A, Rafia R, Pandor A, Harnan S, Evans P, et al. Gene expression profiling and expanded immunohistochemistry tests to guide the use of adjuvant chemotherapy in breast cancer management: a systematic review and cost-effectiveness analysis. Health Technol Assess 2013;17. http://dx.doi.org/10.3310/hta17440.
- Glas AM, Floore A, Delahaye LJ, Witteveen AT, Pover RC, Bakx N, et al. Converting a breast cancer microarray signature into a high-throughput diagnostic test. BMC Genomics 2006;7. http://dx.doi.org/10.1186/1471-2164-7-278.
- Mook S, Knauer M, Bueno-de-Mesquita JM, Retel VP, Wesseling J, Linn SC, et al. Metastatic potential of T1 breast cancer can be predicted by the 70-gene MammaPrint signature. Ann Surg Oncol 2010;17:1406-13. http://dx.doi.org/10.1245/s10434-009-0902-x.
- Foekens JA, Atkins D, Zhang Y, Sweep FC, Harbeck N, Paradiso A, et al. Multicenter validation of a gene expression-based prognostic signature in lymph node-negative primary breast cancer. J Clin Oncol 2006;24:1665-71. http://dx.doi.org/10.1200/JCO.2005.03.9115.
- Ring BZ, Seitz RS, Beck R, Shasteen WJ, Tarr SM, Cheang MC, et al. Novel prognostic immunohistochemical biomarker panel for estrogen receptor-positive breast cancer. J Clin Oncol 2006;24:3039-47. http://dx.doi.org/10.1200/JCO.2006.05.6564.
- Geiss GK, Bumgarner RE, Birditt B, Dahl T, Dowidar N, Dunaway DL, et al. Direct multiplexed measurement of gene expression with color-coded probe pairs. Nature Biotechnol 2008;26:317-25. http://dx.doi.org/10.1038/nbt1385.
- Dowsett M, Sestak I, Lopez-Knowles E, Sidhu K, Dunbier AK, Cowens JW, et al. Comparison of PAM50 risk of recurrence score with oncotype DX and IHC4 for predicting risk of distant recurrence after endocrine therapy. J Clin Oncol 2013;31:2783-90. http://dx.doi.org/10.1200/JCO.2012.46.1558.
- Cuzick J, Dowsett M, Pineda S, Wale C, Salter J, Quinn E, et al. Prognostic value of a combined estrogen receptor, progesterone receptor, Ki-67, and human epidermal growth factor receptor 2 immunohistochemical score and comparison with the Genomic Health recurrence score in early breast cancer. J Clin Oncol 2011;29:4273-8. http://dx.doi.org/10.1200/JCO.2010.31.2835.
- Sotiriou C, Wirapati P, Loi S, Harris A, Fox S, Smeds J, et al. Gene expression profiling in breast cancer: understanding the molecular basis of histologic grade to improve prognosis. J Natl Cancer Inst 2006;98:262-72. http://dx.doi.org/10.1093/jnci/djj052.
- Filipits M, Rudas M, Jakesz R, Dubsky P, Fitzal F, Singer CF, et al. A new molecular predictor of distant recurrence in ER-positive, HER2-negative breast cancer adds independent information to conventional clinical risk factors. Clin Cancer Res 2011;17:6012-20. http://dx.doi.org/10.1158/1078-0432.CCR-11-0926.
- Rakha EA, Soria D, Green AR, Lemetre C, Powe DG, Nolan CC, et al. Nottingham Prognostic Index Plus (NPI+): a modern clinical decision making tool in breast cancer. Br J Cancer 2014;110:1688-97. http://dx.doi.org/10.1038/bjc.2014.120.
- Aigner J, Schneeweiss A, Marme F, Eidt S, Altevogt P, Sinn P, et al. Ki-67 mRNA as a predictor for response to neoadjuvant chemotherapy in primary breast cancer. Cancer Res 2012;72:P3-.
- Paik S, Tang G, Shak S, Kim C, Baker J, Kim W, et al. Gene expression and benefit of chemotherapy in women with node-negative, estrogen receptor-positive breast cancer. J Clin Oncol 2006;24:3726-34. http://dx.doi.org/10.1200/JCO.2005.04.7985.
- Albain KS, Barlow WE, Shak S, Hortobagyi GN, Livingston RB, Yeh IT, et al. Prognostic and predictive value of the 21-gene recurrence score assay in postmenopausal women with node-positive, oestrogen-receptor-positive breast cancer on chemotherapy: a retrospective analysis of a randomised trial. Lancet Oncol 2010;11:55-6. http://dx.doi.org/10.1016/S1470-2045(09)70314-6.
- Mamounas EP, Tang G, Fisher B, Paik S, Shak S, Costantino JP, et al. Association between the 21-gene recurrence score assay and risk of locoregional recurrence in node-negative, estrogen receptor-positive breast cancer: results from NSABP B-14 and NSABP B-20. J Clin Oncol 2010;28:1677-83. http://dx.doi.org/10.1200/JCO.2009.23.7610.
- Tang G, Shak S, Paik S, Anderson SJ, Costantino JP, Geyer CE, et al. Comparison of the prognostic and predictive utilities of the 21-gene Recurrence Score assay and Adjuvant! for women with node-negative, ER-positive breast cancer: results from NSABP B-14 and NSABP B-20. Breast Cancer Res Treat 2011;127:133-42. http://dx.doi.org/10.1007/s10549-010-1331-z.
- Dowsett M, Cuzick J, Wale C, Forbes J, Mallon EA, Salter J, et al. Prediction of risk of distant recurrence using the 21-gene recurrence score in node-negative and node-positive postmenopausal patients with breast cancer treated with anastrozole or tamoxifen: a TransATAC study. J Clin Oncol 2010;28:1829-34. http://dx.doi.org/10.1200/JCO.2009.24.4798.
- Solin LJ, Grey R, Baehner FL, Butler SM, Hughes LL, Yoshizawa C, et al. A multigene expression assay to predict local recurrence risk for ductal carcinoma in situ of the breast. J Natl Cancer Inst 2013;105:701-10. http://dx.doi.org/10.1093/jnci/djt067.
- Schneider JG, Khalil DN. Why does Oncotype DX recurrence score reduce adjuvant chemotherapy use?. Breast Cancer Res Treat 2012;134:1125-32. http://dx.doi.org/10.1007/s10549-012-2134-1.
- Chang JC, Makris A, Gutierrez MC, Hilsenbeck SG, Hackett JR, Jeong J, et al. Gene expression patterns in formalin-fixed, paraffin-embedded core biopsies predict docetaxel chemosensitivity in breast cancer patients. Breast Cancer Res Treat 2008;108:233-40. http://dx.doi.org/10.1007/s10549-007-9590-z.
- Gianni L, Zambetti M, Clark K, Baker J, Cronin M, Wu J, et al. Gene expression profiles in paraffin-embedded core biopsy tissue predict response to chemotherapy in women with locally advanced breast cancer. J Clin Oncol 2005;23:7265-77. http://dx.doi.org/10.1200/JCO.2005.02.0818.
- Tang G, Cuzick J, Costantino JP, Dowsett M, Forbes JF, Crager M, et al. Risk of recurrence and chemotherapy benefit for patients with node-negative, estrogen receptor-positive breast cancer: recurrence score alone and integrated with pathologic and clinical factors. J Clin Oncol 2011;29:4365-72. http://dx.doi.org/10.1200/JCO.2011.35.3714.
- Ademuyiwa FO, Miller A, O’Connor T, Edge SB, Thorat MA, Sledge GW, et al. The effects of oncotype DX recurrence scores on chemotherapy utilization in a multi-institutional breast cancer cohort. Breast Cancer Res Treat 2011;126:797-802. http://dx.doi.org/10.1007/s10549-010-1329-6.
- Albanell J, Gonzalez A, Ruiz-Borrego M, Alba E, Garcia-Saenz JA, Corominas JM, et al. Prospective transGEICAM study of the impact of the 21-gene Recurrence Score assay and traditional clinicopathological factors on adjuvant clinical decision making in women with estrogen receptor-positive (ER+) node-negative breast cancer. Ann Oncol 2012;23:625-31. http://dx.doi.org/10.1093/annonc/mdr278.
- Braybrooke J, Kuchel A, Robinson T, Comins C, Shere M, Varughese M, et al. 2013.
- Holt S, Bertelli G, Humphreys I, Valentine W, Durrani S, Pudney D, et al. A decision impact, decision conflict and economic assessment of routine Oncotype DX testing of 146 women with node-negative or pNImi, ER-positive breast cancer in the U.K. Br J Cancer 2013;108:2250-8. http://dx.doi.org/10.1038/bjc.2013.207.
- Sestak I, Dowsett M, Zabaglo L, Lopez-Knowles E, Ferree S, Cowens JW, et al. Factors predicting late recurrence for estrogen receptor-positive breast cancer. J Natl Cancer Inst 2013;105:1504-11. http://dx.doi.org/10.1093/jnci/djt244.
- Prat A, Parker JS, Fan C, Cheang MC, Miller LD, Bergh J, et al. Concordance among gene expression-based predictors for ER-positive breast cancer treated with adjuvant tamoxifen. Ann Oncol 2012;23:2866-73. http://dx.doi.org/10.1093/annonc/mds080.
- Harris L, Fritsche H, Mennel R, Norton L, Ravdin P, Taube S, et al. American Society of Clinical Oncology 2007 update of recommendations for the use of tumor markers in breast cancer. J Clin Oncol 2007;25:5287-312. http://dx.doi.org/10.1200/JCO.2007.14.2364.
- Genomic Health . Genomic Health Annual Report 2014. http://investor.genomichealth.com/annuals.cfm (accessed 18 December 2015).
- van’t Veer LJ, Dai H, van de Vijver MJ, He YD, Hart AA, Mao M, et al. Gene expression profiling predicts clinical outcome of breast cancer. Nature 2002;415:530-6. http://dx.doi.org/10.1038/415530a.
- van de Vijver MJ, He YD, van’t Veer LJ, Dai H, Hart AA, Voskuil DW, et al. A gene expression signature as a predictor of survival in breast cancer. N Engl J Med 2002;347:1999-200. http://dx.doi.org/10.1056/NEJMoa021967.
- Sapino A, Roepman P, Linn SC, Snel MH, Delahaye LJ, van den Akker J, et al. MammaPrint molecular diagnostics on formalin-fixed, paraffin-embedded tissue. J Mol Diagn 2014;16:190-7. http://dx.doi.org/10.1016/j.jmoldx.2013.10.008.
- Bueno-de-Mesquita JM, Linn SC, Keijzer R, Wesseling J, Nuyten DS, van Krimpen C, et al. Validation of 70-gene prognosis signature in node-negative breast cancer. Breast Cancer Res Treat 2009;117:483-95. http://dx.doi.org/10.1007/s10549-008-0191-2.
- Buyse M, Loi S, van’t Veer L, Viale G, Delorenzi M, Glas AM, et al. Validation and clinical utility of a 70-gene prognostic signature for women with node-negative breast cancer. J Natl Cancer Inst 2006;98:1183-92. http://dx.doi.org/10.1093/jnci/djj329.
- Kunz G. Use of a genomic test (MammaPrint) in daily clinical practice to assist in risk stratification of young breast cancer patients. Arch Gynecol Obstet 2011;283:597-602. http://dx.doi.org/10.1007/s00404-010-1454-9.
- Mook S, Schmidt MK, Weigelt B, Kreike B, Eekhout I, van de Vijver MJ, et al. The 70-gene prognosis signature predicts early metastasis in breast cancer patients between 55 and 70 years of age. Ann Oncol 2010;21:717-22. http://dx.doi.org/10.1093/annonc/mdp388.
- Gluck S, de Snoo F, Peeters J, Stork-Sloots L, Somlo G. Molecular subtyping of early-stage breast cancer identifies a group of patients who do not benefit from neoadjuvant chemotherapy. Breast Cancer Res Treat 2013;139:759-67. http://dx.doi.org/10.1007/s10549-013-2572-4.
- Straver ME, Glas AM, Hannemann J, Wesseling J, van de Vijver MJ, Rutgers EJ, et al. The 70-gene signature as a response predictor for neoadjuvant chemotherapy in breast cancer. Breast Cancer Res Treat 2010;119:551-8. http://dx.doi.org/10.1007/s10549-009-0333-1.
- Knauer M, Mook S, Rutgers EJ, Bender RA, Hauptmann M, van de Vijver MJ, et al. The predictive value of the 70-gene signature for adjuvant chemotherapy in early breast cancer. Breast Cancer Res Treat 2010;120:655-61. http://dx.doi.org/10.1007/s10549-010-0814-2.
- Bueno-de-Mesquita JM, van Harten WH, Retel VP, van’t Veer LJ, van Dam FS, Karsenberg K, et al. Use of 70-gene signature to predict prognosis of patients with node-negative breast cancer: a prospective community-based feasibility study (RASTER). Lancet Oncol 2007;8:1079-87. http://dx.doi.org/10.1016/S1470-2045(07)70346-7.
- Drukker CA, van den Hout HC, Sonke GS, Brain E, Bonnefoi H, Cardoso F, et al. Risk estimations and treatment decisions in early stage breast cancer: agreement among oncologists and the impact of the 70-gene signature. Eur J Cancer 2014;50:1045-54. http://dx.doi.org/10.1016/j.ejca.2014.01.016.
- Drukker CA, Bueno-de-Mesquita JM, Retel VP, van Harten WH, van Tinteren H, Wesseling J, et al. A prospective evaluation of a breast cancer prognosis signature in the observational RASTER study. Int J Cancer 2013;133:929-36. http://dx.doi.org/10.1002/ijc.28082.
- Drukker CA, van Tinteren H, Schmidt MK, Rutgers EJ, Bernards R, van de Vijver MJ, et al. Long-term impact of the 70-gene signature on breast cancer outcome. Breast Cancer Res Treat 2014;143:587-92. http://dx.doi.org/10.1007/s10549-013-2831-4.
- Dowsett M, Allred C, Knox J, Quinn E, Salter J, Wale C, et al. Relationship between quantitative estrogen and progesterone receptor expression and human epidermal growth factor receptor 2 (HER-2) status with recurrence in the Arimidex, Tamoxifen, Alone or in Combination trial. J Clin Oncol 2008;26:1059-65. http://dx.doi.org/10.1200/JCO.2007.12.9437.
- Camp RL, Chung GG, Rimm DL. Automated subcellular localization and quantification of protein expression in tissue microarrays. Nat Med 2002;8:1323-7. http://dx.doi.org/10.1038/nm791.
- Christiansen J, Bartlett JMS, Gustavson M, Rimm D, Robson T, Van De Velde CJH, et al. Validation of IHC4 algorithms for prediction of risk of recurrence in early breast cancer using both conventional and quantitative IHC approaches. J Clin Oncol 2012;30.
- Barton S, Zabaglo L, A’Hern R, Turner N, Ferguson T, O’Neill S, et al. Assessment of the contribution of the IHC4+C score to decision making in clinical practice in early breast cancer. Br J Cancer 2012;106:1760-5. http://dx.doi.org/10.1038/bjc.2012.166.
- Wallden B, Storhoff J, Nielsen T, Dowidar N, Schaper C, Ferree S, et al. Development and verification of the PAM50-based Prosigna breast cancer gene signature assay. BMC Med Genomics 2015;8.
- Nielsen T, Wallden B, Schaper C, Ferree S, Liu S, Gao D, et al. Analytical validation of the PAM50-based Prosigna Breast Cancer Prognostic Gene Signature Assay and Counter Analysis System using formalin-fixed paraffin-embedded breast tumor specimens. BMC Cancer 2014;14. http://dx.doi.org/10.1186/1471-2407-14-177.
- Nielsen TO, Parker JS, Leung S, Voduc D, Ebbert M, Vickery T, et al. A comparison of PAM50 intrinsic subtyping with immunohistochemistry and clinical prognostic factors in tamoxifen-treated estrogen receptor-positive breast cancer. Clin Cancer Res 2010;16:5222-32. http://dx.doi.org/10.1158/1078-0432.CCR-10-1282.
- Gnant M, Filipits M, Greil R, Stoeger H, Rudas M, Bago-Horvath Z, et al. Predicting distant recurrence in receptor-positive breast cancer patients with limited clinicopathological risk: using the PAM50 Risk of Recurrence score in 1478 postmenopausal patients of the ABCSG-8 trial treated with adjuvant endocrine therapy alone. Ann Oncol 2014;25:339-45. http://dx.doi.org/10.1093/annonc/mdt494.
- Filipits M, Nielsen TO, Rudas M, Greil R, Stoger H, Jakesz R, et al. The PAM50 risk-of-recurrence score predicts risk for late distant recurrence after endocrine therapy in postmenopausal women with endocrine-responsive early breast cancer. Clin Cancer Res 2014;20:1298-305. http://dx.doi.org/10.1158/1078-0432.CCR-13-1845.
- Prat A, Galván P, Jimenez B, Buckingham W, Jeiranian HA, Schaper C, et al. Prediction of response to neoadjuvant chemotherapy using core needle biopsy samples with the Prosigna assay. Clin Cancer Res 2016;22:560-6. http://dx.doi.org/10.1158/1078-0432.CCR-15-0630.
- Cheang MC, Voduc KD, Tu D, Jiang S, Leung S, Chia SK, et al. Responsiveness of intrinsic subtypes to adjuvant anthracycline substitution in the NCIC.CTG MA.5 randomized trial. Clin Cancer Res 2012;18:2402-12. http://dx.doi.org/10.1158/1078-0432.CCR-11-2956.
- Martin M, Prat A, Rodriguez-Lescure A, Caballero R, Ebbert MT, Munarriz B, et al. PAM50 proliferation score as a predictor of weekly paclitaxel benefit in breast cancer. Breast Cancer Res Treat 2013;138:457-66. http://dx.doi.org/10.1007/s10549-013-2416-2.
- Jorgensen CL, Nielsen TO, Bjerre KD, Liu S, Wallden B, Balslev E, et al. PAM50 breast cancer intrinsic subtypes and effect of gemcitabine in advanced breast cancer patients. Acta Oncol 2014;53:776-87. http://dx.doi.org/10.3109/0284186X.2013.865076.
- Bartlett JM, Thomas J, Ross DT, Seitz RS, Ring BZ, Beck RA, et al. Mammostrat as a tool to stratify breast cancer patients at risk of recurrence during endocrine therapy. Breast Cancer Res 2010;12. http://dx.doi.org/10.1186/bcr2604.
- Bartlett JM, Bloom KJ, Piper T, Lawton TJ, van de Velde CJ, Ross DT, et al. Mammostrat as an immunohistochemical multigene assay for prediction of early relapse risk in the tamoxifen versus exemestane adjuvant multicenter trial pathology study. J Clin Oncol 2012;30:4477-84. http://dx.doi.org/10.1200/JCO.2012.42.8896.
- Mislick K, Schonfeld W, Bodnar C, Tong KB. Cost-effectiveness analysis of Mammostrat® compared with Oncotype DX(R) to inform the treatment of breast cancer. Clinicoecon Outcomes Res 2014;6:37-4. http://dx.doi.org/10.2147/CEOR.S53142.
- Fan C, Oh DS, Wessels L, Weigelt B, Nuyten DS, Nobel AB, et al. Concordance among gene-expression-based predictors for breast cancer. N Engl J Med 2006;355:560-9. http://dx.doi.org/10.1056/NEJMoa052933.
