Notes
Article history
The research reported in this issue of the journal was commissioned and funded by the Evidence Synthesis Programme on behalf of NICE as project number NIHR128968. The protocol was agreed in June 2019. The assessment report began editorial review in January 2019 and was accepted for publication in July 2020. The authors have been wholly responsible for all data collection, analysis and interpretation, and for writing up their work. The HTA editors and publisher have tried to ensure the accuracy of the authors’ report and would like to thank the reviewers for their constructive comments on the draft document. However, they do not accept liability for damages or losses arising from material published in this report.
Permissions
Copyright statement
Copyright © 2021 Edwards et al. This work was produced by Edwards et al. under the terms of a commissioning contract issued by the Secretary of State for Health and Social Care. This is an Open Access publication distributed under the terms of the Creative Commons Attribution CC BY 4.0 licence, which permits unrestricted use, distribution, reproduction and adaption in any medium and for any purpose provided that it is properly attributed. See: https://creativecommons.org/licenses/by/4.0/. For attribution the title, original author(s), the publication source – NIHR Journals Library, and the DOI of the publication must be cited.
2021 The authors
Chapter 1 Background and definition of the decision problem
Description of Crohn’s disease
Crohn’s disease (CD) is one of the two primary types of inflammatory bowel disease (IBD), the other being ulcerative colitis. 1–3 The symptoms of CD and ulcerative colitis are similar, and both types of IBD are characterised by inflammation of the gastrointestinal tract. CD is a lifelong condition that is characterised by recurring cycles of exacerbation (also referred to as flare) and remission, and for which there is no cure. The frequency of flare and the duration of remission are highly variable among those affected by CD. Some people are at a higher risk of following a more aggressive course of disease, typified by more frequent relapses and the manifestation of penetrating or stricturing complications. 1–3 Identifying those at a higher risk of developing complications of CD could lead to personalised management of an individual’s condition and to an improvement in clinical outcomes.
Aetiology, pathology and prognosis
Neither the underlying aetiology of CD nor the factors that determine the course and prognosis of the disease are fully understood. Environmental factors (e.g. smoking), genetic predisposition and dysregulation of the immune system are thought to play a role in the development and course of CD. 2,4
Crohn’s disease can affect any segment of the gastrointestinal tract from the mouth to the anus, but the most commonly affected areas are the distal ileum (the last part of the small intestine) and the colon. 5 CD that is primarily located in the colon often has a high symptom burden, whereas disease affecting the ileum can be extensive but is associated with relatively few symptoms. 6 Diseased segments of the gastrointestinal tract are frequently separated by intervening areas of healthy bowel tissue. 2,4 The size of the inflamed area may be limited to a few centimetres or it could affect an extensive part of the bowel. As well as affecting the lining of the gastrointestinal tract, CD may penetrate the wall of the bowel. 2,4
As CD can affect any part of the gastrointestinal tract, to differing extents, the symptoms experienced by people with the disease vary markedly, which can sometimes make recognition and diagnosis difficult. 2,4 Moreover, the symptoms and severity of the disease can change over time. People with CD most commonly present with:2,4,7
-
abdominal pain
-
diarrhoea (mucus, pus or blood may be mixed with the diarrhoea)
-
tiredness and fatigue
-
loss of appetite and weight loss
-
anaemia.
Crohn’s disease can also lead to signs and symptoms outside the gastrointestinal tract; these are known as extraintestinal manifestations and have been reported to be more common in CD primarily located in the colon. 6,7 Associated conditions typically occur during flare but can also manifest during remission or before the development of any signs of IBD. Conditions that develop as a result of CD include:7
-
arthritis (most commonly of the large joints of the arms and legs, including the elbows, wrists, knees and ankles)
-
skin problems (most commonly erythema nodosum)
-
eye problems (episcleritis, scleritis and uveitis)
-
liver problems (e.g. primary biliary cholangitis).
Flares of IBD indicate a return to active disease and, potentially, symptoms for an individual. Several factors have been proposed as triggers for flare, including poor adherence to treatment, certain medications (e.g. antibiotics and non-steroidal anti-inflammatory drugs), infection, smoking and emotional stress. 8,9 As has been noted for other immune-mediated diseases,10 the course of CD varies widely among affected individuals, making it challenging to predict the severity or frequency of flare occurrence.
As CD is not curable, the goal of management of the condition is to induce and maintain remission. Population-based studies investigating long-term prognosis of CD report that within the first year of diagnosis, 50–65% of people achieve remission and 15–25% experience a low level of disease activity. 11–13 However, 10–30% of people with CD have a relapse or an exacerbation of their condition in the first year. Long-term follow-up (i.e. 10–15 years) indicates that 67–73% of people with CD experience a chronic relapsing course and 13–20% have a chronic disease course with continuous activity. By contrast, 10–13% of those with CD achieve remission for several years. Among those with CD in remission after treatment, relapse rates at 1, 2, 5 and 10 years are estimated at 20%, 40%, 67% and 76%, respectively. 14
Those who develop CD that follows a non-severe course might achieve prolonged remission with no treatment. In contrast to a non-severe course of CD, those people characterised as following a severe course are likely to experience more frequent flares and typically require early aggressive treatment strategies, including multiple treatment escalations and augmentation. People with severe forms of CD are at a high risk of complications of disease, including intestinal obstruction, fistulae and perianal disease, as well as progressive disability and the need for surgery. 2,4,7
The prognostic factors associated with a more complicated, severe course of CD include bowel damage, extraintestinal manifestations of disease, larger number of flares, need for glucocorticoids, and resultant hospitalisations. 15 Other risk factors for a severe course of disease include smoking and fistula formation. Factors present at CD diagnosis that are found to be associated with a worse prognosis are young age (< 40 years), the presence of perianal disease and an initial need for glucocorticosteroid treatment. 16 The presence of known risk factors for flare and for complications in CD could influence the treating clinician’s management of the condition, but consensus on using risk factors to determine the prognosis of disease is yet to be achieved and treatment can vary.
Epidemiology
Crohn’s disease can appear at any age, but it is most often diagnosed in adolescents and adults between the ages of 20 and 30 years, with a second, albeit smaller, peak in diagnosis between the ages of 60 and 80 years. 17 In the UK, it is estimated that CD affects 1 in every 650 people7 and that at least 115,000 people have the condition. 4 The incidence and prevalence of CD have been rising since the mid-1970s, with the highest rates observed in northern Europe and North America. 18 The incidence of CD in the UK is reported to be about 8 per 100,000 people per year,19,20 with an age- and sex-adjusted point prevalence of 144.8 per 100,000 people. 20
Impact of Crohn’s disease
Affecting men and women equally, CD is a debilitating disease that has a marked impact on physical and emotional health, as well as quality of life. Additionally, CD is associated with a high economic burden as a result of disability, loss of work productivity, surgery and hospitalisation. 21 A UK study22 published in 2015 estimated the annual cost of care for a person with CD to be £6156 (£1800 for those in remission, compared with £10,513 for those experiencing relapse), translating to a total UK annual cost of ≈ £700M. Five years after onset, 15–20% of people are affected by their disease to some degree, and between 50% and 80% of people with CD will eventually need surgery as a result of, for example, the development of strictures, perforation of the bowel or failure of drug therapy. 23
Current diagnostic and treatment pathways
Identification of those at risk of following a severe course of Crohn’s disease
As highlighted in Aetiology, pathology and prognosis, the symptoms of CD are common to various conditions, which makes diagnosis challenging. The diagnosis and determination of the extent of CD is reached through a combination of clinical examination, laboratory tests, radiological imaging and endoscopy. 24 Furthermore, once a diagnosis of CD has been made, no validated test or algorithm is available to stratify people with CD by their risk of developing complications of the disease.
Standard laboratory investigations for a person suspected of having CD include an assessment of full blood count, inflammatory markers (e.g. C-reactive protein and faecal calprotectin), electrolytes and liver enzymes, as well as a microbiological analysis of a stool sample. 24 Although raised inflammatory markers are not specific to IBD, and identification does not differentiate IBD from infectious colitis, high C-reactive protein levels are broadly correlated with the severity of disease activity in CD and can be used to monitor disease progression.
Guidelines25 suggest that, once a diagnosis of CD has been established, subsequent investigations focus on assessing the level of disease activity, as well as the risk of complications in the longer term. Three key areas are assessed when determining the severity of CD: the impact of the disease on the individual (e.g. clinical symptoms, quality of life, fatigue and disability), the burden of the disease (e.g. mucosal lesions, upper gastrointestinal involvement and disease extent) and the course of the disease (e.g. structural damage, perianal disease, number of flares and extraintestinal manifestations). 26
Two clinical tools that are available to assess the level of disease activity are the Crohn’s Disease Activity Index (CDAI)27 and the Harvey–Bradshaw Index (HBI). 28 The HBI is a simple derivative of the CDAI and the two tools are correlated, with a change in the CDAI of 100 points corresponding to a 3-point change in the HBI. 29 Clinical experts commented that, in clinical practice, their preference is the HBI, as the CDAI is impractical for routine clinical assessment and its use is typically limited to clinical trials. Severity of disease activity is categorised as:16
-
clinical remission – a CDAI score of < 150, which corresponds to a HBI score of ≤ 4
-
mild – a CDAI score of 150–220, which corresponds to a HBI score of 4–8
-
moderate to severe – a CDAI score of 221–450, which corresponds to a HBI score of ≥ 8
-
severe fulminant disease – a CDAI score of > 450, which corresponds to a HBI score of ≥ 15.
The activity and severity of CD could be considered a continuum, and some people might not be easily categorised based on their symptoms. Moreover, the CDAI and HBI are based on subjective measures, and there is a move to use more objective parameters and the presence or absence of bowel destruction to assess severity. 25 Using patient-reported outcomes to assess disease activity in CD is also becoming more common. Often used to guide treatment recommendations, the CDAI and HBI scores represent status of activity at one point in time and do not account for the long-term prognosis or course of disease. 15
Endoscopic assessments and biopsies provide data on the level of disease activity in CD but do not provide an insight into factors associated with the relapse and course of the disease. Evaluating blood- and stool-based biomarkers of inflammation, such as C-reactive protein and faecal calprotectin, respectively, is less invasive than endoscopy and such laboratory tests provide reproducible, quantitative results that, together with clinical assessment, can aid clinicians in the diagnosis and management of CD. However, serum and faecal biomarkers are not necessarily specific to CD and they have limited applications in the prediction of the severity of the course of IBD, including CD, in the longer term. 30 There is no consensus or algorithm available outlining how to combine known risk factors to determine the long-term prognosis of CD, and the estimation of the risk of following a severe course of disease is based on subjective clinical judgement together with input from the patient.
Management of Crohn’s disease
The goal of treatment in CD is initially to control or reduce symptoms to induce remission. 31 Once symptoms are under control, maintenance treatment might be given to prolong remission and minimise the risk of relapse. Globally, two pharmacological treatment algorithms are followed in the management of active CD – the ‘step-up’ (SU) and ‘top-down’ (TD) approaches (Figure 1) – both of which involve several tiers of medication and, as the names suggest, are the inverse of each other. 32 Additionally, surgery might be necessary at any stage of the disease but can be considered as an alternative to medical treatment in some people, particularly those in whom the disease is limited to the distal ileum. 31
FIGURE 1.
‘Step-up’ vs. ‘top-down’ treatment algorithms for CD. 5-ASA, 5-aminosalicylate. Note that in the treatment hierarchy shown in the pyramid, the most potent drug therapies are placed at the top.
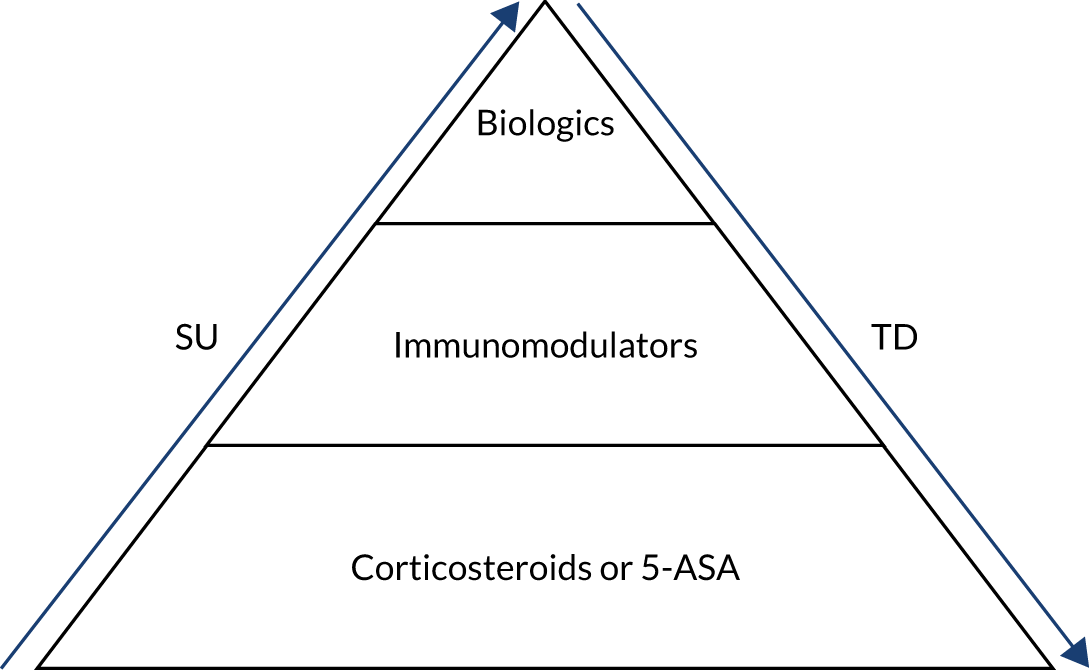
Currently, National Institute for Health and Care Excellence (NICE) guideline 12931 recommends a SU approach for the medical management of CD. The SU algorithm (see ‘Step-up’ approach) involves starting treatment with the least aggressive medical option available and escalating therapy in reactive stepwise stages in response to recurrent flares or persistently active disease. An alternative treatment path involves an ‘accelerated SU’ plan in which patients who are considered to have more severe disease or who have clinical markers of poor outcome advance rapidly up the treatment ladder, receiving earlier aggressive therapy than those with non-severe disease. The Evidence Assessment Group’s (EAG’s) clinical experts advised that, for those people judged to be at risk of a more severe clinical course (e.g. extensive small bowel disease, perianal disease or upper gastrointestinal disease), most clinicians would prefer to take an ‘accelerated SU’ approach rather than follow the slower, conventional SU algorithm.
The TD approach (see ‘Top-down’ approach) was not recommended by NICE at the time of writing. 31 The strategy involves treatment earlier in the pathway with biological therapies, which are more clinically effective but are also potentially associated with a greater risk of adverse effects (e.g. increased rate of infection and malignancy). 33 The early use of biological therapies in a TD approach is thought to modify the course of CD, to increase the possibility of mucosal healing (preventing structural damage of the bowel), and to be more effective than the SU approach at inducing and prolonging remission;32 the goal of achieving mucosal healing during treatment is gaining acceptance but is not yet part of standard care in the UK.
Another challenge in the management of CD is the timing of treatment de-escalation, which can be defined as either decreasing the dose of a drug or completely ceasing therapy. De-escalation of therapy in both the SU and the TD strategies is typically considered when a person achieves deep remission, which comprises clinical and biological remission. De-escalation is proposed for those at highest risk of potential complications of treatment, such as infection or malignancy, or for those at lowest risk of relapse after the cessation of treatment. De-escalation might not be appropriate for all those achieving deep remission. Factors that need to be accounted for when considering de-escalation of therapy include age, sex, treatments given and severity of CD. 34 A systematic review34 evaluating de-escalating anti-tumour necrosis factor (TNF) or immunomodulator (IM) therapy in people with CD who were in deep remission for at least 6 months found that de-escalating medical therapy in this cohort was appropriate for a small proportion of carefully selected people only, predominantly the elderly and those with non-severe disease.
Neither the SU nor the TD approach is suitable for all people with CD. Considering the risk–benefit profile of the TD approach, some clinicians could be reticent to expose those with mild activity of CD at the time of assessment or those thought to be at low risk of experiencing a relapse to the unnecessary risk of an adverse effect. Conversely, those assessed as at risk of experiencing a severe course of disease are also at risk of undertreatment if the conventional SU approach is followed, with consequent prolongation of symptoms and the inadequate control of disease activity, and the associated long-term risks. Another consideration is cost of treatment; the TD approach is typically more expensive than the SU approach. 33
The ability to easily stratify those with CD by risk of course of disease could help identify the most appropriate treatment strategy for each patient.
‘Step-up’ approach
NICE guideline 12931 advises starting treatment with a glucocorticosteroid [prednisolone, methylprednisolone or intravenous hydrocortisone (for inpatients)] to induce remission in those with a first presentation or a single inflammatory exacerbation of CD in a 12-month period. For those with mild disease who cannot tolerate or who are contraindicated to the recommended glucocorticosteroids, alternative treatments for first presentation or a single inflammatory exacerbation in 12 months are budesonide (another glucocorticosteroid) and 5-aminosalicylate (5-ASA). Additionally, budesonide can be considered for those who have one or more of distal ileal, ileocaecal or right-sided colonic disease. For children or young people for whom there is a concern about growth or adverse effects, NICE advises considering enteral nutrition as an alternative to a conventional glucocorticosteroid. 31
Both budesonide and 5-ASA are less effective than the preferred initial treatment of glucocorticosteroids, but they might be associated with fewer adverse effects; clinical experts advise that, increasingly, 5-ASA is considered to have a limited role in the management of CD. Budesonide should not be considered for those presenting with severe disease activity or exacerbations.
Should remission not be achieved after induction therapy, the next step in the treatment pathway is the addition of an IM (azathioprine, mercaptopurine or methotrexate) to conventional glucocorticosteroid or budesonide, specifically in cases where:31
-
a person experiences two or more inflammatory exacerbations in a 12-month period or
-
the glucocorticosteroid dose cannot be tapered.
NICE cautions that before offering a patient azathioprine or mercaptopurine, thiopurine methyltransferase activity should be assessed. Azathioprine or mercaptopurine should not be offered when a patient’s thiopurine methyltransferase activity is deficient (very low or absent) and a lower dose of both IMs should be considered if thiopurine methyltransferase activity is below normal but not deficient (according to local laboratory reference values). Alternatively, if it is thought that the patient would be unable to tolerate mercaptopurine or azathioprine, the addition of methotrexate could be considered.
For adults with severe active CD whose disease has not responded to conventional therapy (including IM and/or glucocorticosteroid treatments), or who are intolerant of or have contraindications to conventional treatment, the recommended therapy is escalation to infliximab or adalimumab within their licensed indications; both of these are TNF-alpha inhibitors. 31 Biosimilars of infliximab and adalimumab are available and can be used interchangeably with originator anti-TNFs in clinical practice. Infliximab and adalimumab can be administered alone or in combination with an IM, and the therapies should be given as a planned course until treatment failure (including the need for surgery) or 12 months after the start of treatment, whichever is earlier. Treatment with infliximab or adalimumab could be continued if there is clear evidence of ongoing active disease as determined by clinical symptoms, biological markers and further investigation, including endoscopy, if necessary. However, NICE advises that disease activity should be reassessed at least every 12 months to determine whether continued treatment with infliximab or adalimumab is still clinically appropriate. People whose CD relapses on cessation of treatment with biological therapy should have the option to recommence treatment with infliximab or adalimumab.
For those with moderately to severely active CD in whom treatment with a TNF-alpha inhibitor has failed (i.e. the disease has responded inadequately or lost response to treatment), or who are intolerant to conventional therapies and are contraindicated to anti-TNFs, other biologics, such as vedolizumab (Entyvio®, Takeda Pharmeceutical Company, Tokyo, Japan) and ustekinumab (STELARA®, Janssen-Cilag, Beerse, Belgium), are additional treatment options. 31
Once a person affected by CD achieves remission, NICE advises discussing with them, together with their family members or carers, the options for managing their condition, one of which may be no further treatment. 31 For those who choose to proceed with therapy to maintain remission, the available options are:
-
azathioprine or mercaptopurine as monotherapy to maintain remission when previously used with glucocorticosteroids (including budesonide) to induce remission and for those who have not previously received these drugs
-
methotrexate –
-
for people who required methotrexate to induce remission
-
for people who tried but could not tolerate azathioprine or mercaptopurine for maintenance
-
for people contraindicated to azathioprine or mercaptopurine.
-
-
continued treatment with biological therapy, if appropriate.
‘Top-down’ approach
Although the ‘top-down’ approach is not recommended by NICE, clinicians in specialist centres might choose to offer the strategy to those they consider to have a poor prognosis in terms of outcomes, for example those with complex perianal disease, significant fistulising disease or multiple risk factors. No accepted treatment strategy is available for the TD approach, with disparity in the definition of ‘aggressive’ therapy across studies. TD can involve the early use of biological therapies or of IMs, or a combination of biological therapy and IMs. In two landmark studies35 evaluating the clinical efficacy of early aggressive therapy in those with CD, ‘top-down’ treatment comprised infliximab in combination with azathioprine. However, evidence in support of the effectiveness of the TD approach when it is compared directly with the SU approach is inconsistent,33 with two studies35,36 finding a benefit of early treatment with biologics and one study37 reporting no benefit of early treatment with biologics over the less aggressive strategy. Variation in results across studies could be related to differences in, for example, the definition of ‘early’ intervention and in trial design, outcomes measured, population and trial duration.
Being able to better predict the course of CD would help clinicians to identify those who could benefit most from the early use of aggressive treatments (IMs and biological therapies) and to decide on the most appropriate treatment to manage symptoms. Tools such as the PredictSURE-IBDTM (PredictImmune Ltd, Cambridge, UK) and IBDX® (Crohn’s disease Prognosis Test; Glycominds Ltd, Lod, Israel) could potentially help achieve the goal of personalising treatment for those with CD.
Description of the technologies under assessment
IBDX
Glycominds envisages that the IBDX tool can be implemented at three key stages in the management of CD:
-
on differential diagnosis of CD from ulcerative colitis
-
to assess the risk of developing a more aggressive disease course in those diagnosed with CD who have not yet experienced complications and/or undergone surgery
-
to predict the risk of future events in those who have experienced a first CD complication or surgery.
The IBDX tool detects serum levels of specific anti-glycan antibodies, which are a set of serological biomarkers reported to be highly specific to CD with a potential predictive value for severe course of disease. 38 Glycans are saccharides that can be attached to various biological molecules through an enzymatic process called glycosylation. Most glycans are found on the exterior of cell walls and they form the main components of the cell wall surface in many microbes, including fungi, yeast and bacteria. 38
An atypical interaction of environmental, genetic and microbial factors with the immune system is thought to lead to the production of antibodies against intestinal microorganisms in those with CD that results in the gastrointestinal inflammation typical of the condition. 39,40 Examples of microbial antibodies include anti-Saccharomyces cerevisiae antibodies (ASCA; also referred to as gASCA), antibodies against Pseudomonas-associated sequence I2 (anti-I2), and antibodies against the bacterial flagellin cBir1 (anti-cBir1). 41 Anti-glycan antibodies comprise antibodies against ASCA, anti-mannobioside antibodies (AMCA), anti-laminaribioside antibodies (ALCA), anti-chitobioside carbohydrate antibodies (ACCA), anti-laminarin antibody (anti-L) and anti-chitin antibody (anti-C).
Antibodies detected by the IBDX tool include:42
-
ACCA
-
ALCA
-
AMCA
-
gASCA
-
anti-L
-
anti-C.
The IBDX tool is supplied as a set of six biomarker kits (listed above), each of which detects a circulating antibody against the kit-specific antigen in patient serum or plasma by an indirect solid-phase enzyme-linked immunosorbent assay (ELISA). Individual kits contain the relevant antiglycan 96-well microplate (12 × eight-well strips), ELISA reagents, negative control, positive control and calibrators. 43 Each kit can assess up to 90 samples, excluding controls, but the company recommends running samples in duplicate (i.e. a maximum of 45 assays per kit, accounting for controls). The microwell plates, conjugates and controls are specific to each kit, but all other reagents are the same. All kits follow the same procedure (including incubation times), so they can easily be processed at the same time, if desired. On completion of incubation, absorbance of the calibrator, controls and samples can be evaluated spectrophotometrically. Optical density is directly proportional to the amount of bound antibody. Arbitrary units are calculated based on sample optical density and calibrator serum sample optical density. 43 The positivity of each biomarker is assessed based on the cut-off values presented in Table 1.
gASCA | ACCA | ALCA | AMCA | Anti-C | Anti-L | |
---|---|---|---|---|---|---|
Negative | < 45 | < 80 | < 55 | < 90 | < 45 | < 45 |
Equivocala | 45–50 | 80–90 | 55–60 | 90–100 | 45–50 | 45–50 |
Positive | > 50 | > 90 | > 60 | > 100 | > 50 | > 50 |
Those people with CD are considered to be at greater risk for disease complication (stricturing or penetrating) or surgery intervention if they are positive for two or more serological markers. 42 Figure 2 presents a flow chart (adapted from that available in the instructions for the IBDX kit43) summarising how to interpret the complete panel of results from the individual biomarkers.
FIGURE 2.
Overview of interpretation of results from individual IBDX ELISA kits [adapted from the IBDX® CE MARK kit insert with permission from Glycominds Diagnostics Ltd (2014)43].
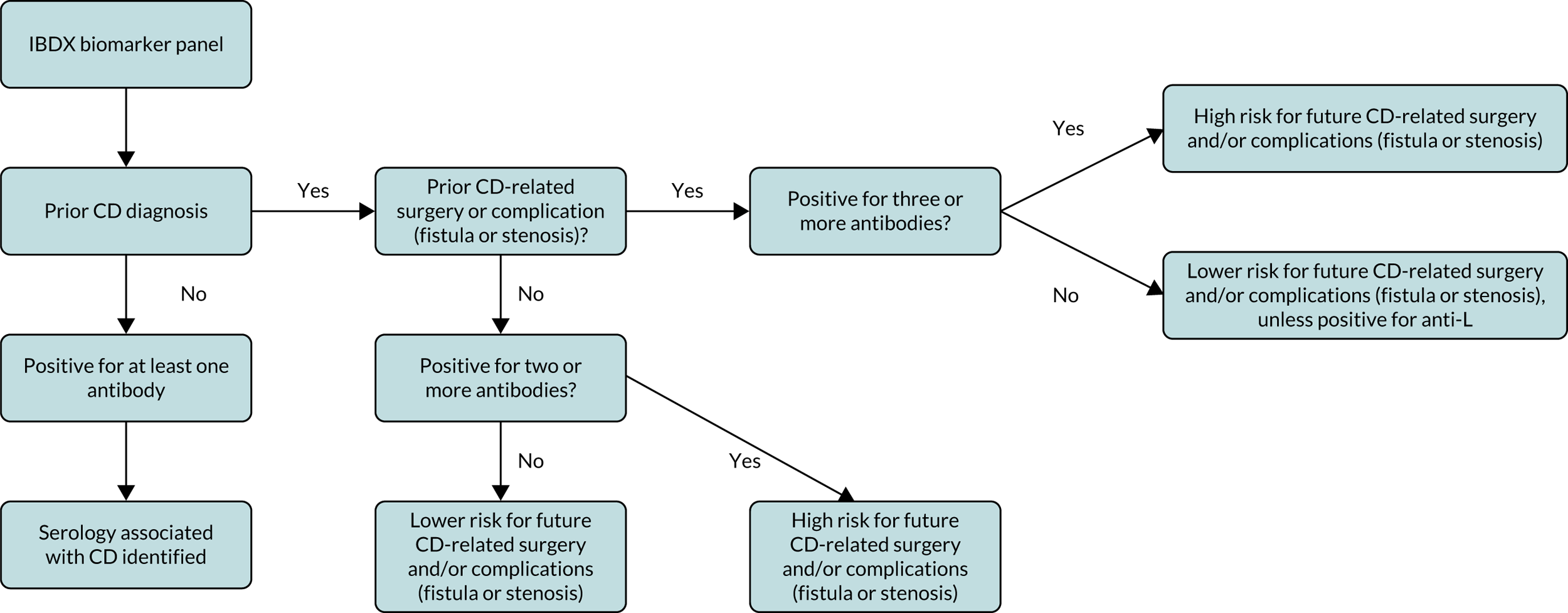
The company highlights that anti-glycan antibodies are also detected at the time of diagnosis in people with coeliac disease. However, as noted by the company, initial positivity for various anti-glycan antibodies is lost after people with coeliac disease follow a long-term gluten-free diet. 44 Coeliac disease and IBD can be comorbid, and studies suggest that people with IBD are at an increased risk of coeliac disease. 45 Therefore, the company recommends against using the IBDX kit without exclusion of diagnosis of coeliac disease in those who have not followed a gluten-free diet. The EAG’s clinical experts fed back that, as the symptoms of CD and coeliac disease overlap, most people referred with suspicion of CD are likely to be tested for coeliac disease, which necessitates a blood test. The EAG’s clinical experts commented that the tests for the risk of severe course of CD and for the presence of coeliac disease could be carried out simultaneously.
PredictSURE-IBD
PredictSURE-IBD is proposed for use in adults (aged ≥ 16 years) with IBD, including CD, who have active disease and are not receiving concomitant glucocorticosteroids, IMs or biological therapies. PredictSURE-IBD could be particularly beneficial for people with:
-
newly or recently diagnosed IBD
-
moderate or severe active IBD (people with mild disease are unlikely to receive early aggressive treatment with biologics)
-
disease that would not require early aggressive treatment with biologics (i.e. the ‘top-down’ approach) with current standard care in the NHS (e.g. people who do not have fistulising and/or complex perianal CD or multiple risk factors).
PredictSURE-IBD facilitates the stratification of people with IBD into high and low risk of a frequently relapsing course of disease through the detection of a gene sequence associated with CD8+ (cluster of differentiation 8) T-cell exhaustion.
Gene expression profiling of peripheral blood CD8+ T cells identified a signature gene sequence that was associated with CD8+ T-cell exhaustion,46–48 a state that is reached through the stepwise and progressive loss of T-cell function and that inhibits the immune response. 49 The level of expression of the genes indicating CD8+ T-cell exhaustion was found to be linked to the course of disease in multiple autoimmune diseases, including IBD. 46–48 People with a CD8+ T-cell signature not associated with T-cell exhaustion were shown to be at a higher risk of a frequently relapsing disease course than those with the signature for T-cell exhaustion. 46–48
The PredictSURE-IBD test determines the presence or absence of the signature gene sequence (15 target genes and two control genes;50 Table 2) indicating CD8+ T-cell exhaustion through in vitro reverse transcription-quantitative polymerase chain reaction (RT-qPCR) of messenger ribonucleic acid (mRNA) isolated from a whole blood sample (2.5 ml). The blood sample must be taken by a trained professional and stored in a sample tube (PAXgene® Blood RNA Tube, PreAnalytiX GmbH, Hombrechtikon, Switzerland); the vessel for the blood sample is not supplied as a component of the PredictSURE-IBD test kit and must be purchased separately. The isolation of mRNA and subsequent RT-qPCR are carried out in a centralised laboratory (Clinical Genetics Laboratory, Addenbrooke’s Treatment Centre, Cambridge University Hospitals NHS Foundation Trust).
Gene ID | Gene name |
---|---|
FCRL5 | Fc receptor-like 5 |
GBP5 | Guanylate-binding protein 5 |
GZMH | Granzyme H |
GZMK | Granzyme K |
HP | Haptoglobin |
IFI44L | Interferon-induced protein 44 like |
IL18RAP | Interleukin-18 receptor accessory protein |
LGALSL | Lectin, galactoside-binding-like protein |
LINC01136 | Long intergenic non-protein coding RNA 1136 |
LY96 | Lymphocyte antigen 96 |
NUDT7 | Nudix (nucleoside diphosphate-linked moiety X)-type motif 7 |
P2RY14 | Purinergic receptor P2Y, G-protein coupled, 14 |
TRGC2/TRGJ1 | T-cell receptor gamma constant 2/T-cell receptor gamma joining 1 |
TRGV3 | T-cell receptor gamma variable 3 |
VTRNA1-1 | Vault RNA 1-1 |
In RT-qPCR, because the starting genetic material is RNA rather than deoxyribonucleic acid (DNA), the first step in the process necessitates the transcription of mRNA into complementary DNA (cDNA) using reverse transcriptase. Next, the cDNA acts as the template for quantitative polymerase chain reaction (qPCR) for DNA amplification. qPCR is carried out in a 384-well plate (16 × 24 wells). Given the requirements for quality control of the assay, a maximum of four samples can be analysed per plate. Each sample of cDNA is amplified in triplicate, which requires 12 rows of the plate. A quality control RNA [supplied as part of the PredictSURE-IBD kit and run in triplicate (three rows)] and a no-RNA control [run singularly (one row)] are tested with each batch of mRNA samples to validate the run. The centralised laboratory uses a LightCycler® 480/480 II platform (Roche Life Sciences, Roche Diagnostics, Hertford, UK), which is a standard platform, to carry out reverse transcription polymerase chain reaction (RT-PCR). Staff training to process the PredictSURE-IBD kits will not be required at the centralised laboratory as the site already provides testing services as part of an ongoing study [PROFILE51 (PRedicting Outcomes For Crohn’s dIsease using a moLecular biomarkEr)]. If required, PredictImmune would support staff training at additional laboratories to facilitate the expansion of testing, with training thought to require 2–3 days at each centre. 52
The results from RT-qPCR are fed into a proprietary algorithm that calculates a continuous risk score and, based on this score, patients are categorised as at high or low risk of following a frequently relapsing form of IBD. A confidence level associated with the result is also reported and presented as a percentage. The turnaround time for the test is 7–10 days.
Comparator
As no validated tool or algorithm is available to determine the course of CD, the relevant comparator is standard clinical care in the NHS.
Reference standard
As no test or algorithm is available to determine the long-term course of disease or an individual’s risk of developing severe course of disease, the estimation of prognosis is based on the subjective clinical judgement of presenting signs and symptoms, together with the potential risk factors for a severe course of the disease. Thus, there is no reference standard for the tools under evaluation.
Aim of the assessment
The aim of this diagnostic assessment review is to assess the prognostic test accuracy, clinical effectiveness and cost-effectiveness of two molecular prognostic tools for IBD in identifying people at high risk of a severe course of CD. The tools assessed in the review reported here are IBDX and PredictSURE-IBD. At the time of writing, no validated test or algorithm is available to stratify people with CD by risk of developing complications of disease. The presence of known risk factors for flare and for complications in CD could influence the treating clinician’s management of the condition, but consensus on using risk factors to determine the prognosis of disease is yet to be achieved and treatment can vary. The accuracy, clinical effectiveness and cost-effectiveness of the tools will be evaluated against standard clinical care in the NHS, based on input from clinical advisors, when assessing the likely course of CD.
Chapter 2 Methods for assessing clinical effectiveness
This report contains reference to confidential information provided as part of the NICE Diagnostic Assessment process. This information has been removed from the report and the results, discussions and conclusions of the report do not include the confidential information.
A systematic literature review was carried out to evaluate, first, the prognostic test accuracy of IBDX53 and PredictSURE-IBD54 tools in the identification of those at high risk versus low risk of developing a severe course of CD; and, second, the clinical impact of using these tools in the management of CD.
Methods for the systematic review were in line with those reported in a prespecified protocol that was registered on the international prospective register of systematic reviews (PROSPERO CRD4201913873755). The general principles followed were those outlined in the Centre for Reviews and Dissemination (CRD) guidance for conducting reviews in health care,56 NICE’s Diagnostics Assessment Programme Manual57 and the Cochrane handbook for systematic reviews of diagnostic test accuracy. 58 The systematic review is reported in accordance with the Preferred Reporting Items for Systematic Reviews and Meta-Analyses (PRISMA) checklist for diagnostic test accuracy studies. See Report Supplementary Material 1 for the PRISMA-diagnostic test accuracy checklist and PRISMA-diagnostic test accuracy for abstracts checklist.
Search strategies
Search strategies for electronic databases were designed with a focus on the target condition of the systematic review (i.e. CD) and the specified prognostic tools (i.e. IBDX and PredictSURE-IBD). Strategies comprised a combination of medical subject heading (MeSH) terms and free-text terms. During the scoping search process, no record was retrieved using the term ‘PredictSURE-IBD’ or any appropriate derivative, and it was noted that terms including trade names of the prognostic tools must be combined with ‘or’ to avoid the omission of known potentially relevant studies. Names for the prognostic tools of interest, and relevant alternative terms, were included in consideration of future updates. No study design filters were applied, and all electronic databases were searched from inception to 14 June 2019. See Report Supplementary Material 2 for the search strategies applied in electronic databases to retrieve records on studies evaluating prognostic accuracy and the impact of using the tools on the management of CD.
The records retrieved from electronic databases were uploaded to and deduplicated in EndNote X7 software [Clarivate Analytics (formerly Thomson Reuters), Philadelphia, PA, USA]. The deduplicated list of records was exported to Rayyan QCRI (Doha, Qatar; https://rayyan.qcri.org/), which was used to co-ordinate the assessment of titles and abstracts by two independent reviewers. The reference lists of relevant systematic reviews and eligible studies were searched by hand to identify additional potentially relevant studies.
Data submitted by the manufacturers of the two prognostic tools that are the focus of this assessment were considered for inclusion in the review.
Electronic databases searched for relevant studies were:
-
MEDLINE (MEDLINE and Epub Ahead of Print, In-Process & Other Non-Indexed Citations and Daily and Versions; via Ovid)
-
EMBASE (via Ovid)
-
the Cochrane Central Register of Controlled Trials (CENTRAL) and Cochrane Database of Systematic Reviews (CDSR).
The following clinical trial registers were searched to identify relevant ongoing clinical trials that, when completed, may have an impact on the results of this review:
-
World Health Organization International Clinical Trials Registry Platform
-
ClinicalTrials.gov.
The website of the US Food and Drug Administration was also searched to identify unpublished data.
Abstracts from key conference proceedings from the past 2 years were screened for additional potentially relevant studies. Conferences that clinical experts identified as being of importance to the assessment were those organised by:
-
British Society of Gastroenterology
-
European Crohn’s and Colitis Organisation
-
Digestive Disease Week®
-
United European Gastroenterology.
Eligibility criteria
Eligibility criteria for the inclusion of studies assessing the prognostic test accuracy or clinical impact of the tools that are the focus of this assessment are presented in Table 3.
Aspect of review | Eligibility criteria | |
---|---|---|
Population | Those with active CD and a diagnosis of disease | |
Prognostic tests (interventions) | IBDX and PredictSURE-IBD | |
Prognostic test accuracy | Clinical impact | |
Comparator | No comparator or comparison of the prognostic tool and clinical judgement vs. clinical judgement alone of high risk of following a severe course of CD | |
Reference standard | Not applicable | Standard care in the NHS |
Outcomes | Prognostic test accuracy:
|
Outcomes are of interest in the subgroups of those assessed as being at high risk vs. not being at high risk of following a severe course of CD:
|
Considering study design, based on scoping searches, and given that the interventions are prognostic tools, the retrieval of relevant randomised controlled trials (RCTs) was deemed to be unlikely. Thus, to ensure that all relevant studies were captured, no limit was applied to study design, with the exception that studies had to be carried out in humans, and had to not be an opinion piece (i.e. an editorial). Studies analysing the clinical validity (the ability of the test to reliably and accurately identify the biomarkers of interest or to determine the risk of developing severe compared with non-severe course of CD) or clinical utility (the ability of the test to improve measurable clinical outcomes, and its usefulness and added value to patient management) of the prognostic tool were eligible for inclusion. Studies evaluating analytical validity were included, where applicable, where analytical validity denotes the ability of the tool to accurately and reliably measure the biomarker of interest as assessed using laboratory tests on samples that are representative of those with CD. Studies not published in the English language were eligible if sufficient relevant data could be extracted from the full-text publication in a language other than English or from an English-language abstract.
For the IBDX tool, to be included a study had to assess all six biomarkers included in the panel:42
-
ACCA
-
ALCA
-
AMCA
-
gASCA
-
anti-L
-
anti-C.
Study selection
First, two reviewers independently assessed the titles and abstracts of studies retrieved from the electronic database searches for potential relevance according to the prespecified eligibility criteria (see Table 3). When consensus could not be achieved, the full texts of potentially relevant studies were ordered. Next, full-text copies of potentially relevant studies were obtained and assessed independently by two reviewers for inclusion against the prespecified eligibility criteria. Any disagreements were resolved by discussion or through consultation with a third reviewer, if necessary.
Data extraction
After a standardised data extraction form was created (including a pilot process), data were extracted by one reviewer and independently checked for accuracy by a second reviewer. Discrepancies were resolved by discussion, with the involvement of a third reviewer when necessary. The information that was extracted included details of the study’s design and methodology, intervention and comparator tests, reference standard, relevant baseline characteristics of participants (e.g. duration of CD, location of CD and presence of complications) and outcome measures, including clinical outcome efficacy and any adverse events (see Table 3). The companies producing the prognostic tests and the corresponding authors of the studies selected for assessment of test accuracy were, when necessary, contacted for missing data or clarification of the data presented.
Quality assessment
In a change from the prespecified protocol, taking into account reviewer feedback and a review of the available checklists, the quality of prognostic test accuracy studies was assessed using the QUIPS59,60 (Quality In Prognosis Studies) tool, rather than the PROBAST (Prediction model Risk Of Bias ASsessment Tool) as originally planned. 61,62 The quality of clinical effectiveness studies was to be assessed based on the study design: RCTs were to be assessed using the Cochrane Risk of Bias Tool;63 non-randomised studies were to be assessed using the Risk Of Bias In Non-randomised Studies-of Interventions (ROBINS-I) tool;64 and qualitative studies were to be assessed using the Critical Appraisal Skills Programme (CASP) tool. 65 However, all studies identified as relevant to the systematic review were prognostic accuracy studies. All quality appraisal assessments were carried out by one reviewer and verified by another reviewer independently.
Methods of analysis and evidence synthesis
Details of results on the accuracy of the prognostic tests and potential impact of their use on clinical outcomes, together with quality assessment for each included study, are presented in structured tables and as a narrative summary. The heterogeneity identified across studies associated with clinical (e.g. baseline characteristics and reported outcomes) characteristics and methodological (e.g. different study designs and limited reporting of data) characteristics precluded quantitative synthesis of the data. For prognostic accuracy, positive predictive values, negative predictive values, sensitivity values and specificity values, with 95% confidence intervals (CIs), are presented for each study, where available.
Potential subgroup analyses
Evidence permitting, the subgroups planned to be investigated were:
-
children with a diagnosis of CD compared with adults with a diagnosis of CD
-
newly diagnosed CD compared with established diagnosis of CD
-
mild activity of disease compared with moderate to severe activity of disease
-
presence fistulising or complex perianal disease compared with absence of fistulising or complex perianal disease.
Sensitivity analyses
The planned sensitivity analyses were to include studies deemed to be at high risk of bias that were excluded from the primary analyses. Sensitivity analyses stratified by risk of bias were not conducted, as a lack of sufficient data precluded such analysis.
Chapter 3 Results of the review of prognostic test accuracy and clinical impact
The sections that follow discuss the quantity and quality of evidence available, including the characteristics and risk of bias of the identified studies, retrieved through literature searches to identify data on the prognostic accuracy and clinical impact of PredictSURE-IBD and IBDX.
Quantity and quality of the available evidence
Results of the systematic literature search
Searches of electronic databases retrieved 6258 records (post deduplication) that were of possible relevance to the review (Figure 3). The initial screening of titles and abstracts led to the identification of 36 publications for review of full texts. Of the 36 articles evaluated, 16 publications, including systematic reviews, were deemed to be relevant to the review. 38,50,66–79 Four records (three full texts38,66,70 and one conference abstract68) provided details for three systematic reviews, the reference lists of which were screened for potentially relevant studies. Additionally, documents supplied by the companies marketing the prognostic tools were reviewed.
FIGURE 3.
The PRISMA flow chart.

Limited evidence is available from the included full-text publications on the prognostic accuracy of PredictSURE-IBD, and no evidence is available on the prognostic accuracy of IBDX, in identifying those at high risk of following a severe course of CD, as determined by measures such as sensitivity and specificity (the prognostic outcomes of interest listed in Table 3). Most of the evidence on the tools’ utility is derived from observational studies that report estimates of the risk of experiencing a clinical outcome associated with an aggressive course of CD, for example need for treatment escalation, development of a complication or surgery. Estimates are presented of an increased risk for those categorised, based on test results, as being at higher risk compared with those determined to be at lower risk of following a severe disease course. No study retrieved reported on the clinical impact of the use of IBDX or PredictSURE-IBD in terms of influencing the treatments given in the management of active CD.
The authors of two studies79,80 were contacted to verify that the kit used in their research was the IBDX tool and not a comparable kit produced by another company. One author confirmed that they had used a kit that was not captured in the scope of this review, and the study was therefore excluded from the review. 80
Summaries of the studies included in the review are presented by prognostic tool evaluated and key characteristics of studies (Table 4). See Report Supplementary Material 3 for a list of full-text publications screened but subsequently excluded (with reasons for exclusion) from the review.
Study (first author and year) | Design; country | Population | Number eligible for analysis | Duration of disease at time of test | Severity of disease at time of test | Outcomes reported |
---|---|---|---|---|---|---|
IBDX | ||||||
Harrell 201067 (conference abstract) | Unclear; unclear | People with CD | 172 | Not reported | Not reported | Association of individual antiglycan biomarkers with:
|
Paul 201569 (full publication) | Cross-sectional; France | People with IBD and a diagnosis for more than 1 year | 107 with CD | Median 9.4 (IQR 1–44) years | Not reported | Differentiating severe from non-severe course of disease |
Rieder 201075 (full publication); related publications73,77 | Prospective cohort; Germany | People with IBD, other GI disease and healthy controls | 363 with CD | Median 66.8 (IQR 11–141) months | Not reported | OR for:
|
Rieder 201076 (full publication) | Prospective cohort; Germany | People with CD and no prior complication or surgery | 76 | Median 10.6 (IQR 1.7–52.3) months | Not reported | Time to complication or surgery analysed by number of positive biomarkers (1, 2 or 3) |
Rieder 201272 (full publication); related publications71,73 | Cross-sectional; Germany | Children (aged < 18 years) with IBD and healthy controls | 59 with CD | Median 18.0 (IQR 12.0–43.0) months | Not reported | Need for CD-related surgery by number of positive biomarkers (1, 2 or 3) |
Seow 200978 (full publication) | Cross-sectional; Canada | People with IBD and healthy controls | 517 with CD | Median 8.9 (IQR 0.02–46.30) years | Not reported | Association of the number of positive biomarkers with key prognostic factors for severe course of disease and need for abdominal surgery |
Wolfel 201779 (conference abstract) | Prospective cohort; unclear | People with CD who had undergone one surgical resection | 118 | Not reported | Not reported | Time to repeat surgery |
PredictSURE-IBD | ||||||
aBiasci 201950 (full publication) | Prospective cohort; UK | People with active CD or UC and who were not receiving concomitant corticosteroids, IMs or biological therapy | 66 with CD (validation cohort) | 61 (92.4%) people were newly diagnosed with CD | Not reported |
|
Ongoing studies
From searches of prespecified sources, together with information supplied by the companies, ongoing studies were identified that were of potential relevance to the review, all of which assess the use of PredictSURE-IBD.
The PROFILE study is a prospective, multicentre randomised study set in the UK. 51 PROFILE has been designed to compare the clinical efficacy of TD and accelerated SU treatment regimens in people with newly diagnosed CD who have first been stratified into subgroups based on the risk of following a severe, relapsing course of CD (high vs. low risk) using the PredictSURE-IBD tool. Within the biomarker-stratified groups, people are randomised (1 : 1) to either TD or accelerated SU treatment. Treatment allocation is open label, but clinicians and patients are masked to subgroup classification. The authors propose that those designated as being at high risk of a severe course of CD will experience a greater benefit of receiving early TD treatment. Conversely, those likely to experience a more indolent course of disease could be managed with the accelerated SU approach and avoid the risk of adverse effects associated with biological therapies. Thus, a goal of the study is to determine whether or not using the PredictSURE-IBD tool can facilitate personalised therapy in CD and improve clinical outcomes. The primary outcome is the incidence of sustained surgery and glucocorticosteroid-free remission from the completion of induction treatment through to study completion (48 weeks). Recruitment began in December 2017, with a planned enrolment of 400 people, generating 100 people in each of the four groups. 51 The estimated end date for the trial listed on the ISRCTN (International Standard Randomised Controlled Trials Number) registry is March 2022. 81
PRECIOUS is a multicentre observational study based in the USA and sponsored by PredictImmune. 82 Set in referral centres and community hospitals, PRECIOUS (Predicting Crohn’s and Colitis Outcomes in the United States) is designed to assess the efficacy of the PredictSURE-IBD tool in stratifying those newly diagnosed with active IBD, including CD, into cohorts at high or low risk of following an aggressive disease course requiring frequent treatment escalations. Patients’ blood will be collected at enrolment and will be tested with PredictSURE-IBD at a later date. Ideally, participants will be treatment naive. Those enrolled will receive treatment as per local standard of care with a SU or accelerated SU regimen, and will be followed prospectively for 12 months. The participants enrolled and the clinicians will be masked to tests results. With a planned recruitment of 200 people, the estimated end date for the study listed on ClinicalTrials.gov is June 2021. 82
Two additional studies evaluating PredictSURE-IBD were highlighted by PredictImmune in its response to a request for information as part of the Diagnostics Assessment Programme process:
-
a prospective, masked study stratifying a paediatric cohort with incident IBD (n = 80)
-
a head-to-head comparison of PredictSURE-IBD with IBDX for stratification of those at higher risk of following a severe course of CD using samples from cohorts previously assessed as part of a study evaluating PredictSURE-IBD.
Results for the head-to-head comparison of PredictSURE-IBD and IBDX are now available in a conference abstract. 83
Evidence provided by the companies
Glycominds
Glycominds provided a list of bibliographic details of the key publications outlining the evidence in support of the IBDX tool. All studies reporting results on the effectiveness of the kit in stratifying those at high risk of following a severe course of CD were retrieved, and subsequently reviewed, by the EAG.
PredictImmune
PredictImmune provided a list of bibliographic details for several publications relating to PredictSURE-IBD, including references describing the research underpinning the development of the signature gene sequence. All studies flagged by the company were retrieved, and subsequently reviewed, by the EAG.
Additionally, in response to queries from the EAG, PredictImmune supplied anonymised individual patient data (IPD) for results from the cohort that provided results for validation of PredictSURE-IBD, together with data for the head-to-head comparison of PredictSURE-IBD with IBDX. The results provided by PredictImmune for this direct comparison are presented and critiqued in Comparison of IBDX and PredictSURE-IBD.
Assessment of prognostic test accuracy
Characteristics of included studies
All studies informing the evidence base on the prognostic accuracy of the IBDX and PredictSURE-IBD biomarker stratification tests were observational in design. Key characteristics of the included studies are summarised in Table 4, with validated data extraction forms for studies available in Report Supplementary Material 5. Twelve publications, describing eight studies, retrieved from electronic searches were included in the assessment of the prognostic accuracy of the tests, with seven of the studies (11 publications) reporting results on the utility of the IBDX kit and one on the utility of PredictSURE-IBD in stratifying those at high-risk of a severe course of CD (see Table 4). Several studies included a mixed population of participants with CD and ulcerative colitis, and reported results separately for those with CD. Most studies included predominantly adults with CD, with one study (three publications) reporting data for an adolescent or a paediatric population. No additional potentially relevant study was identified from hand-searching the bibliographies of three systematic reviews. 38,66,68,70
All included studies assessed outcomes in people reported to have a diagnosis of CD. However, limited reporting was noted across studies relating to the IBDX on stage of diagnosis (newly vs. established) at the time of the test. Baseline characteristics suggest that the samples analysed were provided predominantly by people with established CD (see Report Supplementary Material 5). By contrast, most people enrolled in the study on PredictSURE-IBD had received a recent diagnosis of CD.
Prespecified inclusion criteria for the systematic review presented here required that people have active disease (see Table 3). Although most of the included studies outlined criteria to be met for a diagnosis of CD, only the study evaluating the PredictSURE-IBD tool required people to have active disease to be eligible for enrolment and reported how presence of active disease was determined. 50 In retrospect, given the biomarker targets of the two prognostic tests, the reviewers consider that the criterion of active CD is appropriate for studies assessing PredictSURE-IBD but is not essential for studies reporting on IBDX. As outlined in Chapter 1, Description of the technologies under assessment, the PredictSURE-IBD tool detects a gene sequence associated with CD8+ T-cell exhaustion that arises from an autoimmune response to active disease, and, therefore, it is appropriate to require that people have active CD when blood is taken for analysis; it has been reported that in people with inactive disease after treatment, as determined by endoscopy, the level of CD8+ T-cells increases to a level that is comparable with those observed in healthy controls. 84 By contrast, the IBDX kit detects serum levels of specific anti-glycan antibodies, with specified cut-off values for allocating positive or negative status to each biomarker. Although serum levels of each antibody can change over time, it is purported that status for positivity or negativity for that antibody remains stable throughout the course of disease. 74 Therefore, for IBDX, the reviewers decided to include those studies not specifying a measure of active disease if they met all of the other inclusion criteria and reported an assessment of the six biomarkers included in the IBDX panel.
Analyses presented for evaluation of the six biomarkers forming the IBDX kit typically reported the association of positivity for individual biomarkers, or the positive status for a larger number of biomarkers, with the increased risk of following a severe course of CD, and not the evaluation of all six biomarkers as a collective.
Considering PredictSURE-IBD, the included study described use of the tool in three cohorts, two training cohorts and one validation cohort. 50 Samples from one training cohort (n = 66) were used in biomarker discovery and samples from the second (n = 39) were used in whole blood classifier development. Estimates of prognostic accuracy are available for the validation cohort only. Based on IPD data supplied by the company, the reviewers consider the validation cohort together with the second training cohort (n = 39) to be the most appropriate data set to inform the evidence base on for economic analysis; this is discussed in greater detail in Chapter 4, Development of the health economic model.
Caveats to interpretation of the results for prognostic accuracy of both tests are discussed in Accuracy of prognostic tests.
Quality assessment of included studies
Included studies were assessed for risk of bias and applicability using the QUIPS tool. 59,60 A summary of the results of the assessment of risk of bias and generalisability concerns across studies is presented in Table 5 (see Report Supplementary Material 4 for the full critique of each study).
Study (first author and year) | Participation | Attrition | Measurement of prognostic factor | Outcome assessment | Measurement of confounding factors | Analysis and reporting |
---|---|---|---|---|---|---|
IBDX | ||||||
Harrell 201067 (conference abstract) | Unclear | Unclear | Unclear | Unclear | Unclear | Unclear |
Paul 201569 (full publication) | Low | Low | Low | Low | Unclear | Low |
Rieder 201075 (full publication) | Moderate | Low | Low | Low | Moderate | Low |
Rieder 201076 (full publication) | Low | Low | Low | Low | Moderate | Low |
Rieder 201272 (full publication) | Moderate | Low | Low | Low | Moderate | Low |
Seow 200978 (full publication) | Moderate | Low | Low | Low | Moderate | Low |
Wolfel 201779 (conference abstract) | Unclear | Unclear | Unclear | Unclear | Unclear | Unclear |
PredictSURE-IBD | ||||||
aBiasci 201950 (full publication) | Low | Unclear | Low | Low | Unclear | Low |
The QUIPS tool encompasses six domains for the assessment of the validity and bias of studies evaluating prognosis and factors influencing the course of a condition:59,60
-
participation
-
attrition
-
prognostic factor measurement
-
confounding measurement and account
-
outcome measurement
-
analysis and reporting.
Each domain comprises prompting items (between three and seven) for consideration in the overall rating for an item of high, moderate or low risk of bias. 59,60
The IBDX and PredictSURE-IBD tools were designed with the goal of predicting a course of disease based on the levels of biomarkers produced in response to the presence of CD, with stratification to high or low risk of a severe course of the disease determined by the results of laboratory analysis. The extent to which biomarker levels in blood and serum samples change over time in individual people and what factors influence these fluctuations in levels is uncertain. Additionally, as production of the biomarkers assayed is triggered by changes in cellular processes, the effect of physical characteristics that could influence prognosis in CD, for example smoking status and age, on biomarker levels is unclear. Thus, for the studies informing the evidence on prognostic test accuracy reported here, the EAG considers that the importance of the ‘confounding measurement and account’ domain as a determinant of the risk of bias associated with the studies is also unclear. To reflect the ambiguity around the importance of confounding factors, and to capture uncertainty where limited reporting in the publication precluded an assessment of risk for a particular domain, the EAG adapted the QUIPS tool to include an overall assessment of unclear risk.
Around half of the included studies were deemed to have at least one domain with an unclear risk of bias (see Table 5); for conference abstracts, an unclear rating was predominantly associated with the limited reporting of details as a result of space constraints.
Most studies reporting results for the IBDX tool were determined to be at a moderate risk of bias for the population domain as the studies included those with a recent diagnosis and those with an established diagnosis of CD, and, in some studies, those with presence of severe disease at baseline. Data were not analysed separately for the individual subgroups. The population of greatest relevance to the economic evaluation is those with a new diagnosis of CD and who have moderate or severe disease activity. The study assessing the prognostic accuracy of PredictSURE-IBD enrolled those with a recent diagnosis of CD but included any level of disease activity at sample assessment, with the severity of disease activity determined by endoscopy for some people; severity of disease activity at baseline was not available for all those forming the validation cohort.
Most studies were considered to be at a low risk of bias for attrition and for measurement of prognostic factors because all samples taken were analysed with the relevant tool and results were generated as per the company’s individual protocols. Additionally, outcome assessment was deemed to be at a low risk of bias across many studies as the clinicians were masked to the results of the biomarker assessment.
Accuracy of prognostic tests
The EAG notes that limited data were available from the included studies on the prognostic accuracy of the tools in stratifying the risk of a severe course of CD in terms of standard measures of test accuracy, for example sensitivity and specificity. The EAG is unaware of a validated definition for determining whether or not an individual’s CD has followed a severe course, for example a set number of treatment escalations or the development of a complication or a need for surgery. Thus, the EAG considers the criterion required for a true-positive or false-positive result for IBDX and PredictSURE-IBD to be unclear. The EAG considers that it would be challenging to ascertain an accurate estimate of prognostic accuracy of IBDX and PredictSURE-IBD in stratifying a course of CD. Establishing the prognostic accuracy of the tools would require carrying out a prospective study that included a group that received only SU treatment after determination of their risk of course of CD, using clear prespecified criteria for following a severe course. The ongoing PROFILE RCT randomises people to accelerated SU or TD treatment after they are determined to be at high or low risk of following a severe course of CD, and so the two SU groups will provide additional data to inform estimates of prognostic accuracy. 51 Additionally, no study included in the review prospectively followed people whose treatment was determined by results from IBDX and PredictSURE-IBD; the ongoing PROFILE RCT assesses whether or not early treatment with TD strategy affords clinical benefit to those categorised as being at high risk of severe course of CD and should provide data on the clinical impact of using PredictSURE-IBD.
IBDX
No identified study reported the accuracy of the IBDX kit as a whole (six biomarkers) as per the prespecified prognostic outcome of interest to this review of stratification by risk of following a severe course of CD (see Table 3). One study reported that positivity for ASCA and AMCA had the best prognostic validity for differentiating a severe course of CD from a non-severe course of CD, with an area under the curve of 0.63 and 0.65, respectively. The combination of ASCA and AMCA increased the precision of the differentiation, with an area under the curve of 0.71. 69
In its submission to the Diagnostic Assessment Programme (DAP), Glycominds reported a sensitivity for IBDX of 78%, and a specificity of 85–98% depending on the number of positive biomarkers. Data or details of references to support the reported sensitivity and specificity were not provided in the documentation. None of the studies included by the EAG provided estimates of sensitivity or specificity for the IBDX panel. Additionally, it is unclear whether the reported estimates relate to the sensitivity and specificity of the diagnosis of CD, including differentiation of CD from ulcerative colitis, or that of the stratification of risk of severe course of CD.
The typical test time for IBDX is reported by Glycominds to be around 90 minutes and all samples can be run in parallel.
The instructions on the use of the IBDX kit advise that, in cases of an equivocal test result, the individual biomarker should be tested again. Details on the frequency of an equivocal result are not available from the identified studies.
A longitudinal analysis assessed whether or not levels of the individual biomarkers fluctuate over time. 74 Between two and seven serum samples were available from each person forming the cohort for analysis. Over a median follow-up of 17.4 months (interquartile range 8.0–31.6 months), the authors noted that, despite marked changes in overall immune response and levels in individual biomarkers, the status of positivity or negativity for an individual biomarker remained mostly stable over time.
PredictSURE-IBD
One publication50 assessing the PredictSURE-IBD tool was deemed to meet the inclusion criteria for the review. Several related papers were identified and determined not to be relevant because they described the research underpinning the identification of the signature genetic profile (15 target genes and two control genes) that stratifies those with active CD by high or low risk of a severe course of disease and did not discuss the use of PredictSURE-IBD (see Report Supplementary Material 5 for data extraction).
The included study enrolled people aged ≥ 18 years with active CD or ulcerative colitis who were not receiving concomitant glucocorticosteroids, IMs or biological therapy. Participants were recruited from a specialist IBD clinic before treatment started. Diagnosis of CD or ulcerative colitis was based on standard endoscopic, histological and radiological criteria. Active disease was confirmed by one or more objective markers (raised C-reactive protein, raised calprotectin or endoscopic evidence of active disease) in addition to active symptoms and/or signs. People were treated using a conventional SU strategy in accordance with national and international guidelines.