- Zhao X, Rodland EA, Sorlie T, Vollan HK, Russnes HG, Kristensen VN, et al. Systematic assessment of prognostic gene signatures for breast cancer shows distinct influence of time and ER status. BMC Cancer 2014;14. http://dx.doi.org/10.1186/1471-2407-14-211.
- Lamond NW, Skedgel C, Rayson D, Lethbridge L, Younis T. Cost-utility of the 21-gene recurrence score assay in node-negative and node-positive breast cancer. Breast Cancer Res Treat 2012;133:1115-23. http://dx.doi.org/10.1007/s10549-012-1989-5.
- Vanderlaan BF, Broder MS, Chang EY, Oratz R, Bentley T. Cost-effectiveness of 21-gene assay in node-positive, early-stage breast cancer. Am J Manag Care 2010;17:455-64.
- Eiermann W, Rezai M, Kümmel S, Kühn T, Warm M, Friedrichs K, et al. The 21-gene recurrence score assay impacts adjuvant therapy recommendations for ER-positive, node-negative and node-positive early breast cancer resulting in a risk-adapted change in chemotherapy use. Ann Oncol 2013;24:618-24. http://dx.doi.org/10.1093/annonc/mds512.
- O’Leary B, Foteff C, Byron K, Chang C, Chao C, Ng C, et al. PCN12 cost-effectiveness of the Oncotype DX® assay in Australia: an exploratory analysis. Value Health 2010;13. http://dx.doi.org/10.1016/S1098-3015(11)73105-9.
- Kondo M, Hoshi SL, Yamanaka T, Ishiguro H, Toi M. Economic evaluation of the 21-gene signature (Oncotype DX) in lymph node-negative/positive, hormone receptor-positive early-stage breast cancer based on Japanese validation study (JBCRG-TR03). Breast Cancer Res Treat 2011;127:739-49. http://dx.doi.org/10.1007/s10549-010-1243-y.
- Oestreicher N, Ramsey SD, Linden HM, McCune JS, van’t Veer LJ, Burke W, et al. Gene expression profiling and breast cancer care: what are the potential benefits and policy implications?. Genet Med 2005;7:380-9. http://dx.doi.org/10.1097/01.GIM.0000170776.31248.75.
- Gene Expression Profiling and Expanded Immunohistochemistry Tests for Guiding Adjuvant Chemotherapy Decisions in Early Breast Cancer Management: MammaPrint, Oncotype DX, IHC4 and Mammostrat [DG10]. London: NICE; 2013.
- Ragaz J, Wilson K, Wong H, Muraca G, Hryniuk W, Bajdik C. PD06–01: Molecular classification with 21 gene assay (Oncotype DX) shows in 196,967 ER positive patients high frequency of low recurrence score (LRS) in both node positive (N+) and negative (N-) breast cancer (BrCa) cohorts. Definitions of chemoresistance based on LRS with cost and guideline implications. Cancer Res 2011;71. http://dx.doi.org/10.1158/0008-5472.SABCS11-PD06-01.
- Zarca D, Stork-Sloots L, de Snoo F, Brink G, Dervaux Y, Boubli L. 5167 French cost effectiveness study of the MammaPrint 70-gene signature in early stage breast cancer patients. Eur J Cancer Suppl 2009;7. http://dx.doi.org/10.1016/S1359-6349(09)71059-6.
- Gene Expression Profiling and Expanded Immunohistochemistry Tests to Guide Selection of Chemotherapy Regimes in Breast Cancer Management: MammaPrint, Oncotype DX, IHC4 and Mammostrat: Amended Final Scope. London: NICE; 2011.
- TAILORx . Program for the Assessment of Clinical Cancer Tests (PACCT-1): Trial Assigning Individualized Options for Treatment: The TAILORx Trial 2014. clinicaltrials.gov/show/NCT00310180 (accessed 27 April 2014).
- MINDACT . MINDACT (Microarray In Node-Negative and 1 to 3 Positive Lymph Node Disease May Avoid Chemotherapy): A Prospective, Randomised Study Comparing the 70-Gene Signature With the Common Clinical-Pathological Criteria in Selecting Patients for Adjuvant Chemotherapy in Breast Cancer With 0 to 3 Positive Nodes n.d. clinicaltrials.gov/show/NCT00310180 (accessed 27 April 2014).
- RxPONDER . A Phase III, Randomized Clinical Trial of Standard Adjuvant Endocrine Therapy + - Chemotherapy in Patients With 1–3 Positive Nodes, Hormone Receptor-Positive and HER2-Negative Breast Cancer With Recurrence Score (RS) of 25 or Less. RxPONDER: A Clinical Trial Rx for Positive Node, Endocrine Responsive Breast Cancer n.d. clinicaltrials.gov/show/NCT01272037 (accessed 27 April 2014).
- Zujewski JA, Kamin L. Trial assessing individualized options for treatment for breast cancer: the TAILORx trial. Future Oncol 2008;4:603-10. http://dx.doi.org/10.2217/14796694.4.5.603.
- The EuroQol Group . EuroQol – a new facility for the measurement of health-related quality of life. Health Policy 1990;16:199-208. http://dx.doi.org/10.1016/0168-8510(90)90421-9.
- Cella DF, Tulsky DS, Grey G, Sarafian B, Linn E, Bonomi A, et al. The Functional Assessment of Cancer Therapy scale: development and validation of the general measure. J Clin Oncol 1993;11:570-9.
- Brady MJ, Cella DF, Mo F, Bonomi AE, Tulsky DS, Lloyd SR, et al. Reliability and validity of the Functional Assessment of Cancer Therapy-Breast quality-of-life instrument. J Clin Oncol 1997;15:974-86.
- Colleoni M, Gelber RD. Time to initiation of adjuvant chemotherapy for early breast cancer and outcome: the earlier, the better?. J Clin Oncol 2014;32:717-19. http://dx.doi.org/10.1200/JCO.2013.54.3942.
- Data Protection Act 1998. Chapter 29. London: The Stationery Office; 1998.
- Buckley CJ, Rutherford RB, Diethrich EB, Buckley SD. Inherent problems with randomized clinical trials with observational/no treatment arms. J Vasc Surg 2010;52:237-41. http://dx.doi.org/10.1016/j.jvs.2010.02.255.
- Ellis PM, Butow PN, Tattersall MH, Dunn SM, Houssami N. Randomized clinical trials in oncology: understanding and attitudes predict willingness to participate. J Clin Oncol 2001;19:3554-61.
- Jenkins V, Fallowfield L. Reasons for accepting or declining to participate in randomized clinical trials for cancer therapy. Br J Cancer 2000;82. http://dx.doi.org/10.1054/bjoc.2000.1142.
- Welton AJ, Vickers MR, Cooper JA, Meade TW, Marteau TM. Is recruitment more difficult with a placebo arm in randomised controlled trials? A quasirandomised, interview based study. BMJ 1999;318:1114-17. http://dx.doi.org/10.1136/bmj.318.7191.1114.
- Kidd PS, Parshall MB. Getting the focus and the group: enhancing analytical rigor in focus group research. Qual Health Res 2000;10:293-308. http://dx.doi.org/10.1177/104973200129118453.
- Powell RA, Single HM. Focus groups. Int J Qual Health Care 1996;8:499-504. http://dx.doi.org/10.1093/intqhc/8.5.499.
- Webb C, Kevern J. Focus groups as a research method: a critique of some aspects of their use in nursing research. J Adv Nurse 2001;33:798-805. http://dx.doi.org/10.1046/j.1365-2648.2001.01720.x.
- Onwuegbuzie AJ, Dickinson WB, Leech NL, Zoran AG. A qualitative framework for collecting and analyzing data in focus group research. Int J Qual Methods 2009;8.
- Glaser B, Strauss A. The Discovery of Grounded Theory: Strategies for Qualitative Research. Piscataway, NJ: Transaction Publishers; 1967.
- Rabiee F. Focus-group interview and data analysis. Proc Nutr Soc 2004;63:655-60. http://dx.doi.org/10.1079/PNS2004399.
- Donovan JL, Athene Lane J, Peters TJ, Brindle L, Salter E, Gillatt D, et al. Development of a complex intervention improved randomization and informed consent in a randomized controlled trial. J Clin Epidemiol 2009;62:29-36. http://dx.doi.org/10.1016/j.jclinepi.2008.02.010.
- Tong A, Sainsbury P, Craig J. Consolidated criteria for reporting qualitative research (COREQ): a 32-item checklist for interviews and focus groups. Int J Qual Health Care 2007;19:349-57. http://dx.doi.org/10.1093/intqhc/mzm042.
- Heritage J, Robinson JD. The structure of patients’ presenting concerns: physicians’ opening questions. Health Commun 2006;19:89-102. http://dx.doi.org/10.1207/s15327027hc1902_1.
- Wade J, Donovan JL, Athene Lane J, Neal DE, Hamdy FC. It’s not just what you say, it’s also how you say it: opening the ‘black box’ of informed consent appointments in randomised controlled trials. Soc Sci Med 2009;68:2018-28. http://dx.doi.org/10.1016/j.socscimed.2009.02.023.
- Bartlett JM, Brookes CL, Robson T, van de Velde CJ, Billingham LJ, Campbell FM, et al. Estrogen receptor and progesterone receptor as predictive biomarkers of response to endocrine therapy: a prospectively powered pathology study in the Tamoxifen and Exemestane Adjuvant Multinational trial. J Clin Oncol 2011;29:1531-8. http://dx.doi.org/10.1200/JCO.2010.30.3677.
- Coates AS, Winer EP, Goldhirsch A, Gelber RD, Gnant M, Piccart-Gebhart M, et al. Tailoring therapies-improving the management of early breast cancer: St Gallen International Expert Consensus on the Primary Therapy of Early Breast Cancer 2015. Ann Oncol 2015;26:1533-46. http://dx.doi.org/10.1093/annonc/mdv221.
- Claxton K, Sculpher M, Drummond M. A rational framework for decision making by the National Institute For Clinical Excellence (NICE). Lancet 2002;360:711-15. http://dx.doi.org/10.1016/S0140-6736(02)09832-X.
- Sonnenberg FA, Beck JR. Markov models in medical decision making a practical guide. Med Decis Making 1993;13:322-38. http://dx.doi.org/10.1177/0272989X9301300409.
- Guide to the Methods of Technology Appraisal. London: NICE; 2013.
- Hall PS, Hulme C, McCabe C, Oluboyede Y, Round J, Cameron DA. Updated cost-effectiveness analysis of trastuzumab for early breast cancer. Pharmacoeconomics 2011;29:415-32. http://dx.doi.org/10.2165/11588340-000000000-00000.
- Common Terminology Criteria for Adverse Events (CTCAE) Version 4.0. Washington, DC: US Department of Health and Human Services; 2012.
- Jones S, Holmes FA, O’Shaughnessy J, Blum JL, Vukelja SJ, McIntyre KJ, et al. Docetaxel with cyclophosphamide is associated with an overall survival benefit compared with doxorubicin and cyclophosphamide: 7-year follow-up of US Oncology Research Trial 9735. J Clin Oncol 2009;27:1177-83. http://dx.doi.org/10.1200/JCO.2008.18.4028.
- Roché H, Fumoleau P, Spielmann M, Canon J-L, Delozier T, Serin D, et al. Sequential adjuvant epirubicin-based and docetaxel chemotherapy for node-positive breast cancer patients: the FNCLCC PACS 01 Trial. J Clin Oncol 2006;24:5664-71. http://dx.doi.org/10.1200/JCO.2006.07.3916.
- Cameron D, Barrett-Lee P, Velikova C, Canney P, Moyses H, McDermaid M, et al. TACT2 Randomised Adjuvant Trial in Early Breast Cancer (EBC): Tolerability and Toxicity of Standard 3 Weekly Epirubicin (E) Versus Accelerated Epirubicin (AE) in 129 UK Hospitals (4391 Patients) n.d.
- Townsend N, Wickramasinghe K, Bhatnagar P, Smolina K, Nichols M, Leal J, et al. Coronary Heart Disease Statistics: A Compendium of Health Statistics. London: British Heart Foundation; 2012.
- Cowie M, Wood D, Coats A, Thompson S, Suresh V, Poole-Wilson P, et al. Survival of patients with a new diagnosis of heart failure: a population based study. Heart 2000;83:505-10. http://dx.doi.org/10.1136/heart.83.5.505.
- Baum M, Buzdar A, Cuzick J, Forbes J, Houghton J, Howell A, et al. Anastrozole alone or in combination with tamoxifen versus tamoxifen alone for adjuvant treatment of postmenopausal women with early-stage breast cancer: results of the ATAC (Arimidex, Tamoxifen Alone or in Combination) trial efficacy and safety update analyses. Cancer 2003;98:1802-10. http://dx.doi.org/10.1002/cncr.11745.
- Walkington L, Newsham A, Deverede L, Afshar M, Hall P, Perren T, et al. Patterns of Breast Cancer Recurrence and Associated Health Care Costs of 1000 Patients: A Longitudinal Study n.d.
- Interim Life Tables for England and Wales 2006–2008. London: UK Government; 2009.
- Bhayat F, Das-Gupta E, Smith C, McKeever T, Hubbard R. The incidence of and mortality from leukaemias in the UK: a general population-based study. BMC Cancer 2009;9. http://dx.doi.org/10.1186/1471-2407-9-252.
- Azim HA, de Azambuja E, Colozza M, Bines J, Piccart MJ. Long-term toxic effects of adjuvant chemotherapy in breast cancer. Ann Oncol 2011;22:1939-47. http://dx.doi.org/10.1093/annonc/mdq683.
- Praga C, Bergh J, Bliss J, Bonneterre J, Cesana B, Coombes RC, et al. Risk of acute myeloid leukemia and myelodysplastic syndrome in trials of adjuvant epirubicin for early breast cancer: correlation with doses of epirubicin and cyclophosphamide. J Clin Oncol 2005;23:4179-91. http://dx.doi.org/10.1200/JCO.2005.05.029.
- Oliver S, Taylor F, Bolton ME, Brook C, Ferguson B, Ross H, et al. Haematological Malignancies in England Cancers Diagnosed 2001–2008. London: Northern Cancer Intelligence Network; 2013.
- Curtis L, Netten A. Unit Costs of Health and Social Care 2014. Canterbury: PSSRU, University of Kent; 2014.
- Drugs and Pharmaceutical Electronic Market Information (Emit). London: HMSO; 2011.
- Medicines Complete . British National Formulary n.d. www.medicinescomplete.com/mc/bnf/current/ (accessed 18 December 2015).
- NHS Reference Costs 2012–2013. London: Department of Health; 2013.
- Hall P, Vargas-Palacios A, Newsham A, Walkington L, Glaser A, Hall G. Costs of Hospital Care Over 10 Years from Diagnosis of Early Breast Cancer n.d.
- Report: A Simple Guide to Payment by Results. London: Department of Health; 2014.
- Lin D, Feuer E, Etzioni R, Wax Y. Estimating medical costs from incomplete follow-up data. Biometrics 1997;53:419-34. http://dx.doi.org/10.2307/2533947.
- Her Majesty’s Revenue and Customs . VAT: Clarification of HMRC’s Policy on Laboratory Pathology Services Provided by State-Regulated Institutions, 2013 n.d. www.hmrc.gov.uk/briefs/vat/brief1613.htm (accessed 17 May 2014).
- National Cancer Intelligence Network . Breast Cancer n.d. www.ncin.org.uk/cancer_type_and_topic_specific_work/cancer_type_specific_work/breast_cancer/ (accessed 18 December 2015).
- Peasgood T, Ward SE, Brazier J. Health-state utility values in breast cancer. Expert Rev Pharmacoecon Outcomes Res 2010;10:553-66. http://dx.doi.org/10.1586/erp.10.65.
- Kind P, Dolan P, Gudex C, Williams A. Variations in population health status: results from a United Kingdom national questionnaire survey. BMJ 1998;316:736-41. http://dx.doi.org/10.1136/bmj.316.7133.736.
- Campbell HE, Epstein D, Bloomfield D, Griffin S, Manca A, Yarnold J, et al. The cost-effectiveness of adjuvant chemotherapy for early breast cancer: a comparison of no chemotherapy and first, second, and third generation regimens for patients with differing prognoses. Eur J Cancer 2011;47:2517-30. http://dx.doi.org/10.1016/j.ejca.2011.06.019.
- Kirsch J, McGuire A. Establishing health state valuations for disease specific states: an example from heart disease. Health Econ 2000;9:149-58. http://dx.doi.org/10.1002/(SICI)1099-1050(200003)9:2<149::AID-HEC501>3.0.CO;2-N.
- Briggs AH. Handling uncertainty in cost-effectiveness models. Pharmacoeconomics 2000;17:479-500. http://dx.doi.org/10.2165/00019053-200017050-00006.
- Briggs AH, Claxton K, Sculpher MJ. Decision Modelling for Health Economic Evaluation. Oxford: Oxford University Press; 2006.
- Briggs A, Sculpher M, Dawson J, Fitzpatrick R, Murray D, Malchau H. The use of probabilistic decision models in technology assessment. Appl Health Econ Health Policy 2004;3:79-8. http://dx.doi.org/10.2165/00148365-200403020-00004.
- Strong M, Oakley JE, Brennan A. Estimating multiparameter partial expected value of perfect information from a probabilistic sensitivity analysis sample: a nonparametric regression approach. Med Decis Making 2014;34:311-26. http://dx.doi.org/10.1177/0272989X13505910.
- Lohrisch C, Paltiel C, Gelmon K, Speers C, Taylor S, Barnett J, et al. Impact on survival of time from definitive surgery to initiation of adjuvant chemotherapy for early-stage breast cancer. J Clin Oncol 2006;24:4888-94. http://dx.doi.org/10.1200/JCO.2005.01.6089.
- Donovan JL, Paramasivan S, de Salis I, Toerien M. Clear obstacles and hidden challenges: understanding recruiter perspectives in six pragmatic randomised controlled trials. Trials 2014;15. http://dx.doi.org/10.1186/1745-6215-15-5.
- Paramasivan S, Huddart R, Hall E, Lewis R, Birtle A, Donovan JL. Key issues in recruitment to randomised controlled trials with very different interventions: a qualitative investigation of recruitment to the SPARE trial (CRUK/07/011). Trials 2011;12. http://dx.doi.org/10.1186/1745-6215-12-78.
- Donovan J, Mills N, Smith M, Brindle L, Jacoby A, Peters T, et al. Quality improvement report: improving design and conduct of randomised trials by embedding them in qualitative research: ProtecT (prostate testing for cancer and treatment) study. Commentary: presenting unbiased information to patients can be difficult. BMJ 2002;325:766-70. http://dx.doi.org/10.1136/bmj.325.7367.766.
- de Salis I, Tomlin Z, Toerien M, Donovan J. Qualitative research to improve RCT recruitment: issues arising in establishing research collaborations. Contemp Clin Trials 2008;29:663-70. http://dx.doi.org/10.1016/j.cct.2008.03.003.
- Mills EJ, Seely D, Rachlis B, Griffith L, Wu P, Wilson K, et al. Barriers to participation in clinical trials of cancer: a meta-analysis and systematic review of patient-reported factors. Lancet Oncol 2006;7:141-8. http://dx.doi.org/10.1016/S1470-2045(06)70576-9.