In the publication, the results on stratification to high or low risk of a severe course of CD are presented for a training cohort (N = 118; CD, n = 66; ulcerative colitis, n = 52) and a validation cohort (N = 123; CD, n = 66; ulcerative colitis, n = 57). 50 Additionally, the full-text publication refers to a second training cohort (n = 39) from whom samples were used in the development of a whole blood classifier. Results from the training cohort (n = 66) used in biomarker discovery were used to finalise the signature gene sequence, which was subsequently applied to analysis of the validation cohort. Two different source cells were used in the process, with mRNA extracted from unseparated peripheral blood mononuclear cells for the training cohort informing biomarker discovery and from a venous blood sample for the validation cohort, as would be the case in clinical practice. Both unseparated peripheral blood mononuclear cells and blood samples were processed for the second training cohort (n = 39), but it is unclear from the full publication whether or not the whole blood samples were analysed using the signature gene sequence identified during biomarker discovery. As part of the DAP, the company clarified that blood samples from the second training cohort were analysed using the finalised gene sequence. Thus, the EAG considers results from the validation cohort and the smaller training cohort to be the most appropriate data set to inform the evidence based on the accuracy of PredictSURE-IBD. However, data on specificity and sensitivity are available for the validation cohort only.
Of the 66 people in the validation cohort, 27 (40.9%) were categorised as being at high risk of following a severe course of CD and 39 (59.1%) were categorised as being at low risk. Of the 39 people in the training cohort, 19 (48.7%) and 20 (51.3%) were categorised as being at high risk and low risk, respectively. Baseline characteristics for the validation cohort indicate that most people had newly diagnosed CD (61/66; 92.4%). The EAG notes that level of disease activity at enrolment (mild, moderate or severe) was not reported, and details on the proportion of people with complications of CD (e.g. fistulae and perianal disease) at baseline are not available in the full publication, but were provided by PredictImmune in its response to a request for information as part of the DAR process (see Report Supplementary Material 5);50 complications of CD at baseline could indicate an earlier requirement for surgery in the SU algorithm.
Data on the number of test failures and the number of inconclusive test results were not available.
Sensitivity and specificity
The study by Biasci et al. 50 reports a sensitivity and specificity for predicting the need for multiple escalations within the first 18 months of 72.7% and 73.2%, respectively. The full-text publication does not provide a cut off value as to how the sensitivity and specificity for multiple escalations were derived. As noted earlier, the EAG is unaware of a validated definition for determining whether or not a person has followed a severe course of CD, and, as a consequence, considers the criterion required for a true positive or false positive to be unclear for the prognostic tests assessed in this review.
As part of the DAP process, PredictImmune provided anonymised IPD for the validation cohort, including the 2 × 2 table for calculation of sensitivity and specificity for multiple escalations at 12 and 18 months (Table 6). PredictImmune applied a cut-off point of two or more treatment escalations to categorise people as having followed a more aggressive course of CD. The EAG considers the company’s approach reasonable. However, the EAG notes that people in the validation cohort and second training cohort underwent treatments at the discretion of the treating clinician and so a proportion (29/105; 27.6%) received a therapy other than glucocorticosteroid at entry, including elemental diet, anti-TNF alone or in combination with IMs, and IMs alone. The EAG recognises that the study is of a more pragmatic design but considers that induction treatment would be likely to influence the timing and frequency of treatment escalation and, consequently, sensitivity and specificity. Moreover, some people included in the calculation of sensitivity and specificity for predicting multiple escalations received surgery as a first treatment escalation (7/66; 10.6%) and continued to be monitored for subsequent treatments, including IMs and biological therapies. Given that RCTs assessing clinical effectiveness of treatment strategies in the management of CD typically report CD-related complications (e.g. need for surgery or hospitalisation or development of fistula or stenosis) as a composite clinical outcome or separately, the EAG considers it important to assess the time to and occurrence of surgery independently of other treatment escalations to reflect the outcomes in other studies, including those assessing the effectiveness of IBDX; the EAG’s clinical experts supported the proposal that it would be appropriate to assess CD-related surgery as a separate outcome. The inclusion of people who underwent surgery as a first treatment escalation and received subsequent treatment escalations could influence the accuracy of sensitivity and specificity as assessed by the number of treatment escalations. The EAG notes that the sample size for the validation cohort is small (n = 66) and, moreover, that not all people in the validation cohort were included in analyses at 12 or 18 months. Additionally, a proportion of people in the validation cohort received an anti-TNF biologic with or without an IM (11/66; 16.7%) as their first escalation. 50 The EAG appreciates that the study is pragmatic and is likely to reflect treatment approaches in clinical practice in the UK, but the EAG also considers that analysing those who receive TD or surgery as their first treatment escalation together with those who followed the SU treatment algorithm or were treated at the discretion of the treating clinician is unlikely to reflect the true estimate of the number of treatment escalations that would occur with the SU or accelerated SU strategy.
PredictSURE-IBD categorisation | < 2 treatment escalations, patients (n) | ≥ 2 treatment escalations, patients (n) | Sensitivity | Specificity |
---|---|---|---|---|
Within 12 months | ||||
Categorised as at high risk | 15 | 7 | 77.8% | 70.6% |
Categorised as at low risk | 36 | 2 | ||
Within 18 months | ||||
Categorised as at high risk | 11 | 8 | 72.7% | 73.2% |
Categorised as at low risk | 30 | 3 |
Predictive value
The included study reports a negative predictive value of 90.9% for PredictSURE-IBD of predicting multiple escalations within the first 18 months. 50 Based on the 2 × 2 table supplied by PredictImmune (see Table 6), the EAG calculates a positive predictive value of 42.1% for predicting multiple escalations within the first 18 months.
Results for clinical outcomes
The EAG notes that the results presented in this section are on the risk of experiencing an event among those categorised by the tools as being at high or low risk of following a severe course of CD, and are not related to the clinical outcome of treatment decisions based on the stratification of risk using IBDX and PredictSURE-IBD.
IBDX
Results are reported based on positive status for increasing number of biomarkers, as per the company’s recommendations on the interpretation of outputs from the test (see Figure 2). As noted, all included studies evaluated the full panel of biomarkers constituting the IBDX kit, but there is no single measure of accuracy or clinical outcome for the six biomarkers as a collective.
Clinical and methodological heterogeneity across the identified studies precluded meta-analysis and the results are presented in a narrative review.
Developing a complication
Two studies reported an effect estimate for the risk of experiencing a complication by the number of biomarkers testing positive (the results are available in Appendix 1, Table 26). 75,76 Both studies prospectively followed a cohort of people with CD.
Severe disease behaviour was defined in both studies as the occurrence of fistulae or stenosis. 75,76 In one study, 68% of people (249/363) had a complication before or at the time of sample procurement. 75 The second study enrolled people with or without prior complication and with or without prior CD-related surgery but focused reporting on those with no prior complications and no CD-related surgery before or within 20 days of obtaining the sample (n = 76). 76 Median follow-up was 59 months for one cohort75 and 53.7 months for the other. 76
The median duration of CD was disparate between the two studies, with one study reporting a median of 66.8 months (interquartile range 11–141 months),75 compared with a much shorter 10.6 months (interquartile range 1.7–52.3 months)76 in the other. The EAG’s clinical experts advised that 10.6 months may be insufficient follow-up to monitor the development of a CD-related complication.
In the study including people with complications at baseline,75 an odds ratio (OR) of 1.5 (95% CI 1.3 to 1.9, p < 0.001; see Appendix 1, Table 26) was reported for experiencing a complication compared with not experiencing a complication, with increased risk associated with a positive status for a larger median number of biomarkers. During follow-up, an additional 28 people developed a fistula or stenosis, or both.
Among people with no prior complication, 20 experienced a fistula or stenosis, with a higher risk of experiencing a complication noted for those with positive status on at least two or three biomarkers (see Appendix 1, Table 26), with the risk reaching statistical significance for those testing positive for at least two of the six antibodies [hazard ratio (HR) 2.5, 95% CI 1.03 to 6.1; p = 0.043]. 76 The EAG notes the small sample size informing the estimate of risk.
Increasing the number of positive antibodies was reported to be significantly associated with severe disease behaviour and/or surgery (OR 3.3, 95% CI not reported; p = 0.0005) for a cohort of people with CD from the USA;67 the results were presented in a conference abstract and limited details are available. Severe disease behaviour was defined as intestinal fistula and/or stricture.
One study of a cross-sectional design analysed serum samples from children and adolescents aged ≤ 18 years. 71–73 The authors reported results for this younger cohort that were aligned with those derived from an adult cohort, with a larger number of positive serum biomarkers associated with an increased risk of experiencing severe CD and requiring CD-related surgery (estimates of effect not reported). 72 Additionally, the authors assessed differences in the cut-off levels used to indicate the positivity of biomarkers between the paediatric cohort and adults evaluated in a related study75 and found that lower cut-off points denoted positivity in paediatric samples. In a related conference abstract, the authors reported that in paediatric patients with CD, positivity on at least one marker out of the whole panel compared with no positive marker was independently associated with fibrostenotic or fistulising disease behaviour (p = 0.036) and ileal disease location (p = 0.014). 71 Although the accuracy of the biomarker panel in diagnosing CD and differentiating it from other gastrointestinal conditions was reported to decrease with age at sample procurement, when assessing CD behaviour, the ability of the panel to stratify disease phenotypes remained constant over time. 72
Requirement for surgery
Two out of the three studies reporting on the risk of complications also provided information on the increased likelihood of requiring surgery among people with a higher risk of a severe course of CD. 75,76 A third study78 with a cross-sectional design evaluated serum samples from 517 people with CD who had a median duration of disease of 8.9 years (range 0.02–46.30 years).
One study reported an OR of 1.5 (95% CI 1.3 to 1.8, p < 0.001; see Appendix 1, Table 27) for requiring surgery compared with no requirement for surgery, with increased risk associated with a positive status for a larger median number of biomarkers. 75 At the time of sample procurement, 224 people had undergone surgery related to IBD, with an additional 33 people requiring surgery during follow-up.
For the cohort of people who had not undergone surgery at enrolment, 14 people required surgery, with a statistically significantly higher risk for surgery (HR 3.6, 95% CI 1.2 to 11.0, p = 0.023; see Appendix 1, Table 27). 76 The EAG notes the small sample size informing the analysis, and the large CI accompanying the estimate of risk.
The third study identified a trend towards a larger proportion of people requiring surgery with increasing number of biomarkers testing positive (see Appendix 1, Table 27). 78 A statistically significant difference across the categories assessed was identified (p < 0.0001).
A conference abstract provided results for a cohort of people (n = 118) who had undergone one surgical intestinal resection related to CD. 79 Most people evaluated (92%) underwent first surgery for internal penetrating and/or stricturing disease. Serum samples for analysis with the IBDX kit were taken after surgery. After a median follow-up of 100 months, the authors reported that, when considering the full panel of six biomarkers, neither the quartile sum score nor the number of positive biomarkers combined predicted a shorter time to repeat intestinal surgery. After adjustment for ileal disease location and use of IMs or anti-TNF biologic after first surgery, analysis of individual biomarkers identified that positivity for AMCA (HR 2.6, 95% CI 1.1 to 5.9; p = 0.026) and ALCA (HR 2.3, 95% CI 1.04 to 5.3; p = 0.039) predicted a shorter time to second surgery. 79 Another study reported that, of the panel of tested antibodies, only AMCA tended to be associated with higher risk of CD-related surgery, with an OR of 2.1 (95% CI 0.8 to 5.1; p = 0.10), but the association did not reach statistical significance. 69
PredictSURE-IBD
Time to treatment escalation
The full-text publication50 reported that those categorised as at high risk of following a severe course had a statistically significantly higher risk of first treatment escalation than those categorised as at low risk, with a HR of 2.65 (95% CI 1.32 to 5.34; p = 0.006).
The EAG notes that, based on the IPD supplied by PredictImmune, people in the validation cohort underwent treatments at the discretion of the treating clinician, and so a proportion (14/66; 21.2%) received a therapy other than glucocorticosteroid at entry. 50 Choice of and time to first treatment escalation is likely to be influenced by the response to treatment at study entry, which in turn is likely to be affected by the risk of following a severe course of CD. The EAG recognises that the study is of a more pragmatic design but considers that, as people in the validation cohort have not followed a standardised algorithm of treatment, analysis of time to first treatment escalation is subject to a level of bias, the direction of which is unclear.
The EAG analysed IPD provided by PredictImmune for incorporation into the economic model, with a focus on those with a new diagnosis of CD as per the protocol.
Comparison of IBDX and PredictSURE-IBD
For the head-to-head comparison of PredictSURE-IBD and IBDX, the cohort analysed comprised those with active CD as confirmed by one objective marker (i.e. raised C-reactive protein, raised calprotectin or endoscopic signs of active disease) in addition to active symptoms. Participants had been recruited from a single site in the UK for an observational study evaluating PredictSURE-IBD. All those enrolled were treated with the accelerated SU regimen in accordance with UK guidelines. Samples for analysis by the two biomarker tests were taken concurrently from the same bleed: PredictSURE-IBD requires whole-blood RNA and IBDX uses serum. A conference abstract outlining the results of the comparison has now been published. 83 Results reported in the conference abstract indicate that those categorised as being at high-risk of following a severe course of disease using PredictSURE-IBD experienced a more aggressive disease, characterised by a shorter time to treatment escalation, compared with those designated as at low risk. 83 The authors also commented that seropositivity for antiglycan antibodies at diagnosis did not predict the need to escalate treatment due to frequently-relapsing or chronically-active disease. 83
Summary of findings for prognostic test accuracy
Sensitivity, specificity and negative predictive value
The evidence base on the prognostic accuracy of the IBDX and PredictSURE-IBD tools in identifying those at high risk of following a severe course of CD is limited. No study was identified that provided an assessment of the prognostic accuracy of the full panel of six biomarkers for the IBDX, and only one observational study provided results for PredictSURE-IBD in stratifying those with a recent diagnosis of CD and disease of any level of activity at the time of sample procurement, with the severity of disease activity determined by endoscopy for some people; severity of disease activity at baseline was not available for all those forming the validation cohort.
Use of PredictSURE-IBD was associated with a sensitivity and specificity of 77.8% and 70.6%, respectively, in stratifying by need for multiple treatment escalations within 12 months. The corresponding sensitivity and specificity for multiple escalations within 18 months were 72.7% and 73.2%, respectively. A negative predictive value of 90.9% for PredictSURE-IBD of predicting multiple escalations within the first 18 months was also reported. The EAG notes that the cut-off point for multiple escalations applied in the determination of sensitivity and specificity was two treatment escalations, and comprised any type of treatment, including surgery. The EAG is unaware of a validated definition for determination of whether a person has followed a severe course of CD and considers the choice of two escalations to be an arbitrary value. Additionally, the EAG’s clinical experts fed back that it would be appropriate to consider escalation to CD-related surgery separately from progression to drug treatment, and also to use development of a complication of CD (fistula or stenosis) as another marker of sensitivity and specificity. The full-text publication presenting results for PredictSURE-IBD indicates that those in the validation cohort were treated at the discretion of the treating clinician. IPD data provided by PredictImmune indicate that, of those in the validation cohort, 21.2% (14/66) received a therapy other than glucocorticosteroid at entry. Choice of and time to first treatment escalation is likely to be influenced by the response to treatment at study entry, which in turn is likely to be affected by the risk of following a severe course of CD. The EAG recognises that the study is of a more pragmatic design but considers that, as people within the validation cohort have not followed a standardised algorithm of treatment, induction treatment would likely influence the timing and frequency of subsequent escalations, and consequently sensitivity and specificity. The risk of bias of the study as assessed by the QUIPS tool was determined to be low across most domains. Considering the caveats highlighted by the EAG, together with the small sample size (n = 66) informing calculation of prognostic accuracy for PredictSURE-IBD, the EAG considers that the results are potentially unreliable and should be interpreted with caution.
Clinical outcomes
Clinical outcomes that could be considered proxies for predicting prognosis are those that are typically associated with following a severe course of CD, including higher risk of developing a complication of CD (fistula or stenosis), of needing CD-related surgery, and a shorter time to and increased frequency of treatment escalations.
Seven studies67,69,72,75,76,78,79 evaluating the IBDX kit were deemed to be of relevance to the review, all of which were observational in nature: three studies were prospective cohorts75,76,79 and three were of a cross-sectional design. 69,72,78 Of those studies reporting estimates of effect, people enrolled in the studies predominantly had an established, rather than a recent, diagnosis of CD. Clinical heterogeneity across studies in terms of various characteristics (prior complication versus no complication, previous IBD-related surgery or no surgery, and unclear whether people had active disease at baseline) was noted, which led to a determination of moderate risk of bias for the population domain based on the QUIPS tool. Two prospective cohort studies reported increased risk of experiencing a complication or of requiring surgery for those testing positive for at least two of the six biomarkers included in the IBDX kit. In addition, some estimates were informed by small sample sizes. Risks of experiencing a complication by positive biomarker status were reported to be:
-
OR 1.5 (95% CI 1.3 to 1.9; p < 0.001; n unclear) based on positivity for a median of two biomarkers
-
HR 2.5 (95% CI 1.03 to 6.1; p = 0.043; n = 20 with no prior complication or surgery) based on positivity for at least two biomarkers
-
HR 2.6 (95% CI 0.92 to 7.2; p = 0.072; n = 20 with no prior complication or surgery) based on positivity for at least three biomarkers.
Considering surgery, three studies reported on the increased risk of surgery. One study reported a trend towards a larger proportion of people with CD requiring abdominal surgery with increasing number of positive biomarkers (n = 517; p < 0.0001 across the groups). Other estimates of higher risk of requiring surgery were:
-
OR 1.5 (95% CI 1.3 to 1.8; p < 0.001; n unclear) based on positivity for a median of two biomarkers
-
HR 3.6 (95% CI 1.2 to 11.0; p = 0.023; n = 14 with no prior complication or surgery) based on positivity for at least two biomarkers
-
HR 2.8 (95% CI 0.80 to 9.6; p = 0.11; n = 14 with no prior complication or surgery) based on positivity for at least three biomarkers.
Estimate of the increased risk of treatment escalation by number of positive biomarkers was not available for IBDX.
In a study evaluating IBDX in an adolescent population, results for adolescents aligned with those derived from an adult cohort, with a higher number of positive serum biomarkers associated with an increased risk of experiencing severe CD and requiring CD-related surgery. Research suggests that, although the levels of biomarkers fluctuate over time, the positive or negative status for an individual biomarker remains constant.
Estimates of increased risk of developing a complication or requirement for surgery were not available for PredictSURE-IBD. The study evaluating PredictSURE-IBD reported that those categorised as at high risk of following a severe course of CD had a statistically significantly higher risk of first treatment escalation compared with those designated as at low risk, with a HR of 2.65 (95% CI 1.32 to 5.34; p = 0.006). As noted earlier, based on the IPD supplied by PredictImmune, some of the validation cohort received a therapy other than glucocorticosteroid at entry. The EAG considers that choice of and time to first treatment escalation is likely to be influenced by the response to treatment at study entry, which in turn is likely to be affected by the risk of following a severe course of CD. As people in the validation cohort have not followed a standardised algorithm of treatment, the EAG considers analysis of time to first treatment escalation as subject to a level of bias, the direction of which is unclear. The EAG reiterates that clinical experts fed back that it would be useful to assess CD-related surgery as an independent outcome.
Given the disparity in the clinical outcomes assessed for the IBDX and PredictSURE-IBD, the EAG considers that no conclusions can be drawn on the comparative effectiveness of the two tools in stratifying people by the risk of a severe course of CD.
Chapter 4 Methods for assessing cost-effectiveness
Systematic literature review for cost-effectiveness studies
Methods
A systematic literature review (SLR) was undertaken in July 2019 to identify published economic evaluations of the PredictSURE-IBD and IBDX tools, as well as economic evaluations of treatments for newly diagnosed patients with moderate to severe CD. The searches were also used to identify potential model parameters in case a de novo model was needed. The searches were used to identify resource use and cost data, together with the natural history of CD. Separate searches were carried out for supporting information on utility data.
The following databases were searched for relevant studies:
-
Ovid MEDLINE® Epub Ahead of Print, In-Process & Other Non-Indexed Citations, Daily and Versions® (via Ovid)
-
EMBASE (via Ovid)
-
NHS Economic Evaluation Database (NHS EED) (CRD)
-
CDSR (via Cochrane)
-
CENTRAL (via Cochrane)
-
Database of Abstracts of Reviews of Effects (DARE) (CRD)
-
Health Technology Assessment (HTA) database (CRD).
Further to the database searches, experts in the field were contacted with a request for details of relevant published and unpublished studies, and reference lists of key identified studies were also reviewed for any potentially relevant studies.
The search strategy for existing economic evaluations of prognostic tests combined terms capturing the tests of interest (PredictSURE-IBD and IBDX) and the target population (adults who have been newly diagnosed with moderate to severe CD, and who have not been offered biologics under current standard care) with economic and health-care resource use terms (adapted from the Canadian Agency for Drugs and Technologies in Health’s search filter for economic evaluations). 85
The target population considered in the SLR to identify economic evaluations of treatments for CD and health-related quality-of-life evidence (adults with moderate to severe CD) was broader than the population considered in the SLR to identify economic evaluations of prognostic tests to account for the fact that patients’ characteristics change along the treatment pathway. The search strategy for existing economic evaluations of treatments for CD also replaced prognostic tool terms with terms related to corticosteroid, IM and biologic treatments. The search strategy for health-related quality-of-life data was not restricted by prognostic tools or treatments, and it combined terms capturing the target population with health-related quality-of-life terms (adapted from Arber et al. 86).
Limits were applied to searches to remove animal studies, letters, editorials, comments or case studies. Only conference abstracts published within the last 2 years were considered for inclusion; it was assumed that any high-quality studies reported in abstract form before that date would have been published in a peer-reviewed journal. Searches were also restricted to studies published in the English language; however, no restriction by setting or geographical location was applied to the search strategy. Full details of the search strategies are presented in Report Supplementary Material 2.
The titles and abstracts of the papers identified through the searches were independently assessed for inclusion by two reviewers using predefined eligibility criteria. The inclusion and exclusion criteria for each of the three reviews are outlined in Box 1. The methodological quality of the full economic evaluations identified in the review was assessed using the Drummond checklist. 87
-
Prognostic tests according to the scope of the assessment (PredictSURE-IBD and IBDX).
-
Study population according to the scope of the assessment (adults aged ≥ 16 years newly diagnosed with moderate to severe CD and who have not been offered biologics under current standard care).
-
Full economic evaluations (cost–utility, cost-effectiveness, cost–benefit or cost–consequences analyses) that assess both costs and outcomes associated with the prognostic tests of interest.
-
Economic evaluations of treatment strategies for CD, including the TD and SU (standard and accelerated) approaches; however, if insufficient data can be identified on those approaches, economic evaluations of individual treatments will be considered.
-
Study population included in the conceptual model (adults aged ≥ 16 years with moderate to severe CD).
-
Full economic evaluations (cost–utility, cost-effectiveness, cost–benefit or cost–consequences analysis) that assess both costs and outcomes associated with the treatment of interest.
-
Studies reporting utility data elicited using a generic or condition-specific preference-based measure, or vignette and a validated, choice-based technique for valuation (i.e. time trade-off or standard gamble); however, if sufficient EQ-5D data are found during the searches for utility data, the EAG will restrict the data extraction to EQ-5D data.
-
Studies reporting utility data referring to specific health states associated with the treatment of CD patients in the economic model.
-
Studies in adults (aged ≥ 16 years) with moderate to severe CD.
-
Primary sources of utility data.
-
Non-English language.
-
Abstracts with insufficient methodological details.
-
Conference papers published 2 years before the search was performed (papers published before 2017).
-
Papers published before NICE was formed (1999).
EQ-5D, EuroQol-5 Dimensions.
Economic evaluations of prognostic tests
The SLR identified a total of 115 papers after deduplication and, based on titles and abstracts, a total of three papers were identified as potentially relevant and were obtained for full-text review. Of the three papers identified for full-text review, none was considered relevant for inclusion. Reasons for exclusion are provided in Report Supplementary Material 3. The results of the process to identify evidence are summarised in Figure 4.
FIGURE 4.
The PRISMA diagram of SLR to identify economic evaluations of prognostic tests. DARE, Database of Abstracts of Reviews of Effects; HTA, Health Technology Assessment; NHS EED, NHS Economic Evaluation Database.

Economic evaluations of treatments for Crohn’s disease
The SLR identified a total of 2403 papers after deduplication and, based on titles and abstracts, a total of 80 papers were identified as potentially relevant and were obtained for full-text review. Of the 80 papers identified for full-text review, 32 were considered relevant for inclusion. Of those 32, one Italian study88 specifically compared the cost-effectiveness of the TD and SU approaches. Nice guideline 129 compared nine induction treatment sequences, in a UK setting, composed of four treatment lines. 31
The remaining studies compared individual treatment steps. Given the large number of such studies, data extractions were restricted to UK studies plus the Italian study that compared the TD with the SU approach. Reasons why papers were excluded are provided in Report Supplementary Material 3. The results of the process to identify evidence are summarised in Figure 5.
FIGURE 5.
The PRISMA diagram of SLR to identify economic evaluations of treatments for CD. DARE, Database of Abstracts of Reviews of Effects; HTA, Health Technology Assessment; NHS EED, NHS Economic Evaluation Database.
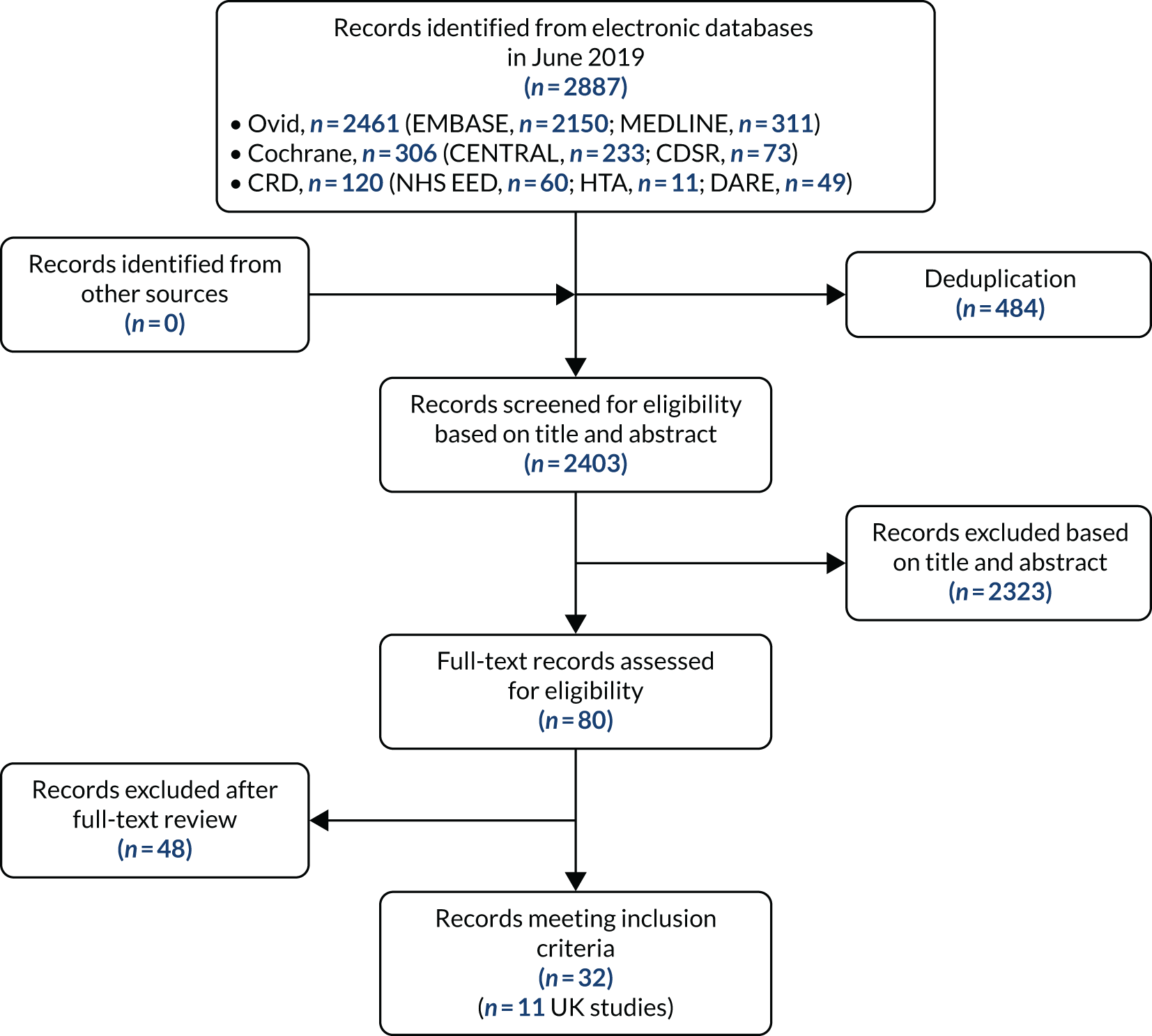
The type of economic evaluation included in each of the 11 extracted studies was a cost–utility analysis, where the incremental cost-effectiveness ratio (ICER) was expressed as the cost per quality-adjusted life-year (QALY) gained. Of the 11 extracted studies, five were related to NICE guidance, including three NICE technology appraisals (TAs),89–91 one NICE clinical guideline31 and one NICE diagnostics guidance. 92 For NICE guideline 129,31 two economic evaluations were developed, one on treatment sequences for the induction of remission and a second on treatments for the maintenance of remission.
The most frequent type of decision-analytic model used to estimate cost-effectiveness was a Markov model. Three papers also included a decision tree followed by a Markov model to disaggregate the short- and long-term effects. 90,91,93 The time horizons in these analyses ranged from 1 to 60 years (lifetime), while the cycle lengths ranged from 2 weeks to 2 months. Decision trees without any Markov component were used to estimate cost-effectiveness over shorter time horizons (30 weeks and 1 year) in the two remaining analyses. 31,94 A summary of the 11 extracted studies is provided in Table 7 and detailed data extractions can be found in Report Supplementary Material 5; see Report Supplementary Material 4 for the quality assessment of the studies.
Study (first author and year) | Population | Interventions/comparators | Model type (cycle length) | Time horizon |
---|---|---|---|---|
Marchetti 201388 | Newly diagnosed luminal moderate to severe CD |
|
Markov model (1 month) | 5 years |
Dretzke 201189 (TA187) |
|
|
Markov model (4 weeks) | 1 year |
Hodgson 201891 (TA456) | Adults with moderate to severe CD in two subpopulations:
|
|
Decision tree followed by Markov model (2 weeks) | 1 year |
Rafia 201690 (TA352) | Moderate to severe active disease after failure of initial therapy in three subpopulations:
|
|
Decision tree followed by Markov model (8 weeks) | 10 years |
Mayberry 201395 (NG129)a |
|
|
Decision tree Markov model (2 months) |
30 weeks 2 years |
Freeman 201696 (DG22) | Moderate to severe active CD treated with infliximab or adalimumab in two subpopulations:
|
|
Markov model (4 weeks) | 10 years |
Saito 201394 | Moderate to severe CD refractory to conventional therapies and naive to biologic therapy |
|
Decision tree | 1 year |
Bodger 200993 | Moderate to severe active CD |
|
Decision tree followed by Markov model (8 weeks) | Lifetime (60 years) |
Loftus 200997 |
|
|
No decision-analytic model, costs and benefits were attached to estimated rates of hospitalisation | 1 year |
Lindsay 200898 |
|
|
Markov model (luminal active CD, 2- to 4-week cycles until week 14 and then 8-weekly; fistulising active CD, one 14-week cycle and one 16-week cycle and then 24-weekly) | 5 years |
Clark 200399 |
|
|
Markov model (2 months) | Lifetime (40 years) |
Health-related quality-of-life evidence
The SLR identified a total of 2221 papers after deduplication and, based on titles and abstracts, a total of 137 papers were identified as potentially relevant and were obtained for full-text review. Of the 137 papers identified for full-text review, 37 were considered relevant for inclusion and 11 of those reported EuroQol-5 Dimensions (EQ-5D) data. The remaining papers considered generic measures, including the SF-36 (Short Form Questionnaire-36 items), the SF-12 (Short Form Questionnaire-12 items), the Psychological General Well-Being Index, the Cleveland Global Quality of Life and the EQ-5D visual analogue scale, and disease-specific measures, including the CDAI and the Inflammatory Bowel Disease Questionnaire. Owing to the large number of relevant papers, the availability of EQ-5D data in these papers and NICE’s preference for EQ-5D data, the EAG decided to restrict the data extraction to primary sources of EQ-5D data. Reasons for exclusion of the ordered papers are provided in Report Supplementary Material 2. The results of the process to identify evidence are summarised in Figure 6.
FIGURE 6.
The PRISMA diagram of SLR to identify health-related quality-of-life evidence. DARE, Database of Abstracts of Reviews of Effects; HTA, Health Technology Assessment; NHS EED, NHS Economic Evaluation Database.

Of the 11 studies that reported EQ-5D data, 10 used the EuroQol-5 Dimensions, three-level version (EQ-5D-3L), and one of those 10 also collected EuroQol-5 Dimensions, five-level version (EQ-5D-5L), data. The remaining paper did not specify which version of the EQ-5D was used. EQ-5D-3L responses were converted into utilities using UK population tariffs in four studies, which were undertaken in Italy,100,101 Germany102 and Hungary. 103 However, each of those four studies used different sources of UK population tariffs to value EQ-5D-3L responses. The sources included Dolan et al. 104 for Benedini et al. ,100 Badia et al. 105 for Mozzi et al. ,101 Dolan et al. 106 for Stark et al. 102 and Dolan107 for Rencz et al. 103 See Report Supplementary Material 5 for full data extractions and see Report Supplementary Material 4 for quality assessment of the studies.
Six of the 11 studies that reported EQ-5D data were undertaken in Spain, and four of those used Spanish population tariffs developed by Badia et al. 108 (for Casellas et al. ,109 Casellas et al. 110 and Huaman et al. 111) or by Rue and Badia112 (for Casellas et al. 113) to convert EQ-5D-3L responses into utilities. The other two studies undertaken in Spain114,115 did not report the sources used to value EQ-5D-3L responses. Finally, one study undertaken in Poland116 valued EQ-5D-3L responses using a Polish population tariff developed by Golicki et al. 117 As for the study that collected EQ-5D-5L responses in Hungary,103 English tariffs developed by Devlin et al. 118 were employed.
PredictImmune’s economic model
During the diagnostic assessment review subgroup meeting, the EAG became aware of the existence of an economic model built by PredictImmune to assess the cost-effectiveness of PredictSURE-IBD. As a result of a request from the EAG, the company supplied the economic model.
Development of the health economic model
As reported in Chapter 3, despite extensive systematic searches of the literature, no robust evidence was identified on the prognostic accuracy of the biomarker stratification tools IBDX and PredictSURE-IBD. Furthermore, the EAG considers that it would be challenging to ascertain an accurate estimate of prognostic accuracy of the tools in stratifying people by the risk of a severe course of CD.
Therefore, the development of an economic model to accurately assess the cost-effectiveness of IBDX and PredictSURE-IBD was not possible, based on the currently available data. Instead, the EAG developed an economic model that provides a structural framework for analysing future available data on prognostic accuracy, and to assess the costs and consequences of treating high- and low-risk patients with both TD and SU strategies. Furthermore, the EAG did not find any robust evidence on the effectiveness of the complete TD or SU treatment sequences, including no evidence on the effectiveness of these strategies by patients’ risk of disease severity.
As the ongoing PROFILE RCT51 randomises people to accelerated SU or TD treatment after the determination of high or low risk of following a severe course of CD, the EAG considers that the trial could provide additional data to inform estimates of prognostic accuracy and patients’ outcomes, stratified by risk and type of treatment received.
As no model found through the SLR met the requirements of the review, the EAG developed its own model. The latter is described in the following sections.
Population
The population included in the economic analysis is adults (aged ≥ 16 years) who have been newly diagnosed with moderate to severe CD and who have not been offered biologics under current standard care. The population in the economic model is largely based on the Biasci et al. 50 population. The paper included a training (n = 38 CD patients) cohort and a validation (n = 66 CD patients) cohort; nonetheless, the published paper did not provide sufficient detail on the treatments received by the validation or training cohorts. Therefore, the EAG asked PredictImmune to provide additional treatment data for the study cohort in Biasci et al. ,50 and in response, the company provided the available individual patient data (IPD).
The IPD included 88 patients with newly diagnosed CD and a classification of high- or low-risk disease. However, the EAG had to remove patients from the IPD (as explained in detail in Time to treatment escalation in high- and low-risk patients); therefore, the final population in the model was reduced to 40 patients (23 high-risk patients and 17 low-risk patients). The average age in the EAG-modelled population was 35 years; 65% of patients were non-smokers, with 25% being smokers and 8% being ex-smokers (smoking status was missing for 2%). Thirty-three per cent of patients were male and 55% were female (12% of patients had no information on sex collected). The study did not collect data on patients’ weight, so the EAG assumed a mean weight of 71.4 kg in the model based on results provided in TA456. 119
Intervention and comparator
As per the final protocol, the interventions of interest are the IBDX and PredictSURE-IBD tests. Nonetheless, the base-case economic model included the PredictSURE-IBD test only, while a scenario analysis was undertaken to compare the IBDX™ test against standard care. Although the EAG considers that there are no robust prognostic accuracy data for either test, the development of the model was based mainly on the IPD provided by PredictImmune pertaining to the use of PredictSURE-IBD.
The comparator included in the analysis is standard care. As no test or algorithm is available in the NHS to determine the long-term course of disease or an individual’s risk of developing a severe course of disease, the estimation of prognosis is based on clinical judgement of presenting signs and symptoms, together with the potential risk factors for developing a severe course of disease (more details are provided in Chapter 2, Search strategies).
For the purpose of the economic model, the EAG assumed that the PredictSURE-IBD test (and the IBDX in the scenario analysis) ultimately categorises patients into high- and low-risk disease categories, so that treatment sequences can be allocated accordingly. The treatment sequences included in the economic model were based on clinical expert opinion provided to the EAG and are intended to describe standard care in the NHS for the SU arm and the accelerated treatment pathway of the TD arm, which is not currently recommended in the NHS. The clinical experts added that < 10% of CD patients receive TD therapy in the NHS; thus, the EAG assumed that patients in the standard care arm of the model can receive SU therapy only. The TD treatment approach is assumed to be received only by high-risk patients who have been tested with either PredictSURE-IBD or IBDX.
The two treatment strategies include an induction treatment with prednisolone for 100% of patients in the model. The difference in treatment strategies thereafter is based solely on the fact that the SU strategy includes an additional treatment step with IMs at the beginning of the sequence. The modelled treatment steps include four bundles of different types of therapy: IMs, anti-TNF biologics, second-line biologics and third-line biologics. Clinical expert opinion was used to derive the distribution of treatments in each treatment bundle. The bundles were defined as follows:
-
IM bundle – 80% azathioprine; 10% mercaptopurine; and 10% methotrexate
-
anti-TNF bundle – 40% infliximab, 60% adalimumab and 30% of all patients get the IM bundle
-
second-line biologic bundle – 50% vedolizumab, 50% ustekinumab and 20% of all patients get the IM bundle
-
third-line biologic bundle – 50% vedolizumab, 50% ustekinumab and 20% of all patients get the IM bundle (patients receiving vedolizumab as second-line treatment are assumed to receive ustekinumab as third-line treatment and vice versa).
The order of treatments received in the TD and SU strategies is described in Figure 7.
FIGURE 7.
The TD and SU treatment strategies.

The clinical experts advising the EAG stated that although all newly diagnosed CD patients (in the TD and SU strategies) start treatment with corticosteroids, patients with moderate to severe CD are extremely unlikely to respond to treatment with corticosteroids alone. Therefore, as a model simplification, the EAG did not include this step in the model, as the results would have been the same in both strategies, given that 100% of patients in the high-risk group (in both the TD and the SU arms) would receive initial induction treatment with corticosteroids and move on to the next treatment step. The EAG appreciates that this may result in a minor discrepancy in the costs associated with the two pathways, that is, SU patients may receive a full course of corticosteroids and TD patients are likely to receive a partial course of corticosteroids only. However, given the lack of robust data around the different lengths of treatment with corticosteroids, and the low cost of corticosteroids, the authors consider that this assumption would have a minimal impact on the results. Regarding the potential risk of additional complications associated with the SU strategy, given the delay in initiating treatment with biologics, the EAG notes that Hoekman et al. 120 concluded that in the long term (10-year follow-up) no difference was found in complications, such as new fistulas or surgery, between the TD and SU arms. Furthermore, although not based on comparative evidence, the Biasci et al. data showed only very few events that required surgery, and no patients underwent more than one surgery during their follow-up period while receiving a SU strategy.
Model structure
The EAG adopted a hybrid modelling approach, whereby a decision tree was developed to allocate patients to a response category after initial induction therapy in either the TD or the SU treatment arm. The decision tree is followed by a cohort model, in which state membership was estimated through a series of different Markov health states.
Patients enter the decision tree model (Figure 8) after they are allocated to the test arm (with either PredictSURE-IBD in the base case or IBDX in the scenario analysis) or to the no test arm (standard care). In the test arm, patients are categorised as being at high- or low-risk of following a complicated course of disease, according to test results, whereas those in the no test arm are designated as at high- or low-risk of following a complicated course of disease based on clinical judgement alone. Given that patients in the standard care arm of the model can receive the SU treatment approach only and that the TD treatment approach is assumed to be received by high-risk patients only, the economic model ultimately assesses the cost-effectiveness of TD therapy compared with SU therapy in high-risk patients. The EAG did not identify any direct evidence on the latter. There is, however, an ongoing study (PROFILE51) that will provide data on the relative effectiveness of these treatment strategies in high-risk patients. The EAG considers that this study should also be able to inform the costs and health consequences of ‘misdiagnosing’ patients as high or low risk. The EAG has undertaken a scenario analysis to account for the cost-effectiveness of misdiagnosed cases. The analysis is described in more detail in Chapter 5.
FIGURE 8.
Model for induction treatment.

After being allocated to either the TD or the SU treatment strategy, patients are allocated to induction therapy, at the end of which they are classified as responders (an improvement in CDAI score of > 70) or non-responders (deterioration, no change or an improvement in CDAI score of < 70). Duration of induction therapy differs by class of treatment (i.e. IM, anti-TNF and second-line biologic). If patients respond to induction therapy, they move to the maintenance cohort model (Figure 9), whereas non-responders escalate to the next step in their allocated treatment strategy.
FIGURE 9.
Cohort model for TD and SU maintenance steps. Blue shading indicates the absorbing state of the model.
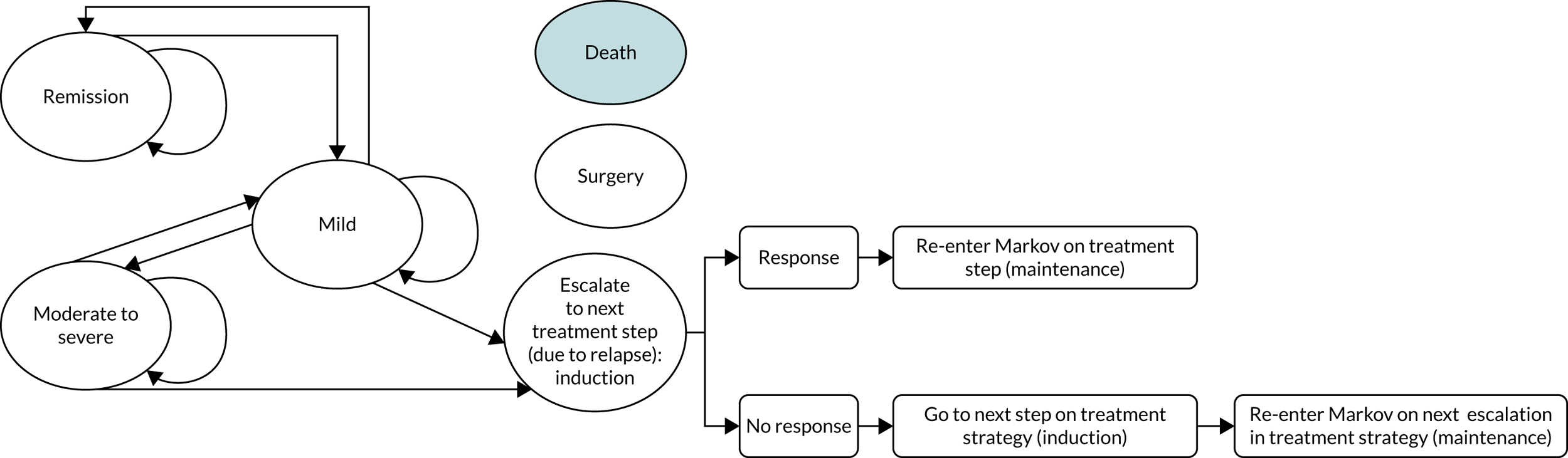
Responders to their first induction therapy enter the maintenance cohort model in the remission (CDAI score of < 150), mild (CDAI score of 150–220) or moderate to severe (CDAI score of 221–600) health states. Patients can then move between these states during maintenance therapy, reflecting the different levels of response to maintenance therapy. The probability of patients transitioning between these states is also dependent on the treatment class received.
Non-responders to induction therapy escalate to induction in the next step of their treatment strategy, to which they can become responders or non-responders. Patients receiving their second induction therapy are assessed for response and escalation to the next treatment step, similar to patients receiving their first induction therapy (portrayed by the loop in Figure 8).
Patients in the mild and moderate to severe states are at risk of escalating to the next treatment step, and death is the absorbing state in the model.
Escalation to the next treatment step occurs, therefore, for one of two reasons in the model: lack of response to induction therapy or relapse while on maintenance therapy. The former is a default assumption in the model, as 100% of patients who do not respond to induction therapy move to the next step in their treatment strategy. The latter is not estimated explicitly in the economic model, but instead it is assumed that time to treatment escalation (TTE) (taken from Biasci et al. 50) reflects a relapse while on maintenance treatment. This issue is further discussed in Time to treatment escalation in high- and low-risk patients.
The EAG had to estimate surgical events as a standalone outcome in the model. This modelling simplification means that patients do not explicitly leave their health state in a specific cycle to move to the surgery state. Instead, in every model cycle, a proportion of surgeries is estimated, and the associated costs and impact on patients’ quality of life are calculated (this is further discussed in Effectiveness of top-down compared with step-up treatment strategy on time to treatment escalation). Patients who receive surgery in the model have an increased probability of dying which is associated with the procedure.
The economic assessment is taken from the perspective of the NHS and Personal Social Services, and both costs and benefits are discounted at 3.5% per annum. Cycle length in the model is 2 weeks, and the time horizon of the model is 65 years (when modelled patients would be 100 years old).
Clinical input parameters
As mentioned in Model structure, the economic model is ultimately assessing the cost-effectiveness of TD therapy compared with SU therapy for high-risk patients. However, the EAG did not identify any direct evidence on the latter; thus, the clinical data informing the economic analysis had to be derived from multiple sources. This approach is not ideal and creates a patchwork network of evidence, introducing uncertainty to the economic results. The EAG anticipates that this problem will be (at least partially) overcome when the results from the PROFILE trial are available to populate the economic model. The EAG considers that the PROFILE study should also be able to inform the costs and health consequences of ‘misdiagnosing’ patients as high and low risk, thereby allowing an estimation of the cost-effectiveness of undertreating or overtreating CD patients in the NHS.
The EAG notes that the clinical input parameters in the base-case economic model for PredictSURE-IBD and in the scenario analysis for IBDX are the same. The only difference in the cost-effectiveness analyses of the two diagnostic tests is the cost of the test.
The EAG found two main sources of evidence that could be used to model TTE and time to surgery (TTS). Nevertheless, each source could only partially inform the TTE and TTS analyses in the economic model. Whereas the Biasci et al. 50 paper could inform TTE and TTS according to high and low risk of CD complications (for the SU strategy), the D’Haens et al. 35 paper (and its 10-year follow-up study120) could inform TTE and TTS according to TD and SU treatments (for a population with a mixed risk of disease complications).
The Biasci et al. 50 study enrolled patients with active CD who were not receiving concomitant corticosteroids, IMs or biological therapy. Forty patients received treatment with a corticosteroid, followed by an IM (of whom 50% escalated to treatment with an anti-TNF). This treatment strategy was considered to be a good representation of the first three steps in the SU pathway described by the EAG’s clinical experts. Biasci et al. ’s50 included TTE outcomes, however, differentiated outcomes not by treatment strategy, but by risk of severe disease course. Therefore, the data provided in the study could only potentially inform the difference in TTE and TTS for high- compared with low-risk patients receiving SU.
The D’Haens et al. 35 study evaluated the clinical efficacy of early immunosuppression compared with conventional therapy. The study was a 2-year open-label randomised trial at 18 centres in Belgium, the Netherlands and Germany, and randomly assigned 133 patients to either early combined immunosuppression or conventional treatment. The study collected outcome data on time to relapse for 62 patients: 20 patients who received conventional therapy and 42 patients assigned to combined immunosuppression, who received three infusions of infliximab (5 mg/kg of body weight) at weeks 0, 2 and 6, with azathioprine. Additional treatment was given with infliximab and, if necessary, corticosteroids to control disease activity.
Patients assigned to conventional management received corticosteroids followed, in sequence, by azathioprine and infliximab, if needed. If patients responded to treatment with corticosteroids, treatment tapering was initiated. If patients’ symptoms worsened during the course of corticosteroid tapering and did not respond to an increase in treatment dose, treatment with azathioprine was initiated (2–2.5 mg/kg per day). Patients who relapsed after withdrawal of corticosteroids were given a second course of corticosteroids in combination with azathioprine. Any patient who remained symptomatic after 16 weeks of azathioprine treatment received an induction course of infliximab (5 mg/kg body weight at weeks 0, 2 and 6) and continued antimetabolite treatment.
Therefore, although the study forms a reasonable evidence base for measuring the relative effectiveness of anti-TNF versus corticosteroid followed by IM and anti-TNF, it does not differentiate outcomes by risk of severe disease course, only by treatment received. 35 Furthermore, the treatment sequences included in the D’Haens et al. 35 trial only partially reflect the TD and the SU strategies as described by the clinical experts advising the EAG; the TD and SU strategies in the UK include an initial induction with steroid treatment. In the UK, these clinical strategies are differentiated after steroid treatment only, whereby TD patients are given treatment with an anti-TNF and SU patients are given an IM treatment. As the TTE data taken from D’Haens et al. 35 were based on time to relapse, the EAG assumed that relapse meant failure on first treatment in both strategies in the study and, therefore, time to relapse data were based on the comparison of anti-TNF with corticosteroids. Furthermore, D’Haens et al. 35 included a mix of high- and low-risk patients. This means that low-risk patients were overtreated with first-line anti-TNF. The study concluded that TD patients took a longer time to relapse than SU patients.
The Hoekman et al. 120 study was a retrospective review of medical records of patients included in the D’Haens et al. 35 trial, which collected data on hospitalisation, flares, surgery, clinical activity and other outcomes for a median follow-up of 10 years. The study concluded that, in the long term, no difference was found in clinical remission rate, endoscopic remission, hospitalisation, surgery or new fistulas. During the follow-up period, the proportion of patients who received an IM was similar across arms (88% SU and 86% TD; p = 0.76), while the use of anti-TNF was higher in the SU arm than in the TD arm (73% vs. 54%; p = 0.04). However, the authors explained that the lower use of anti-TNF agents observed during long-term follow-up in TD-treated patients was not directly relevant to current clinical practice because it was related to the previous practice of episodic anti-TNF treatment with no anti-TNF maintenance.
Given that the EAG did not find any sources of evidence combining CD outcomes differentiated by risk of disease and by treatment received, the EAG had to choose between Biasci et al. ,50 which differentiated outcomes by patients’ risk of severe disease course, and D’Haens et al. 35 (and Hoekman et al. 120), which differentiated outcomes by type of treatment strategy received (a proxy for TD vs. SU) to form the baseline treatment measure in the model. The EAG chose Biasci et al. 50 because it considered that estimating a relative treatment effect of TD compared with SU (from D’Haens et al. 35 for TTE and from Hoekman et al. 120 for TTS) and applying it to a different population was based on a less flawed assumption than estimating the relative risk of disease to be applied in a different group of patients. Furthermore, given that the purpose of the diagnostic tests is to categorise patients into high- and low-risk disease, the EAG’s preference was to prioritise robust evidence for this component of the model. Additionally, the D’Haens et al. 35 data did not cover the sequences of treatments included in the TD or the SU approaches as per clinical practice in the UK.
The EAG discusses the TTE and TTS data analysis undertaken using Biasci et al. ,50 D’Haens et al. 35 and Hoekman et al. 120 in the next subsections of the report. However, the EAG notes that the caveat of the results of the theoretical economic analysis is the lack of robust evidence available for the relative clinical effectiveness of TD compared with SU strategies for the population defined in the scope.
Time to treatment escalation in high- and low-risk patients
Of the 105 patients included in the Biasci et al. 50 IPD provided to the EAG, 88 were newly diagnosed with CD (Figure 10). Of these 88 patients, 75 received initial treatment with corticosteroids. The EAG also removed 35 patients from the analysis who never received a subsequent IM after corticosteroids (leaving 40 patients for the TTE analysis; see Figure 10). The EAG did not model time to escalation from corticosteroid treatment to IM (SU) or to anti-TNF (TD). This decision was based on the fact that the economic analysis is driven by the impact of giving high-risk patients TD therapy compared with SU therapy; therefore, considering that 100% of patients in the high-risk group would receive initial treatment with corticosteroids, the impact of treatment would cancel out across the TD high-risk and the SU high-risk arms, as the treatment effect from D’Haens et al. 35 was applied for IM compared with anti-TNF (and subsequent treatment steps) in the model only.
FIGURE 10.
Selection of patients from Biasci et al. 50 for time to escalation analysis. Blue shading indicates the number of patients remaining for TTE analysis.

The TTE data from Biasci et al. 50 were used to estimate time to next treatment step in all SU arms of the economic model (the test and no-test arms of the model and the high- and low-risk arms of the no-test model). To extrapolate TTE data to the model time horizon, the EAG analysed the IPD data to create TTE data for time to first escalation.
The final data set comprised 23 high-risk and 17 low-risk patients, with 16 escalation events observed in the high-risk group and four escalation events observed in the low-risk group). The EAG censored patients who did not have an escalation event. Time to treatment escalation was statistically significantly different between the high- and low-risk arms (p = 0.02). Overall, the EAG notes that both the number of patients and events in the analysis are very small and, therefore, the results of the EAG’s analysis need to be interpreted with extreme caution.
The EAG had to make some assumptions in its base-case analysis to use the Biasci et al. 50 data. These consisted of the following:
-
Treatment escalations in the model correspond to patients’ relapse while on current treatment (or a flare).
-
Patients have the same baseline probability of escalating to the next step in the SU treatment strategy (which is estimated from time to first escalation in Biasci et al. 50) regardless of the number of previous escalations.
The EAG acknowledges that these assumptions are a simplification of clinical reality, where time to escalation is likely to depended on the number of previous treatments. Nonetheless, given that patients in remission are assumed not to escalate treatment while they are on maintenance therapy, and given that the probability of remission changes according to treatment step, the total number of patients escalating treatment differs by treatment step, across all treatments.
Furthermore, as mentioned in this section, the EAG did not find any sources of evidence containing complete treatment sequences (for the TD and SU strategies), which would have allowed the estimation of TTE by treatment step and response status.
The EAG considered the possibility of splitting the Biasci et al. 50 TTE data by first escalation and second (or more) escalations. However, the number of events in the second (or more) escalations data set was too small (three events overall) and, therefore, the Kaplan–Meier data were deemed unreliable. The EAG also considered the possibility of splitting the TTE data according to patients’ initial response to treatment (by using a proxy of time to escalation in Biasci et al. 50). However, the EAG decided against this given the already very small size of the Biasci et al. 50 population for the TTE data available.
The TTE Kaplan–Meier data were fitted with exponential, Weibull, Gompertz, log-logistic, log-normal and generalised gamma models in accordance with guidance in NICE Decision Support Unit Technical Support Document 14. 121 The fit of each parametric model was compared with the observed Kaplan–Meier data, and statistical fit was assessed using the Akaike information criterion (AIC) and the Bayesian information criterion (BIC). The fitted curves were also validated by clinical expert opinion. Given the small numbers of patients and events across treatment arms, the curves were initially fit dependently for high- and low-risk patients. However, clinical expert opinion provided to the EAG supported the use of different models for high- and low-risk patients, as the clinical expectation is that all high-risk patients will eventually escalate from IM to anti-TNF but that only 65% of low-risk patients will escalate from IM. Among the best-fitting curves, those that support the clinical predictions are the Gompertz curve for low-risk patients and the log-normal curve for high-risk patients.
The EAG acknowledges that the Decision Support Unit advises against fitting different models to same-study arms unless a strong clinical argument exists. The EAG considers that such a clinical argument is present in this case (as supported by clinical expert opinion) and that the nature of the modelled outcome (TTE for different disease severity course) lends plausibility for the difference in the curves’ shape.
According to the AIC and BIC statistics reported in Appendix 2 (see Tables 28 and 29 for high- and low-risk patients, respectively), the three best-fitting models to the high-risk Kaplan–Meier data from Biasci et al. 50 are log-normal, log-logistic and gamma, while gamma, exponential and Gompertz are the three best-fitting models for the low-risk group. The EAG chose the log-normal (for high-risk patients) and the Gompertz (for low-risk patients) curves.
Effectiveness of top-down compared with step-up treatment strategy on time to treatment escalation
To estimate TTE in the high-risk TD strategy arm of the model, the EAG applied a hazard function, derived from D’Haens et al. ,35 to reflect the treatment effect of TD compared with SU treatment on escalations.
The EAG had to make some assumptions in its base-case analysis to use the D’Haens et al. 35 data. These consisted of the following:
-
Randomisation has resulted in balanced populations of high- and low-risk patients in each treatment group.
-
The relative treatment effect of TD compared with SU in a mixed-risk population is the same as the relative treatment effect of TD compared with SU in a high-risk population.
-
Time to relapse is a proxy measure for time to next treatment escalation.
-
The effectiveness of the treatment strategies in D’Haens et al. 35 is a proxy for the treatment effectiveness of the first step in the TD and SU strategies modelled.
The first regimens in the treatment strategies included in D’Haens et al. 35 (corticosteroid vs. anti-TNF) are likely to overestimate the relative effectiveness of the modelled first step treatment in the TD strategy (anti-TNF) compared with the first step in the SU strategy (IM). Counterbalancing the direction of this bias, the anti-TNF regimen in the study consisted of only three infliximab infusions (at weeks 0, 2 and 6), followed by maintenance monotherapy treatment with azathioprine or methotrexate and additional infliximab infusions in the case of clinical deterioration only. As pointed out by the authors of the Hoekman et al. 120 study, since the D’Haens et al. 35 trial, clinical practice has evolved to continued maintenance treatment with infliximab (in cases of a favourable response to induction treatment), which is consistent with UK clinical practice and NICE guidelines. Therefore, although it is not possible to anticipate the overall magnitude or direction of these biases in the data, they work in opposite directions, and so at least partially alleviate the impact of the overall bias in the analysis.
The EAG digitised the time to relapse Kaplan–Meier data in D’Haens et al. 35 and used the number of patients at risk provided in the study to simulate the pseudo-individual patient data using the Guyot et al. 122 method and the algorithm in the survHE R package [R version 1.0.65; The R Foundation for Statistical Computing, Vienna, Austria; https://CRAN.R-project.org/package=survHE (accessed June 2019)]. Subsequently, the EAG fitted a variety of parametric curves to the Kaplan–Meier data (Figure 11) using the process described in Time to treatment escalation in high- and low-risk patients. The EAG notes that the time to relapse was statistically significantly different between the TD and SU arms (p = 0.04).
FIGURE 11.
Time to relapse estimated by the EAG.
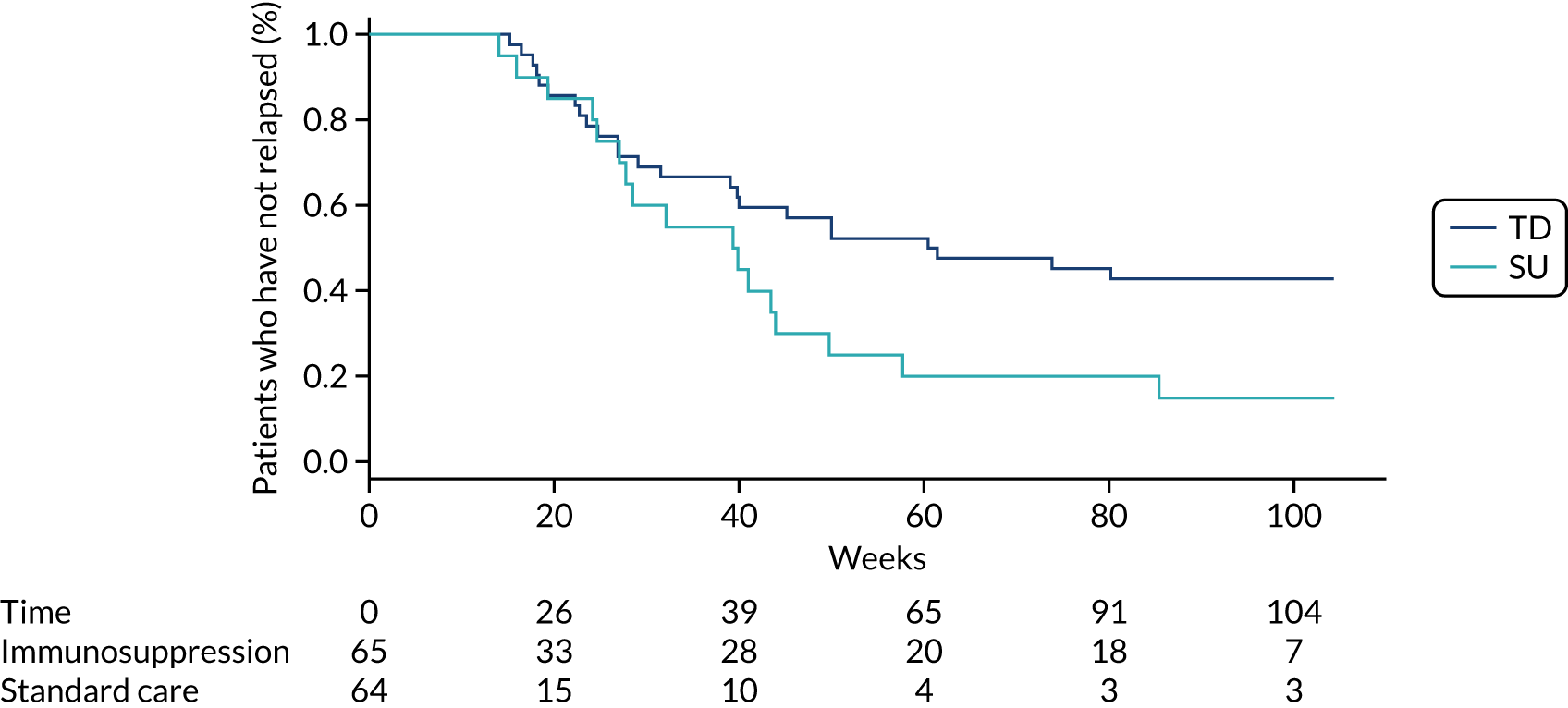
The EAG restricted the modelling of the D’Haens et al. 35 data to dependently fitted survival models only. This was to ensure that the relative effect estimated between the two treatment groups was a scaling factor only. Allowing both the scale and the shape of the curves to vary would have resulted in implausible estimates of a relative effect, particularly in the probabilistic sensitivity analysis (PSA), where samples of the curves could theoretically cross.