- Bogaerts J, Cardoso F, Buyse M, Braga S, Loi S, Harrison JA, et al. Gene signature evaluation as a prognostic tool: challenges in the design of the MINDACT trial. Nat Clin Pract Oncol 2006;3:540-51. http://dx.doi.org/10.1038/ncponc0591.
- Rutgers E, Piccart-Gebhart MJ, Bogaerts J, Delaloge S, Veer LV, Rubio IT, et al. The EORTC 10041/BIG 03–04 MINDACT trial is feasible: results of the pilot phase. Eur J Cancer 2011;47:2742-9. http://dx.doi.org/10.1016/j.ejca.2011.09.016.
- Ramsey SD, Barlow WE, Gonzalez-Angulo AM, Tunis S, Baker L, Crowley J, et al. Integrating comparative effectiveness design elements and endpoints into a phase III, randomized clinical trial (SWOG S1007) evaluating oncotypeDX-guided management for women with breast cancer involving lymph nodes. Contemp Clin Trials 2013;34:1-9. http://dx.doi.org/10.1016/j.cct.2012.09.003.
- Elston CW, Ellis IO. Pathological prognostic factors in breast cancer. I. The value of histological grade in breast cancer: experience from a large study with long-term follow-up. Histopathology 1991;19:403-10. http://dx.doi.org/10.1111/j.1365-2559.1991.tb00229.x.
- Blamey RW, Hornmark-Stenstam B, Ball G, Blichert-Toft M, Cataliotti L, Fourquet A, et al. ONCOPOOL – a European database for 16,944 cases of breast cancer. Eur J Cancer 2010;46:56-71. http://dx.doi.org/10.1016/j.ejca.2009.09.009.
- Walker RA, Bartlett JM, Dowsett M, Ellis IO, Hanby AM, Jasani B, et al. HER2 testing in the UK: further update to recommendations. J Clin Pathol 2008;61:18-24. http://dx.doi.org/10.1136/jcp.2007.054866.
- McCullough AE, Dell’Orto P, Reinholz MM, Gelber RD, Dueck AC, Russo L, et al. Central pathology laboratory review of HER2 and ER in early breast cancer: an ALTTO trial [BIG 2–06/NCCTG N063D (Alliance)] ring study. Breast Cancer Res Treat 2014;143:85-92. http://dx.doi.org/10.1007/s10549-013-2827-0.
- Bueno-de-Mesquita J, Nuyten D, Wesseling J, van Tinteren H, Linn S, van De Vijver M. The impact of inter-observer variation in pathological assessment of node-negative breast cancer on clinical risk assessment and patient selection for adjuvant systemic treatment. Ann Oncol 2010;21:40-7. http://dx.doi.org/10.1093/annonc/mdp273.
- Bartlett JM, Starczynski J, Atkey N, Kay E, O’Grady A, Gandy M, et al. HER2 testing in the UK: recommendations for breast and gastric in-situ hybridisation methods. J Clin Pathol 2011;64:649-53. http://dx.doi.org/10.1136/jcp.2011.089847.
- Perez EA, Press MF, Dueck AC, Jenkins RB, Kim C, Chen B, et al. Immunohistochemistry and fluorescence in situ hybridization assessment of HER2 in clinical trials of adjuvant therapy for breast cancer (NCCTG N9831, BCIRG 006, and BCIRG 005). Breast Cancer Res Treat 2013;138:99-108. http://dx.doi.org/10.1007/s10549-013-2444-y.
- McCabe C, Claxton K, Culyer AJ. The NICE cost-effectiveness threshold. Pharmacoeconomics 2008;26:733-44. http://dx.doi.org/10.2165/00019053-200826090-00004.
- Scottish Intercollegiate Guidelines Network . Scottish Intercollegiate Guidelines Network n.d. www.sign.ac.uk/index.html (accessed 18 December 2015).
- Shabaruddin FH, Chen L-C, Elliott RA, Payne K. A systematic review of utility values for chemotherapy-related adverse events. Pharmacoeconomics 2013;31:277-88. http://dx.doi.org/10.1007/s40273-013-0033-x.
- Moro-Valdezate D, Peiró S, Buch-Villa E, Caballero-Gárate A, Morales-Monsalve MD, Martínez-Agulló Á, et al. Evolution of health-related quality of life in breast cancer patients during the first year of follow-up. J Breast Cancer 2013;16:104-11. http://dx.doi.org/10.4048/jbc.2013.16.1.104.
- Shih V, Chan A, Xie F, Ko Y. Health state utility assessment for breast cancer. Val Health Reg Issues 2012;1:93-7. http://dx.doi.org/10.1016/j.vhri.2012.03.009.
- Matalqah LM, Radaideh KM, Yusoff ZM, Awaisu A. Health-related quality of life using EQ-5D among breast cancer survivors in comparison with age-matched peers from the general population in the state of Penang, Malaysia. J Public Health 2011;19:475-80. http://dx.doi.org/10.1007/s10389-011-0406-6.
- Farkkila N, Roine R, Jahkola T, Sintonen H, Hanninen J, Taari K, et al. Health state utilities in breast cancer. Value Health 2011;14. http://dx.doi.org/10.1016/j.jval.2011.08.1238.
- Delgado-Sanz MC, García-Mendizábal MJ, Pollán M, Forjaz MJ, López-Abente G, Aragonés N, et al. Heath-related quality of life in Spanish breast cancer patients: a systematic review. Health Qual Life Outcomes 2011;9. http://dx.doi.org/10.1186/1477-7525-9-3.
- Anhoury P, DiBonaventura M, Wagner R. Health-related quality of life among women with a history of breast cancer in Europe. J Clin Oncol 2011;29.
- Prescott R, Kunkler I, Williams L, King C, Jack W, van der Pol M, et al. A randomised controlled trial of postoperative radiotherapy following breast-conserving surgery in a minimum-risk older population: the PRIME trial. Health Technol Assess 2007;11. http://dx.doi.org/10.3310/hta11310.
- Kind P, Hardman G, Macran S. UK Population Norms for EQ-5D n.d. www.york.ac.uk/media/che/documents/papers/discussionpapers/CHE_Discussion_Paper_172.pdf (accessed April 2014).
- Hayman JA, Fairclough DL, Harris L, Weeks JC. Patient preferences concerning the trade-off between the risks and benefits of routine radiation therapy after conservative surgery for early-stage breast cancer. J Clin Oncol 1997;15:1252-60.
- Sorensen SV, Brown R, Benedict A, Flood E, Revicki D. QL4 patient-rated utilities in postmenopausal early breast cancer (EBC): a cross-country comparison. Value Health 2004;7:641-2.
- Stalmeier PF. Discrepancies between chained and classic utilities induced by anchoring with occasional adjustments. Med Decis Making 2002;22:53-64.
- Suh WW, Hillner BE, Pierce LJ, Hayman JA. Cost-effectiveness of radiation therapy following conservative surgery for ductal carcinoma in situ of the breast. Int J Radiat Oncol Biol Physics 2005;61:1054-61.
- Chie WC, Huang CS, Chen JH, Chang KJ. Utility assessment for different clinical phases of breast cancer in Taiwan. J Formos Med Assoc 2000;99:677-83.
- Cykert S, Phifer N, Hansen C. Tamoxifen for breast cancer prevention: a framework for clinical decisions. Obstet Gynecol 2004;104:433-42.
- Grann VR, Jacobson JS, Sundararajan V, Albert SM, Troxel AB, Neugut A. The quality of life associated with prophylactic treatments for women with BRAC1/2 mutations. Cancer J Sci Am 1999;5:283-92.
Appendix 1 Methods used for multiparameter assay cost-effectiveness literature review
A literature review was conducted to identify economic evaluations of multiparameter genomic tests within the relevant population. The search was conducted in Ovid MEDLINE(R) In-Process and Other Non-Indexed Citations and Ovid MEDLINE(R), from 1946 to April 2014, using a predefined search filter together with relevant key words related to breast cancer and the different tests [Intercollegiate, S.G.N., 2012197 (InterTASC Information Specialists’ Sub-Group search filters resource – economic evaluations)]. References of identified studies were searched for additional relevant literature. A total of 33 potentially relevant studies were identified in Ovid, of which 29 were discarded for not being full economic evaluations owning to use of an inappropriate population. Full economic evaluations are studies in which a comparison of two or more treatments or care alternatives is undertaken in which both costs and outcomes of the alternatives are examined. This includes cost–benefit analyses, cost–utility analysis and cost-effectiveness analyses. A total of seven studies,74,111–116 published between 2005 and 2013, were identified in the review: four directly from Ovid, and three via additional hand-searching. No head-to-head comparisons of the alternative genomic tests were identified.
Appendix 2 Patient information sheet and consent form
Appendix 3 Consolidated criteria for reporting qualitative research guidelines
The reporting of the qualitative methods was checked against the COREQ (a 32-item checklist for interviews and focus groups). The checklist is shown below; the final column details where evidence can be found that the relevant criterion is satisfied (or information provided justify why the criterion is not satisfied). (Table source: http://intqhc.oxfordjournals.org/content/19/6/349.long141)
Number | Item | Guide questions/description | Cross-reference where included in report/reason for non-inclusion |
---|---|---|---|
Domain 1: research team and reflexivity | |||
Personal characteristics | |||
1. | Interviewer/facilitator | Which author/s conducted the interview or focus group? | Included in Chapter 2, Qualitative recruitment study methods |
2. | Credentials | What were the researcher’s credentials? e.g. PhD, MD | Included in QRS researcher biographies (see Appendix 11) |
3. | Occupation | What was their occupation at the time of the study? | Included in QRS researcher biographies (see Appendix 11) |
4. | Gender | Was the researcher male or female? | Included in QRS researcher biographies (see Appendix 11) |
5. | Experience and training | What experience or training did the researcher have? | Included in QRS researcher biographies (see Appendix 11) |
Relationship with participants | |||
6. | Relationship established | Was a relationship established prior to study commencement? | Included in Appendix 12 |
7. | Participant knowledge of the interviewer | What did the participants know about the researcher? e.g. personal goals, reasons for doing the research | Included in Appendix 12 |
8. | Interviewer characteristics | What characteristics were reported about the interviewer/facilitator? e.g. bias, assumptions, reasons and interests in the research topic | Included in Appendix 12 |
Domain 2: study design | |||
Theoretical framework | |||
9. | Methodological orientation and theory | What methodological orientation was stated to underpin the study? e.g. grounded theory, discourse analysis, ethnography, phenomenology, content analysis | Included in Chapter 2, Qualitative recruitment study methods |
Participant selection | |||
10. | Sampling | How were participants selected? e.g. purposive, convenience, consecutive, snowball | Included in Chapter 2, Qualitative recruitment study methods |
11. | Method of approach | How were participants approached? e.g. face to face, telephone, mail, e-mail | Included in Chapter 2, Qualitative research guidelines methods |
12. | Sample size | How many participants were in the study? | Included in Chapter 2, Qualitative recruitment study methods and Chapter 4, qualitative recruitment study results |
13. | Non-participation | How many people refused to participate or dropped out? Reasons? | Included in Chapter 2, Qualitative recruitment study methods |
Setting | |||
14. | Setting of data collection | Where was the data collected? e.g. home, clinic, workplace | Included in Chapter 2, Qualitative recruitment study methods |
15. | Presence of non-participants | Was anyone else present besides the participants and researchers? | No other individuals were present in interviews |
16. | Description of sample | What are the important characteristics of the sample? e.g. demographic data, date | Included in Chapter 2, Qualitative recruitment study methods |
Data collection | |||
17. | Interview guide | Were questions, prompts, guides provided by the authors? Was it pilot tested? | Included in QRS methods and the topic guide included in Appendix 4 |
18. | Repeat interviews | Were repeat interviews carried out? If yes, how many? | Repeat interviews were not carried out in this study, and have therefore not been reported |
19. | Audio/visual recording | Did the research use audio or visual recording to collect the data? | Included in Chapter 2, Qualitative recruitment study methods |
20. | Field notes | Were field notes made during and/or after the interview or focus group? | Included in Chapter 2, Qualitative recruitment study methods |
21. | Duration | What was the duration of the interviews or focus group? | Included in Chapter 2, Qualitative recruitment study methods |
22. | Data saturation | Was data saturation discussed? | Included in Chapter 2, Qualitative recruitment study methods |
23. | Transcripts returned | Were transcripts returned to participants for comment and/or correction? | Transcripts were not returned to participants in this study |
Domain 3: analysis and findings | |||
Data analysis | |||
24. | Number of data coders | How many data coders coded the data? | Included in Chapter 2, Qualitative recruitment study methods |
25. | Description of the coding tree | Did authors provide a description of the coding tree? | Included in Chapter 2, Qualitative recruitment study methods (analysis) |
26. | Derivation of themes | Were themes identified in advance or derived from the data? | Included in Chapter 2, Qualitative recruitment study methods (analysis) |
27. | Software | What software, if applicable, was used to manage the data? | Included in Chapter 2, Qualitative recruitment study methods (analysis) |
28. | Participant checking | Did participants provide feedback on the findings? | Included in QRS findings (i.e. description of feedback meetings with research participants) |
Reporting | |||
29. | Quotations presented | Were participant quotations presented to illustrate the themes/findings? Was each quotation identified? e.g. participant number | Yes – demonstrated throughout QRS findings |
30. | Data and findings consistent | Was there consistency between the data presented and the findings? | Yes – demonstrated throughout QRS Findings |
31. | Clarity of major themes | Were major themes clearly presented in the findings? | Yes – demonstrated throughout QRS findings. Themes rather than descriptive detail have been included, with some explanation of ‘one-off’ cases where relevant |
32. | Clarity of minor themes | Is there a description of diverse cases or discussion of minor themes? | Yes – demonstrated throughout QRS findings. Themes rather than descriptive detail have been included, with some explanation of ‘one-off’ cases where relevant |
Appendix 4 Qualitative research study interview schedule (final version used)
Appendix 5 Qualitative research study interview schedules (original version)
Appendix 6 Additional details of the multiparameter tests
Oncotype DX
Provides risk classification based on risk of distant recurrence at 10 years:
-
< 10% risk – low, RS < 18
-
10–20% risk – intermediate, RS 18–31
-
> 20% risk – high, RS > 31.
The test was developed in a node-negative population of both pre- and post-menopausal women treated with tamoxifen for 5 years.
IHC4 conventional staining
Provides risk classification based on risk of distant recurrence at 10 years. The risk groups were chosen for consistency with other tests. The IHC4 values that define the cut-off points were derived from an unpublished analysis of the data presented in Cuzick et al. 56 (I Sestak, J Cuzick, Queen Mary University of London, 12 May 2014, personal communication).
-
< 10% risk – low
-
10–20% risk – low/intermediate
-
> 20% risk – high.
The test was developed in a population of post-menopausal women treated with tamoxifen or AI for 5 years.
MammaPrint
Provides a high- or low-risk classification based on risk of distant recurrence at 5 years.
The test was developed in a population of both pre- and post-menopausal women with either ER-positive or ER-negative disease who did not receive any systemic adjuvant therapy.
BluePrint
Provides subtyping classification of either luminal, basal or HER2 type. Luminal tumours may be divided into luminal A and luminal B according to MammaPrint risk classification (low vs. high).
Prosigna
The ROR_PT or Prosigna score is provided on a scale of 0 to 100, which is correlated with the probability of distant recurrence at 10 years for post-menopausal women with hormone receptor-positive, early-stage breast cancer. The score is calculated using coefficients from a Cox model that includes the Pearson correlation to a 46-gene subset of the 50 genes used to calculate each intrinsic subtype, a proliferation score and tumour size. Predefined risk categories based on cut-off points for ROR determined in a clinical validation study are provided in Table 39. The test was developed in a population of post-menopausal women treated with tamoxifen or AI for 5 years.
Risk category | Criteria | Node negative | One positive node | Two positive nodes | Three positive nodes | Four or more positive nodes |
---|---|---|---|---|---|---|
Low | < 10% risk of DR | 0–40 | 0–35 | 0–25 | NA | NA |
Intermediate | 10–20% risk of DR | 41– 60 | 36–55 | 26–45 | 0–25 | NA |
High | > 20% risk of DR | 61–100 | 56–100 | 46–100 | 26–100 | 0–100 |
NanoString provide the following information for each sample, where ROR is the risk of recurrence, P indicates proliferation weighting, T incorporates tumour size and N represents the nodal status:
-
ROR_PT: cleared algorithmic result of Prosigna available through appropriate use of tumour size
-
ROR_P: approximated by setting tumour size to ≤ 2 cm for all samples
-
ROR_PTN: defined risk categories using nodal status information to define cut-off points – cleared result of Prosigna available through appropriate use of tumour size and nodal status (low, intermediate, high)
-
ROR_PN: defined risk categories using nodal status information to define cut-off points
-
ROR_P: defined risk categories using node-negative cut-off points
-
ROR_PT: defined risk categories using node-negative cut-off points
-
intrinsic subtypes: luminal A, luminal B, HER2 enriched, basal-like.
MammaTyper
Provides subtyping classification (luminal A, luminal B, HER2-positive, triple-negative breast cancer).
In addition, luminal B tumours are further classified into:
-
low-risk luminal B tumours if Ki67 < 36
-
high-risk luminal B tumours if Ki67 ≥ 36.
References
Cuzick J, Dowsett M, Pineda S, Wale C, Salter J, Quinn E, et al. Prognostic value of a combined estrogen receptor, progesterone receptor, Ki-67, and human epidermal growth factor receptor 2 immunohistochemical score and comparison with the Genomic Health recurrence score in early breast cancer. J Clin Oncol 2011;29:4273–8. 56
Appendix 7 Health utility literature review
Aim
An extensive literature review was carried out to identify the relevant health-utility values for the OPTIMA study. This search was aimed to update the search performed for Health-state utility values in breast cancer by Peasgood et al. in 2010172 as the information provided in this paper was used primarily as a source of information for health states. The main focus was to update health utility information for breast cancer patients in the following stages:
-
disease free (no chemotherapy) (DFnC)
-
disease free (after chemotherapy) (DFC)
-
local recurrence (LR)
-
distant recurrence (DR).
Methodology
To achieve the aim, the following sources were used.
The Cochrane Library databases (Wiley)
Date range searched: 1992 to July 2013.
Date of search: July 2013.
EconLit (EBSCOhost)
Date range searched: 1886 to July 2013.
Date of search: July 2013.
Cost-Effectiveness Analysis Registry (Tufts)
Date range searched: 2009 to present.
Date of search: July 2013.
EMBASE (Ovid)
Date range searched: 1947 to 10 July 2013.
Date of search: July 2013.
Google Scholar
Date range searched: 2009 to present (July 2013).
Date of search: July 2013.
MEDLINE (Ovid)
Date range searched: 1946 to July 2013.
Date of search: July 2013.
MEDLINE In Process & Other Non-Indexed Citations (Ovid)
Date range searched: inception to 19 July 2013.
Date of search: July 2013.
Web of Science (Science Citation Index Expanded, Conference Proceedings Citation Index)
Date range searched: inception to July 2013.
Date of search: July 2013.