Given that no relapse events took place for the first 14 weeks of the analysis, both Kaplan–Meier curves show a plateau from week 0 to week 14 (see Figure 11). This made the curve-fitting exercise challenging as the shape of the fitted curves was heavily influenced by the plateau.
According to the AIC and BIC statistics (see Appendix 2, Table 30), the three best-fitting models to the time to relapse Kaplan–Meier data were log-normal, log-logistic and gamma. Figure 12 shows the fitted curves for TD patients along with the time to relapse Kaplan–Meier data, and Figure 13 shows the equivalent curves for SU patients. The log-normal model provided the second-best fit according to AIC and BIC statistics. Given that the TTE data were fitted with a log-normal model and that the hazard function derived from D’Haens et al. 35 was to be applied to the TTE data, the EAG chose the log-normal curve.
FIGURE 12.
Step-up time-to-relapse curves fitted with Weibull, log-normal and log-logistic.
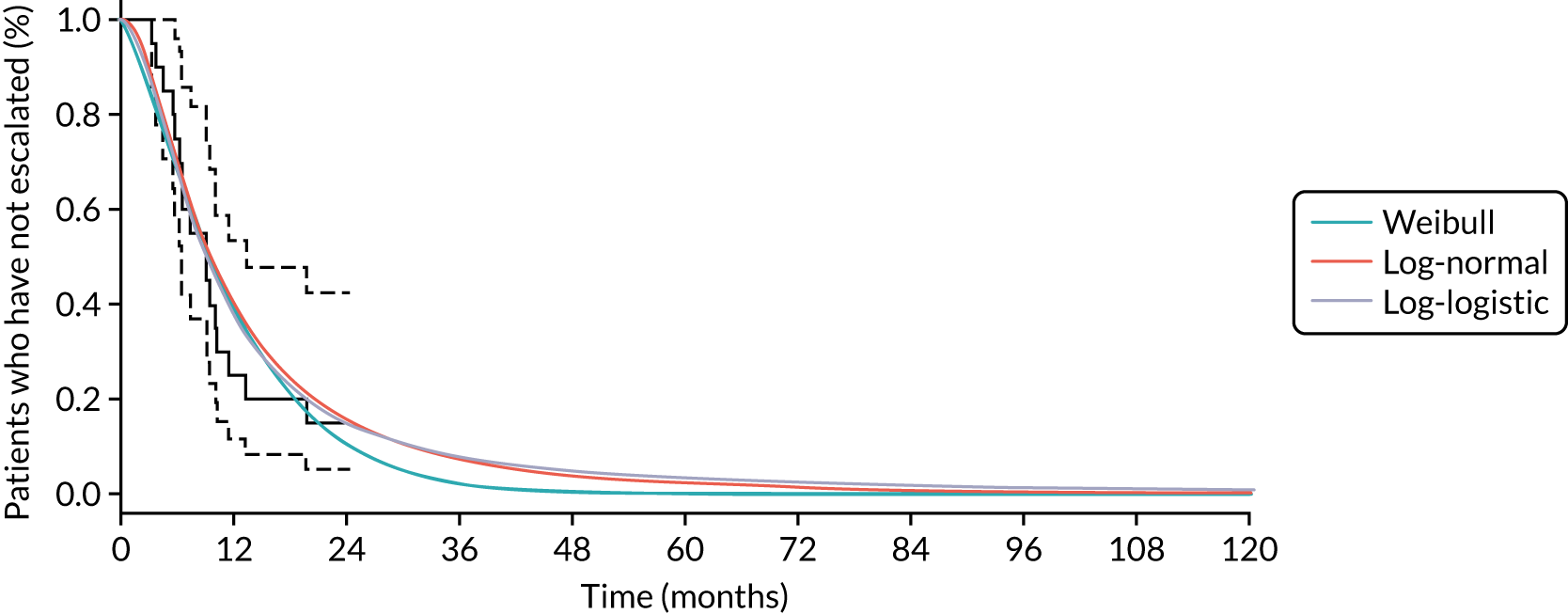
FIGURE 13.
Top-down time-to-relapse curves fitted with Weibull, log-normal and log-logistic.

Given that none of the three best-fitting curves provided a great visual fit to the Kaplan–Meier data (owing to the plateau observed for the initial 14 weeks), the EAG explored the option of truncating the Kaplan–Meier data at 12 weeks (Figure 14) to fit the survival curves.
FIGURE 14.
Time to relapse (truncated) estimated by the EAG.

According to the AIC and BIC statistics (see Appendix 2, Table 31), the three best-fitting models to the truncated Kaplan–Meier data were gamma, log-normal and Gompertz. The log-normal provided the best fit according to the AIC and BIC statistics. Figure 25 (see Appendix 3) shows the fitted curves for TD patients along with the time to relapse Kaplan–Meier data, and Figure 26 (see Appendix 3) shows the equivalent curves for SU patients.
Although the curves fitted to the truncated data provide a better visual fit, the EAG was wary of eliminating 12 weeks of time to relapse data from the analysis. Therefore, the EAG ran the economic analysis with both sets of log-normal curves (i.e. based on the truncated and the original Kaplan–Meier data) and concluded that the impact on the final ICER was minimal. Thus, the EAG decided to use the non-truncated log-normal curves in the model (Figure 15).
FIGURE 15.
Time-to-relapse curves fitted with log-normal curves.

The EAG used the log-normal fitted curves to estimate a hazard function to apply to the high-risk TD arm of the economic model. The EAG applied the relative hazard function to TTE curves in the first step in the TD strategy (anti-TNF). The TTE associated with the remaining treatment steps in both the TD and SU arms was assumed to be the same as TTE for anti-TNF in the TD arm (see Appendix 4, Figure 27).
The underlying assumption in the EAG’s base-case approach is that high-risk patients who initiate treatment with IMs (SU arm) escalate treatment quicker than high-risk patients who initiate treatment with anti-TNF (supported by the data presented in D’Haens et al. 35); however, once SU patients initiate treatment with anti-TNF (their second treatment step), they ‘catch up’ with patients on the TD treatment strategy.
As some high-risk patients who receive SU treatment respond to IM treatment (see Effectiveness of induction and maintenance therapies), having the additional IM step in the SU strategy is advantageous to patients in the EAG’s base-case analysis as patients still subsequently receive treatment with biologics, which are assumed to have the same benefit as biologics in the TD arm. Given the paucity of data to substantiate any further benefits of subsequent treatment steps in the TD and SU approaches, the EAG considered this to be the most conservative modelling approach.
As mentioned in Intervention and comparator and Time to treatment escalation in high- and low-risk patients, the first treatment step modelled in the TD sequence is anti-TNF, while the first step in the SU strategy is IM treatment. Therefore, there is no modelling of escalation from corticosteroids, nor is there any difference captured across TD and SU arms in time to corticosteroid failure and beginning of first treatment.
The assumption that all patients receive steroids but that only patients in the SU strategy would receive a full course of treatment, rather than being switched to biologics in the TD strategy as soon as the test results become available, was not modelled. Including this step in the model for SU only would add further benefits to the SU strategy as it would allow patients a further chance to respond, as well as reducing the chances of receiving the highly expensive biologic treatments (also considering the very low cost of corticosteroids).
The specialist committee members raised a concern about the potential risk of additional complications associated with the SU strategy given the delay in initiating treatment with biologics. The EAG notes that Hoekman et al. concluded that, in the long term (10-year follow-up), there was no difference found in complications, such as new fistulas or surgery, between the TD and SU arms. Furthermore, although not based on comparative evidence, the Biasci et al. 50 IPD reported very few events that required surgery, and no patients underwent more than one surgery during their follow-up period while receiving a SU strategy.
Therefore, the EAG considers that the specialist committee members’ view that early biologics are better than later biologics may apply to those who do not respond to treatment with IMs only. However, removing this step entirely from the model would mean removing the benefit for those who do respond to IMs. As well as this, highly expensive biologics would be added that are potentially unnecessary for those who respond well to IMs.
Nonetheless, the EAG has varied these assumptions in a range of scenario analyses described in Chapter 5, Scenario analyses. Regarding the measure of treatment effectiveness of TD versus SU in the model, the EAG ran three scenario analyses in the model:
-
High-risk patients on anti-TNF after IMs (second step in SU arm) do not do as well as high-risk patients on first-line anti-TNF (first step in TD arm) and, thus, the former group escalate treatment quicker than the latter. Given that the EAG did not find any data to support this reduction in relative treatment effect, a theoretical estimate of half of the base-case relative hazard was assumed (see Appendix 4, Figure 28). Comparison of TTE curves across treatment strategies for high-risk patients (scenario analysis 1 with SU time to escalation from step 2 estimated with half of the base-case relative hazard; Figure 28);
-
Combining scenario 1 with the base-case approach, the EAG assumed that high-risk patients on TD derive a benefit during the first step of the treatment strategy only (anti-TNF in TD compared with SU patients on IM treatment); however, once patients have moved on to the second step in both strategies there is no relative benefit for TD compared with SU. This scenario differs from the base case as the benefit assumed is the same as that used in scenario 1 (see Appendix 4, Figure 29).
-
Assuming that high-risk patients do not respond to treatment with IMs; that is, 100% of patients who receive SU do not respond to treatment and therefore escalate to anti-TNF after induction with IMs.
The three TTE curves for high-risk patients used in the base case and both scenario analyses are reported in Figure 16. The results of these analyses are reported in Chapter 5.
FIGURE 16.
High-risk curves with TD vs. SU effect.
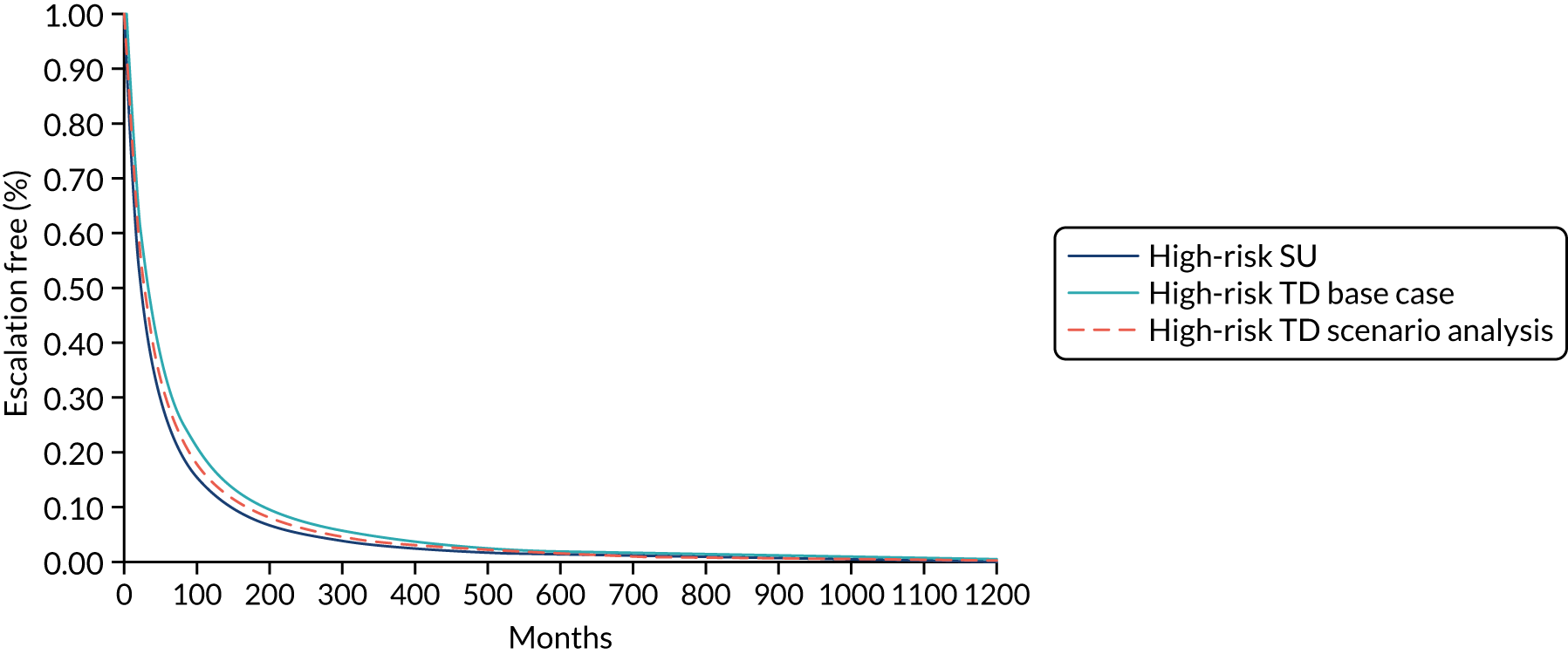
Effectiveness of induction and maintenance therapies
To estimate the effectiveness of the different therapies included in the modelled TD and SU strategies, the EAG sought evidence informing the probability of response and remission for the induction and maintenance periods of each treatment step in the corresponding sequences. The EAG also aimed to identify the proportions of patients expected to be in either a mild or moderate to severe health state among those who experienced a response.
Initially, advice was sought from clinical experts to verify clinical practice in England relating to the administration, scheduling and doses of the SU and TD strategies in the induction of remission of CD, and treatments given to maintain response or remission. Owing to time and resource constraints, a pragmatic approach was taken to identify studies that had data on clinical outcomes for people receiving induction and maintenance therapies for CD.
A search of electronic databases was carried out to identify systematic reviews of SU or TD treatments for CD. The following electronic databases were searched from inception to 14 June 2019:
-
MEDLINE (MEDLINE and Epub Ahead of Print, MEDLINE In-Process & Other Non-Indexed Citations and Daily and Versions; via Ovid)
-
EMBASE (via Ovid)
-
CENTRAL and CDSR.
Search strategies for electronic databases included MeSH terms for CD and free-text terms for CD and for SU and TD strategies; search strategies are provided in Appendix 5 (see Tables 34–37). The searches retrieved 507 records (post deduplication), which were imported into Rayyan QCRI for the assessment of titles and abstracts by two independent reviewers. The review of titles and abstracts generated 15 studies for assessment of the full-text publication; reasons for excluding the 14 studies are provided in Appendix 5 (see Table 37). Two reviewers independently identified one systematic review as the most comprehensive review to be used as a source of studies on SU and TD treatments. 123 The full-text publication of all studies listed in Tsui and Huynh123 was assessed independently by two reviewers.
For a study to be included in the analysis of clinical effectiveness of induction and maintenance strategies, it should have evaluated therapies at (or similar to) the dose and schedule outlined in the licence of the drug for use in the management of CD in England. No IM has marketing authorisation for use in CD in England; instead, doses reported in the British National Formulary124 were applied to determine the inclusion of studies. For induction therapy, data should be reported for those with a new or recent diagnosis of CD and with moderate to severe activity of CD at baseline, as per the population of interest in the economic evaluation. For the SU treatment pathway, those moving on to receive second-line biological therapy should have failed treatment with first-line anti-TNF biologic, as per NICE guidance. 31
TA352125 (for vedolizumab) and TA456119 (for ustekinumab) were the sources used to identify studies evaluating the clinical effectiveness of non-anti-TNF biological therapies, and also as supplementary sources on anti-TNF therapies as used in SU treatment. The full texts of all studies included in the network meta-analyses presented in TA352 and TA456 were reviewed independently by two reviewers for potential relevance.
One RCT identified by the SLR was deemed relevant to the economic evaluation. 36 The RCT provided results on effectiveness of induction therapy with IMs alone for SU treatment and on anti-TNF monotherapy for TD strategy.
Six additional studies included in TA352125 and TA456119 were considered to be relevant to inform estimates of clinical effectiveness of induction treatment; two RCTs reported results for anti-TNF biological therapy with or without IM in people naive to anti-TNF,126,127 and four RCTs provided data on ustekinumab or vedolizumab with or without IMs as a second-line biological therapy in people who failed treatment with an anti-TNF. 128–131
Three studies from TA352125 and TA456119 informed on maintenance of response or remission for SU treatment: one RCT evaluated anti-TNF biologics with or without IMs132 and two RCTs assessed ustekinumab or vedolizumab with or without IMs. 128,129
The goal of the economic evaluation is to compare the cost-effectiveness of the SU and TD treatment pathways rather than to determine which therapy within a class of treatments is the most effective at each step. Given the aim of the economic evaluation, and considering the available evidence, a class effect was assumed for each class of treatments (i.e. IM, anti-TNF ± IM, and second-line biologic ± IM) to simplify the complexity of the analyses. Clinical experts fed back that the assumption of a class effect was reasonable. Additionally, the EAG considered using the network meta-analyses reported in TA352125 (vedolizumab) and TA456119 (ustekinumab) as potential sources of estimates of clinical effectiveness for anti-TNF and non-anti-TNF biological therapies in the economic model. However, after reviewing the underlying trials as described above, the EAG had concerns around the generalisability of the studies selected (see Appendix 5 and Table 38 for more details). Considering the network meta-analyses (NMAs) reported in TA352 and TA456, and given the EAG’s assessments of the trials included, the EAG has reservations around the reliability of the results of network meta-analyses for use in the economic model.
Data were extracted from the included studies by one reviewer and validated by a second reviewer. Substantial clinical heterogeneity was identified across the studies included for both induction and maintenance analyses, given that studies:
-
enrolled a mix of people with a new or recent diagnosis of CD and those with an established diagnosis
-
evaluating treatment with non-anti-TNF biological therapies included people who had failed treatment with more than one anti-TNF (26–63% had failed more than one anti-TNF), which does not reflect clinical practice in England, where patients not responding to treatment with anti-TNF biologics move to a different class of biologic rather than receive a second anti-TNF
-
assessing maintenance treatment evaluated different doses and schedules.
Given the anticipated heterogeneity across the studies, a random-effects model was selected for synthesis of data. Data for each treatment bundle were synthesised using single-arm meta-analysis in Comprehensive Meta-Analysis version 3 (Biostat, Englewood, NJ, USA).
The pragmatic search for evidence did not provide a complete set of data to allow an estimation of the transitions between health states for all treatment steps over time. No studies provided the proportional split of patients between the mild and moderate to severe states for those who achieved a response or those who maintained their response. Furthermore, the only treatment step that provided a complete set of response and remission probabilities for both induction and maintenance was the anti-TNF step for the SU pathway. A summary of the required parameter inputs for the model populated by the data extracted from the included studies, where available, is given in Appendix 6 (see Table 39).
Despite the limitations identified in the network meta-analysis in TA352,125 this data set proved to be the best available to complete the required response and remission outcomes for the economic model. The EAG identified complete data sources in table 7.3.1.4 of the company’s submission for TA352,125 which provided estimates based on network meta-analyses for induction and maintenance, and separated the outcomes by an anti-TNF-naive population and an anti-TNF-failure population. The EAG considered it unreliable to combine different data sources for a particular class of treatment and thus it retained only the SU anti-TNF data from its meta-analysis, which was the only complete set of data. The EAG also applied this to the TD anti-TNF treatment, but used TA352 data for biologics and IMs.
For the missing SU data, IM outcomes were informed by the conventional therapy group for the anti-TNF-naive population and biologics were informed by vedolizumab from the anti-TNF-naive population, the latter also being used for TD biologics. For the second-line biologics, the same transitions as the first-line (non-anti-TNF) biologics were assumed to apply to both SU and TD.
The combined set of outcomes that the EAG used to estimate transition probabilities is given in Table 8. Note that the response values were recalculated to exclude those in remission, as was the case in table 7.3.1.4 of the company’s submission in TA352. 125
Clinical outcome | Induction (%) | Maintenance (%) | ||
---|---|---|---|---|
Response | Remission | Response | Remission | |
TD | ||||
Biologics | 32 | 13 | 2 | 28 |
Anti-TNF | 26 | 37 | 10 | 33 |
SU | ||||
Biologics | 32 | 13 | 2 | 28 |
Anti-TNF | 26 | 37 | 10 | 33 |
IM | 23 | 16 | 15 | 25 |
The next step in estimating transition probabilities was to estimate the proportion of patients who were in the moderate to severe health state or in the mild health state, both after achieving a response and at the end of the maintenance phase. The EAG did not identify any data in the trials from the SLR, so instead the EAG used the values presented in TA352125 as an estimate and assumed the same value for both induction and maintenance, given the lack of more robust data sources. The company from TA352 reported that 21.2% of patients in the mixed population were in the moderate/severe health state after response. The EAG did not consider there to be sufficient evidence to apply specific values for treatment-naive and treatment-failure patients, so it applied the value based on the combined patient population for all treatments.
The resulting induction and the maintenance vectors for each treatment when this estimate of the mild and moderate to severe split is applied are given in Table 9.
Clinical outcome | Response (%) | |||
---|---|---|---|---|
Remission | Mild | Moderate to severe | No response | |
Induction | ||||
TD | ||||
Biologics | 13 | 25 | 7 | 55 |
Anti-TNF | 37 | 20 | 5 | 38 |
SU | ||||
Biologics | 13 | 25 | 7 | 55 |
Anti-TNF | 37 | 20 | 5 | 38 |
IM | 16 | 18 | 5 | 62 |
Maintenance | ||||
TD | ||||
Biologics | 28 | 1 | 0 | 70 |
Anti-TNF | 33 | 8 | 2 | 57 |
SU | ||||
Biologics | 28 | 1 | 0 | 70 |
Anti-TNF | 33 | 8 | 2 | 57 |
IM | 25 | 12 | 3 | 60 |
The economic model developed by the EAG applies transitions for those who are responding to treatment and deals with those who do not respond to treatment or who lose response to treatment separately based on TTE data. Therefore, to estimate the transitions for responders (including remission), the data for the three responder states were taken and reweighted to sum to 100%. These data were then used to perform the estimation of transitions.
The Optim function from the Stats package in R was used to perform the estimation of transitions. This was done in two stages: first, to optimise a 52-week transition matrix without constraints and second, to estimate 2-weekly transitions with constraints applied to prevent transitions progressing across two health states in one model cycle. For example, transitions could go from remission to mild or mild to moderate/severe, but not from remission straight to moderate/severe. This was based on clinical expert opinion that the latter would not happen in a period as short as 2 weeks.
The optimisation approach for both steps required an initial transition matrix to be defined with initial values, which were varied by the Optim function to minimise a specified objective function. The objective function was defined for the first step as the sum of the squared difference between the product of the induction vector and the 52-week transition matrix, and the maintenance vector; and for the second step as the sum of the squared differences between the values of the estimated 52-week transition matrix and the 26th power of the estimated 2-week transition matrix.
The initial matrix values applied in the optimisation can have an impact on the resulting transitions derived from the optimisation, and some starting values provided poor estimations or even provided negative probabilities. Therefore, the EAG varied these values until plausible values were generated that produced relatively accurate estimations of the maintenance vectors when the estimated transition matrices were applied to the induction vectors. The initial values were specified as the parameters of beta distributions that were linked to the transition matrix entries to ensure that values were between zero and one. The minimum values of the objective functions and the resulting predicted maintenance outputs are shown in Table 40 (see Appendix 6) as a measure of goodness of fit. The resulting 2-weekly transition probabilities for each treatment are given in Table 10.
Annual transitions | Remission | Mild | Moderate to severe |
---|---|---|---|
TD | |||
Anti-TNF | |||
Remission | 0.9787 | 0.0213 | 0.0000 |
Mild | 0.1059 | 0.8941 | 0.0000 |
Moderate to severe | 0.0000 | 0.0346 | 0.9654 |
First- and second-line biologics | |||
Remission | 0.9982 | 0.0018 | 0.0000 |
Mild | 0.1136 | 0.8864 | 0.0001 |
Moderate to severe | 0.0000 | 0.0795 | 0.9205 |
SU | |||
IM | |||
Remission | 0.9736 | 0.0264 | 0.0000 |
Mild | 0.0616 | 0.9302 | 0.0082 |
Moderate to severe | 0.0000 | 0.0482 | 0.9518 |
Anti-TNF | |||
Remission | 0.9787 | 0.0213 | 0.0000 |
Mild | 0.1059 | 0.8941 | 0.0000 |
Moderate to severe | 0.0000 | 0.0346 | 0.9654 |
First- and second-line biologics | |||
Remission | 0.9982 | 0.0018 | 0.0000 |
Mild | 0.1136 | 0.8864 | 0.0001 |
Moderate to severe | 0.0000 | 0.0795 | 0.9205 |
The EAG also performed a scenario analysis that used only data from TA352125 to inform the induction and maintenance vectors. The transition probabilities were re-estimated using these data, and these data, along with the induction vectors, were applied in the model to test the impact on the results. The induction and maintenance vectors for the scenario are given in Tables 41 and 42 (see Appendix 6), respectively, and the updated transitions for TD and SU are given in Table 11. The results of the scenario analysis are presented in Chapter 5, Scenario analyses.
Annual transitions | Remission | Mild | Moderate to severe |
---|---|---|---|
Top down | |||
Anti-TNF | |||
Remission | 0.9691 | 0.0309 | 0.0000 |
Mild | 0.1665 | 0.8335 | 0.0000 |
Moderate to severe | 0.0000 | 0.0548 | 0.9452 |
First- and second-line biologics | |||
Remission | 0.9982 | 0.0018 | 0.0000 |
Mild | 0.1136 | 0.8864 | 0.0001 |
Moderate to severe | 0.0000 | 0.0795 | 0.9205 |
Step up | |||
IM | |||
Remission | 0.9736 | 0.0264 | 0.0000 |
Mild | 0.0616 | 0.9302 | 0.0082 |
Moderate to severe | 0.0000 | 0.0482 | 0.9518 |
Anti-TNF | |||
Remission | 0.9691 | 0.0309 | 0.0000 |
Mild | 0.1665 | 0.8335 | 0.0000 |
Moderate to severe | 0.0000 | 0.0548 | 0.9452 |
First- and second-line biologics | |||
Remission | 0.9982 | 0.0018 | 0.0000 |
Mild | 0.1136 | 0.8864 | 0.0001 |
Moderate to severe | 0.0000 | 0.0795 | 0.9205 |
Time to surgery in high- and low-risk patients
The goal of including surgical events in the model was to capture the impact of TD treatment in terms of potentially reducing the need for surgery in high-risk patients. Clinical expert opinion provided to the EAG reflected that CD patients can receive surgery for multiple reasons, including having exhausted other treatment options or the severity of disease (or symptoms) related to developing strictures or perforation of the bowel.
Conversely, the EAG acknowledges that surgery might have a beneficial impact on patients’ quality of life as there is a disease ‘reset’ for a period of time after surgery. Even though the EAG has not captured this potential benefit of surgery in the economic analysis, it notes that to do so would benefit the SU strategy, as a higher proportion of patients receive surgery in the SU arm than in the TD arm of the model.
The EAG analysed the IPD available for the 88 patients in the Biasci et al. 50 cohort for surgical events and removed one patient who had surgery at study entrance. The EAG began by analysing the data separately by risk of disease complications; however, it considered the data insufficiently mature to be able to separate TTS by high- and low-risk groups and, thus, it pooled the TTS data across both study arms. The implication of this approach is that TTS is the same for high- and low-risk patients, which is unlikely to be an accurate reflection of clinical reality. Nonetheless, the estimated treatment effect of TD compared with SU was applied to the baseline population of high-risk patients on SU treatment to allow the estimation of the incremental costs and benefits for high-risk patients receiving TD compared with those for high-risk patients receiving SU.
The limitation of this assumption is that it does not allow an estimation of the impact of misdiagnosis on TTS. However, presently there are no data to allow the estimation of the cost-effectiveness of misdiagnosing patients (as discussed throughout Chapter 5).
The SLRs of economic evaluations in CD did not produce any data to inform state-specific transition probabilities to or from surgery. Therefore, the EAG had to estimate TTS as a standalone outcome in the model. This modelling simplification means that patients do not explicitly leave their health state in a specific cycle to move to the surgery state. Instead, in every model cycle, a proportion of surgeries is estimated and the associated costs and impact on patients’ quality of life are calculated. To avoid double-counting issues, the EAG adjusted treatment costs, based on the assumption that patients receiving surgery stop their current treatment in the model, and applied a surgery-related disutility to patients’ total utility in that model cycle. In clinical practice, it is expected that patients might need to change treatment (or to receive no treatment for a period of time) after surgical events, and, furthermore, that surgery is dependent on patients’ level of response to current treatment. However, the EAG could not find data to reflect all of the possible time-dependent transitions from the different health states in the model.
As this was a scenario analysis, the EAG allowed a proportion of patients to receive surgery as a final treatment step in the economic model. The results of this analysis are reported in Chapter 5.
To extrapolate TTS data into the model time horizon, the EAG fitted a variety of parametric curves to the Kaplan–Meier data. The pooled TTS data were fitted using the process described in Time to treatment escalation in high- and low-risk patients. Clinical experts were shown the fitted curves and they informed the EAG that 50% of CD patients would be expected to receive surgery during the first 10 years after their initial diagnosis, while 25% of patients would receive surgery in the subsequent 5-year period. The EAG decided to use the exponential model in the base-case analysis. AIC and BIC statistics are reported in Appendix 2 (see Table 32).
Effectiveness of top-down compared with step-up treatment strategy on surgery
To estimate TTS in the high-risk TD strategy arm of the model, the EAG applied a hazard function taken from Hoekman et al. 120 The study concluded that TTS was not statistically significantly different across treatment arms. The authors discussed several potential explanations for the lack of statistical differences across study outcomes. These included the reasons already discussed in Effectiveness of top-down compared with step-up treatment strategy on time to treatment escalation regarding the D’Haens et al. 35 trial, in addition to the following:
-
The authors mention the relatively early introduction of IMs or infliximab in the treatment regime for patients receiving conventional management as a potential factor in underestimating the relative effectiveness of early immunosuppressant therapy (at the start of follow-up, 66% of SU patients had received IMs and 15% had received anti-TNF treatment, compared with 82% and 20% of TD patients, respectively).
-
The EAG does not necessarily agree with the point above, as the ‘early’ introduction of anti-TNF or IMs in the conventional treatment arm of the study could have been a reflection of the poor performance of conventional therapy and, thus, the need to escalate to anti-TNF treatment faster.
-
The authors also mention the study’s potential lack of statistical power. Conversely, the authors also argue that observed statistically significant differences between groups merely reflect type I errors due to multiple testing (multiple testing correction was not applied in the study).
-
Finally, the study reports that the treatment received by patients beyond year 2 (the end of the D’Haens et al. 35 trial and the beginning of the follow-up study by Hoekman et al. 120) was at the discretion of the treating physician. Consequently, patients’ outcomes might have be influenced by different treatment strategies at the participating sites. The authors added that patients in both arms of the trial were evenly distributed across the participating hospitals, and, thus, in theory, were equally exposed to the treating physicians’ preferences.
In conclusion, the EAG cannot be sure if the timing of immunosuppression therapy has an impact on TTS events, as the data demonstrate a non-statistically significant effect. However, given that there are also plausible reasons that could explain an underestimation of the effect (or a lack of statistical power to detect it), the EAG has applied the hazard function taken from Hoekman et al. 120 to the TTS in the high-risk TD arm of the model in its base-case analysis. As an exploratory analysis, the EAG has assumed that TTS is the same in the TD and the SU arms for high-risk patients. The results of this scenario analysis are reported in Chapter 6.
The EAG digitised the TTS Kaplan–Meier data in Hoekman et al. 120 The study did not provide numbers at risk (except for the total number of patients entering the study). Therefore, the EAG had to manually reconstruct the numbers at risk by visually analysing the Kaplan–Meier data and estimating when (and how many) events happened over time. This task was simplified by the fact that there were no censored events in the TTS data. Subsequently, the EAG used the number of patients at risk to simulate the pseudo-individual patient data using the Guyot et al. 122 method and the algorithm in the survHER package. The EAG obtained the Kaplan–Meier data (Figure 17) and fitted survival models (dependently, owing to the small number of events across the arms) using the process described in Time to treatment escalation in high- and low-risk patients. The EAG notes that TTS was not statistically significantly different between the TD and SU arms in the EAG analysis (p = 0.2).
According to the AIC and BIC statistics reported in Appendix 2 (see Table 33), the three best-fitting models are the exponential, log-normal and log-logistic. Figure 30 (see Appendix 7) shows the fitted curves for SU patients along with the time to relapse Kaplan–Meier data, while Figure 31 (see Appendix 7) shows the equivalent curves for TD patients. The EAG chose the exponential model, given that it was the best fitting (Figure 18) and for the reasons discussed in Time to surgery in high- and low-risk patients. The EAG used the fitted curves to estimate a hazard function, which was then applied to the TTS curve in the high-risk TD arm of the economic model (Figure 19).
FIGURE 18.
Time-to-surgery curves fitted with exponential curves.

FIGURE 19.
Time to surgery for high-risk patients with TD vs. SU effect.

Mortality
The EAG assumed that CD does not have a direct impact on patients’ mortality. Instead, background survival rates matched for sex and age were used to estimate patients’ survival in the economic model. 133 The EAG assumed that surgery events were associated with a risk of death; hence, after every surgery in the model, patients undergoing surgery have a higher probability of dying than patients who do not undergo surgery.
In the company’s model and in the Marchetti et al. 88 study, surgery-related mortality was derived from Silverstein et al. 134 (0.0015 increase in the probability of dying per month). The EAG acknowledges that Silverstein et al. 134 is an old study (1999), and so surgery procedures and surgery-related death rates might have improved since then; however, the EAG did not identify more recent sources to populate this parameter in the model. The study is a 24-year follow-up of a population-based ‘inception cohort’ of 174 patients with CD in Olmsted County, MN, USA, and provides data on the progress of patients from remission through mild and more severe disease states.
In summary, mortality in the model differed (albeit very slightly) for high-risk TD compared with high-risk SU patients only because of the difference in TTS outcomes for the two groups. Survival in the model is reported in Figure 32 (see Appendix 8) for both general population survival and general population adjusted with surgery-related mortality. The impact of the latter on the former is visually negligible, and hence the curves overlap.
Adverse events
The EAG decided not to include adverse events in the economic analysis. The rational for this decision was twofold: the Evidence Review Group in TA352 concluded that the exclusion of adverse events associated with treatment with biologics (vedolizumab, infliximab and adalimumab) in the model did not have a relevant impact on the final ICER; and the aim of the economic model is to assess the cost-effectiveness of different treatment sequences for high-risk patients, not to compare the cost-effectiveness of isolated treatments.
Furthermore, the EAG did not find any evidence on the impact of the long-term use of biologics on patients’ quality of life in the TD arm compared with the SU arm. However, if adverse events were included in the analysis, given that a higher proportion of patients receive biologic treatment in the TD arm, this would have a negative impact on the outcomes in the TD arm of the model.
Utility values
All utilities were adjusted to account for the age and sex of the modelled population, in accordance with Ara and Brazier. 135
Remission, mild, and moderate to severe health states
The EAG used the two most recent NICE TAs on CD to inform its choice of utility values for the different CDAI states in the model (TA456 and TA456). Although TA456 is more recent than TA352, the Evidence Review Group in TA456 reported that it was:
[. . .] unclear why the company did not make use of the utilities used in TA352 which were based on EQ-5D data from GEMINI studies; [. . .] The estimated utility values in the GEMINI studies were elicited directly from the EQ5D using pooled data from the GEMINI II and GEMINI III studies and were estimated by health state regardless of study visit or treatment received.
The Evidence Review Group concluded that the utility values derived from the GEMINI studies were:
. . . theoretically superior to the values estimated from the mapping algorithm because they are directly elicited. The utility values from GEMINI studies are, however, similar to those used in the company’s base-case and therefore it is not expected to impact on estimated QALYs greatly.
Therefore, the EAG used the utility values accepted in TA352 in the base-case analysis and ran a scenario analysis using the TA456 utility values. Both sets of values are reported in Table 12 and the results of the scenario analysis are reported in Chapter 6.
Surgery disutility
To capture the impact of surgery, the EAG used the disutility values reported by Marchetti et al. 88 The estimates were based on assumptions made by the authors and were also used in the company’s model. Marchetti et al. 88 assumed that patients undergoing surgery retained 0.5 of their utility estimate for 1 month. This resulted in a disutility estimate associated with surgery of 0.4.
Costs
The following costs are considered in the model:
-
diagnostic test costs
-
treatment costs
-
acute and chronic care costs of CD (including costs of surgery).
All costs considered in the model are valued in 2019 Great British pounds. Where unit costs have been obtained from the published literature before 2019, costs were uplifted using the Office for National Statistics’ Consumer Price Inflation Index for Medical Services (DKC3). 136
Diagnostic test costs
To estimate the cost of PredictSURE-IBD and IBDX, the EAG had to make some assumptions. The EAG took the mid-point cost in the range provided by the IBDX company (Glycominds) for the cost of the kit and then multiplied the cost by 6 (to reflect the six available kits) and divided by 45 (as the full set of tests need to be run twice and the EAG assumed that full plates are used). This resulted in the estimation of the cost of the test per patient. The EAG then increased the cost to account for laboratory tests and other miscellaneous costs (as suggested by Glycominds). The total cost of PredictSURE-IBD was estimated at £1250 and the cost of IBDX was estimated at £347 (using Her Majesty’s Revenue and Customs exchange rate from USD to GBP of 1.2483). The results of the cost-effectiveness analysis using the IBDX costs are reported in Chapter 6.
Treatment costs
The treatments included in the model are those described in the TD and SU strategies in Intervention and comparator. The different treatment costs are reported in Table 13. Treatment schedules and doses varied according to induction and maintenance stages (Table 14). As a modelling simplification, the EAG fixed the time on induction (and thus induction costs) by class of treatment in the model. For the base-case analysis, the EAG looked at all treatments integrated in the treatment class – for example, for anti-TNF, the EAG looked at duration of induction treatment for adalimumab and infliximab – and chose the maximum induction period (4 weeks with infliximab) to estimate the duration of induction with anti-TNF therapy in the model. As a scenario analysis, the EAG used the minimum induction period from the treatment class in the model (in the case of anti-TNF, this would be 2 weeks as per the adalimumab schedule). The results of the scenario analysis are reported in Chapter 6.
Treatment | Dose per unit (mg) | List price/unit (£) | Source |
---|---|---|---|
Ustekinumab | 130 | 2147.00 | BNF124 |
Vedolizumab | 300 | 2050.00 | BNF124 |
Infliximab | 100 | 377.66 | BNF124 |
Adalimumab | 40 | 308.13 | BNF124 (per syringe) based on HulioTM (Mylan N.V., Hatfield, UK) |
Azathioprine | 50 | 0.04 | BNF124 (per tablet, 56-tablet pack) |
6-Mercaptopurine | 50 | 1.97 | BNF124 (per tablet, 25-tablet pack) |
Methotrexate | 25 | 16.64 | BNF124 (pre-filled pen) |
Methotrexate | 15 | 14.92 | BNF124 (pre-filled pen) |
Prednisolone | 2.5 | 0.04 | BNF124 (per tablet, 28-tablet pack) |
i.v. administration (outpatient) | 1 |
First: 199.00 Follow-up: 212.00 |
NHS Reference Costs 2017–18 137 SB12Z Deliver Simple Parenteral Chemotherapy at First Attendance (outpatient) SB15Z Deliver Subsequent Elements of a Chemotherapy Cycle |
Treatment | Induction (mg per week, unless stated) | Maintenance (mg per week, unless stated) | Source |
---|---|---|---|
Ustekinumab | For body weight up to 56 kg: 260 mg, 90 mg after 8 weeks | 90 every 8 weeks | Clinical expert opinion |
For body weight 56–85 kg: 390 mg, 90 mg after 8 weeks | |||
For body weight ≥ 86 kg: 520 mg, 90 mg after 8 weeks | |||
Vedolizumab | Initially 300 mg, then 300 mg after 2 weeks, followed by 300 mg after 4 weeks | 300 every 8 weeks | Clinical expert opinion |
Infliximab | Initially 5 mg/kg, then 5 mg/kg after 2 weeks, then 5 mg/kg after 4 weeks | 5 mg/kg every 8 weeks | Clinical expert opinion |
Adalimumab | Initially 160 mg, then 80 mg after 2 weeks | 40 every 2 weeks | Clinical expert opinion |
Azathioprine | 2.5 mg/kg | 2.5 mg/kg | Clinical expert opinion |
6-Mercaptopurine | 1.25 mg/kg | 1.25 mg/kg | Clinical expert opinion |
Methotrexate | 25 | 15 | Clinical expert opinion |
Prednisolone | 40 mg and then taper by 5 mg per week for 8 weeks | No maintenance with prednisolone | Clinical expert opinion |
The clinical experts advising the EAG consistently reported that treatment with anti-TNF and second-line biologics would be given as long as patients continued to show a response. Therefore, the base case analysis assumed that patients receive treatment with first- and second-line biologics until escalation to next treatment steps occurs. The EAG included two scenario analyses in the model to explore the uncertainty around this assumption and reports the results in Chapter 5:
-
assuming that a proportion of patients in remission are cured and therefore stop treatment permanently
-
capping the duration of treatment with biologics in the model.
Acute and chronic care costs of Crohn’s disease
The EAG took the resource use reported in TA352 as a basis for discussion with clinical experts. After receiving input from clinical experts, the EAG combined the estimates on resource use by taking the mid-point between estimates when clinical opinion was different, or the estimate provided by the experts when there were no discrepancies. The estimates used in the economic analysis are reported in Table 15. The unit costs were sourced from NHS National Schedule of Reference Costs 2017 to 2018. 137
Resource use/year (source: clinical expert opinion) (number of visits or exams per year) | Unit costs (£) | Code | |||
---|---|---|---|---|---|
Remission | Mild | Moderate to severe | |||
Outpatient | |||||
IBD consultant | 0.5 | 0.75 | 2.0 |
First: 165 Follow-up: 132 |
NHS National Schedule of Reference Costs 2017 to 2018.137 Currency code WF01A/B, service code: 301, gastroenterology |
Dietitian | – | 0.38 | 2.35 | 81 | NHS National Schedule of Reference Costs 2017 to 2018.137 Community Health Services; Currency Code A03 Dietitian |
Other IBD nurse | 0.86 | 1.82 | 5.11 | 77 | NHS National Schedule of Reference Costs 2017 to 2018.137 Community Health Services Currency Code N29AF Other Specialist Nursing, Adult, Face to face |
Helpline | 0.59 | 1.52 | 6.09 | 33 | NHS National Schedule of Reference Costs 2017 to 2018.137 Community Health Services Currency Code N29AN Other Specialist Nursing, Adult, Non face to face |
Pharmacist | – | 0.17 | 0.63 | 8 |
Assuming 10 minutes of pharmacist time Pharmacist cost per hour taken from PSSRU138 |
Nutritional support | – | – | 0.5 | 71 | NHS National Schedule of Reference Costs 2017 to 2018.137 Outpatient attendances; Service Code 654 Dietetics (non-consultant led) |
Radiology | |||||
Plain X-ray | – | – | 0.94 | 30 | NHS National Schedule of Reference Costs 2017 to 2018.137 DAPF, Direct access plain film |
CT scan of abdomen/pelvis | – | – | 1.16 | 137 | NHS National Schedule of Reference Costs 2017 to 2018.137 Outpatient, RD28Z, Complex CT scan |
MRI scan of abdomen/pelvis | 0.25 | 0.30 | 0.63 | 301 | NHS National Schedule of Reference Costs 2017 to 2018.137 Outpatient, RD03Z, Magnetic Resonance Imaging Scan requiring extensive patient repositioning |
DEXA scan | 0.31 | 0.31 | 0.31 | 71 | NHS National Schedule of Reference Costs 2017 to 2018.137 Outpatient, RD50Z, Dexa scan |
MRI scan of small bowel | – | – | 0.5 | 205 | NHS National Schedule of Reference Costs 2017 to 2018.137 Outpatient, RD03Z, Magnetic Resonance Imaging Scan, one area, pre and post contrast |
Endoscopies | |||||
Oesophagogastroduodenoscopy | – | – | 0.4 | 299 | NHS National Schedule of Reference Costs 2017 to 2018.137 Day case, FZ60Z Diagnostic Endoscopic Upper Gastrointestinal Tract Procedures, 19 years and over |
Sigmoidoscopy | 0.25 | 0.35 | 0.78 | 319 | NHS National Schedule of Reference Costs 2017 to 2018.137 Day case, FZ55Z Diagnostic Flexible Sigmoidoscopy, 19 years and over |
Colonoscopy | 0.2 | 0.3 | 1.23 | 517 | NHS National Schedule of Reference Costs 2017 to 2018.137 Day case, FZ52Z Diagnostic Colonoscopy with Biopsy, 19 years and over |
Double-balloon enteroscopy | – | – | 0.08 | 265 | NHS National Schedule of Reference Costs 2017 to 2018.137 Endoscopies. Currency Code FZ13C Minor Therapeutic or Diagnostic, General Abdominal Procedures, 19 years and over |
Wireless capsule endoscopy | – | – | 0.15 | 734 | NHS National Schedule of Reference Costs 2017 to 2018.137 Endoscopies. Currency Code FZ42A Wireless Capsule Endoscopy, 19 years and over |
Hospitalisations | – | – | 0.6 | 2773 | NHS National Schedule of Reference Costs 2017 to 2018.137 Non-elective inpatients (average length of stay 7 days). Currency Code FZ37P Inflammatory Bowel Disease without Interventions, with CC Score 5+ |
The total health-care costs per 2-week cycle (excluding surgery) associated with the remission, mild, and moderate to severe states were £17, £27 and £122, respectively.
The EAG matched the type of surgical procedures observed in the Biasci et al. 50 IPD to the Healthcare Resource Group 2017/18 reference costs grouper. The EAG then used the Healthcare Resource Group code to cost the specific procedure in the NHS National Schedule of Reference Costs 2017 to 2018. 137 The resulting costs and average length of stay for the specific procedures underpinning the TTS data used in the model can be found in Table 16, along with the number of occurrences for each surgery observed in the Biasci et al. 50 IPD.
Procedure | HRG code | NHS National Schedule of Reference Costs 2017 to 2018137 description | Cost (£)137 | Average length of stay (days) | Number of occurrences in Biasci et al.50 IPD |
---|---|---|---|---|---|
Right hemicolectomy | FF32 | Proximal Colon Procedures, 19 years and over, with CC Score 6+ | 9225 | 10 | 3 |
Ileal resection | FF22 | Major Small Intestine Procedures, 19 years and over, with CC Score 7+ | 10,480 | 16 | 11 |
Defunctioning ileostomy | VA11 | Multiple Trauma with Diagnosis Score < = 23, with Intervention Score 1–8 | 1907 | 1 | – |
Perianal surgery (percutaneous drain) | FF41 | Intermediate Anal Procedures, 19 years and over, with CC Score 3+ | 2469 | 2 | – |
Surgery (enterocutaneous fistula) | FF02 | Major Therapeutic Endoscopic, Upper or Lower Gastrointestinal Tract Procedures, 19 years and over, with CC Score 3+ | 3635 | 4 | – |
Several perianal operations | FF33 | Distal Colon Procedures, 19 years and over, with CC Score 3+ | 7675 | 6 | – |
Weighted average | – | – | 8813 | 12.17 | – |
To estimate surgery costs in the model, the EAG applied a weighted average of the unit costs outlined in Table 16 using data on the number of occurrences of each type of surgery from the Biasci et al. 50 IPD. The weighted average cost was calculated by the EAG as £8813 and this was assumed to apply to the proportion of patients who receive surgery in each model cycle based on the estimated TTS survival curves.
The EAG used the length of stay estimates for each procedure from NHS costs137 to determine how long surgical patients might be expected to temporarily discontinue pharmacological treatment (with IM, anti-TNF or biologics) in the model. The weighted length of stay for surgery was estimated to be 12.17 days (see Table 16). As this estimate is within a single model cycle of 2 weeks, the EAG assumed that patients would discontinue treatment for one full cycle when they received surgery.
The EAG assumed that the risk of surgery was not dependent on the step in the treatment pathway. Therefore, the EAG estimated that the pharmacological treatment costs not incurred in each cycle for the proportion of patients who receive surgery were weighted equally across each of the treatment steps. This was estimated by multiplying the total per-cycle pharmacological treatment costs across all steps by the per-cycle proportion of patients receiving surgery, and then removing these costs from the total per-cycle costs.
Summary of base-case model inputs and assumptions
Table 43 (see Appendix 6) reports the key model inputs used in the EAG’s base-case model and how these were varied in the PSA. Table 17 summarises the key assumptions in the EAG’s economic analysis, together with the rationale for these.
Description | Assumption | Justification |
---|---|---|
Structural | ||
Relative treatment effect for TD vs. SU for TTE | The EAG applied the relative hazard function to TTE curves in the first step in the TD strategy (anti-TNF) vs. the first step in the SU strategy (IM). The TTE associated with the remaining treatment steps in both the TD and the SU arms was assumed to be the same as the TTE for anti-TNF in the TD arm |
The only evidence available for the relative treatment effectiveness of TD vs. SU35 on time to relapse (and therefore on treatment escalation) compares anti-TNF with corticosteroids. The EAG assumed this measure to be a proxy for the relative treatment effect of anti-TNF vs. IM. However, the EAG considered that applying a relative treatment effect for TD vs. SU across all treatment steps in the model was inappropriate The underlying assumption in the EAG’s base-case approach is that high-risk patients who initiate treatment with IMs (SU arm) escalate treatment quicker than high-risk patients who initiate treatment with anti-TNF; however, once SU patients initiate treatment with anti-TNF (their second treatment step), they ‘catch up’ with patients on the TD treatment strategy Furthermore, Hoekman et al. 120 concluded that in the long term (10-year follow-up) a TD strategy had not been proven to alter the natural history of CD Given the paucity of data to substantiate any further benefits in subsequent treatment steps in the TD vs. SU approaches, the EAG considered this to be the most conservative modelling approach |
Surgery modelling | Surgery was modelled with TTE data as a standalone health state, with no explicit transitions from/to any other states (except death) in the model |
The SLRs of economic evaluations in CD did not produce any data to inform state-specific transition probabilities to surgery In every model cycle, a proportion of surgeries is estimated, and the associated costs and impact on patients’ quality of life are calculated. To avoid double-counting issues, the EAG applied an adjustment to treatment costs, based on the assumption that patients receiving surgery stop their current treatment in the model, and applied a surgery-related disutility to patients’ total utility in that model cycle In clinical practice, it is expected that patients might need to change treatment (or receive no treatment for a period) after surgical events, and, furthermore, that surgery is dependent on patients’ level of response to current treatment. However, the EAG could not find the data to reflect all the possible time-dependent transitions from the different health states in the model |
Relative treatment effect for TD vs. SU for TTS | The EAG applied a relative hazard function to TTS curves | The Hoekman et al.120 data do not suggest that there is a statistically significant difference in TTS between TD and SU therapy. However, given that there are also plausible reasons that could explain an underestimation of the effect (or a lack of statistical power to detect it), the EAG has applied the hazard function taken from Hoekman et al. to the TTS in the high-risk TD arm of the model in their base-case analysis and, as an exploratory analysis, the EAG has assumed that TTS is the same in the TD and the SU arms for high-risk patients |
Transition between disease severity stages while on maintenance treatment | Patients experience different levels of response to maintenance therapy over time | As discussed in TA352,125 the DSU has reported the importance of capturing partial response to maintenance treatment (as well as remission, relapse, surgery and post-surgical remission) in CD modelling approaches.139 Therefore, the EAG based its model on the Bodger et al.93 structure to capture different levels of response |
Flares | The EAG assumed that treatment escalations in the model correspond to a relapse to patients’ current treatment (or a severe flare) | The EAG assumed that the Biasci et al.50 TTE data captured flares leading to treatment escalation |
TTE modelling | ||
TTE high-risk | Log-normal curve fitted to IPD KM from Biasci et al.50 (cohort of 40 patients as per Time to treatment escalation in high- and low-risk patients) |
The EAG aimed to use TTE data whenever available Furthermore, the EAG restricted its analysis set to the 40 patients in the Biasci et al. 50 IPD who had received treatments representative of the SU strategy in the NHS pathway The EAG used the time to first escalation only (IM to anti-TNF) from the Biasci et al. 50 IPD as data on further escalations were deemed too incomplete and not robust enough for analysis |
TTE low-risk | Gompertz curve fitted to IPD KM from Biasci et al.50 (cohort of 40 patients as per Time to treatment escalation in high- and low-risk patients) | |
TTE TD | Log-normal (dependent-fit) curve fitted to IPD KM from D’Haens et al.35 | |
TTE SU | ||
TTE high-risk TD | Log-normal curve fitted to IPD KM from Biasci et al.50 with hazard function from D’Haens et al.35 | |
TTE high-risk SU | Same as TTE high-risk | |
TTE low-risk SU | Same as TTE low-risk | |
TTS modelling | ||
TTS high-risk | Exponential (pooled high- and low-risk curves) fitted to IPD KM from Biasci et al.50 |
The EAG aimed to use TTE data whenever these were available Furthermore, the EAG in TA352 criticised the company in the same appraisal for modelling surgery as a constant probability in the economic analysis125 |
TTS low-risk | ||
TTS TD | Exponential (dependent fit) fitted to IPD KM from Hoekman et al.120 | |
TTS SU | ||
TTS high-risk TD | Exponential (pooled high- and low-risk curves) fitted to IPD KM from Biasci et al.50 with hazard function from Hoekman et al. | |
TTS high-risk SU | Same as TTS high-risk | |
TTS low-risk SU | Same as TTS low-risk | |
Surgery costs | ||
The EAG assumed that patients would discontinue treatment when they receive surgery | Patients stop treatment for one model cycle (14 days) |
The EAG’s approach aimed to avoid double-counting surgery and treatment costs. In clinical practice, it is expected that patients might need to change treatment (or to receive no treatment for a period of time) after surgical events and, furthermore, that surgery is dependent on patients’ level of response to their current treatment. However, the EAG could not find data to reflect all of the possible time-dependent transitions from the different health states in the model The weighted length of stay for surgery procedures observed in Biasci et al. 50 was estimated to be 12.17 days The EAG acknowledges that surgery might have a beneficial impact on patients’ quality of life, as there is a disease ‘reset’ for a period of time after surgery when patients might not need any treatment. Even though the EAG has not captured this potential benefit of surgery in the economic analysis, it notes that to do so would benefit the SU strategy, as a higher proportion of patients receive surgery in the SU arm than in the TD arm of the model |
Biologic costs | ||
Treatment duration | Treatment given until escalation to next treatment step | The clinical experts advising the EAG consistently reported that treatment with anti-TNF and second-line biologics would be given as long as patients continued to show a response. Therefore, the base-case analysis assumed that patients receive treatment with first- and second-line biologics until escalation to next treatment steps occurs. The EAG included two scenario analyses in the model to explore the uncertainty around this assumption and reports the results in Chapter 5:
|
Chapter 5 Cost-effectiveness results
Base-case deterministic and probabilistic results
In the EAG’s model, TTE is dependent on the time since starting the model and not on the time since starting a particular course of treatment. The implication of this assumption is that once patients escalate to their second (or further) treatment, the probability of treatment escalation does not reset to the same as it was when patients started their first treatment. In fact, the probability of treatment escalation decreases over time, according to the Kaplan–Meier data in Biasci et al. 50 and the log-normal curve used to fit the latter.
The EAG’s approach is based on the assumption that as patients escalate to more aggressive treatments their probability of escalating to the next treatment (or the escalation hazard) diminishes compared with the less aggressive initial treatment received, to which patients end up losing response and thus need to escalate. However, the EAG acknowledges that this assumption is based on a clinical assumption. An equally valid assumption is that TTE is dependent on the time from treatment initiation and ‘resets’ when a new treatment begins. Therefore, the EAG has implemented the latter assumption in its alternative base-case model and provides results for both assumptions below.
Table 18 presents the deterministic base-case ICER for PredictSURE-IBD compared with standard care when TTE is dependent on the time since starting the model. The results show that the TD strategy (via the use of PredictSURE-IBD in the model) is dominated by SU (via the standard care arm of the model), with an additional cost of £7636 and a QALY loss of 0.10.
Intervention | Total costs (£) | Total QALYs | Incremental costs (£) | Incremental QALYs | ICER |
---|---|---|---|---|---|
Standard of care | 207,857 | 15.96 | – | – | – |
PredictSURE-IBD | 215,493 | 15.85 | 7636 | –0.10 | Dominated |
Table 19 presents the deterministic base-case ICER for PredictSURE-IBD compared with standard care when TTE is reset at the beginning of every new treatment. The results show that the TD strategy (via the use of PredictSURE-IBD in the model) is still dominated by SU (via the standard care arm of the model), with an additional cost of £9084 and a QALY loss of 0.08.
Intervention | Total costs (£) | Total QALYs | Incremental costs (£) | Incremental QALYs | ICER |
---|---|---|---|---|---|
Standard of care | 201,925 | 15.86 | – | – | – |
PredictSURE-IBD | 211,009 | 15.79 | 9084 | –0.08 | Dominated |
The EAG conducted a PSA to assess the impact of the combined uncertainty from all parameters in the model. This was performed by sampling from distributions of the uncertain parameters 10,000 times to generate the equivalent number of sampled ICERs. The methods for the inclusion of parameter uncertainty are discussed for each parameter type in turn.
There are many sources of uncertainty in the economic model and the key parameters that can have a meaningful impact on the results include the induction vector values to inform the initial cohort distribution across the health states, the transition probability estimates, and the time to escalation survival curves.
The induction vectors and each row of the transition matrices were varied using Dirichlet distributions to ensure that the rows summed to one. These were sampled in R using the Dirichlet function of the MCMCpack140 package to generate 10,000 samples, which were copied into the economic model and sampled consecutively for each iteration of the PSA.
Each time-to-escalation curve applied in the model was sampled in a similar way by deriving 10,000 samples of each curve, using the vcov function of the Stats package in R to estimate covariance matrices for the parameters, which were then used along with the mean parameter estimates in the mvrnorm function of the MASS141 package to generate 10,000 correlated samples for each parameters, which were subsequently used to generate 10,000 survival curves.
For cost estimates, gamma distributions were applied using 20% of the mean value to estimate standard errors, and for probabilities and utilities beta distributions were applied, again with an assumption that the standard errors are 20% of the mean estimate. A summary of the full parameterisations of these estimates varied in the PSA is given in Appendix 6 (see Table 43), and the probabilistic ICERs are reported in Tables 20 and 21 for the assumptions of TTE not resetting and resetting with new treatments, respectively. The incremental costs and QALYs relative to standard care are shown in the cost-effectiveness plane in Figures 20 and 21, and the cost-effectiveness acceptability curves showing the probability that PredictSURE-IBD is cost-effective compared with standard care over a range of willingness-to-pay thresholds are given in Figures 33 and 34 (see Appendix 9).
Intervention | Total costs (£) | Total QALYs | Incremental costs (£) | Incremental QALYs | ICER |
---|---|---|---|---|---|
Standard of care | 228,609 | 15.72 | – | – | – |
PredictSURE-IBD | 238,920 | 15.66 | 10,312 | –0.06 | Dominated |
Intervention | Total costs (£) | Total QALYs | Incremental costs (£) | Incremental QALYs | ICER |
---|---|---|---|---|---|
Standard of care | 224,904 | 15.70 | – | – | – |
PredictSURE-IBD | 237,036 | 15.67 | 12,132 | –0.03 | Dominated |
FIGURE 20.
Cost-effectiveness plane (assuming that TTE does not reset with time). WTP, willingness to pay.

FIGURE 21.
Cost-effectiveness plane (assuming that TTE resets with time). WTP, willingness to pay.

Both probabilistic ICERs are dominated against PredictSURE-IBD and the cost-effectiveness acceptability curves show that the diagnostic test has a 0% probability of being cost-effective compared with standard care at the ICER threshold of £20,000–30,000 used by NICE. 142
The EAG varied the willingness-to-pay threshold to assess when the cost-effectiveness acceptability curves would begin to converge and, at a threshold of £500,000 per QALY gained, the probability of PredictSURE-IBD being cost-effective was 21% compared with 79% for the standard care arm for the ICER provided in Table 20, and just below 35% compared with approximately 65% for the standard care arm for the ICER provided in Table 21.