The search strategy main concepts were breast cancer and health utilities. A series of search filters were constructed for each of the electronic databases used in the search. (Full details on this search can be found in Search strategy.) An initial search was run in December 2012, and later updated in July 2013. After removing the duplicates, a first selection was made based on published title. Articles not making any reference to health utility and breast cancer were eliminated. A second sift based on the published abstract and a set of predefined of inclusion and exclusion criteria was performed. Table 40 shows the inclusion and exclusion criteria followed.
Inclusion criteria | Exclusion criteria |
---|---|
Studies whose main objective was to estimate the quality of life of breast cancer patients either by direct estimation or via a systematic review of the literature | Studies where estimating the quality of life of breast cancer patients was not their primary objective |
Studies focused on stages not related to those of interest: DFnC, DFC, LR, DR | |
Studies referring to concomitant conditions or treatments (except chemotherapy) that might affect the estimation of the quality of life of the studied population |
After the second sift, a more detailed third selection was made by reviewing both the published abstract and the full text. Only those articles following both the inclusion and the exclusion criteria were selected. Articles in which the estimation of the quality of life was based on clinical trials, systematic reviews or via recollection of patient information through questioners were considered. These were subject to a more thorough review. Only those articles with information that most closely resembled the disease stages of interest were selected and the parameters retrieved.
Results
Figure 20 shows the flow diagram of the article retrieval and selection process. The full search results can be found in Results tables.
FIGURE 20.
Flow diagram of the search and selection process.

The search and selection process identified 10 relevant articles. 172,174,198–205 Table 41 shows the author, year, title, country, quality of life or QALY estimation method and published journal. Relevant data were extracted from each of the relevant articles. Based on the information retrieved, two articles were selected as a potential source of health utility data. 172,174 Table 42 describes the outcome and the reasons for the selection status.
Author and year | Title | Country | Quality of life or QALY estimation | Journal, volume number and page range |
---|---|---|---|---|
Shabaruddin et al., 2013198 | A systematic review of utility values for chemotherapy-related adverse events | NA | Via the identification of published studies that reported utility values for chemotherapy-related ADEs, from: standard gamble, time trade-off and visual analogue scale. The search was performed in MEDLINE (1948–June 2011) and EMBASE (1980–June 2011) | Pharmacoeconomics 31:277–88 |
Moro-Valdezate et al., 2013199 | Evolution of health-related quality of life in breast cancer patients during the first year of follow-up | Spain | Observational, multicenter and prospective study of a cohort of breast cancer patients, assessing HRQOL at 1, 6, and 12 months after surgery using three questionnaires: EuroQol-5D-3L, EORTC QLQ-C30, and EORTC QLQ-BR23 | Journal of Breast Cancer 16:104–11 |
Shih et al., 2012200 | Health State Utility Assessment for Breast Cancer | Singapore | Twenty hypothetical health states and their descriptions were developed on the basis of literature review and oncology expert panel reviews. Health state utilities were obtained from 20 oncology nurses by using the VAS and SG | Value in Health Regional Issues 1:93–7 |
Campbell et al., 2011174 | The cost-effectiveness of adjuvant chemotherapy for early breast cancer: a comparison of no chemotherapy and first, second, and third generation regimens for patients with differing prognoses | UK | Women entering the model were assumed to have HRQoL on average equivalent to women in age-match UK general population. During chemotherapy, the decrements in HRQoL (using the EQ-5D) were estimated for each regimen based on trial data: ABC, NEAT, and TACT. Some estimations were made based on a literature review | European Journal of Cancer 47:2517–30 |
Matalqah et al., 2011201 | Health-related quality of life using EQ-5D among breast cancer survivors in comparison with age-matched peers from the general population in the state of Penang, Malaysia | Malaysia | The EQ-5D instrument was used in face-to-face interviews for valuating and describing the HRQoL | Journal of Public Health 19:475–480 |
Farkkila et al., 2011202 | Health state utilities in breast cancer | Finland | Breast cancer patients assessed their utility values using different quality of life instruments, such as the ED-5D VAS and the cancer-specific QLQ-C30 | Value in Health 14:A459 |
Delgado-Sanz et al., 2011203 | Heath-related quality of life in Spanish breast cancer patients: a systematic review | Spain | Search performed in MEDLINE, PubMed, EMBASE, PsycINFO, Dialnet, and other Spanish and Latin American databases | Health and Quality of Life Outcomes 9:3 |
Anhoury et al., 2011204 | Health-related quality of life among women with a history of breast cancer in Europe | UK, Germany, France, Italy and Spain | Women with a history of breast cancer (BC+ group) were compared with women without a history of breast cancer (BC- group) on HRQoL (using the physical (PCS) and mental component summary (MCS) scores and health state utilities (SF6D) from the SF-12v2 | Journal of Clinical Oncology 29(Suppl.15):e19615 |
Peasgood et al., 2010172 | Health-state utility values in breast cancer | Systematic review of the literature | The systematic review of the literature (performed in 13 databases) included articles that contained at least one original estimate of the utility values using VAS, SG or TTO | Pharmacoeconomics and Outcomes Research 10:553–66 |
Prescott et al., 2007205 | A randomised controlled trial of postoperative radiotherapy following breast-conserving surgery in a minimum-risk older population. The PRIME trial | UK | The PRIME trial objective was to determine whether or not adjuvant breast irradiation significantly changes the QoL of older women with breast cancer treated with breast-conserving surgery and adjuvant endocrine therapy | Health Technology Assessment 11(31) |
Author | Status | Comment |
---|---|---|
Peasgood et al., 2010172 | Selected | Contains information related to the disease free, local recurrence and distant recurrence event |
Campbell et al., 2011174 | Selected | Contains information related to the disease free, local recurrence and distant recurrence event |
Shabaruddin et al., 2013198 | Not selected | Utility estimates mostly based on adverse events or concomitant events related to breast cancer |
Moro-Valdezate et al., 2013199 | Not selected | Utility estimates based on different aspects of live after breast cancer |
Shih et al., 2012200 | Not selected | Contains information about local recurrence, distant recurrence. However, it is based on an Asian population. Some parameters might be of use if other sources of information are not available |
Matalqah et al., 2011201 | Not selected | Information not detailed in terms of disease free, local recurrence or distant recurrence |
Farkkila et al., 2011202 | Not selected | Some of the descried health states might be useful if no other sources of information are available such as after remission, metastatic disease and terminal care |
Delgado-Sanz et al., 2011203 | Not selected | Not enough information to populate the intended health states |
Anhoury et al., 2011204 | Not selected | Only one utility parameter was reported: disease-free patients |
Prescott et al., 2007205 | Not selected | Only radiotherapy vs. no radiotherapy QALY parameters were estimated |
The two selected articles were chosen because of the type of information provided. 172,174 Campbell et al. 174 was selected as the primary source of information as its data came from clinical trials performed in the UK and it was published more recently than Peasgood et al. 172 The values reported are shown as decrements of a baseline HRQoL quality of life based estimates on the EQ-5D outcome measure (Table 43). A detailed description of each of the 10 selected articles can be found in Tables 47–55.
Search strategy
Health state utilities for breast cancer: update on previous search done at the School of Health and Related Research in 2009
The Cochrane Library: Cochrane Central Register of Controlled Trials, Database of Abstracts of Reviews of Effects and NHS Economic Evaluation Database
Search strategy
#1 MeSH descriptor: [Breast Neoplasms] explode all trees
#2 MeSH descriptor: [Neoplasms] explode all trees
#3 MeSH descriptor: [Carcinoma] explode all trees
#4 MeSH descriptor: [Adenocarcinoma] explode all trees
#5 MeSH descriptor: [Breast] explode all trees
#6 #2 or #3 or #4
#7 #5 and #6
#8 (carcinoma near/3 breast*):ti,ab,kw
#9 (neoplas* near/3 breast*):ti,ab,kw
#10 (adenocarcinoma near/3 breast*):ti,ab,kw
#11 (cancer* near/3 breast*):ti,ab,kw
#12 (tumour* near/3 breast*):ti,ab,kw
#13 (tumor* near/3 breast*):ti,ab,kw
#14 (malignan* near/3 breast*):ti,ab,kw
#15 #8 or #9 or #10 or #11 or #12 or #13 or #14
#16 #1 or #7 or #15
#17 (HALex):ti,ab,kw
#18 (euroqol or “euro qol*” or eq5d or “eq 5d*”):ti,ab,kw
#19 qaly*:ti,ab,kw
#20 “quality adjusted life year*”:ti,ab,kw
#21 hye*:ti,ab,kw
#22 “health* year* equivalent*”:ti,ab,kw
#23 “health utilit*”:ti,ab,kw
#24 “hui”:ti,ab,kw
#25 “quality of well being”:ti,ab,kw
#26 “quality of wellbeing”:ti,ab,kw
#27 “qwb”:ti,ab,kw
#28 (qald* or qale* or qtimes*):ti,ab,kw
#29 (“quality adjusted life day*” or “quality adjusted life expectanc*” or “quality adjusted survival*”):ti,ab,kw
#30 MeSH descriptor: [Quality-Adjusted Life Years] explode all trees
#31 “standard gamble*”:ti,ab,kw
#32 “time trade off”:ti,ab,kw
#33 “time tradeoff”:ti,ab,kw
#34 “tto”:ti,ab,kw
#35 “visual analog* scale*”:ti,ab,kw
#36 “discrete choice experiment*”:ti,ab,kw
#37 (sf6 or “sf 6” or “short form 6” or “shortform 6” or “sf six” or “sfsix” or “short form six”):ti,ab,kw
#38 (sf12 or “sf 12” or “short form 12” or “shortform 12” or “sf twelve” or sftwelve or “short form twelve”):ti,ab,kw
#39 (sf6d or “sf 6d” or “short form 6d” or “shortform 6d” or “sf 6 d”):ti,ab,kw
#40 “health state* utilit*”:ti,ab,kw
#41 “health state* value*”:ti,ab,kw
#42 “health state* preference*”:ti,ab,kw
#43 #17 or #18 or #19 or #20 or #21 or #22 or #23 or #24 or #25 or #26 or #27 or #28 or #29 or #30 or #31 or #32 or #33 or #34 or #35 or #36 or #37 or #38 or #39 or #40 or #41 or #42
#44 #16 and #43 from 2009 to 2012 (Word variations have been searched)
Cost-Effectiveness Analysis Registry
Only simple search allowed: used Breast Cancer – c. 50 hits since 2009, test showed hits already found via other searches.
EconLit: via EBSCOhost
Search strategy
S1 AB ( cancer* or neoplasm* or carcinoma* or adenocarcinoma* or malignan* or tumour* or tumor*) OR TI ( cancer* or neoplasm* or carcinoma* or adenocarcinoma* or malignan* or tumour* or tumor* )
S2 AB ( breast or breasts ) OR TI ( breast or breasts )
S3 TI (HALex or euroqol or “euro qol” or eq5d or “eq 5d”) OR AB ( HALex or euroqol or “euro qol” or eq5d or “eq 5d”)
S4 TI (qaly* or “quality adjusted life year*”) OR AB ( qaly* or “quality adjusted life year*”)
S5 TI ( hye* or “health* year* equivalent*”) OR AB ( hye* or “health* year* equivalent*”)
S6 TI (health utilit* or hui) OR AB (health utilit* or hui)
S7 TI ( “quality of well being” or “quality of wellbeing” or qwb ) OR AB ( “quality of well being” or “quality of wellbeing” or qwb )
S8 AB ( “Standard gamble*” or “time trade off” or “time tradeoff” or tto or “visual analog* scale*” or “discrete choice experiment*”) OR TI ( “Standard gamble*” or “time trade off” or “time tradeoff” or tto or “visual analog* scale*” or “discrete choice experiment*”)
S9 AB ( sf6 or “sf 6” or “short form 6” or “shortform 6” or “sf six” or sfsix or “short form six” or “shortform six”) OR TI ( sf6 or “sf 6” or “short form 6” or “shortform 6” or “sf six” or sfsix or “short form six” or “shortform six”)
S10 AB ( sf12 or “sf 12” or “short form 12” or “shortform 12” or “sf twelve” or sftwelve or “short form twelve” or “shortform twelve”) OR TI ( sf12 or “sf 12” or “short form 12” or “shortform 12” or “sf twelve” or sftwelve or “short form twelve” or “shortform twelve”)
S11 AB ( sf6d or “sf 6d” or “short form 6d” or “shortform 6d” or “sf 6 d”) OR TI ( sf6d or “sf 6d” or “short form 6d” or “shortform 6d” or “sf 6 d”)
S12 AB “health state*” OR TI “health state*"
S13 AB ( “health state* utilit*” or “health state* value*” or “health state* preference*”) OR TI ( “health state* utilit*” or “health state* value*” or “health state* preference*”)
S14 S8 OR S9 OR S10 OR S11 OR S12 OR S13
S15 S3 OR S4 OR S5
S16 S6 OR S7
S17 S14 OR S15 OR S16
S18 S1 AND S2 AND S17
EMBASE Classic and EMBASE
Date range searched: 1947 to 10 December 2012.
Search strategy
-
exp breast tumor/ (332,292)
-
exp neoplasm/ (3,374,500)
-
exp breast/ (92,709)
-
2 and 3 (57,167)
-
1 or 4 (344,576)
-
(carcinoma adj3 breast$).tw. (34,630)
-
(neoplas$ adj3 breast$).tw. (2667)
-
(adenocarcinoma adj3 breast$).tw. (2312)
-
(cancer$ adj3 breast$).tw. (220,550)
-
(tumour$ adj3 breast$).tw. (5856)
-
(tumor$ adj3 breast$).tw. (23,736)
-
(malignan$ adj3 breast$).tw. (7722)
-
or/5-12 (372,624)
-
HALex.mp. [mp=title, abstract, subject headings, heading word, drug trade name, original title, device manufacturer, drug manufacturer, device trade name, word] (27)
-
(euroqol or euro qol or eq5d or eq 5d).tw. (5077)
-
qaly$.tw. (6910)
-
quality adjusted life year$.tw. (6789)
-
hye$.tw. (1031)
-
health$ year$ equivalent$.tw. (41)
-
health utilit$.tw. (1315)
-
hui.tw. (799)
-
quality of well being.tw. (344)
-
quality of wellbeing.tw. (18)
-
qwb.tw. (174)
-
(qald$ or qale$ or qtimes$).tw. (140)
-
(quality adjusted life day$ or quality adjusted life expectanc$ or quality adjusted survival$).tw. (937)
-
exp quality adjusted life year/ or short form 12/ (10,976)
-
standard gamble$.tw. (726)
-
time trade off.tw. (863)
-
time tradeoff.tw. (213)
-
tto.tw. (765)
-
visual analog$ scale$.tw. (32,527)
-
discrete choice experiment$.tw. (443)
-
(sf6 or sf 6 or short form 6 or shortform 6 or sf six or sfsix or short form six).tw. (1457)
-
(sf12 or sf 12 or short form 12 or shortform 12 or sf twelve or sftwelve or short form twelve).mp. [mp=title, abstract, subject headings, heading word, drug trade name, original title, device manufacturer, drug manufacturer, device trade name, keyword] (3523)
-
(sf6d or sf 6d or short form 6d or shortform 6d or sf 6 d).tw. (528)
-
health state$ utilit$.tw. (391)
-
health state$ value$.tw. (144)
-
health state$ preference$.tw. (95)
-
or/14-39 (57,626)
-
letter*.pt,ti. (845,685)
-
editorial*.pt,ti. (445,924)
-
comment*.pt,ti. (92,999)
-
or/41-43 (1,358,961)
-
40 and 13 (1249)
-
45 not 44 (1213)
-
(2009* or 2010* or 2011* or 2012*).em. (4,696,303)
-
46 and 47 (675)
Google Scholar (advanced screen)
At least one of the following: HUI OR TTO OR SG OR EQ-5D OR SF-6D OR SF-12 OR VAS OR QWB OR HALex OR Euroqol
Exact phrases “breast cancer” AND “health state utilities”
Limits Material since 2009 and in subject areas: Medicine, Pharmacology, and Veterinary Science OR Social Sciences, Arts, and Humanities
Note: special interface used: http://scholar.google.co.uk/advanced_scholar_search?hl=en&as_sdt=0,5
Ovid MEDLINE(R)
Date range searched: 1946 to week 3, November 2012.
Search strategy
-
exp breast neoplasms/ (202,474)
-
exp neoplasms/ (2,414,772)
-
exp carcinoma/ (457,980)
-
exp adenocarcinoma/ (265,518)
-
exp breast/ (30,049)
-
or/2-4 (2,414,772)
-
5 and 6 (16472)
-
(carcinoma adj3 breast$).tw. (25,132)
-
(neoplas$ adj3 breast$).tw. (2066)
-
(adenocarcinoma adj3 breast$).tw. (1673)
-
(cancer$ adj3 breast$).tw. (158,629)
-
(tumour$ adj3 breast$).tw. (3909)
-
(tumor$ adj3 breast$).tw. (17,709)
-
(malignan$ adj3 breast$).tw. (5633)
-
or/8-14 (184,068)
-
1 or 7 or 15 (238,389)
-
HALex.mp. [mp=title, abstract, original title, name of substance word, subject heading word, protocol supplementary concept, rare disease supplementary concept, unique identifier] (14)
-
(euroqol or euro qol or eq5d or eq 5d).tw. (2952)
-
qaly$.tw. (4186)
-
quality adjusted life year$.tw. (4825)
-
hye$.tw. (587)
-
health$ year$ equivalent$.tw. (36)
-
health utilit$.tw. (873)
-
hui.tw. (556)
-
quality of well being.tw. (307)
-
quality of wellbeing.tw. (5)
-
qwb.tw. (154)
-
(qald$ or qale$ or qtimes$).tw. (91)
-
(quality adjusted life day$ or quality adjusted life expectanc$ or quality adjusted survival$).tw. (707)
-
quality-adjusted life years/ (6160)
-
standard gamble$.tw. (595)
-
time trade off.tw. (640)
-
34 tto.tw. (490)
-
visual analog$ scale$.tw. (22,860)
-
discrete choice experiment$.tw. (284)
-
(sf6 or sf 6 or short form 6 or shortform 6 or sf six or sfsix or short form six).tw. (924)
-
time tradeoff.tw. (192)
-
(sf12 or sf 12 or short form 12 or shortform 12 or sf twelve or sftwelve or short form twelve).mp. [mp=title, abstract, original title, name of substance word, subject heading word, protocol supplementary concept, rare disease supplementary concept, unique identifier] (2085)
-
(sf6d or sf 6d or short form 6d or shortform 6d or sf 6 d).tw. (318)
-
health state$ utilit$.tw. (215)
-
health state$ value$.tw. (105)
-
health state$ preference$.tw. (72)
-
or/17-42 (38,587)
-
letter.pt. (766,872)
-
editorial.pt. (310,993)
-
comment.pt. (493,546)
-
or/44-46 (1,166,749)
-
43 not 47 (37,884)
-
16 and 48 (660)
-
(200903* or 200904* or 200905* or 200906* or 200907*or 200908* or 200909* or 200910* or 200911* or 200912* or 2010* or 2011* or 2012*).ed. (2,851,442)
-
49 and 50 (230)
Ovid MEDLINE(R) In-Process and Other Non-Indexed Citations
Date searched: 6 December 2012.
Same as OVID MEDLINE. Eleven results.
Web of Science
Search strategy
# 1 1737 Topic=(HALex or euroqol or “euro qol” or eq5d or “eq 5d”) OR Title=(HALex or euroqol or “euro qol” or eq5d or “eq 5d”)
Databases = SCI-EXPANDED, SSCI, CPCI-S Timespan = 1 January 2009 – 18 December 2012
Lemmatization = Off
# 2 2304 Topic=(qaly* or “quality adjusted life year*”) OR Title=(qaly* or “quality adjusted life year*”)
Databases = SCI-EXPANDED, SSCI, CPCI-S Timespan = 1 January 2009 – 18 December 2012
Lemmatization = Off
# 3 893 Topic=(hye* or “health* year* equivalent*”) OR Title=(hye* or “health* year* equivalent*”)
Databases = SCI-EXPANDED, SSCI, CPCI-S Timespan = 1 January 2009 – 18 December 2012
Lemmatization = Off
# 4 5853 Topic=(health utilit* or hui) OR Title=(health utilit* or hui)
Databases = SCI-EXPANDED, SSCI, CPCI-S Timespan = 1 January 2009 – 18 December 2012
Lemmatization = Off
# 5 57 Topic=(“quality of well being” or “quality of wellbeing” or qwb) OR Title=(“quality of well being” or “quality of wellbeing” or qwb)
Databases = SCI-EXPANDED, SSCI, CPCI-S Timespan = 1 January 2009 – 18 December 2012
Lemmatization = Off
# 6 8435 Topic=(“Standard gamble*” or “time trade off” or “time tradeoff” or tto or “visual analog* scale*” or “discrete choice experiment*”) OR Title=(“Standard gamble*” or “time trade off” or “time tradeoff” or tto or “visual analog* scale*” or “discrete choice experiment*”)
Databases = SCI-EXPANDED, SSCI, CPCI-S Timespan = 1 January 2009 – 18 December 2012
Lemmatization = Off
# 7 1388 Topic=((sf6 or “sf 6” or “short form 6” or “shortform 6” or “sf six” or sfsix or “short form six” or “shortform six”)) OR Title=((sf6 or “sf 6” or “short form 6” or “shortform 6” or “sf six” or sfsix or “short form six” or “shortform six”))
Databases = SCI-EXPANDED, SSCI, CPCI-S Timespan = 1 January 2009 – 18 December 2012
Lemmatization = Off
# 8 1009𠀃Topic=((sf12 or “sf 12” or “short form 12” or “shortform 12” or “sf twelve” or sftwelve or “short form twelve” or “shortform twelve”)) OR Title=((sf12 or “sf 12” or “short form 12” or “shortform 12” or “sf twelve” or sftwelve or “short form twelve” or “shortform twelve”))
Databases = SCI-EXPANDED, SSCI, CPCI-S Timespan = 1 January 2009 – 18 December 2012
Lemmatization = Off
# 9 235 Topic=((sf6d or “sf 6d” or “short form 6d” or “shortform 6d” or “sf 6 d”)) OR Title=((sf6d or “sf 6d” or “short form 6d” or “shortform 6d” or “sf 6 d”))
Databases = SCI-EXPANDED, SSCI, CPCI-S Timespan = 1 January 2009 – 18 December 2012
Lemmatization = Off
# 10 1238 Topic=(“health state*”) OR Title=(“health state*”)
Databases = SCI-EXPANDED, SSCI, CPCI-S Timespan = 1 January 2009 – 18 December 2012
Lemmatization = Off
# 11 19,991 #10 OR #9 OR #8 OR #7 OR #6 OR #5 OR #4 OR #3 OR #2 OR #1
Databases = SCI-EXPANDED, SSCI, CPCI-S Timespan = 1 January 2009 – 18 December 2012
Lemmatization = Off
# 12 40,529 TI=((cancer* or neoplasm* or carcinoma* or adenocarcinoma* or malignan* or tumour* or tumor* or oncol*) AND (breast or breasts or mammary))
Databases = SCI-EXPANDED, SSCI, CPCI-S Timespan = 1 January 2009 – 18 December 2012
Lemmatization = Off
# 13 206 #12 AND #11
Databases = SCI-EXPANDED, SSCI, CPCI-S Timespan = 1 January 2009 – 18 December 2012
Lemmatization = Off
Results tables
Database and dates covered | Date searched | Concept search strategy | Hits | Notes |
---|---|---|---|---|
The Cochrane Library: CENTRAL (Wiley) | 12 December 2012 | Breast cancer + health utilities | 41 | Three databases searched at once on the same interface (Wiley): but results are exported from each individual database |
The Cochrane Library: DARE (Wiley) | 12 December 2012 | Breast cancer + health utilities | 1 | |
The Cochrane Library: NHS Economic Evaluations Database (Wiley) | 12 December 2012 | Breast cancer + health utilities | 59 | |
Cost-Effectiveness Analysis Registry | 19 December 2012 | Breast cancer | 0 | |
EconLit (EBSCOhost) | 18 December 2012 | 13 | ||
EMBASE Classic + EMBASE: searched from 1947 to 10 December 2012 | 12 December 2012 | Breast cancer + health utilities Since 2009 |
675 | |
Google Scholar | 19 December 2012 | 153 | ||
Ovid MEDLINE(R): searched from 1946 to week 3 November 2012 | 11 December 2012 | Breast cancer + health utilities Since April 2009 |
230 | |
Ovid MEDLINE(R) In-Process and Other Non-Indexed Citations: searched 6 December 2012 | 11 December 2012 | Same as above | 11 | |
Web of Science | 18 December 2012 | 206 |
Database and dates covered | Date searched | Concept search strategy | Hits | Notes |
---|---|---|---|---|
The Cochrane Library: CENTRAL (Wiley) | 23 July 2013 | Breast cancer + health utilities | 6 | Limit of 2012 to 2013 applied |
The Cochrane Library: DARE (Wiley) | 23 July 2013 | Breast cancer + health utilities | 0 | |
The Cochrane Library: NHS Economic Evaluations Database (Wiley) | 23 July 2013 | Breast cancer + health utilities | 14 | |
Cost-Effectiveness Analysis Registry | 23 July 2013 | Breast cancer | 0 | All from 2012 already in library |
EconLit – EBSCOhost | 23 July 2013 | Breast cancer + health utilities | 15 | No date limit applied as EBSCOhost interface working only intermittingly |
EMBASE Classic + EMBASE searched from 1947 to 19 July 2013 | 22 July 2013 | Breast cancer + health utilities since 2009 | 143 | Limit 2012 to 2013 applied |
Google Scholar | 23 December 2012 | Breast cancer + health utilities2012–2013 | 25 | About 100 results. Chose those where “health state utilities” and “Breast cancer” were prominent |
Ovid MEDLINE(R): searched from 1946 to week 2 July 2013 | 22 July 2013 | Breast cancer + health utilities since April 2009 | 39 | |
Ovid MEDLINE(R) In-Process and Other Non-Indexed Citations. Date searched 19 July 2013 | 22 July 2013 | Same as above | 39 | |
Web of Science | 23 July 2013 | 78 | Limit 2012 to 2013 applied | |
RePEc | 23 July 2013 | Breast cancer | 3 | Imported via Google Scholar |
Detailed descriptions of the relevant articles
Authors (year) | Title | Country | Population |
---|---|---|---|
Campbell et al., 2011174 | The cost-effectiveness of adjuvant chemotherapy for early breast cancer: a comparison of no chemotherapy and first, second, and third generation regimens for patients with differing prognoses | UK | Women diagnosed with early breast cancer. Women were divided into those under and those over 50 years of age |
QALY estimation method | Women entering the model were assumed to have HRQoL on average equivalent to women in age-matched UK general population. During chemotherapy, the decrements in HRQoL (using the EQ-5D) were estimated for each regimen based on trial data: ABC, NEAT and TACT. Some estimations were made based on a literature review | ||
Relevant QALY measures | Value | Source | |
Baseline HRQoL based on EQ-5D | Age and sex adjusted. UK population norms | (Department of Health, 1998) | |
Decrements in HRQoL from underlying levels (early breast cancer) | |||
Patients not receiving chemotherapy | –0.003 | ABC trial | |
Patients receiving CMF | –0.057 | NEAT, TACT | |
Patients receiving E-CMF/FEC60 | –0.067 | TACT | |
Patients receiving FEC-D | –0.099 | ABC trial, NEAT, TACT | |
Decrements in HRQoL from underlying levels (metastatic breast cancer) | |||
With CMF year 1 | –0.057 | ABC trial | |
With E-CMF/FEC60 year 1 | –0.038 | ABC trial | |
With FEC-D year 1 | –0.035 | NEAT, TACT | |
With local recurrence | –0.108 (SE 0.04) | TACT trial | |
With metastatic recurrence | –0.303 (SE 0.16) | (Hayman et al. 1997;207 Sorensen et al. 2004;208 Stalmeier 2002;209 Suh et al. 2005210) | |
With contralateral/second primary breast tumour | –0.108 (SE 0.04) | (Chie et al. 2000;211 Cykert et al. 2004;212 Grann et al. 1999;213 Stalmeier 2002209) | |
HRQoL final 3 months of life | 0.159 (SE 0.04) | (Sorensen et al. 2004;208 Stalmeier 2002;209 Suh et al. 2005210) | |
Status | Selected |
Authors (year) | Title | Country | Population |
---|---|---|---|
Shabaruddin et al. 2013198 | A systematic review of utility values for chemotherapy-related adverse events | Not country-specific | Articles that assess chemotherapy treatments that value utility of chemotherapy-related AEs. No discrimination on the type of cancer being treated |
QALY estimation method | The review aim was to identify published articles that have reported utility values for chemotherapy based on different instruments such as standard gamble, visual analogue scale and time trade-off | ||
Relevant QALY measures | Value | Source/comments | |
Nausea and/or vomiting | 32 values measuring different degrees of nausea and/or vomiting. Expressed in utility decrements or means in comparison with a base state | Several. Details can be found in the online appendix 3 of the published paper198 | |
Neuropathy | 21 values measuring different degrees/types of neuropathy. Expressed in utility decrements or means in comparison with a base state | ||
Neutropenia | 12 values measuring different degrees/types of neutropenia. Expressed in utility decrements or means in comparison with a base state | ||
Anaemia | 34 values measuring different degrees of anaemia. Expressed in utility decrements or means in comparison with a base state | ||
Alopecia | Seven values measuring different degrees/types of alopecia. Expressed in utility decrements or means in comparison with a base state | ||
Skin reaction | Five values measuring different degrees of skin reactions. Expressed in utility decrements or means in comparison with a base state | ||
Hand–foot syndrome | Five values measuring different degrees of hand–foot syndrome. Expressed in utility decrements or means in comparison with a base state | ||
Fatigue | Eight values measuring different degrees of fatigue. Expressed in utility decrements or means in comparison with a base state | ||
Diarrhoea | 12 values measuring different degrees of diarrhoea. Expressed in utility decrements or means in comparison with a base state | ||
Stomatitis | 10 values measuring different degrees of stomatitis. Expressed in utility decrements or means in comparison with a base state | ||
Secondary cancers | Two utility measures of secondary malignant neoplasms. Expressed in means in comparison with a base state | ||
Pyrexia | Three values measuring different degrees of pyrexia. Expressed in utility decrements or means in comparison with a base state | ||
Cardiovascular disease | Three values measuring different degrees of cardiovascular disease. Expressed in utility decrements or means in comparison with a base state | ||
Ototoxicity | Two values measuring different degrees of ototoxicity disease. Expressed in utility decrements or means in comparison with a base state | ||
Flu-like syndrome | Three values measuring different degrees of flu-like syndrome. Expressed in terms decrements or means in comparison with a base state | ||
Oedema | Two values measuring different degrees of oedema. Expressed in decrements or means in comparison with a base state | ||
Infection-related events | Five utility measures of infection-related events: pneumonia, infection without hospitalisation and sepsis. Expressed in terms of means in comparison with a base state | ||
Myalgia pain | Two values measuring different degrees of myalgia pain. Expressed in means in comparison with a base state | ||
Common adverse events specific to chemotherapy drug | Six utility measures of common AEs: toxicity from paclitaxel, docetaxel, and vinorelbine. Expressed in means utility values | ||
Adverse events leading to hospital stay | Six utility measures of AEs leading to hospitalisation: 1-day inpatient or outpatient stay; hospitalisation from 2 to 5 days. Expressed in utility decrements in comparison with a base state | ||
Non-specific event relating to AEs | 37 utility measures of non-specific AE related to AEs: low, moderate, high or extremely high AE, low, moderate or high treatment efficacy and poor or positive well-being. Expressed in terms of means utility values | ||
Status | Not selected. Potentially useful for some toxicity adverse events |
Authors (year) | Title | Country | Population |
---|---|---|---|
Moro-Valdezate et al., 2013199 | Evolution of health-related quality of life in breast cancer patients during the first year of follow-up | Spain | Total of 364 women after surgery followed for 1 year. Patients on Stage IV were excluded. Participants‘ age ranged from < 50 years to > 70 years, with a mean age of 59 years (most frequent 60–69 years age group). Most of respondents had breast-conserving surgery 61%, radiotherapy 74% and adjuvant chemotherapy 97% |
QALY estimation method | Observational, multicentre and prospective study of a cohort of breast cancer patients, assessing HRQoL at 1, 6 and 12 months after surgery using three questionnaires: EuroQol-5D-3L, EORTC QLQ-C30 and EORTC QLQ-BR23 | ||
Relevant QALY measures | Value | Source/comments | |
EQ-5D-3L | |||
VAS | 1 month: 70; 6 months: 70; 1 year: 80 | 321 respondents | |
Score | 1 month: 0.81; 6 months: 0.83; 1 year: 0.83 | 336 respondents | |
EORTC QLQ-C30 | |||
Global health status | 1 month: 66.67; 6 months: 83.33; 1 year: 100 Specific utilities values were also estimated such as physical, role, emotional, cognitive, social functioning, fatigue, pain, nausea/vomiting, dyspnoea, insomnia, appetite loss, constipation, diarrhoea and financial difficulties. Values can be found in the published article197 |
335 respondents | |
EORTC QLQ-C30 | |||
Body image | 1 month: 83.33; 6 months: 91.67; 1 year: 100 | 316 respondents | |
Sexual functioning | 1 month: 100; 6 months: 86.67; 1 year: 86.67 | 268 respondents | |
Sexual enjoyment | 1 month: 66.67; 6 months: 66.67; 1 year: 66.67 | 116 respondents | |
Future perspective | 1 month: 33.33; 6 months: 66.67; 1 year: 66.67 | 334 respondents | |
Systematic therapy side effects | 1 month: 19.05; 6 months: 14.29; 1 year: 9.52 | 263 respondents | |
Breast symptoms | 1 month: 25.00; 6 months: 16.67; 1 year: 16.67 | 326 respondents | |
Arms symptoms | 1 month: 22.22; 6 months: 11.11; 1 year: 11.11 | 330 respondents | |
Upset by hair loss | 1 month: 33.33; 6 months: 33.33; 1 year: 0.00 | 77 respondents | |
Status | Not selected. Potentially useful for patients in the disease-free stage |
Authors (year) | Title | Country | Population | ||
---|---|---|---|---|---|
Shih et al., 2012200 | Health state utility assessment for breast cancer | Singapore | Men or women over the age of 21 years with at least 2 years’ experience in the oncology department | ||
QALY estimation method | Health states utilities were obtained from using the VAS and SG methods | ||||
Relevant QALY measures | Value | ||||
VAS score, RAWa | VAS score adjusteda | SG score, RAWa | SG score, adjustedb | Source/comments | |
No recurrence with no side effect | 0.870 | 0.860 | 0.678 | 0.673 | Medical personal survey in an oncology department hospital in Singapore |
No recurrence with hip fracture | 0.514 | 0.482 | 0.504 | 0.353 | |
No recurrence with wrist fracture | 0.508 | 0.475 | 0.533 | 0.386 | |
No recurrence with spine fracture | 0.508 | 0.372 | 0.458 | 0.383 | |
No recurrence with vaginal bleeding | 0.725 | 0.704 | 0.554 | 0.473 | |
No recurrence with deep-vein thrombosis | 0.582 | 0.554 | 0.515 | 0.357 | |
No recurrence with pulmonary embolism | 0.368 | 0.329 | 0.463 | 0.395 | |
No recurrence with cataract | 0.547 | 0.519 | 0.519 | 0.355 | |
No recurrence with ischaemic cerebrovascular events | 0.299 | 0.256 | 0.408 | 0.289 | |
No recurrence with common side effects musculoskeletal disorder | 0.653 | 0.629 | 0.510 | 0.371 | |
No recurrence with common side effects hot flushes | 0.749 | 0.730 | 0.588 | 0.520 | |
No recurrence with endometrial cancer | 0.506 | 0.472 | 0.501 | 0.365 | |
New contralateral breast cancer | 0.500 | 0.468 | 0.443 | 0.284 | |
Locoregional recurrence with no side effects | 0.525 | 0.491 | 0.473 | 0.376 | |
Locoregional recurrence with side effects: general | 0.506 | 0.473 | 0.438 | 0.336 | |
Distant recurrence with no side effects | 0.438 | 0.400 | 0.470 | 0.371 | |
Distant recurrence with side effects: chemotherapy | 0.404 | 0.365 | 0.458 | 0.356 | |
Distant recurrence with side effects: endocrine therapy | 0.410 | 0.370 | 0.445 | 0.299 | |
Worse health | 0.033 | –0.032 | 0.364 | –0.160 | |
Current health | 0.944 | 0.941 | 0.916 | 0.973 | |
Status | Probable. Possible problems as values are based on Asian populations |
Authors (year) | Title | Country | Population |
---|---|---|---|
Matalqah et al., 2011201 | Health-related quality of life using EQ-5D among breast cancer survivors in comparison with age-matched peers from the general population in the state of Penang, Malaysia | Malaysia | Women age 20 years or older with confirmed diagnosis of breast cancer compared with a similar group of women without |
QALY estimation method | The EQ-5D instrument in two languages, the English version for Malaysia and the Malay version, was used in face-to-face interviews for valuating and describing the HRQoL | ||
Relevant QALY measures | Value | Source/comments | |
Breast cancer survivors | General population | ||
General EQ–5D | 0.71 ± 0.25 | 0.87 ± 0.16 | |
< 50 years of age | 0.79 ± 0.22 | 0.91 ± 0.13 | |
> 50 years of age | 0.67 ± 0.26 | 0.83 ± 0.17 | |
General EQ VAS | 72.7 ± 16.9 | 74.6 ± 13.2 | |
< 50 years of age | 75.0 | 75.3 | |
> 50 years of age | 71.1 | 74.0 | |
Status | Not selected. Not enough data to populate the model. The values might be used for the disease-free stage |
Authors (year) | Title | Country | Population |
---|---|---|---|
Farkkila et al., 2011202 | Health state utilities in breast cancer | Finland | Patients diagnosed with breast cancer between the ages of 31 and 90 years in a hospital in Helsinki, Finland |
QALY estimation method | A total of 778 BC patients assessed their HRQoL with different quality of life questionnaires. Patients were divided based on disease state | ||
Relevant QALY measures | Value | Source/comments | |
Generic 15D | EQ-5D | ||
Baseline before treatment | 0.896 ± 0.083 | 0.818 ± 0.228 | |
First year remission after diagnosis or recurrence | 0.901 ± 0.80 | 0.860 ± 0.178 | |
Second year or following years after remission | 0.884 ± 0.103 | 0.843 ± 0.189 | |