Scenario analyses
The EAG conducted scenario analyses to assess the potential impact of the uncertainty around some of the assumptions made in the model. The results are reported in Table 22:
-
The EAG ran the economic model using the IBDX cost (reported in Chapter 4 Costs). The EAG notes that the clinical input parameters in the base-case economic model for PredictSURE-IBD and in the scenario analysis for IBDX are the same.
-
The EAG used the utility values in TA456 in a scenario analysis.
-
The EAG applied the induction vectors and transition probabilities based on TA352 studies.
-
-
As an exploratory analysis, the EAG assumed that TTS is the same in the TD and the SU arms for high-risk patients.
-
The EAG removed the age and sex utility adjustments from the economic analysis.
-
-
As a scenario analysis, the EAG used the minimum induction period from the treatment class in the model to estimate induction costs.
-
The EAG assumed that 100% of high-risk patients who receive SU do not respond to treatment and therefore escalate to anti-TNF after induction with IMs.
Intervention | Total costs (£) | Total QALYs | Incremental costs (£) | Incremental QALYs | ICER (£) |
---|---|---|---|---|---|
Scenario 1: applying IBDX cost | |||||
Standard of care | 207,857 | 15.96 | – | – | – |
IBDX | 214,590 | 15.85 | 6733 | –0.10 | Dominated |
Scenario 2: applying utilities from TA456119 | |||||
Standard of care | 207,857 | 15.68 | – | – | – |
PredictSURE-IBD | 215,493 | 15.57 | 7636 | –0.11 | Dominated |
Scenario 3: applying induction vectors and transition probabilities based on TA352125 studies | |||||
Standard of care | 207,587 | 15.95 | – | – | – |
PredictSURE-IBD | 215,294 | 15.85 | 7707 | –0.10 | Dominated |
Scenario 4: applying equivalent TTS curves for TD and SU | |||||
Standard of care | 207,857 | 15.96 | – | – | – |
PredictSURE-IBD | 216,059 | 15.85 | 8202 | –0.11 | Dominated |
Scenario 5: removing Ara and Brazier135 utility adjustment | |||||
Standard of care | 207,857 | 16.03 | – | – | – |
PredictSURE-IBD | 215,493 | 15.92 | 7636 | –0.11 | Dominated |
Scenario 6: using the minimum induction period from the treatment class to estimate induction costs | |||||
Standard of care | 201,623 | 15.93 | – | – | – |
PredictSURE-IBD | 208,901 | 15.82 | 7278 | –0.11 | Dominated |
Scenario 7: 100% of high-risk patients who receive SU do not respond to IM treatment | |||||
Standard of care | 214,678 | 15.85 | – | – | – |
PredictSURE-IBD | 215,493 | 15.85 | 815 | –0.0001 | Dominated |
All of the scenario analyses undertaken produced dominated ICERs against PredictSURE-IBD compared with standard care.
Table 23 presents the fully incremental analysis of cost-effectiveness results and demonstrates that, out of the diagnostic tools under consideration, PredictSURE-IBD is dominated by IBDX and both tools are dominated by standard care. However, as discussed throughout the report, despite extensive systematic searches of the literature, no robust evidence was identified on the prognostic accuracy of the biomarker stratification tools and the EAG considers that it would be challenging to ascertain an accurate estimate of prognostic accuracy of the tools in stratifying course of CD. Therefore, the only difference in the analysis of cost-effectiveness for the two diagnostic tools is the cost of the tests.
Intervention | Total costs (£) | Total QALYs | Incremental costs (£) | Incremental QALYs | ICER (£) |
---|---|---|---|---|---|
Standard of care | 207,857 | 15.96 | – | – | – |
IBDX | 214,590 | 15.85 | 6733 | –0.10 | Dominated |
PredictSURE-IBD | 215,493 | 15.85 | 903 | 0 | Dominated |
The EAG also ran a scenario analysis to include price discounts on the cost of anti-TNF and second-line biologic treatments in the analysis. The discounts were applied to the treatment class and a range of discounts was considered: 25%, 50% and 75%. The results of the analysis are reported in Appendix 10 (see Table 44) and show that PredictSURE-IBD remains dominated by standard of care in all scenarios. Although increasing the discount on the drugs results in a decreased incremental cost overall, this is not enough to cause the PredictSURE-IBD group total costs to be lower than the standard of care total costs.
As discussed throughout the report, and in particular in Chapter 4, Effectiveness of top-down compared with step-up treatment strategy on time to treatment escalation, the EAG conducted a range of additional analyses to test extreme scenarios around increasing the relative treatment effectiveness of the TD approach while decreasing the relative costs associated with TD. These scenarios are described below, together with the corresponding results.
Accounting for the cost-effectiveness of misdiagnosed cases
The test accuracy in the base-case economic model for PredictSURE-IBD and in the scenario analysis included in the DAR for IBDX was the same and assumed to be 100%. This is unlikely to reflect the tests’ actual accuracy in clinical practice; however, no robust diagnostic data were found to inform this in the analysis.
There is, however, an ongoing study (PROFILE) that will provide data on the relative effectiveness of these treatment strategies in high-risk patients. The EAG considers that this study should also be able to inform the costs and health consequences of misdiagnosing patients as high or low risk.
In the absence of real data to inform the costs and consequences of misdiagnosing patients according to their risk of disease severity, the EAG has undertaken a theoretical scenario analysis. The EAG assumed that both diagnostic tools are 75% accurate and, therefore, 25% of CD cases are assumed to be misdiagnosed in the analysis.
The EAG assumed that a proportion of patients who are incorrectly diagnosed as having a low-risk course of CD (i.e. high-risk patients) and receive SU therapy do not respond to IMs and, thus, move to anti-TNF treatment after induction therapy. Conversely, patients who are incorrectly diagnosed as being at high-risk (i.e. low-risk patients) and initiate TD treatment are assumed to enter remission with anti-TNF treatment and do not have the need to escalate treatment any further.
The rationale for the EAG’s assumptions is that low-risk patients (misdiagnosed as high risk) do not need to escalate from anti-TNF to other treatment options (second-line biologics) in the model. Given that these are low-risk patients, the EAG assumed that, after 2 years of anti-TNF treatment, 100% of these patients would be in a treatment-free remission state. Similarly, a proportion of high-risk patients (identified as low-risk patients) do not respond to IMs and so move on to anti-TNF. The proportion of high-risk patients who do not respond to IMs was assumed to be the same as in the base-case model (62%). The EAG acknowledges that these assumptions are a simplification of clinical reality; however, no robust evidence was found to inform this scenario.
Varying the assumptions around the measure of relative treatment effectiveness for time to treatment escalation
As some high-risk patients who receive SU treatment respond to IM treatment, having the additional IM step in the SU strategy is advantageous to patients in the EAG’s base-case analysis, as patients in the SU still subsequently receive treatment with biologics, which are assumed to have the same benefit as biologics in the TD arm. Given the paucity of data to substantiate any further benefits in subsequent treatment steps in the TD approach, the EAG considered this to be the most conservative modelling approach.
Nonetheless, the EAG varied these assumptions in two scenario analyses. The scenarios are explained below and summarised in Appendix 10 (see Table 45):
-
High-risk patients on anti-TNF after IMs (second step in SU arm) do not do as well as high-risk patients on first-line anti-TNF (first step in TD arm) and, thus, the former group escalates treatment quicker than the latter. Given that the EAG did not find any data to support this reduction in relative treatment effect, a theoretical assumption was made and varied:
-
Anti-TNFs in the SU approach are assumed to be only half as effective as anti-TNFs in the TD approach.
-
Anti-TNFs in the SU approach are assumed to be as effective as anti-TNFs in the TD approach.
-
This scenario also assumes that the relative benefit in the anti-TNF step of the TD strategy compared with the anti-TNF step in the SU strategy carries through the next treatment steps. Therefore, patients on second-line biologic treatment in the TD strategy have a relative benefit to second-line biologic treatment in the SU arm (as do patients on third-line biologics). It is also assumed that second- and third-line biologic treatment is as effective as anti-TNF treatment in the TD and SU arms (see Appendix 10, Table 45).
-
High-risk patients on anti-TNF after IMs (second step in SU arm) do not do as well as high-risk patients on first-line anti-TNF (first step in TD arm) and, thus, the former group escalate treatment quicker than the latter group. However, once patients have moved on to second- and third-line biologics, SU patients ‘catch up’ with TD patients and there is no further benefit for TD compared with SU. This scenario also assumes, by default, that second- and third-line biologic treatments are less effective than anti-TNF treatment in the TD arm (see Appendix 10, Table 45).
Assumptions around treatment discontinuation in the model
-
The EAG assumed that, after 2 years in remission with any biologic treatment, a proportion of patients experience mucosal healing and, therefore, stop treatment permanently. The EAG used the Marchetti et al. 88 paper to inform this scenario. The study reports that, after 2 years in remission, 76% of patients in the TD strategy experience mucosal healing, whereas 40% of patients in the SU arm experience the same outcome (illustrated in scenario 5.3.2ai).
The EAG also varied the Marchetti et al. 88 assumptions and explored the possibility of the TD and SU therapies having the same impact on the 2-year probability of mucosal healing. Therefore, the EAG assumed that both arms would experience the same probability (76% in scenario 5.2.3aii and 40% in scenario 5.2.3aiii) of mucosal healing.
The EAG notes that Hoekman et al. 120 concluded in their 10-year follow-up study that:
mucosal healing 2 years after the start of treatment was associated with a reduced use of anti-TNF treatment [. . .]. Other outcomes, however, did not differ significantly between patients with and without mucosal healing 2 years after the start of treatment.
Hoekman et al. 120
Furthermore, Hoekman et al. 120 also reported that another study has shown that, 2–4 years after randomisation, mucosal healing at week 104 after randomisation, but not treatment allocation, was associated with stable, corticosteroid-free remission. 143
Therefore, although there is some evidence to support the association of 2-year endoscopic mucosal healing with long-term, corticosteroid-free clinical remission, there does not seem to be any evidence that mucosal healing at 2 years differs according to treatment (TD or SU). Of note is that estimates used in Marchetti et al. 88 were taken from another study,143 which the EAG did not have access to.
The company in TA352 assumed that patients discontinued treatment with biologic agents approximately 1 year after maintenance treatment. The Evidence Review Group in TA352 was concerned that a discontinuation rule may not have been appropriate for patients who are not in remission as the NICE recommendation for infliximab and adalimumab suggests that, ‘specialists should discuss the risks and benefits of continued treatment with patients and consider a trial withdrawal from treatment for all patients who are in stable clinical remission. People who continue treatment with infliximab or adalimumab should have their disease reassessed at least every 12 months to determine whether ongoing treatment is still clinically appropriate. People whose disease relapses after treatment is stopped should have the option to start treatment again’ (© NICE 2015 Vedolizumab for treating moderately to severely active Crohn’s disease after prior treatment. Available from www.nice.org.uk/guidance/ta352 All rights reserved. Subject to Notice of rights. NICE guidance is prepared for the National Health Service in England. All NICE guidance is subject to regular review and may be updated or withdrawn. NICE accepts no responsibility for the use of its content in this product/publication.). The EAG notes that duration of treatment with biologics in clinical practice remains uncertain. The clinical experts advising the EAG reported that treatment with anti-TNF and second-line biologics would be given as long as patients continue to show a response.
For completeness, the EAG ran an additional scenario analysis assuming that 100% of patients in continuous remission for 12 months with maintenance treatment of any biologic (i.e. anti-TNF, second-or third-line biologics) discontinue treatment.
Surgery as a final treatment step in the economic model
The clinical experts advising the EAG explained that once patients exhaust all the biologic treatments available, they receive surgery. Therefore, the EAG ran a scenario analysis in which patients escalating from third-line biologic treatment in the model receive surgery. The EAG assumed that surgery had a temporary ‘curative’ effect of 2 years, where patients experience the costs and utility associated with being in the remission state. After 2 years, it was assumed that patients revert to the moderate to severe state, where they remain for the rest of the model.
To test the sensitivity of the results of the model to assumptions relating to surgery, the EAG ran a scenario analysis excluding surgeries from the model.
Accounting for the cost-effectiveness of misdiagnosed cases and assumptions around treatment discontinuation in the model
The EAG combined a range of scenarios to assess the impact of increasing the effectiveness of the TD strategy while decreasing costs with biologic treatments. Scenarios 5.2.5a, 5.2.5b and 5.2.5c explored changing the effectiveness of the diagnostic tool (and TD) through the assumptions made for the misdiagnosis scenario:
-
The EAG combined the misdiagnosis scenario 5.2.1 with scenario 5.2.3ai, where it was assumed that after 2 years in remission, 76% of patients in the TD strategy experience mucosal healing, while 40% of patients in the SU arm experience the same outcome.
-
The EAG also combined scenario 5.2.1 with scenario 5.2.3aii, where it was assumed that after 2 years in remission, 76% of patients in both the TD and the SU strategies experience mucosal healing.
-
The EAG also combined scenario 5.2.1 with scenario 5.2.3aiii, where it was assumed that after 2 years in remission, 40% of patients in both the TD and the SU strategies experience mucosal healing.
Varying the assumptions around the measure of relative treatment effectiveness for time to treatment escalation and assumptions around treatment discontinuation in the model
As with scenario 5.2.5, the EAG explored the impact of combining scenario 5.2.3 (where costs associated with biologics were decreased) with changing the effectiveness of the diagnostic tool (and TD) through the assumptions made for time to treatment discontinuation in the model. The EAG used scenario 5.2.2aii for all the analyses as this is the scenario that assumes the highest relative effective for TD versus SU in terms of TTE:
-
The EAG combined scenario 5.2.2aii with scenario 5.2.3ai, where it was assumed that, after 2 years in remission, 76% of patients in the TD strategy experience mucosal healing, while 40% of patients in the SU arm experience the same outcome.
-
The EAG also combined scenario 5.2.2aii with scenario 5.2.3aii, where it was assumed that, after 2 years in remission, 76% of patients in the TD and the SU strategies experience mucosal healing.
-
The EAG also combined scenario 5.2.2aii with scenario 5.2.3aiii, where it was assumed that, after 2 years in remission, 40% of patients in the TD and the SU strategies experience mucosal healing.
Varying the proportion of patients who respond to immunomodulators and varying the assumptions around the measure of relative treatment effectiveness for time to treatment escalation
One of the scenario analyses carried out by the EAG assumed that no high-risk patients respond to IMs (and therefore these patients do not derive any benefit from response to this treatment). This scenario was intended to portray an extreme clinical reality in which high-risk patients need treatment with a biologic to achieve a response and the impact of this assumption on the final ICER. The ICER for PredictSURE-IBD compared with SU changed from the EAG’s base case of dominated (against the diagnostic tool) to £71,294. Of note is that the EAG tested the impact of varying the proportion of patients who do not respond to IM treatment in the analysis, and when 92% of patients were assumed not to respond to IM treatment the two strategies (TD and SU) became clinically equivalent.
The EAG combined scenario 5.2.2aii with varying the proportion of high-risk patients who receive SU therapy and do not respond to IMs, thereby increasing the relative effectiveness of TD and decreasing the effectiveness of SU in terms of both TTE and the probability of response and remission in the model.
The EAG tested the assumption that 100% of patients do not respond to IMs and varied this percentage to assess the impact on the final ICERs.
Varying the proportion of patients who respond to immunomodulators; varying the assumptions around the measure of relative treatment effectiveness for time to treatment escalation; and varying treatment discontinuation assumptions
-
The EAG combined scenario 5.2.6a with varying the proportion of high-risk patients who receive SU therapy and do not respond to IMs (therefore not deriving any benefit from response to this treatment).
-
The EAG combined scenario 5.2.6b with varying the proportion of high-risk patients who receive SU therapy and do not respond to IMs (therefore not deriving any benefit from response to this treatment).
-
The EAG combined scenario 5.2.6c with varying the proportion of high-risk patients who receive SU therapy and do not respond to IMs (therefore not deriving any benefit from response to this treatment).
All of the above scenarios increased the relative effectiveness of TD in terms of TTE and decreased the costs associated with biologic treatment (to different amounts). For all scenarios, the EAG tested the assumption that 100% of patients do not respond to IMs and varied this percentage to assess the impact on the final ICERs.
Results
The results of the EAG’s scenario analyses are reported in Table 24. The majority of the scenarios still produced a dominated ICER, showing that the TD strategy (via the use of PredictSURE-IBD in the model) is dominated by SU (via the standard care arm of the model), with additional costs and a QALY loss.
Intervention | Total costs (£) | Total QALYs | Incremental costs (£) | Incremental QALYs | ICER (£) |
---|---|---|---|---|---|
Scenario 5.2.1: misdiagnosis | |||||
Standard of care | 207,857 | 15.96 | – | – | – |
PredictSURE-IBD | 215,516 | 16.07 | 7659 | 0.11 | 67,741 |
Scenario 5.2.2ai: assuming half of the base case relative effectiveness for TD on TTE for further steps | |||||
Standard of care | 204,720 | 15.90 | – | – | – |
PredictSURE-IBD | 213,724 | 15.82 | 9004 | –0.08 | Dominated |
Scenario 5.2.2aii: assuming the same as the base case relative effectiveness for TD on TTE for further steps | |||||
Standard of care | 200,403 | 15.82 | – | – | – |
PredictSURE-IBD | 210,640 | 15.77 | 10,237 | –0.05 | Dominated |
Scenario 5.2.2bi: assuming half of the base case relative effectiveness for TD on TTE for anti-TNF | |||||
Standard of care | 204,720 | 15.90 | – | – | – |
PredictSURE-IBD | 212,848 | 15.81 | 8128 | –0.09 | Dominated |
Scenario 5.2.2bii: assuming the same as the base case relative effectiveness for TD on TTE for anti-TNF | |||||
Standard of care | 200,403 | 15.82 | – | – | – |
PredictSURE-IBD | 208,949 | 15.74 | 8546 | –0.08 | Dominated |
Scenario 5.2.3ai: assuming discontinuation of biologic treatment for 76% TD, 40% SU | |||||
Standard of care | 186,932 | 15.96 | – | – | – |
PredictSURE-IBD | 182,311 | 15.85 | –4621 | –0.10 | 44,103a |
Scenario 5.2.3aii: assuming discontinuation of biologic treatment for 76% TD, 76% SU | |||||
Standard of care | 168,099 | 15.96 | – | – | – |
PredictSURE-IBD | 173,362 | 15.85 | 5263 | –0.10 | Dominated |
Scenario 5.2.3aiii: assuming discontinuation of biologic treatment for 40% TD, 40% SU | |||||
Standard of care | 186,932 | 15.96 | – | – | – |
PredictSURE-IBD | 193,319 | 15.85 | 6387 | –0.10 | Dominated |
Scenario 5.2.3b: assuming discontinuation of biologic treatment for 100% TD, 100% SU | |||||
Standard of care | 155,544 | 15.96 | – | – | – |
PredictSURE-IBD | 160,058 | 15.85 | 4514 | –0.10 | Dominated |
Scenario 5.2.4a: assuming surgery as last treatment step | |||||
Standard of care | 209,767 | 16.22 | – | – | – |
PredictSURE-IBD | 217,480 | 16.13 | 7713 | –0.09 | Dominated |
Scenario 5.2.4b: removing surgery from the model | |||||
Standard of care | 203,768 | 15.97 | – | – | – |
PredictSURE-IBD | 211,987 | 15.87 | 8219 | –0.11 | Dominated |
Scenario 5.2.5a (scenario 5.2.1 + scenario 5.2.3ai) | |||||
Standard of care | 186,932 | 15.96 | – | – | – |
PredictSURE-IBD | 180,063 | 16.07 | –6869 | 0.11 | Dominant |
Scenario 5.2.5b (scenario 5.2.1 + scenario 5.2.3aii) | |||||
Standard of care | 168,099 | 15.96 | – | – | – |
PredictSURE-IBD | 171,483 | 16.07 | 3384 | 0.11 | 29,932 |
Scenario 5.2.5c (scenario 5.2.1 + scenario 5.2.3aiii) | |||||
Standard of care | 186,932 | 15.96 | – | – | – |
PredictSURE-IBD | 192,341 | 16.07 | 5409 | 0.11 | 47,842 |
Scenario 5.2.6a (scenario 5.2.2aii + scenario 5.2.3ai) | |||||
Standard of care | 180,487 | 15.82 | – | – | – |
PredictSURE-IBD | 177,932 | 15.77 | –2555 | –0.05 | 50,936a |
Scenario 5.2.6b (scenario 5.2.2aii + scenario 5.2.3aii) | |||||
Standard of care | 162,563 | 15.82 | – | – | – |
PredictSURE-IBD | 169,411 | 15.77 | 6848 | –0.05 | Dominated |
Scenario 5.2.6c (scenario 5.2.2aii + scenario 5.2.3aiii) | |||||
Standard of care | 180,487 | 15.82 | – | – | – |
PredictSURE-IBD | 188,940 | 15.77 | 8453 | –0.05 | Dominated |
Scenario 5.2.7 (Scenario 5.2.2aii + assuming that 100% of SU patients do not respond to IMs) | |||||
Standard of care | 207,282 | 15.71 | – | – | – |
PredictSURE-IBD | 210,640 | 15.77 | 3357 | 0.06 | 60,056 |
Scenario 5.2.1 produced an ICER of £67,741 per QALY gained, with PredictSURE-IBD being more costly than standard care but generating a QALY gain of 0.11. Even though this scenario assumes lower test accuracy, the assumed consequences of misdiagnosis produced a QALY gain with the diagnostic tool. This is related to the assumption of allocating low-risk patients (misdiagnosed as high-risk) to the anti-TNF state in the model, without need for further escalation. Given that treatment with anti-TNF holds the highest remission rate in the EAG’s analysis, and that 62% of high-risk patients (misdiagnosed as low-risk) in the SU arm were assumed to not derive any benefit from treatment with IMs, the results produced positive incremental QALYs for the diagnostic tool (and, thus, for the TD strategy). The EAG also combined this scenario with reducing the costs associated with TD through reducing the time spent on biologic treatment (as per scenario 5.2.3) and presents the results in scenario 5.2.5.
Scenario 5.2.3ai produced an ICER of £44,103 for standard care compared with PredictSURE-IBD, meaning that the diagnostic tool is less expensive than standard care (by £4621) but also less effective (0.10 QALY loss). This scenario reduced the costs of biologic treatment in the TD arm by assuming that a higher proportion of patients in the TD arm achieve mucosal healing and, thus, stop treatment. Even though these patients were ‘kept’ in the remission state, the QALYs generated using this assumption were not enough to produce a QALY gain compared with the benefit patients derive from initial treatment with IMs in SU. The EAG also notes that scenario 5.2.3ai can also be interpreted as a proxy for a scenario assuming de-escalation from biologic treatment in the TD arm to IMs. This is because the scenario reduced treatment costs (by stopping treatment with biologics), which would be similar to replacing biologic treatment with IMs in the model as a result of the low cost of IM treatment.
The other variations of scenario 5.2.3, where the same proportions of patients were assumed to achieve mucosal healing in the TD and SU arms, produced dominated ICERs against the diagnostic tool (and, thus, TD). The EAG notes that Hoekman et al. 120 did not show a difference in mucosal healing for TD versus SU (although it is not clear if the authors investigated the impact that the strategies had on this outcome). Notwithstanding, the authors reported that the rate of mucosal healing reported in another study143 had shown that 2–4 years after randomisation, treatment allocation was associated with stable, treatment-free remission.
Scenario 5.2.5a resulted in a dominant ICER for PredictSURE-IBD (and TD), with the diagnostic tool associated with lower costs and higher QALYs than standard care (and SU). This scenario combines modelling misdiagnosed cases with reducing the costs associated with TD, and therefore generates additional QALYs for the diagnostic tool at a lower cost, given the assumption that a proportion of patients on TD enter a permanent stage of remission. Given that scenario 5.2.5a assumes a difference in the rate of treatment discontinuation for biologics (whereby TD patients have a higher probability of discontinuing treatment – owing to mucosal healing – than SU patients), this scenario produced the highest cost savings for TD. Scenarios 5.2.5b and 5.2.5c produced higher ICERs as the relative costs associated with treatment with biologics (and the diagnostic tool) increased; however, scenario 5.2.5b resulted in an ICER of £29,932 per QALY gained, close to the upper threshold (£30,000) typically used in the NICE decision-making process.
Scenarios 5.2.6a, 5.2.6b and 5.2.6c explored increasing the effectiveness of TD versus SU with respect to TTE, combined with decreasing the treatment costs with biologics. As demonstrated, all scenarios generate a QALY loss for the diagnostic tool compared with standard care. When it is assumed that a higher proportion of patients in the TD arm achieve mucosal healing (scenario 5.2.3ai) than in the SU arm, the diagnostic tool (and TD) becomes cost saving (–£4621), but less effective (–0.10).
Scenarios 5.2.7 and 5.2.8 explored increasing the effectiveness of TD versus SU with respect to TTE, combined with decreasing the treatment costs with biologics and with varying the assumption around the rate of response to IM treatment in the SU strategy.
Scenario 5.2.7 shows that when the relative TTE benefit in the anti-TNF step of the TD strategy compared with the IM step in the SU strategy carries through all the next treatment steps in the model (scenario 5.2.2aii) and when 100% of SU patients are assumed not to respond to treatment with IMs, the ICER amounts to £60,056 per QALY gained. Therefore, even when 100% of high-risk patients do not respond to IMs, the ICER for the diagnostic tool (and TD) compared with standard care (and SU) is still above the NICE £30,000 threshold.
Scenario 5.2.8a shows that when the relative TTE benefit in the anti-TNF step of the TD strategy compared with the IM step in the SU strategy carries through all the next treatment steps in the model (scenario 5.2.2aii), when a higher proportion of patients in the TD arm achieves mucosal healing (scenario 5.2.3ai), and when 100% of SU patients are assumed to not respond to treatment with IMs, the final ICER becomes dominant for PredictSURE-IBD (and TD), with the diagnostic tool being associated with lower costs and more QALYs than standard care (and SU). The diagnostic tool remains dominant until the assumption around the proportion of high-risk SU patients not responding to IM treatment is decreased from 100% to 79%. Of note is that the EAG’s base-case analysis estimates that 62% of high-risk patients do not respond to initial treatment with IMs.
Scenarios 5.2.8b and 5.2.8c show that when the relative TTE benefit in the anti-TNF step of the TD strategy compared with the IM step in the SU strategy carries through all the next treatment steps in the model (scenario 5.2.2aii), when the same proportion of patients in the TD and SU arms achieves mucosal healing (scenario 5.2.3aii for 76% and 40%, respectively), and when 100% of SU patients are assumed not to respond to treatment with IMs, the final ICERs are £28,192 and £43,286, respectively. Both scenarios generate a QALY gain for the diagnostic tool (and TD) compared with standard care (and SU); however, the additional costs associated with TD are higher in scenario 5.2.8c (40% of patients in remission stop treatment with biologics in both the TD and SU arms) than in scenario 5.2.8b (76% of patients in remission stop treatment with biologics in both the TD and the SU arms).
The EAG has produced plots to demonstrate the impact of reducing the percentage of high-risk patients who do not respond to IMs from 100% to 0%. The plot in Figure 22 shows the changes in the incremental costs and QALYs on the cost-effectiveness plane and demonstrates the ICER changing from dominant at 100% non-response to IMs, moving into the south-west quadrant (less costly and less effective for TD) at 79%, then becoming dominated from below 43%. Figure 23 shows the resulting final ICERs, and the drastic variation in these at 79% non-response, when the incremental QALYs become negative.
FIGURE 22.
Incremental costs and QALYs as percentage of high-risk IM non-responders varies.

FIGURE 23.
Resulting ICERs as the percentage of high-risk IM non-responders varies.
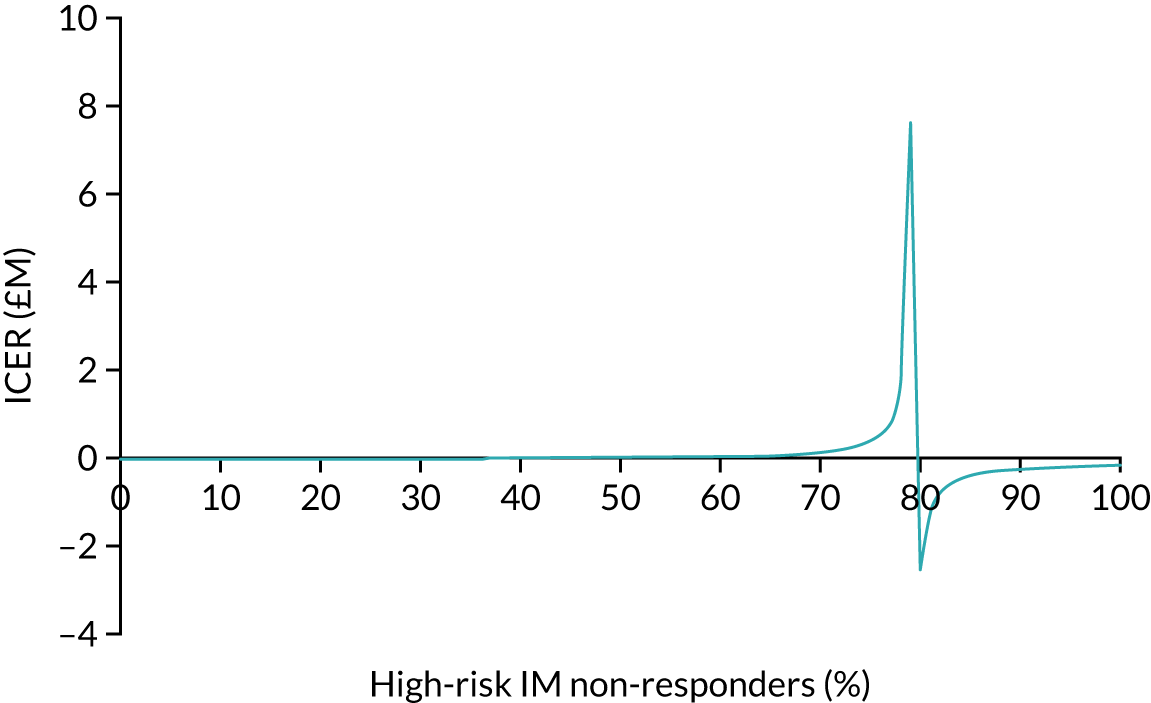
Conclusions
-
Estimating the impact of reducing test accuracy was only possible by combining this with an increase in the relative effectiveness of the TD strategy (to attribute consequences to misdiagnosing patients). However, changing this alone in the model still produced ICERs above the NICE upper cost-effectiveness threshold of £30,000 (scenario 5.2.1). When this assumption was combined with decreasing the costs associated with biologic treatment (by assuming different rates of mucosal healing leading to remission), the ICER ranged from dominant to £47,842 for PredictSURE-IBD (and TD) (scenarios 5.2.5a and 5.2.5c, respectively).
-
By itself, increasing the relative effectiveness of TD on TTE did not have an impact on the dominance of standard care over TD (scenario 5.2.2).