Metastatic disease | 0.825 ± 0.113 | 0.746 ± 0.251 | |
Terminal care | 0.756 ± 0.110 | 0.514 ± 0.300 | |
Status | Probable. Some health stages might be useful, in particular those after remission, metastatic disease and terminal care |
Authors (year) | Title | Country | Population |
---|---|---|---|
Delgado-Sanz et al., 2011203 | Heath-related quality of life in Spanish breast cancer patients: a systematic review | Spain | Systematic review on existing literature related to health-related quality of life in Spanish population |
QALY estimation method | Authors consulted MEDLINE, PubMed, EMBASE, PsycINFO, Dialnet, and other Spanish and Latin American databases: ‘Índice Bibliográfico Español en ciencias de la Salud’ and ‘Literatura Latinoamericana y del Caribe en ciencias de la Salud’. Two researchers participated in the selection and extraction of the relevant information. Detailed analysis was performed only on those articles reporting Spanish studies | ||
Relevant QALY measures | Value | Source/comments | |
None provided | |||
Status | Not selected. A brief description of the main findings of the selected papers is provided. The authors did not extract utility values from the retrieved articles. It is based only on Spanish population |
Authors (year) | Title | Country | Population |
---|---|---|---|
Anhoury et al., 2011204 | Health-related quality of life among women with a history of breast cancer in Europe | UK, Germany, France, Italy and Spain | Authors used data from the 2010 EU (UK, Germany, France, Italy and Spain) National Health and Wellness Survey (NHWS). Data on 579 women reported history of breast cancer. Most were cancer free (76.9%) |
QALY estimation method | The history of women with and without breast cancer was compared and adjusted using demographic characteristics to estimate their utility values | ||
Relevant QALY measures | Value | Source/observations | |
Breast cancer positive (BC+) | Breast cancer negative (BC–) | ||
Health states utilities | 0.69 | 0.71 | Subsequent analyses of just the BC+ group revealed that the primary predictors of health state utilities included age (b = 0.002), employment (b = 0.43), exercise behaviour (b = 0.04), alcohol use (b = 0.04), comorbidity burden (b = –0.01), years diagnosed (b = 0.002), and current cancer stage (b’s = –0.01 to –0.08) (all p values < .05) |
Status | Not selected. Only one utility parameter reported. This might be useful if no other information on disease-free patients is found |
Authors, year | Title | Country | Population | |||||
---|---|---|---|---|---|---|---|---|
Peasgood et al., 2010172 | Health-state utility values in breast cancer | Systematic review of the literature | Review focused on health states utility for conditions relating to breast cancer | |||||
QALY estimation method | A total of 13 databases were searched for articles in March 2009 using key terms to identify HSUVs related to breast cancer. Articles included contained at least one original, unique utility value. HSUVs were estimated using an existing multiattribute health state descriptive system such as the generic EQ-5D, SF-6D, HUI3 or condition-specific questionnaires. The preferences of patient populations towards their own current health may be measured directly using VAS, SG or TTO. Health utility was divided into six categories: screening-related states; preventive states; adverse events in breast cancer and its treatment; non-specific breast cancer; early breast cancer states; and metastatic breast cancer states. Meta-regression analysis was performed using different models to weight data | |||||||
Relevant QALY measures | Value (p-value) | Source/comments | ||||||
Early breast cancer | Metastatic breast cancer | |||||||
Variables | Model 1 | Model 2 | Model 3 | Variables | Model 1 | Model 2 | Model 3 | |
Surgery (reference: breast-conserving surgery) | Treatment type (reference: chemotherapy) | |||||||
Mastectomy and reconstruction | –0.020 (0.657) | –0.029 (0.468) | –0.049 (0.096) | Hormonal | 0.165 (0.023) | 0.234 (0.000) | 0.213 (0.213) | |
Mastectomy only | 0.041 (0.124) | 0.003 (0.914) | 0.017 (0.564) | Radiotherapy | 0.134 (0.001) | 0.134 (000) | 0.140 (0.005) | |
Surgery type non-specific | 0.036 (0.264) | 0.000 (0.990) | 0.023 (0.601) | Treatment not specified | –0.105 (0.014) | –0.112 (0.008) | –0.153 (0.000) | |
Surgery non-specific | 0.038 (0.165) | –0.010 (0.790) | 0.030 (0.508) | |||||
Non-surgical treatments (reference: chemotherapy) | Response to treatment (reference: stable) | |||||||
Radiotherapy | 0.078 (0.018) | 0.090 (0.003) | 0.104 (0.005) | Response | –0.085 (0.001) | 0.094 (0.001) | 0.088 (0.008) | |
Chemotherapy with toxicity | –0.026 (0.632) | Progression | –0.126 (0.159) | –0.205 (0.000) | –0.197 (0.001) | |||
Hormonal | 0.077 (0.017) | –0.074 (0.013) | 0.074 (0.085) | Terminal | –0.352 (0.000) | –0.390 (0.000) | –0.461 (0.000) | |
Treatment non-specific | 0.083 (0.030) | 0.087 (0.007) | 0.078 (0.048) | Response not specified | –0.187 (0.013) | –0.267 (0.000) | –0.244 (0.000) | |
Time period (reference: under 1 year) | Side effects (reference: no side effects) | |||||||
Peripheral neuropathy | –0.085 (0.063) | –0.138 (0.004) | –0.142 (0.010) | |||||
Over 1 year | 0.100 (0.004) | 0.038 (0.096) | 0.058 (0.019) | Oedema | –0.017 (0.755) | –0.011 (0.664) | –0.015 (0.519) | |
Time non-specific | 0.053 (0.123) | 0.006 (0.844) | 0.045 (0.322) | Febrile neuropathy | –0.192 (0.000) | –0.172 (0.002) | ||
Sepsis | –0.228 (0.009) | –0.160 (0.001) | –0.192 (0.005) | |||||
Hypercalcaemia | –0.628 (0.000) | –0.672 (0.000) | –0.856 (0.000) | |||||
Other side effect | 0.172 (0.125) | 0.194 (0.003) | 0.176 (0.021) | |||||
Whose values (reference: community sample) | Whose values (reference: community) | |||||||
Clinician | 0.164 (0.017) | 0.179 (0.010) | 0.130 (0.080) | Clinician | 0.033 (0.655) | 0.000 (0.997) | 0.016 (0.717) | |
Patient’s own health | 0.171 (0.001) | 0.209 (0.000) | 0.162 (0.007) | Patient’ own health | 0.240 (0.000) | 0.243 (0.000) | 0.209 (0.000) | |
Patient’s scenario | 0.085 (0.119) | 0.084 (0.108) | 0.077 (0.186) | Patient’ scenario | 0.156 (0.000) | 0.126 (0.001) | 0.138 (0.003) | |
Valuation method (SG) | Valuation method (reference: SG) | |||||||
VAS worst–best | –0.194 (0.002) | –0.187 (0.000) | NA | VAS worst–best | 0.045 (0.436) | 0.066 (0.107) | NA | |
VAS dead–full health | –0.205 (0.000) | –0.181 (0.000) | NA | VAS dead–full health | –0.068 (0.155) | –0.060 (0.062) | NA | |
EQ-5D | –0.215 (0.000) | –0.168 (0.001) | –0.112 (0.026) | EQ-5D | 0.107 (0.105) | 0.160 (0.062) | NA | |
TTO top not full health | 0.074 (0.176) | 0.099 (0.073) | NA | TTO top not full health | –0.0519 (0.470) | 0.0173 (0.593) | 0.0464 (0.337) | |
TTO different anchor | –0.014 (0.550) | 0.008 (0.785) | 0.016 (0.587) | TTO different anchor | 0.205 (0.013) | 0.257 (0.000) | NA | |
TTO top full health | –0.157 (0.021) | –0.133 (0.002) | –0.110 (0.023) | TTO top full health | 0.087 (0.233) | 0.143 (0.000) | 0.173 (0.003) | |
HUI3 | –0.248 (0.000) | –0.188 (0.001) | –0.132 (0.023) | Constant (base scenario) | 0.614 (0.000) | 0.640 (0.000) | 0.632 (0.000) | |
Constant (base scenario) | 0.725 (0.000) | 0.663 (0.000) | 0.648 (0.000) |
Authors (year) | Title | Country | Population |
---|---|---|---|
Prescott et al., 2007205 | A randomised controlled trial of postoperative radiotherapy following breast-conserving surgery in a minimum-risk older population. The PRIME trial | UK | Patients ≥ 65 years of age, provided that their cancer was considered to be at low risk of local recurrence, were suitable for breast-conserving surgery, were receiving endocrine therapy and were able and willing to give informed consent |
QALY estimation method | The objective of the PRIME trial was to determine whether or not adjuvant breast irradiation significantly changes the QoL of older women with breast cancer treated with breast-conserving surgery and adjuvant endocrine therapy. The QLC-C30 and QLQ-BR23 were used to complement the EORTC QLQ-C30 scale QoL measures | ||
Relevant QALY measures | Value | Source/comments | |
Radiotherapy | No radiotherapy | ||
EQ-5D | |||
Baseline | 0.77 (0.73 to 0.80) | 0.74 (0.70 to 0.77) | |
3.5 months | 0.78 (0.74 to 0.81) | 0.76 (0.73 to 0.79) | |
9 months | 0.76 (0.71 to 0.81) | 0.72 (0.68 to 0.76) | |
15 months | 0.74 (0.70 to 0.78) | 0.73 (0.69 to 0.77) | |
Unadjusted QALYs | 0.95 (0.90 to 0.99) | 0.92 (0.88 to 0.95) |
Appendix 8 Detailed findings from qualitative recruitment study phase 1: understanding recruitment issues in the OPTIMA prelim study
This appendix presents the detailed findings about recruitment issues in OPTIMA prelim, which were undertaken as part of phase 1 of the integrated QRS. They form the basis on which QRS interventions to improve recruitment were developed and implemented in close collaboration with the CfI and TMG. These data were obtained, and the interventions implemented, during recruitment.
Qualitative recruitment study data set
Fourteen semistructured interviews were conducted with participants across six sites, including eight oncologists (three of whom were also members of the TMG); four research nurses; one surgeon; and a non-recruiting member of the TMG. Interviews were conducted at ‘mid-range’ centres by chance rather than by design. One of the centres included in the QRS had failed to recruit any patients; the remaining centres’ recruitment rates ranged from 0.32 to 1.85 patients per month compared with an overall range of 0 to 2.20 in the study. At least one site from each of the OPTIMA prelim geographical clusters participated in interviews. Eight structured telephone interviews with research nurses were conducted to get a broad overview of the patient pathway for each site. A total of 36 audio-recordings were obtained from 29 patient consultations. First and second oncology consultations were recorded for 7 of the 29 patients. First oncology consultations were available for 21 patients and the second oncology consultation available for one patient. Twelve different recruiters led the consultations (10 consultant oncologists and two oncology registrars) and audio-recordings were obtained from all geographical clusters.
Presentation of data
Quotations from semistructured interviews and audio-recorded consultations have been selected on the basis of how clearly and succinctly they illustrate the key themes to emerge from the research. Quotations from divergent cases presented if these existed. Some themes have been supported through data from interviews and audio-recorded consultations. The following identifiers have been used for quotations:
‘Int’ = interviewer (LR or JD); ‘Onc’ = oncologist; ‘RN’ = research nurse; ‘Pat’ = patient; ‘Relative’ = patient’s relative; ‘Friend’ = patient’s friend.
Phase 1: understanding recruitment issues
The recruitment issues identified through the QRS were in three overarching themes:
-
eligibility processes and patient pathways
-
missed opportunities for discussing the trial
-
opportunities for improving communication and information provision.
Structured and semistructured interviews were the principal data collection methods informing recruitment difficulties that manifested outside of recruitment consultations. Recruitment challenges that occurred within recruitment consultations not only arose through listening to audio-recordings, but were also hinted at in interviews with recruiters and screening log analysis. Each of the above categories of ‘recruitment difficulties’ will be discussed in turn, with ‘potential action points’ indicated for the QRS intervention presented at the end of the section for phase 1 in the main body of the report.
Eligibility processes and patient pathways
Eligibility processes and patient pathways were felt to be difficult by recruiters. These included clinicians’ and triallists’ decision-making about patients’ eligibility and the series of OPTIMA prelim-related events patients experienced in the lead up to entering (or declining) the trial. These processes had potential to hinder recruitment, and so provided an initial focus for phase 1. Obtaining an overview of these processes from the OPTIMA prelim staff also provided a useful framework for placing specific barriers to recruitment into context. At the commencement of the trial, screening logs recorded patients from only the point of registration and randomisation; this made elucidating the full patient pathway challenging. The details of eligibility processes and patient pathways described below are based on interview data, but quotations are not included as the information presented consists of factual details of processes (rather than views or opinions). Much of the information was based on LR’s field notes made during structured interviews with research nurses.
Eligibility assessment processes
The first stage of assessing patient eligibility occurred during the MDT meetings. This was the first point at which patients’ post-surgical pathology results were discussed by the clinical team. Research nurses and oncologists working on the study were principally responsible for flagging potentially eligible patients, but the final decision to pursue the trial was based on team consensus. Research nurses reassessed identified patients’ eligibility by reviewing patient notes and double-checking pathology results following the MDT meeting; if still eligible, the patient would be approached about OPTIMA prelim. Any drop-outs owing to ineligibility after this point were a result of ineligibility on central screening or the emergence of new knowledge about medical history or health status.
Patient pathway: events occurring from the patient’s perspective
The pathway for patients potentially eligible for OPTIMA prelim was simple and consistent across centres, with good continuity of care owing to the support given by research nurses. The pathway broadly consisted of an initial surgical consultation followed by two consultations with oncologists on separate days (Figure 21).
FIGURE 21.
The OPTIMA prelim patient pathway: from surgery to decision to accept/decline the trial.
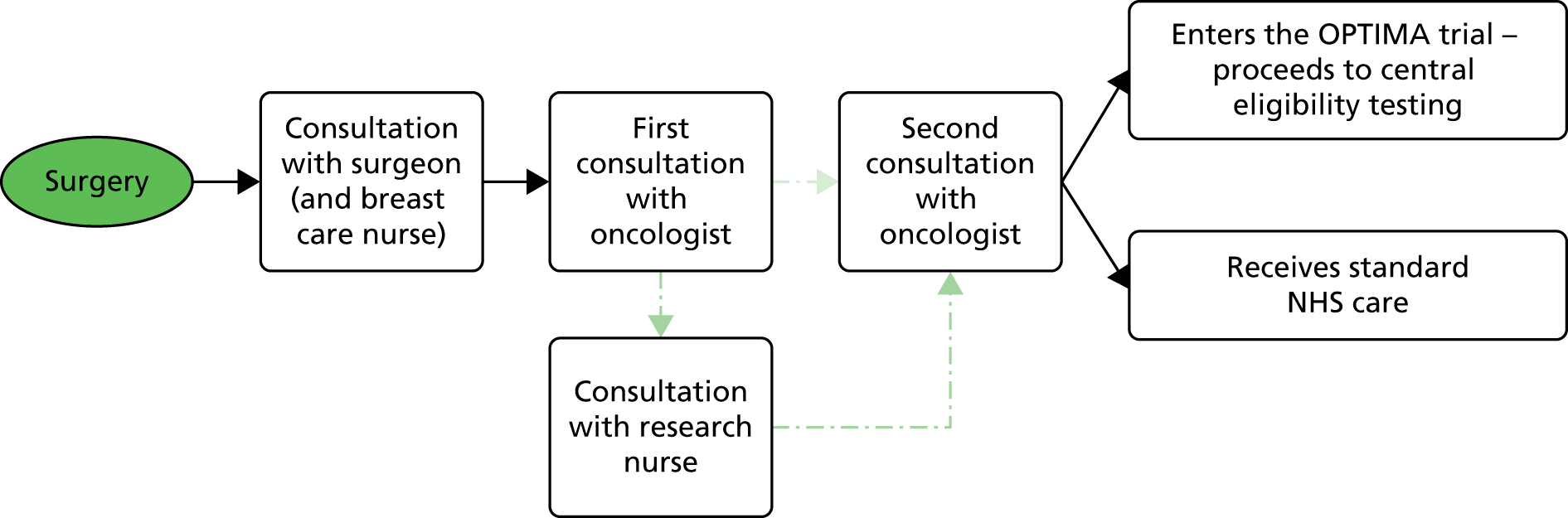
The OPTIMA prelim study became potentially relevant to a patient’s pathway after surgical removal of the tumour. The first point of clinical contact after surgery was with the surgeons, sometimes accompanied by breast care nurses. The main purpose of this consultation was to review the results of the surgery. The study and potential further treatments were sometimes briefly mentioned to inform the patient of what to expect at their next consultation. However, the first consultation with the oncologist was usually the first point at which OPTIMA prelim was introduced and explained in depth. Information sheets were distributed at this first oncology consultation, and patients were introduced to research nurses as their primary point of contact for study-related matters. Patients generally gave their decisions regarding trial participation in a second oncology consultation, organised approximately 1 week after the first. Regardless of the patient’s decision about the trial, the next steps in the patient’s treatment pathway were discussed and set into motion in this second oncology consultation.
Research nurse involvement
Research nurse services were generally well integrated into patient pathways. Aside from being the first point of contact for patient queries, research nurses supported patients through offering face-to-face consultations immediately after the first oncology consultation (where feasible), or giving patients the option of a follow-up telephone conversation. In some cases, research nurses telephoned patients within a few days of the first oncology consultation as a matter of routine.
Declining the OPTIMA study via telephone
Patients’ initial decisions relating to trial participation were generally discussed face to face in the second oncology consultation. This system allowed oncologists the opportunity to explore patients’ views and reasoning. In contrast, patients’ decisions were initially expressed over the telephone (to research nurses) at two of the QRS centres. If patients declined OPTIMA prelim, the second oncology consultation focused on organising the next steps in the treatment plan, with no further discussion of the trial. This removed potential for oncologists to answer questions or clear misunderstandings about the trial and reduced the possibility for in-depth discussion.
Summary of eligibility processes and patient pathways
Eligibility processes appeared straightforward, but systems were not always in place to map eligibility from the first point at which patients were considered for the study. This probably led to potentially eligible patients not being offered the opportunity to consider OPTIMA prelim. Patient pathways were clear, simple and appeared successfully integrated into research nurses’ services, but discussion of the patients’ decision regarding OPTIMA prelim participation via telephone (at some centres) meant that some patients may not have discussed their thoughts on participation with an oncologist. Consequently, patients’ reasons for declining the trial could not always be assessed through audio-recordings of consultations.
Missed opportunities for discussing OPTIMA prelim with patients
Interviews and early TMG meetings showed recurring concern among staff that fewer than anticipated patients were being approached about the study. Regular telephone conversations with research nurses frequently suggested that no eligible patients had been identified. Six interview informants from five different centres talked about the limited flow of eligible patients they had encountered at the time of interview. This was the most frequently reported issue raised by informants when asked about their views on the main barriers to recruitment:
I don’t know why we’re not actually identifying (any); it’s not that we’re deciding that we’re . . . no, it’s not that we’re approaching patients and they’re saying ‘No’.
RN1
With the starting pool of potential eligible patients unknown, little could be deduced with regard to the numbers of patients deemed ineligible or the associated underlying reasons for ineligibility. This led to TMG members producing broad, non-specific explanations of why the numbers of patients approached for the OPTIMA prelim were lower than expected when the study was conceived:
[Dr A] will probably tell you but at the meeting yesterday [they] said how surprised [they were] about how small the pool of eligible patients is. It’s much smaller than (they) thought it would be [. . .]; that’s like a change of tune, really, from before we started recruiting (they) were very positive about how big the pool was [. . .]. So I think (their) experiences in trying to identify eligible patients have shown that perhaps the expectation of how many eligible patients there would be, were a bit, perhaps slightly over-enthusiastic.
TMG member
Further evidence supporting the theory that the full pool of eligible patients was not being considered for the study came in the form of recruitment figures and acceptance rates. Actual recruitment figures trailed behind target figures throughout the recruitment period, while ‘acceptance’ rates consistently exceeded targets. Interviews with recruiters gave insight into potential attitudinal factors that may have played a role in restricting the pool of eligible patients. These explanations were viewed as potential contributors rather than absolute reasons; in reality, the factors described below may have acted in conjunction with a genuine over-estimation in the prevalence of the target population.
Discomfort surrounding eligibility criteria
Discomfort surrounding various aspects of the eligibility criteria appeared to be widespread, reported by staff members from every centre participating in interviews. Research staff varied in their readiness to accept increasing risk (in terms of disease status). Increasing lymph node involvement, tumour size and grade caused discomfort surrounding the upper thresholds of the eligibility criteria stated in the protocol.
Research nurses and oncologists from four different centres commented that they felt that the upper limit of permitted lymph node involvement in the eligibility criteria was particularly high (nine nodes). This was especially pertinent given that oncologists discussed how their clinical training had always connected any lymph node involvement with chemotherapy:
The only . . . yeah, the only thing that surprised me with the criteria is the lymph node status: one, because it’s almost ingrained in our practice and certainly as oncology trainees as we go through doing the exams and so on, that lymph node positivity is a risk factor for a recurrence, and that those patients who are highest risk benefit from chemotherapy most . . .
Onc7
Two research nurses shared this discomfort about the upper permitted levels of lymph node involvement. While one dismissed her views as unimportant, the second felt that her feelings on this matter were shared by her clinical team; this led on to her suggestion that patients at the upper limits of inclusion criteria may not have been considered for the trial at her centre:
Yeah, so you were mentioning about you feel eight nodes should get chemo, yet the eligibility criteria says [up to] nine nodes. Have you had any patients with that level of nodal . . .
No, we haven’t, no. I think they wouldn’t have been offered the trial myself.
Discomfort surrounding high levels of lymph node involvement could be alleviated if recruiters had sufficient confidence in the Oncotype DX test’s accuracy in determining appropriate chemotherapy provision, as explained by one recruiting oncologist. This oncologist was deemed a standalone case, being the only recruiter outside the TMG to express confidence in the Oncotype DX test (which in this case, alleviated concerns about ‘higher-risk’ patients):
But I have to say I’m fairly confident if they had the testing they’d probably end up having the chemo anyway because they’d probably come out at high risk, so I’m not worried that we’d under treat people.
Onc4
Recruiters’ confidence in the Oncotype DX test was only one facet of a complex decision-making process for offering the trial to patients. The views of the wider clinical team (i.e. within MDT meetings) appeared to be just as important as recruiters’ attitudes. For example, one of the oncologists (Onc7) spoke of the lymph node criteria being problematic for surgical colleagues, although these attitudes had started to change with increasing confidence in the Oncotype DX test:
Actually you can [i.e. are permitted to] put into OPTIMA patients with really quite high-risk disease, like nine positive nodes, and initially my surgical colleagues would only allow us to offer OPTIMA to patients with low-/intermediate-risk disease, which severely restricted the pool. But in the last couple of weeks I’ve noticed they’re becoming much more relaxed about it and they’re saying things like ‘Oh, well if this patient needs chemo, the testing will be able to pick them out’.
Onc7
A number of participants – research nurses and oncologists – talked about a combination of lymph node involvement and other proxies for risk (e.g. tumour size, grade), as factors that collectively influenced views on whether the OPTIMA prelim was an appropriate option for patients:
Well . . . I think the smaller tumours and with maybe just one or two positive nodes I feel fine about that [comfortable with entering into OPTIMA], but maybe the larger ones like that last lady I discussed, I’m not sure about what I’ve heard about it at all.
RN4
Ultimately, a range of clinical characteristics contributed to clinicians’ ‘gut feelings’ about the likely benefit of chemotherapy. The oncologist below gave an example of a specific patient being excluded because of the MDT’s assessment of multiple factors that labelled the patient as ‘high risk’. As expressed below, overcoming clinical intuition could be problematic:
There was a girl, last week, who I think was premenopausal but who had eight positive nodes, so I think we [inaudible] felt if she [laughs] . . . although she was eligible for the trial, we would probably be uncomfortable if she had received hormones. Whether or not that’s based on our preconceptions and not on what the tumour biology might tell us, is another matter.
Onc5
The reliance on ‘gut feelings’ conflicted with one of the OPTIMA prelim study’s major aims: to investigate whether or not the Oncotype DX test was a more sophisticated approach to assessing potential to benefit from chemotherapy – one that went above and beyond the crude indicators informing clinicians’ instincts.
Where OPTIMA becomes very interesting, it is, OPTIMA is supposed to tell you in a way better than your own gut feelings.
Onc6
Finally, although not widely reported across the sample, staff members from one particular centre talked about applying their own eligibility filters to determine systematically who should be considered for OPTIMA prelim. This extra filter was an online predictor tool that estimated the benefits of chemotherapy provision, and patients scoring above a certain estimated level of benefit were automatically deemed inappropriate for the study:
So I’m a little bit uncomfortable with entering patients into the OPTIMA trial where I think I would have liked to have seen an upper limit of benefit, which means that if the patient benefit from chemotherapy using accepted criteria would save more than 8% in 10 years, then they shouldn’t be considered for the OPTIMA trial.
Surgeon
It should be noted that this centre was deemed a stand-alone case within the QRS, but it is possible that similar practices were occurring in centres that were not participating in the QRS.
Concern about patients’ ability to handle information
Some clinical informants gave general or specific examples of refraining from offering the trial to patients on the basis of their clinical judgement. Three individuals from two different centres suspected cases or had experienced cases where patients were anticipated to have likely difficulties understanding the trial, and so were not approached:
I suspect more may have been eligible but there’s been reasons for not going ahead and discussing it with them. In fact there’s one that I haven’t put in my sheet and it’s initially because they felt that this lady couldn’t take that information. That she wasn’t . . . it was a step too far for her, it wasn’t even discussed with her.
RN4
These judgments about patients’ abilities to interpret information – whether or not intentional – had the potential to influence recruiters’ efforts in explaining the trial. In these cases, they avoided providing clear, full explanations of the trial:
So we . . . we might . . . if we thought they weren’t really suitable, we might say ‘Well, we do have a trial that would help us choose whether to have chemotherapy or not and would you like us to explain that to you?’ in a kind of very general way, and kind of probably hoping the patient will say ‘No, I don’t want anything like . . . oh no I don’t want that.’
RN2
Some recruiters alluded to abandoning efforts if they sensed that the patient did not have the capacity to absorb information about the trial, be this because of underlying health issues or the way the patient was feeling at the time. This was often a conscious choice on the recruiters’ part:
I’m thinking of one situation where somebody I didn’t think had the mental capacity to get her head around the trial. So we just decided what to do (outside of that). And that will happen occasionally when you see people and you think this is going to be too much to cope with [. . .]. You just don’t want to burden them with extra information.
Onc4
Failure to approach patients owing to a clinician’s discretion would not breach the study protocol as the eligibility criteria clearly stated that the ‘Patient must be fit to receive chemotherapy and other trial-specified treatments with no concomitant medical, psychiatric or social problems that might interfere with informed consent, treatment compliance or follow up.’
However, the issue arises about whether or not judgments not to inform patients about the opportunity to take part in the OPTIMA prelim study were always appropriate or well founded. Such judgments, if applied differently across different centres, could have had implications for the numbers of patients being invited to join the study.
Priority of OPTIMA prelim at multidisciplinary team meetings and among surgeons
Although research nurses were well versed in the inclusion and exclusion criteria, some felt that they did not always have the necessary expertise or voice to flag a patient as an OPTIMA prelim candidate at an MDT meeting:
I tend to just whisper across the table, ‘the OPTIMA trial,’ and [clinician x] or [clinician y] will maybe say ‘No’, or ‘We can think about it’, but I think I might need to have a word with them as well and say . . . I think we ought to, possibly tomorrow, we have an MDT, maybe have a word with them before we start and say ‘Look can we really look at the OPTIMA trial if the patients are eligible,’ . . .
Rather than . . . I see . . . so has OPTIMA ever been discussed in the MDT then?
Not in any great depth, no I don’t think. It’s because it’s about four surgeons and they’re all trying to get their patients discussed and what we’re going to do and everything else. It’s quite manic.
Some research nurses also expressed this view in QRS feedback meetings.
Summary of missed opportunities for discussing OPTIMA prelim with patients
To summarise, findings from QRS interviews and informal discussion/observation in meetings suggested that there had been missed opportunities to approach eligible patients about the OPTIMA prelim study. This was a key finding from the QRS and suggested that there would be opportunities to increase levels of recruitment. These findings were supported through the observation that while numbers of eligible participants were falling short of targets, the study seemed very acceptable to patients who were informed about it, as the percentage of eligible patients agreeing to randomisation consistently exceeded targets.
Opportunities for improving communication and information provision
Recruitment consultations varied in length and structure across and between centres, with some formats more conducive to explaining OPTIMA prelim than others. Difficulties emerged in relation to how trial processes and concepts such as ‘uncertainty’ were explained to patients. Staff attitudes also played a role in influencing the direction consultations took, particularly when it came to exploring patients’ views and perspectives on study participation. Each of these areas will be briefly summarised in turn, with supporting data from consultation recordings, interviews and screening logs (where relevant).
Explaining randomised controlled trial processes: treatment allocation and blinding
A fundamental challenge to recruitment identified by interview informants was the difficulty perceived in explaining study design. Five interview informants commented on OPTIMA prelim’s particular complexity relative to other trials they had worked on:
I think it’s probably a bit more difficult for patients to understand and I think from my experience of patients that we have seen and discussed with, I think that it takes them a while to work out how the trial is designed [. . .].
RN3
Having an arm that split into two further arms, combining random and test-directed treatment allocations, and the partially blinded design were all thought of as potentially confusing to patients. Some interview informants had directly experienced patients dismissing the trial on this basis:
This particular lady just didn’t go into any detail at all she said, I just didn’t know what it was talking about, and I said, and I tried to explain bits of it too her, but no, she said, I just, I don’t, I just want to, I don’t understand it, she just said locked her mind off completely to it.
RN4
In the light of the above, analysis of audio-recorded consultations focused on recruiters’ explanations of trial processes and scrutinised information exchange that led up to evidence of patient misconceptions or confusion. Particular practices were identified as being potentially detrimental to patient understanding. This included absent or incomplete explanations of RCT processes, and scope for greater clarity in the communication of what ‘uncertainty’ related to and meant.
Most evidence of patient misunderstandings tended to occur in relation to the OPTIMA prelim design. While individual patient knowledge and experiences undoubtedly played a role in comprehension, there was an opportunity for some recruiters to focus on, or refine, explanations of trial processes and terminology. This was particularly relevant for explanations of randomisation and descriptions of what each of the study arms entailed.
Recruiters sometimes provided incomplete descriptions of what randomisation entailed and why it was carried out. In a number of cases, the term ‘random’ was not explained at all:
Then it’s decided at random whether you go down the Oncotype testing path or whether you just get the standard treatment.
Onc14 (consultation)
Where randomisation was explained, recruiters usually conveyed the idea that there would be two groups of patients of equal size, but did not explain the removal of patient or clinician choice in allocation. This was sometimes in contrast to recruiters’ interview accounts of how they would explain randomisation, as seen below:
Yeah. I usually say that there are two arms of the trial, and half the people go into one and half into the other, and we don’t choose. And we don’t know which one you’ve been put in; that’s done on a special basis.
Onc4 (interview)
It’s a randomised trial so half the people get the standard treatment; so if you go into the study, you may just get chemotherapy irrespective of what the test shows because that’s our standard. That would be considered the control arm of the study [. . .]. The other arm, which is the experimental arm, will have a decision based on the result of that test.
Onc4 (consultation)
Incomplete explanations of randomisation are likely to have contributed to common misconceptions, including patients’ assumptions that they were ‘choosing’ to receive the Oncotype DX test or that their treatment allocation would be based on the clinician’s view of what is ‘best’ for them (see extracts 1–3, below). These understandings were all reasonable interpretations of the limited information provided by recruiters.
Extract 1
We might as well let them do the test, mightn’t we?
Pat (consultation)
Extract 2
So – the tumours that were taken out get tested . . .
Well it’s if they do it, yes.
If they do it, yes.
If you say you want it done.
Consultation
Extract 3
But you still get the best treatment for you even though you go into this computer?
Pat (consultation)
Explanations of the trial arms also revealed examples of ‘loaded’ terminology, including use of the words ‘standard’ and ‘experimental’ to describe the arms. Use of these words does not enable the arms to be considered as equivalent, leading to implications for how acceptable randomisation would be.
The concept of blinding was often omitted or not fully explained by recruiters in the first oncology consultation. Where blinding was covered, patients’ reactions were usually a good indication of how well they had understood the trial design. While some understood, others asked for clarification of misconceived ideas, or expressed confusion.
Extract 4
But I don’t get the actual result; I get told whether you’re going to have chemo or not. When you’re tested, if it comes back as a low score, then you get no chemotherapy. If it comes back as anything, intermediate or higher-risk score, you get chemo.
Either you make the decision, or you let someone else make it for you?
Consultation
Extract 5
One thing, however, is that the study is what is called ‘partially blind’, which means that if you end up having chemotherapy, neither I nor you will know whether or not you actually had the Oncotype test.
Each lady has the Oncotype test?
Consultation
Recruiters’ descriptions of what happens in each study arm were also sometimes confusing. Explanations did not always specify that endocrine therapy would be provided in all treatment arms, possibly encouraging patients’ assumptions that this was a trial of ‘treatment’ versus ‘no treatment’, or ‘chemotherapy’ versus some other unknown treatment.
Extract 6
It’s a randomised trial so half the people get the standard treatment . . . so if you go into the study, you may just get chemotherapy irrespective of what the test shows because that’s our standard, that would be considered the control arm of the study. Make sense so far?
Mhm.
Yeah.
The other arm which is the experimental arm will have a decision based on the result of that test [. . .] if you’re high risk you get the chemo, if you’re low risk they think actually the chemo’s not going to be much benefit, you don’t get the chemotherapy.
[Later in consultation]
So how, if [patient] didn’t have the chemo . . . and she had this new trial, how is that taken, how is that given?
Consultation
Tailoring consultations to the OPTIMA prelim study
Recruiters varied in the extent to which they presented OPTIMA prelim as the primary focus of the discussion. This was reflected most clearly by the point at which the study was introduced in the consultation, and the way in which non-study-related information was framed. Details of surgery outcomes, pathology results and non-chemotherapy treatment recommendations were generally covered prior to introducing OPTIMA prelim, and this ‘lead up’ time ranged from approximately 2 to 25 minutes. Some consultations were particularly long (up to 52 minutes), covering non-trial-related information in some detail prior to introducing the study.
In some ways, it could be considered that many consultations were not ‘recruitment consultations’. Although there was the opportunity to present the OPTIMA prelim study, this opportunity was not always fully utilised. In some cases, OPTIMA prelim was presented as a ‘next step’ in a series of ‘information blocks’, rather than the heart of the consultation.
Uncertainty: chemotherapy provision and accuracy of the Oncotype DX test
The issue of uncertainty was an important theme to arise from audio-recorded consultations, particularly in relation to the discussions about chemotherapy benefits and the accuracy of the test. These areas of uncertainty were the essence of OPTIMA prelim’s aims and thus fundamental points to communicate. Apart from issues of trial design, most examples of patient confusion or misconception arose from incomplete understandings of uncertainty in relation to chemotherapy and/or the Oncotype DX test.
Chemotherapy as an uncertainty
There were occasional examples where patients still believed that they would definitely benefit from and/or receive chemotherapy, even after the trial had been explained. This prompted us to scrutinise how chemotherapy was first introduced into recruitment consultations by the oncologist. Broadly speaking, descriptions fell into two categories: ‘chemotherapy as a beneficial treatment’, and ‘chemotherapy as an uncertainty’.
In some consultations, chemotherapy was initially presented as a treatment that would offer some benefit, with varying descriptions of what this benefit was and how it was expressed (i.e. in quantitative or qualitative terms). This would later be countered as recruiters explained that chemotherapy might not be beneficial at all, leading on to descriptions of the trial. These consultations tended to be longer than others, and there were increased opportunities for patients to become confused about the role of chemotherapy. As a consequence, it became more difficult to explain the rationale behind the trial. Patients who were introduced to chemotherapy as a certainty were more likely to show signs of confusion about chemotherapy provision later in the consultation:
Extract 7
Back to drugs. The other thing that we have to consider, and it’s I would say a case of icing on the cake, but nevertheless, we should talk about it, is the benefits of a course of chemotherapy [. . .].
Would you recommend it then?
I think with what you have there is probably enough benefit for it to be worthwhile. It should be considered quite seriously. [Introduces OPTIMA and the Oncotype DX test.]
You’ve completely confused me now. OK, so I accept that I’ve got to have the chemotherapy and tablets . . .
Consultation
Extract 8
And, a lot of – you know – we would normally give chemotherapy, um, for, a tumour such as this.
Yes.
Because again it reduces the risk of it coming back.
Right.
[Later]
I want to get everything finished with.
You just want to get on with it.
[Yeah, yeah].
[And just] have the chemo.
Yeah. Bad enough I got to have both.
Consultation
Within consultations that presented chemotherapy as a certainty, there were some examples where the language carried subtle suggestions that chemotherapy was the optimal (e.g. ‘icing on the cake’) or ‘responsible’ choice:
I usually say this sort of treatment that we offer, like chemotherapy is a bit like having fire insurance on your house. You probably don’t need it because you’re going to be OK without it. But you’d feel a bit silly if your house catches fire and you haven’t got it.
Currently there is no straightforward method of identifying better who is likely to benefit and not benefit, so we play safe.
Interview
In contrast, some recruiters framed chemotherapy as an uncertainty from the outset, alluding to this being a ‘difficult decision’, or a ‘question mark’. This approach to introducing chemotherapy seemed mostly to lead to clearer patient understanding of OPTIMA prelim and the role of chemotherapy.
Extract 9
We would certainly recommend [endocrine therapy]. The one that can sometimes be a little bit more difficult is the chemotherapy treatment, and the reason for that is when you start to think about side effects and down sides of the treatment, it is more complicated. Um, there are more potential side effects, so you have to be really sure that that treatment is of benefit.
Onc10 (consultation)
Extract 10
That leaves the question of whether we should also give you a course of chemotherapy or not, which is a slightly more tricky question [explains probability of benefits]. What we’d love to do is be able to pick which those five are, and not treat everyone else.
But you can’t do that . . .
Consultation
Extract 11
The thing that’s a little harder to decide is whether you need chemotherapy or not.
It’s the big one, yeah.
It’s a harder decision for two reasons. One is, that its, lots of patients like yourself would be fine even if they didn’t have it, and, uh, also it has obviously more side effects [explains probability of benefit]. That means, though, that 90 of them or more who have had chemotherapy, have had no benefit.
It’s just whether I’m one of those ones.
Consultation
Uncertainties surrounding the Oncotype DX test
Uncertainty surrounding the use of the Oncotype DX test in treatment decision-making was a difficult concept to communicate according to recruiters’ interview accounts (i.e. oncologists and research nurses). Recruiters’ reported experiences and screening log date showed that patients sometimes declined the trial with the intention of paying for the Oncotype DX test privately to guarantee receipt of the test (8% of reasons for declining the trial). In contrast, others did not trust the test’s ability to accurately inform appropriate chemotherapy provision. One recruiter described difficulties in giving a balanced explanation of the benefits and potential risks of the test. Over-selling the benefits could prompt patients to pay for the test privately, whereas an overly cautious approach ran the risk of obscuring any incentives for participating in OPTIMA prelim. These considerations raised the dilemma of how best to reassure patients while conveying the inherent risk of participating a study where treatment allocation may be determined by a test that requires further research.
The trouble is if in our initial interview we sort of really down play the test as not being useful, then nobody would want to go in the trial in the first place. And if we play it too much they think ‘well it’s such a great test, I don’t want to risk not having it in the trial’ and go and find out the information. So again, it’s that fine balance.
Onc4 (interview)
The TMG meetings revealed that a particular point of concern was a line in the PIS that highlighted the uncertainty surrounding the test’s accuracy. Based on TMG members’ experiences (not audio-recorded), patients had reportedly refused the trial once they had read this:
The test might not work so well for patients with larger tumours or involved lymph glands. We do not believe this to be the case. However, if it was, then in the future we might realise that we should have given you chemotherapy.
It was noted that this issue reportedly materialised after (rather than within) the first oncology consultation (i.e. once the patients had read the information sheets). This prompted us to consider how the Oncotype DX test was described in consultations, with a focus on how uncertainty was communicated and responded to.
Uncertainty was most commonly conveyed through the idea of having insufficient evidence and/or information about using the test in node-positive women, or the desire to ‘learn more’ about use of the test in this group of women; however, some recruiters were less specific, making indirect references to uncertainty (n = 5):
Extract 12
There is a new very sophisticated test that has been developed, that is only available in one laboratory in America, and is now used as a routine for patients who have lymph node negative cancers where there is no involvement of the lymph nodes. [1-second pause] And it’s now being looked at in women who have a lymph node involved.
Onc17 (consultation)
Extract 13
It’s a test that is reasonably well established, but the exact . . . where exactly we should be using it is still kind of being looked at.
Onc1
Some patients questioned the purpose of the trial in response to indirect or non-specific communication of uncertainty:
Extract 14
Now. This is something that’s already used in the US for people without a lymph node. So they do this already [. . .]. So the next stage of that is to look to people who have one lymph node that’s moved slightly away. And, as I say, it helps with the ‘not sure whether or not we should give chemotherapy’. [Later explains trials design].
Why don’t – if that’s the case – why don’t they just save the money on chemotherapy for those people who don’t need it? Why not just do the test on everybody?
The test is to see if it’s accurate.
Oh I see – you’re not sure how accurate the test is?
Consultation
Recruiters also varied in the extent to which they coupled uncertainty with reassurance. It was common for recruiters to talk about the routine use of the Oncotype DX test in America, and less frequently, the existence of retrospective data to support its use in patients with lymph node involvement. Some recruiters went one step further by expressing their personal views on the test’s accuracy. All recruiters who did this expressed confidence in the Oncotype DX test coupled with a specific statement about uncertainty (e.g. insufficient evidence, not enough information):
Extract 15
Obviously, we wouldn’t do this if it put some patients at extra risk. We’re confident that this test predicts which patients need chemotherapy better than the old-fashioned way we did it with just the size of the tumour and the nodes. This test is, actually, now available on the NHS for a different group of patients [. . .]. What we’re trying to find out is to prove that it’s a good test to do for patients who’ve got involved lymph nodes. So we already know this is a good test, basically, it’s just a question of trying to expand its use in patients who’ve got involved lymph nodes.
Onc12 (consultation)
Patient preferences
Screening logs and interview informants’ accounts suggested that patients’ preference either for or against chemotherapy was the most common reason for declining the trial (50% of reasons for decline in final screening logs: 33% for and 17% against):
We have a very good functioning wider unit [. . .]. There’s nothing that’s blocking us from doing it other than patient preference.
Onc3 (interview)
A common thread that ran throughout informants’ portrayal of patient preferences was the implication that the outcome of recruitment consultations was fixed, and independent of events and discussions occurring within the consultations. Breast cancer patients in particular were thought likely to have set preferences given the influence of the media, peers and the well-publicised nature of the disease. As such, some recruiters completely disassociated themselves from patients’ preferences, evoking a sense of powerlessness:
The people who say ‘well my friend down the road had breast cancer and she had chemotherapy and so therefore that’s what I’m going to have or I don’t want to have it’ – those people are more difficult I think because then they’ve already made their decision I guess.
Onc2 (interview)
The idea that surgeons/breast care nurses may create or reinforce expectations for a particular treatment regimen was considered as a possibility by most interview informants, but generally dismissed as not being an issue at their particular centre; their own clinical teams were presented as cohesive in their support of the OPTIMA prelim trial. Despite this, a number of audio-recordings showed examples where patients had come to the recruitment consultation with clear expectations for or against chemotherapy, based on advice received in previous consultations with surgeons (i.e. prior to the first consultation with the oncologist)
Extract 16
‘Cos – I know, before today, my wife had decided that she wasn’t going to go on the trial.
OK.
Um, purely because when I spoke to [Mr X], my husband asked him what would he actually do. And he said if it was his sister, he would highly advise the chemo.
Consultation
Extract 17
Because at first, initially, she was given the impression that it would just be take the lump out, be given radiotherapy, end of.
Tablets, yes.
So you’re saying that’s not the case?
Consultation
Do patients really have fixed views?
Patient preferences were difficult to assess based on the limited number of audio consultations, especially given that most of these captured the first oncology consultation rather than the consultations where patient decisions were expressed. Patients tended to take a passive role in consultations, quietly absorbing the information provided by the oncologist. There was usually space provided for patients to comment. These spaces were usually filled with indications of assent (e.g. elongating words such as ‘ahhh’, or ‘I see’, definitively stating ‘right’), or patients inviting the oncologist to continue speaking (e.g. ‘right’, ‘uh hum’, stated with a rising intonation). There was little evidence of patients voicing strong preferences for a particular treatment at this stage of the pathway, although evidence of concerns or preconceived ideas sometimes appeared, as shown in the previous section. Nonetheless, recordings of first oncology consultations did not provide any evidence to support the assertion that patients had ‘fixed views’ (although equally, it cannot be said that these fixed views were not internalised).
Exploring patients’ decision and preferences
The small numbers of recordings of second oncology consultations gave the opportunity to analyse how patient decisions and preferences were handled. Generally, these revealed recruiters’ reluctance to explore patients’ reasons for declining the trial. This seemed to be related to recruiter discomfort with discussing such views, or the belief that exploring decisions had no practical value. Recruiters’ discomfort stemmed from concerns about the potential for coercion, or that raising these issues would jeopardise their relationships with patients. When asked how they reacted when patients declined the trial, all interview informants expressed acceptance and a shift in direction within the consultation:
Well I think it’s quite important not to . . . it has the potential to kind of damage the relationship with the patient because the patient then feels . . . there’s risk that they might feel they’re letting you down.
We don’t do a hard sell you know, we don’t really force them. We don’t want to coerce anybody into it, so we respect their wish and go with that.
Interview
Recruiters who saw no value in exploring patients’ decisions were also more likely to dissociate the outcome of recruitment consultations from their own actions, instead focusing on factors that were not amenable to change (e.g. patient personality). If patients’ decisions were to be explored, this was merely seen as a tick-box exercise for trial-reporting purposes:
I go back to it, perhaps at the end of it and say would you mind just answering a couple of questions about your reasons why because it’s really helpful to the trial, trying to work out why it’s acceptable or not.
Would you feel comfortable once a patient had said no, personally would you feel comfortable exploring that?
No. Well I don’t know. I mean . . . I think I wouldn’t . . . I wouldn’t explore it there and then basically because there’s not . . . there isn’t the time and is not . . . but I have no problem to go back afterwards and discuss that with them.
Have you . . . have you done that or . . .?
No I haven’t done it because I’ve not . . . I’ve not seen the [. . .] If it was part of the study I would be willing to do it, but I think I would . . . I just . . . I feel that I accept their decision basically.
Yeah. Yeah no I can . . . can understand. I can understand that. And you feel it is kind of partly due to logistics of not really having time to go in and . . .?
Well it also the . . . what would I learn from exploring it?
[Later]
I suspect that there will be certain characteristics about the patient that if you have the right questionnaire to give them before they come to see me, if they have certain traits the chance of accepting a trial will be kind of less than 5%.
Interview
Recordings of patients declining the trial were available for seven consultations. Even in this small sample, there was evidence of patients declining the trial based on concerns that could potentially have been alleviated. This included concern about the safety of treatment delays resulting from participation in OPTIMA prelim and a worry about hair loss. Recruiters appeared to accept patient decisions without any attempt to rectify these misconceptions or offer potential solutions to concerns. Only one recruiter actively explored patients’ decisions by reviewing details of the trial that were misconceived and discussing new information to alleviate concerns. These measures could only be taken if relevant and appropriate, but this could not be determined if there was a reluctance to initially explore patients’ decisions. Aside from missed opportunities to address misconceptions and fully inform patients, recruiters’ reluctance to explore patients’ reasons for declining the study impeded the potential to learn about the acceptability of OPTIMA prelim to patients. According to screening logs, no reason was given in 24% of cases where patients declined the trial.
Delay
Patients’ concern about the delay attributed to the Oncotype DX test was a dominant theme to emerge from audio-recorded consultations. Almost every consultation analysed supported this, with most patients asking about how long the testing process would take.
Extract 18
I’m just thinking every minute counts, but, yeah.
Pat (consultation)
Two staff interview informants gave specific examples of patients declining the trial owing to the delay this imposed on initiating treatment; likewise, screening logs suggested that this was the principal reason for declining the trial in 4% of cases. While some recruiters reassured patients that the delays associated with OPTIMA prelim were safe, this was not consistently done across consultations. This therefore also represented a missed opportunity to reassure patients.
Summary of opportunities for improving communication and information provision
Analysis of audio-recorded consultations revealed opportunities to clarify and refine recruiters’ explanations of trial specific processes to patients, reconsider use of terminology and frame explanations of treatment options around the OPTIMA prelim trial design. Both interview data and audio-recorded consultations revealed recruiters’ discomfort and reluctance to explore patients’ decisions to decline the trial. These attitudes stemmed from concerns of being seen to coerce and the belief that patients’ views are fixed at the outset of consultations. We found no evidence of clear ‘fixed’ patient preferences in the consultations we listened to, but there were examples of patient expectations for chemotherapy/no chemotherapy, which had stemmed from previous interactions with clinicians (e.g. surgeons) prior to the first oncology consultation.
Appendix 9 The OPTIMA prelim guidance for recruiters
Appendix 10 Limitations of the qualitative research study methods
In addition to the limitations presented in Chapter 6, the discussion below relates to the specific limitations of the QRS methodology.
Data saturation was achieved within the interview study, as sampling was under the control of the QRS researcher. Such claims cannot be made for collection of audio-recorded consultations. It should be kept in mind that listening to audio-recorded consultations primarily served a practical purpose, and to this end, successfully identified challenges that recruiters were able to engage with in feedback meetings. However, the study would have benefited from a larger sample of audio-recordings, from a wider range of centres. Despite encouragement from the QRS team and TMG, recording of consultations did not occur as a matter of routine and the full range of interactions with patients was not captured. This is particularly true for second oncology consultations (where patients sometimes gave their decisions about participation). Incomplete recordings of each patient’s ‘journey’ made it difficult to track events, and made deciphering patients’ reasons for declining the trial problematic. In addition, limited numbers of audio-recordings for each individual recruiter restricted opportunities to assess the impact of QRS interventions through ‘before-and-after’ comparisons. Reluctance to routinely record consultations may have been an indirect consequence of recruiters perceiving the QRS as an ‘optional’ additional task. Future studies of this nature will need to frame the QRS as an integrated component of the trial.
The practices reported in interviews might have been influenced by recollection bias and/or the temptation to offer socially desirable answers. This is particularly true given the potentially sensitive nature of subjects discussed in consultations and the possibility of clinicians perceiving that their actions were under scrutiny. Similar sources of bias may also have influenced behaviours in consultations that were audio-recorded. In some cases, interviews focused on recruiters’ perceptions of why patients declined participation in OPTIMA prelim. Recruiters’ responses will have been based on their subjective interpretation of events, which may not have reflected reality. This consideration is also applicable to the data reported in screening logs, where recruiters were required to state the reason(s) why patients declined the OPTIMA prelim. Interviewing patients directly could have provided source triangulation (or contradictions), but the OPTIMA prelim QRS prioritised addressing the challenges already identified through recruiter interviews and audio-recorded consultations. There was an intention to interview patients if necessary, but given the exploratory nature of the QRS, the nature and extent of challenges identified early on could not be anticipated when the QRS was designed. Consideration should be given to interviewing patients if the OPTIMA main study proceeds.
Appendix 11 Qualitative research study profiles
The authors LR, SP and JLD are non-clinical (female) researchers employed by the University of Bristol (both currently and at the time of conducting data collection). LR and SP work within a team that specialises in identifying and addressing recruitment issues in challenging RCTs, which is led by JLD. At the time of interviews, LR (the main researcher undertaking data collection) had 1 year of post-doctoral experience, with over 4 years of training in the use of qualitative methodology. JLD, who conducted one interview, is a professor of social medicine with over 20 years of experience in the application of qualitative (among other) research methods.
LR approached this project as a naive researcher, with little knowledge of the field or literature. JLD and SP had prior experience of working on identifying recruitment challenges across a number of other RCTs, and thus had extensive knowledge of the field through their awareness of the literature and personal research. This prior knowledge may have influenced SP’s and JLD’s interpretation of data, although their contributions were largely based on LR’s initial interpretation and analytical thoughts, rather than raw data. SP’s prior knowledge may have influenced her double coding (conducted on 10% of transcripts), but analytical thoughts were largely similar to those expressed by LR (who was more likely to have a more purely inductive approach to analysis). Any differences in views were discussed and resolved in double-coding exercises. We have no reason to believe that any of the researchers’ personal experiences or life histories would have influenced their approaches to data collection and analysis in a noteworthy way.
Appendix 12 Qualitative research study prior relationship/contact with research participants
The authors LR and JLD had no prior contact or relationships with the majority of research participants prior to initiation of the OPTIMA prelim. There was one exception, where JLD had met one of the research participants during an academic meeting, which in turn prompted JLD’s collaboration in OPTIMA prelim. LR met a number of the research participants in advance of interviews at the launch meeting for OPTIMA prelim, but these encounters were limited to introductions.
Research participants all received study information sheets in advance of data collection processes, which explained why the research was being undertaken. Information sheets contained no personal details about the researchers or their personal aspirations. As such, interview participants will not have been informed of any personal information about the researchers prior to being interviewed, although they will have known the identity of the researchers (through e-mail correspondence), and thus had opportunity to read about researchers’ research interests in advance (e.g. via the internet). Although this was not explicit in interviews, there is a possibility that participants’ prior knowledge of researchers’ interests influenced their accounts and responses.
List of abbreviations
- ABCSG
- Austrian Breast and Colorectal Cancer Study Group
- AI
- aromatase inhibitor
- AQUA
- automated quantitative immunofluorescence
- ATAC
- Arimidex, Tamoxifen, Alone or in Combination trial
- BCI
- Breast Cancer IndexSM
- CfI
- chief investigator
- CI
- confidence interval
- CMF
- cyclophosphamide, methotrexate and fluorouracil
- COREQ
- consolidated criteria for reporting qualitative research checklist
- CRF
- case report form
- CTU
- Clinical Trials Unit
- E-CMF
- epirubicin, cyclophosphamide, methotrexate and fluorouracil
- EQ-5D
- European Quality of Life-5 Dimensions
- ER
- oestrogen receptor
- EVPPI
- expected value of perfect parameter information
- EVSI
- expected value of sample information
- FACT
- Functional Assessment of Cancer Therapy questionnaire
- FEC
- fluorouracil, epirubicin and cyclophosphamide (succeeding numbers refer to the dose of epirubicin)
- FEC-Pw
- fluorouracil, epirubicin, cyclophosphamide and weekly paclitaxel
- FEC-T
- fluorouracil, epirubicin, cyclophosphamide and docetaxel
- FFPE
- formalin-fixed paraffin-embedded
- FISH
- fluorescence in situ hybridisation
- GEP
- gene expression profiling
- HER2
- human epidermal growth factor receptor 2
- HRT
- oestrogen replacement therapy
- HTA
- Health Technology Assessment
- I-SPY
- Investigation of Series Studies to Predict Your Therapeutic Response With Imagine and Molecular Analysis trial
- ICER
- incremental cost-effectiveness ratio
- ICPV
- Independent Cancer Patients’ Voice
- IDFS
- invasive disease-free survival
- IQR
- interquartile range
- MammaTyper Int.
- MammaTyper Intermediate
- MDT
- multidisciplinary team
- MINDACT – EORTC 1004
- Microarray In Node-negative and 1- to 3-positive lymph node Disease may Avoid ChemoTherapy – European Organisation for Research and Treatment of Cancer Trial 1004
- mRNA
- messenger ribonucleic acid
- NICE
- National Institute for Health and Care Excellence
- NIHR
- National Institute for Health Research
- NPI
- Nottingham Prognostic Index
- NSABP
- National Surgical Adjuvant Breast and Bowel Project
- OICR
- Ontario Institute for Cancer Research
- ONC
- oncologist
- OPTIMA
- Optimal Personalised Treatment of early breast cancer usIng Multi-parameter Analysis trial
- PCR
- polymerase chain reaction
- PgR
- progesterone receptor
- PIS
- patient information sheet
- PPI
- patient and public involvement
- ProtecT
- Prostate testing for cancer and Treatment study
- QALY
- quality-adjusted life-year
- QRS
- qualitative recruitment study
- RCT
- randomised controlled trial
- RFS
- recurrence-free survival
- RN
- research nurse
- RNA
- ribonucleic acid
- ROR
- risk of recurrence
- ROR_PT
- risk of recurrence weighted for proliferation score and tumour size
- RS
- recurrence score
- RxPONDER
- The Rx for Positive Node, Endocrine Responsive Breast Cancer trial
- SD
- standard deviation
- SE
- standard error
- SWOG
- Southwest Oncology Group
- TAILORx
- The Trial Assigning IndividuaLized Options for Treatment (Rx)
- TC
- docetaxel and cyclophosphamide
- TEAM
- Tamoxifen Exemestane Adjuvant Multinational study
- TMA
- tissue microarray
- TMG
- Trial Management Group
- transATAC
- transitional cohort of the ATAC trial
- TSC
- Trial Steering Committee
- VAT
- value-added tax