-
Assuming that 40% and 76% of patients in remission after 2 years (and 100% of patients in remission after 1 year) on maintenance treatment with anti-TNF and second- and third-line biologics discontinued treatment in both treatment arms also did not have an impact on the dominance of standard care over TD. Nonetheless, when a higher proportion of patients discontinued treatment with biologics in the TD arm than in the standard care arm, this generated a cost saving for TD, although still with fewer QALYs than for SU (scenario 5.2.3).
-
Excluding surgeries from the model did not have an impact on the dominance of standard care over TD, and neither did assuming that surgery has a curative effect for 2 years (scenario 5.2.4).
-
Combining the increase in the relative effectiveness of TD on TTE with the reduction in the costs of biologic treatment did not have an impact on the dominance of standard care over TD when the same proportion of patients were assumed to discontinue treatment with biologics in the TD and SU arms. When a higher proportion of patients discontinued treatment with biologics in the TD arm than in the standard care arm, this generated a cost saving for TD, but with fewer QALYs than for SU (scenario 5.2.6).
-
Increasing the relative effectiveness of TD on TTE and additionally reducing the effectiveness of SU (through assuming a 0% probability of response to IM treatment for high-risk patients) still generated an ICER above the NICE cost-effectiveness upper threshold of £30,000 (scenario 5.2.7).
-
When the increase in the relative effectiveness of TD on TTE and the additional reduction in the effectiveness of SU are combined with a reduction of time on treatment with biologics, the ICERs for PredictSURE-IBD (and TD) drop below the £30,000 per QALY gained threshold with standard care (and SU), depending on the assumptions made for the proportion of patients who discontinue treatment with biologics. When the proportion of patients discontinuing treatment with biologics is 76% in the TD arm compared with 40% in the SU arm, the final ICER is dominant for PredictSURE-IBD against standard care, as long as the proportion of high-risk patients who do not respond to initial treatment with IMs is 79% (or above).
In conclusion, once the relative effectiveness of TD is artificially increased (through TTE, the probability of response to initial treatment, and the impact that it has on low-risk patients) and is combined with decreased time on biologic treatment, the ICERs for PredictSURE-IBD (and TD) compared with standard care (and SU) fall below £30,000, which is the upper threshold typically used in the decision-making process by NICE. However, the EAG notes that these results need to be interpreted with extreme caution as the assumptions made in these scenarios were designed to test extreme clinical scenarios where TD was assumed to be more effective than SU. Nonetheless, the EAG did not find any evidence to substantiate the benefits modelled in these scenarios, and thus concludes that its base-case analysis showing that TD is dominated by SU remains the most conservative assessment of the relative cost-effectiveness of these treatment strategies.
Sensitivity analyses
One-way sensitivity analysis
The EAG conducted a number of deterministic one-way sensitivity analyses around the model inputs, as described in Table 25. Figure 24 ranks the key drivers of the model by their impact on the incremental net monetary benefit of PredictSURE-IBD compared with standard care, based on a willingness-to-pay threshold of £30,000 per QALY. The lower and upper bounds of each parameter input were derived from the lower and upper bounds of the 95% CIs of the distributions specified for the PSA. The inputs with the highest impact on the model results were the response to biologic treatments in both the TD and the SU arms. Details of each of the distributions are given in Appendix 6 (see Table 43).
Model parameter | Lower bound | Upper bound | Lower ICER (£) | Upper ICER (£) |
---|---|---|---|---|
Age | 21.3 | 48.7 | –68,002 | –86,923 |
CD expected body weight | 43.8 | 100.2 | –74,787 | –70,567 |
Proportion of males | 0.2280 | 0.5220 | –72,402 | –73,386 |
Probability of being high risk | 0.3496 | 0.8004 | –80,104 | –69,903 |
Proportion on infliximab in anti-TNF biologics class | 0.2432 | 0.5568 | –72,861 | –72,902 |
Proportion on vedolizumab in non-anti-TNF biologics class | 0.3040 | 0.6960 | –70,349 | –75,413 |
Proportion on azathioprine for IMs | 0.4864 | 1.0000 | –73,370 | –72,641 |
Proportion of 6-mercaptopurine for IMs | 0.0608 | 0.1392 | –72,913 | –72,861 |
Proportion of anti-TNF with IM bundle | 0.1824 | 0.4176 | –72,921 | –72,836 |
Proportion of biologics with IM bundle | 0.1216 | 0.2784 | –72,792 | –72,984 |
Response TD biologic | 0.1918 | 0.4390 | 4874 | 277,662 |
Remission TD biologic | 0.0795 | 0.1821 | –9314 | 1,026,662 |
Response TD anti-TNF | 0.1565 | 0.3583 | –59,548 | –110,878 |
Remission TD anti-TNF | 0.2231 | 0.5108 | –55,244 | –148,135 |
Response SU biologic | 0.1918 | 0.4390 | 484,370 | 3588 |
Remission SU biologic | 0.0795 | 0.1821 | –877,995 | –7071 |
Response SU anti-TNF | 0.1565 | 0.3583 | –123,227 | –40,429 |
Remission SU anti-TNF | 0.2231 | 0.5108 | –160,422 | –32,784 |
Response SU IM | 0.1380 | 0.3160 | –75,825 | –69,471 |
Remission SU IM | 0.0950 | 0.2176 | –77,255 | –68,744 |
Probability of death following surgery | 0.0009 | 0.0021 | –72,260 | –73,646 |
Health state cost: remission | £10 | £23 | –73,296 | –72,376 |
Health state cost: mild | £16 | £37 | –73,425 | –72,221 |
Health state cost: moderate/severe | £74 | £170 | –66,698 | –80,388 |
Health state cost: no response | £74 | £170 | –73,516 | –72,110 |
Induction cost per cycle: anti-TNF | £927 | £2123 | –72,368 | –73,503 |
Induction cost per cycle: biologic | £940 | £2151 | –71,130 | –75,007 |
Induction cost per cycle: IM | £3 | £6 | –72,923 | –72,829 |
Maintenance cost per cycle: anti-TNF | £326 | £747 | –78,669 | –65,853 |
Maintenance cost per cycle: biologic | £399 | £914 | –49,436 | –101,345 |
Maintenance cost per cycle: IM | £7 | £17 | –73,717 | –71,866 |
i.v. administration: first attendance | £121 | £277 | –72,683 | –73,122 |
i.v. administration: follow-up | £129 | £295 | –67,441 | –79,486 |
Cost of surgery | £5359 | £12,268 | –75,004 | –70,303 |
Utility: remission | 0.50 | 1.00 | 680,595 | –65,775 |
Utility: mild | 0.44 | 1.00 | –256,508 | –54,680 |
Utility: moderate/severe | 0.35 | 0.79 | –34,293 | 1,975,750 |
Disutility for surgery | 0.02 | 0.06 | –73,231 | –72,463 |
FIGURE 24.
Tornado plot showing one-way sensitivity analyses for PredictSURE-IBD vs. standard care. INMB, incremental net monetary benefit. Note that the bars in the graph represent the change in INMB and the corresponding ICERs are presented at both ends of the bars. Light blue bars represent the lower bound of the parameter changes and dark blue bars represent the upper bound of the parameter changes.
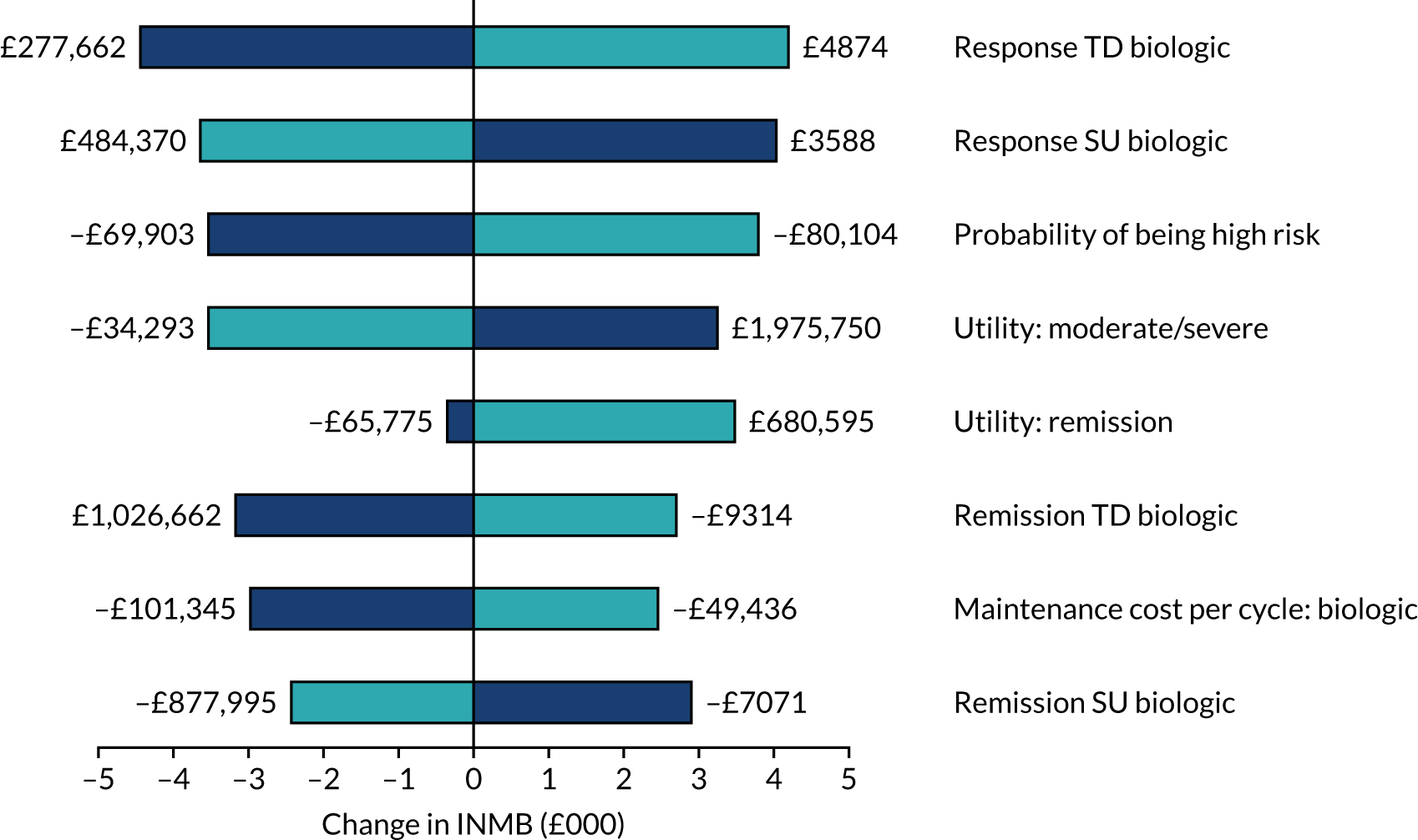
Chapter 6 Discussion
Statement of principal findings
Prognostic accuracy
Twelve publications50,67,69,71–79 describing eight studies were included in the assessment of the prognostic accuracy of the tests. Seven of the studies67,69,72,75,76,78,79 reported results on the utility of the IBDX kit and one study provided data on PredictSURE-IBD for stratifying those at high risk of a severe course of CD. Limited evidence is available from the included full-text publications on the prognostic accuracy of PredictSURE-IBD, and no evidence is available on prognostic accuracy of IBDX as determined by measures such as sensitivity and specificity. Most evidence on the utility of the two tools is derived from observational studies that report estimates of the risk of experiencing a clinical outcome associated with an aggressive course of CD, for example need for treatment escalation, development of a complication or surgery. No study retrieved reported on the clinical impact of the use of IBDX or PredictSURE-IBD in terms of influencing the treatments given in the management of active CD.
All included studies assessed outcomes in people reported to have a diagnosis of CD. However, limited reporting was noted across studies relating to IBDX on the stage of diagnosis (newly vs. established) at the time of the test. Baseline characteristics suggest that samples analysed were predominantly provided by people who had established CD. By contrast, most people enrolled in the study on PredictSURE-IBD had received a recent diagnosis of CD. Although most of the included studies outlined criteria to be met for a diagnosis of CD, only the study evaluating PredictSURE-IBD required people to have active disease to be eligible for enrolment, and reported how the presence of active disease was determined. Given the biomarker targets of the two prognostic tests, the reviewers consider that a criterion of active CD is appropriate for the inclusion of studies assessing PredictSURE-IBD but is not essential for studies reporting on IBDX.
The use of PredictSURE-IBD was associated with a sensitivity and specificity of 72.7% and 73.2%, respectively, in stratifying by need for multiple treatment escalations within 12 months. A negative predictive value of 90.9% for PredictSURE-IBD of predicting multiple escalations within the first 18 months was also reported. The cut-off point for multiple escalations applied in the determination of sensitivity and specificity was two treatment escalations and comprised any type of treatment, including surgery.
Seven studies67,69,72,75,76,78,79 evaluating the IBDX kit were deemed to be of relevance to the review, all of which were observational in nature: three studies75,76,79 were prospective cohorts and three69,72,78 had a cross-sectional design. Clinical heterogeneity across studies in terms of various characteristics (prior complication vs. no complication, previous IBD-related surgery or no surgery, and unclear whether or not people had active disease at baseline) was noted. Two prospective cohort studies75,76 reported an increased risk of experiencing a complication or of requiring surgery among those testing positive for at least two of the six biomarkers included in the IBDX kit.
Two studies reported an increased risk of experiencing a complication or of requiring surgery for those testing positive for at least two of the six biomarkers included in the IBDX kit. Risks of experiencing a complication by positive biomarker status were reported to be:
-
OR of 1.5 (95% CI 1.3 to 1.9; p < 0.001; number unclear) based on positivity for a median of two biomarkers
-
HR of 2.5 (95% CI 1.03 to 6.1; p = 0.043; n = 20 with no prior complication or surgery) based on positivity for at least two biomarkers
-
HR of 2.6 (95% CI 0.92 to 7.2; p = 0.072; n = 20 with no prior complication or surgery) based on positivity for at least three biomarkers.
Considering surgery, three studies reported on the increased risk of surgery. One study reported a trend towards a larger proportion of people with CD requiring abdominal surgery with increasing number of positive biomarkers (n = 517; p < 0.0001 across the groups). Other estimates of higher risk of requiring surgery were:
-
OR of 1.5 (95% CI 1.3 to 1.8; p < 0.001; number unclear) based on positivity for a median of two biomarkers
-
HR of 3.6 (95% CI 1.2 to 11.0; p = 0.023; n = 14 with no prior complication or surgery) based on positivity for at least two biomarkers
-
HR of 2.8 (95% CI 0.80 to 9.6; p = 0.11; n = 14 with no prior complication or surgery) based on positivity for at least three biomarkers.
The study evaluating PredictSURE-IBD reported that those categorised as at high risk of following a severe course of disease had a statistically significantly higher risk of first treatment escalation compared with those designated as at low risk, with a HR of 2.65 (95% CI 1.32 to 5.34; p = 0.006).
Economic
As no robust evidence was identified on the prognostic accuracy of the biomarker stratification tools, the development of the economic model sets a structural framework for analysing future available data on prognostic accuracy and assesses the costs and consequences of treating high- and low-risk patients with both the TD and the SU strategies.
The EAG found two main sources of evidence that could be used to model TTE and TTS. Nevertheless, each source could only partially inform the TTE and TTS analyses in the model. Therefore, clinical data informing the analysis had to be derived from multiple sources.
One of the key underlying assumptions in the EAG’s base-case analysis is that high-risk patients who initiate treatment with IMs (SU arm) escalate treatment quicker than high-risk patients who initiate treatment with anti-TNF (supported by the data presented in D’Haens et al. 35). However, once SU patients initiate treatment with an anti-TNF (their second treatment step), they ‘catch up’ with patients on the TD treatment strategy. As some high-risk patients who receive SU treatment respond to IM treatment, having the additional IM step in the SU strategy is advantageous to patients in the EAG’s base-case analysis as patients still subsequently receive treatment with biologics, which are assumed to have the same effect as biologics is the TD arm. Given the paucity of data to substantiate any further benefits in subsequent treatment steps in the TD approach compared with the SU approach, the EAG considered this to be the most conservative modelling approach.
The EAG also notes that although, in theory, a TD approach would suggest a ‘de-escalation’ of treatments, the clinical experts advising the EAG consistently reported that IMs would not be given to patients who respond well to biologics (instead, treatment with biologics would be continued until loss of response). The experts also explained that, after loss of response with first- or second-line biologics, patients would not be given IMs but instead would undergo surgery as a last treatment option. Nonetheless, the EAG undertook a scenario analysis (5.2.3ai) to explore the impact of de-escalation in the model.
The long-term follow-up study by Hoekman et al. 120 found no difference between SU and TD in 10-year clinical remission rate, endoscopic remission, hospitalisation, surgery or new fistulas. Furthermore, the study concluded that, in the long term, a TD strategy had not been proven to alter the natural history of CD. However, time to relapse was found to be statistically significantly different between the TD and SU arms in the 2-year analysis of the same data (by D’Haens et al. 35).
Hoekman et al. concluded that their study was the first to compare the long-term outcomes for newly diagnosed CD patients who received combined immunosuppression compared with those for patients who received conventional management. The authors added that early combined immunosuppression may be a preferential strategy, given the associated delay in time to relapse. However, the authors noted that the costs and risks of potentially overtreating patients with a potentially ‘benign’ disease course mean that a TD approach should not be recommended as a universal treatment strategy for all patients with newly diagnosed CD.
The EAG’s cost-effectiveness analyses are consistent with the conclusions from Hoekman et al. 120 The ICERs indicate that standard care (and so SU) dominates the use of both diagnostic tools (and so TD), even when assuming that the tests are 100% accurate. In the base-case results, the incremental analysis of cost-effectiveness demonstrates that the TD strategy (via the use of PredictSURE-IBD in the model) is dominated by SU (via the standard care arm of the model), regardless of whether it is assumed that TTE does or does not reset with every new treatment in the model.
To mitigate some of the concerns raised by the specialist committee members, the EAG conducted a range of analyses to test extreme scenarios around increasing the relative treatment effectiveness of the TD approach while decreasing the relative costs associated with TD. The EAG concluded the following:
-
Estimating the impact of reducing test accuracy was only possible through combining this with an increase in the relative effectiveness of the TD strategy (to attribute consequences to misdiagnosing patients). However, changing this alone in the model still produced ICERs above NICE’s upper cost-effectiveness threshold of £30,000. When this assumption was combined with decreasing the costs associated with biologic treatment (through assuming different rates of mucosal healing leading to remission), the ICER ranged from dominant to £47,842 for PredictSURE-IBD (and TD).
-
By itself, increasing the relative effectiveness of TD on TTE did not have an impact on the dominance of standard care over TD.
-
Assuming that 40% and 76% of patients in remission after 2 years (and 100% of patients in remission after 1 year) on maintenance treatment with anti-TNF, second- and third-line biologics discontinued treatment in both treatment arms also did not have an impact on the dominance of standard care over TD. Nonetheless, when a higher proportion of patients discontinued treatment with biologics in the TD arm compared with the standard care arm, this generated a cost saving for TD, albeit still with fewer QALYs than for SU.
-
Excluding surgeries from the model did not have an impact on the dominance of standard care over TD, and neither did assuming that surgery has a curative effect at 2 years.
-
Combining the increase in the relative effectiveness of TD on TTE with reducing the costs of biologic treatment did not have an impact on the dominance of standard care over TD when the same proportion of patients were assumed to discontinue treatment with biologics in the TD and the SU arms. When a higher proportion of patients discontinued treatment with biologics in the TD arm than in the standard care arm, this generated a cost saving for TD, albeit with fewer QALYs than for SU.
-
Increasing the relative effectiveness of TD on TTE and additionally reducing the effectiveness of SU (through assuming a 0% probability of response to IM treatment from high-risk patients) still generated an ICER above NICE’s upper cost-effectiveness threshold of £30,000.
When the increase in the relative effectiveness of TD on TTE and the additional reduction in the effectiveness of SU are combined with a reduction in time on treatment with biologics, the ICERs for PredictSURE-IBD (and TD) can become cost-effective compared with standard care (and SU), depending on the assumptions made for the proportion of patients who discontinue treatment with biologics. When the proportion of patients discontinuing treatment with biologics is 76% in the TD arm compared with 40% in the SU arm, the final ICER is dominant for PredictSURE-IBD against standard care, as long as the proportion of high-risk patients who do not respond to initial treatment with IMs is 79% (or above).
Strengths and limitations of the analysis
Clinical
Despite extensive systematic searches of the literature, no robust evidence was identified on the prognostic accuracy of the biomarker stratification tools IBDX and PredictSURE-IBD. In terms of sensitivity and specificity as estimates of prognostic accuracy, the EAG is unaware of a validated definition for determining whether or not a person has followed a severe course of CD, and, thus, considers the criterion for a true positive or false positive using IBDX and PredictSURE-IBD to be unclear. The EAG considers that it would be challenging to ascertain an accurate estimate of prognostic accuracy of the tools in stratifying the course of CD and to do so would require carrying out a prospective study that included a group that received only SU treatment after determination of the risk of a severe course of CD. The ongoing PROFILE RCT randomises people to accelerated SU or TD treatment after determining whether they are at high or low risk of following a severe course of CD, and so will provide additional data to inform estimates of prognostic accuracy. One study50 reporting on the sensitivity and specificity of PredictSURE-IBD was identified. The EAG has reservations about the generalisability of the estimates. To determine sensitivity and specificity, the authors of the study applied a cut-off point of two or more treatment escalations to denote a high risk of severe course of CD, with surgery included as treatment escalation. The EAG considers the choice of two escalations to be arbitrary. Additionally, the EAG’s clinical experts fed back that it would be appropriate to consider escalation to CD-related surgery separately from progression to drug treatment, and also to use the development of a complication of CD (fistula or stenosis) as an alternative marker of sensitivity and specificity.
Studies informing the evidence around the effectiveness of the tools predominantly estimated an increased risk of experiencing a clinical outcome for those designated as at high risk compared with those designated as at low risk of following a severe course of CD. Clinical outcomes that could be considered proxies for predicting prognosis of CD are developing a complication (fistula or stenosis), needing CD-related surgery, and a shorter time to and increased frequency of treatment escalations.
For IBDX, estimates were available for increased risk of developing a complication and for need for surgery for those classified as at high risk of following a severe disease course, but estimates were not available for TTE. Conversely, estimates were available for PredictSURE-IBD for TTE but not for risk of developing a complication or need for surgery. Given the disparity in the clinical outcomes assessed with IBDX and PredictSURE-IBD, the EAG considers that no conclusions can be drawn on the comparative effectiveness of the two tools in stratifying people by risk of a severe course of CD.
Another limitation of the identified evidence base is that no study included in the review prospectively followed people whose treatment was determined by results from IBDX and PredictSURE-IBD. The ongoing PROFILE RCT assesses whether or not early treatment with TD strategy affords clinical benefit to those categorised as being high risk of severe course of CD. However, given that people are first stratified as high or low risk using PredictSURE-IBD and, subsequently, are randomised to SU or TD treatment, the EAG considers that there is potential for the misdiagnosis of people who are truly low risk but are categorised as high risk to go undetected. However, an analysis of those randomised to accelerated SU after determination of being at high or low risk of following a severe course of CD will provide additional data to inform estimates of prognostic accuracy.
Economic
The EAG’s model offers methodological advantages when compared with the PredictSURE-IBD model. The main strength of the economic analysis is that it captures partial response to maintenance treatment (as well as remission, relapse, surgery and post-surgical remission). The analysis also uses time to event data (TTE and TTS) more extensively than previous models. Furthermore, the EAG has conducted a series of scenario analyses exploring structural and parameter uncertainty in the economic model. The EAG also conducted a series of scenarios testing extreme clinical assumptions around the potential benefit of TD compared with SU in order to mitigate the concerns raised by the specialist committee members.
However, clinical data informing the analysis had to be derived from multiple sources. This approach is not ideal and creates a patchwork network of evidence, introducing uncertainty into the economic results. Nonetheless, the EAG anticipates that this problem will be potentially overcome when the results of the PROFILE trial are available to populate the economic model.
The test accuracy in the base-case economic model for PredictSURE-IBD and in the scenario analysis for IBDX is the same and assumed to be 100%. The only difference in the cost-effectiveness analyses of the two diagnostic tests is the cost of the test. This is unlikely to reflect the tests’ actual accuracy in clinical practice; however, no robust diagnostic data were found in the analysis to inform this.
The potential benefits of TD treatment for high-risk patients are dependent on two questions that remain unanswered: (1) do some high-risk patients derive a benefit from receiving IM treatment before moving to biologic treatment? and (2) do SU high-risk patients have the same benefits as TD high-risk patients once they initiate the TD treatment pathway (i.e. treatment with anti-TNF)? In the EAG’s model, the potential disadvantage of waiting to initiate treatment with anti-TNF was based on only the increased risk of surgery in the SU arm; however, the negative impact of surgery in the analysis was not enough to offset the advantages of initial treatment with IMs for SU patients.
Finally, the EAG acknowledges that adverse events, specifically those relating to the long-term use of biologics, and the potential benefits associated with surgery were not included in the economic analysis. However, if adverse events were included in the analysis, given that a higher proportion of patients receive biologic treatment in the TD arm, this would have a negative impact on the outcomes in the TD arm of the model compared with the SU arm. Similarly, although the EAG has not captured the potential benefit of surgery in the economic analysis, it notes that to do so would benefit the SU strategy, as a higher proportion of patients receive surgery in the SU arm than in the TD arm. Therefore, including adverse events and the benefits of surgery in the analysis would not change the conclusions likely to be drawn from the current results.
Chapter 7 Conclusions
Clinical effectiveness
Despite extensive systematic searches of the literature, no robust evidence was identified on the prognostic accuracy of the biomarker stratification tools IBDX and PredictSURE-IBD. In terms of sensitivity and specificity as estimates of prognostic accuracy, the EAG is unaware of a validated definition for determining whether or not a person has followed a severe course of CD, and, thus, considers the criterion for a true positive or false positive using IBDX and PredictSURE-IBD to be unclear. The EAG considers that it would be challenging to ascertain an accurate estimate of prognostic accuracy of the tools in stratifying the course of CD as to do so would require carrying out a prospective study that included a group that received only SU treatment after the determination of risk of a severe course of CD. The ongoing PROFILE RCT randomised people to accelerated SU or TD treatment after determining whether they were at high or low risk of following a severe course of CD and so will provide additional data to inform estimates of prognostic accuracy.
Estimates of risk of experiencing a clinical outcome associated with a severe course of CD were not available for comparable outcomes for IBDX and PredictSURE-IBD. Given the disparity in the outcomes assessed for IBDX and PredictSURE-IBD, the EAG considers that no conclusions can be drawn on the comparative effectiveness of the two tools in stratifying people by risk of a severe course of CD.
Cost-effectiveness
Given the lack of robust evidence on the prognostic accuracy of the biomarker stratification tools, the development of the economic model to assess the cost-effectiveness of IBDX and PredictSURE-IBD mainly consisted of a theoretical exercise. The EAG anticipates that the economic model developed will provide a structural framework for analysing future available data on prognostic accuracy and assessing the costs and consequences of treating high- and low-risk patients with both TD and SU strategies.
The economic model ultimately assesses the cost-effectiveness of TD therapy compared with SU therapy for high-risk patients. However, the EAG did not identify any robust evidence on the latter; thus, the clinical data informing the economic analysis had to be derived from multiple sources. This approach is not ideal and creates a patchwork network of evidence, introducing uncertainty into the economic results.
One of the key underlying assumption in the EAG’s base-case analysis is that high-risk patients who initiate treatment with IMs (SU arm) escalate treatment quicker than high-risk patients who initiate treatment with anti-TNF (supported by the data presented in the study by D’Haens et al. 35). However, once SU patients initiate treatment with an anti-TNF (their second treatment step), they ‘catch up’ with patients on the TD treatment strategy. As some high-risk patients who receive SU treatment respond to IM treatment, having the additional IM step in the SU strategy is advantageous to patients in the EAG’s base-case analysis as patients still subsequently receive treatment with biologics, which are assumed to have the same effect as biologics is the TD arm. Given the paucity of data to substantiate any further benefits in subsequent treatment steps in the TD versus SU approaches, the EAG considered this to be the most conservative modelling approach.
The EAG also notes that although, in theory, a TD approach would suggest a ‘de-escalation’ of treatments, the clinical experts advising the EAG consistently reported that IMs would not be given to patients who respond well to biologics (instead, treatment with biologics would be continued until loss of response). The experts also explained that, after loss of response with first- or second-line biologics, patients would not be given IMs but instead would undergo surgery as a last treatment resource.
The long-term follow-up study by Hoekman et al. 120 found no difference in 10-year clinical remission rate, endoscopic remission, hospitalisation, surgery or new fistulas. Furthermore, the study concluded that, in the long term, a TD strategy had not been proven to alter the natural history of CD. However, time to relapse was found statistically significantly different across the TD and SU arms in the 2-year analysis of the data. 35
Hoekman et al. 120 concluded that their study was the first to compare the long-term outcomes for newly diagnosed CD patients who received combined immunosuppression compared with those for patients who received conventional management. The authors added that early combined immunosuppression may be a preferential strategy, given the associated delay in time to relapse. However, the authors noted that the costs and risks of potentially overtreating patients with a potentially ‘benign’ disease course mean that a TD approach should not be recommended as a universal treatment strategy for all patients with newly diagnosed CD.
The EAG’s analysis has shown that too much uncertainty remains around the potential benefit of TD treatment for high-risk patients. The cost-effectiveness of a TD strategy compared with a SU strategy in high-risk patients is highly dependent on two unanswered questions: (1) do some high-risk patients derive a benefit from receiving IM treatment before moving to biologic treatment? and (2) do SU high-risk patients have the same benefits as TD high-risk patients once they initiate the TD treatment pathway (i.e. treatment with anti-TNF)? In the EAG’s model, the potential disadvantage of waiting to initiate treatment with anti-TNF was based on only the increased risk for surgeries in the SU arm; however, the negative impact of surgeries in the analysis was not enough to offset the advantages on initial treatment with IMs for SU patients.
For the reasons discussed above, most of the EAG’s ICERs have shown that standard care (and SU) dominates both diagnostic tools (and TD). To mitigate some of the concerns raised by the specialist committee members, the EAG conducted a range of analyses to test extreme scenarios around increasing the relative treatment effectiveness of the TD approach while decreasing the relative costs associated with TD. The EAG concluded that once the relative effectiveness of TD is artificially increased (through both TTE and the probability of response to initial treatment) and combined with decreasing time on biologic treatment, the ICERs for PredictSURE-IBD (and TD) compared with standard care (and SU) are below £30,000. However, the EAG notes that these results need to be interpreted with extreme caution as the assumptions made in these scenarios were designed to test extreme clinical scenarios where TD was assumed to be more effective than SU. The EAG did not find any evidence to substantiate the benefits modelled in these scenarios and, thus, concludes that its base-case analysis showing that TD is dominated by SU remains the most conservative assessment of the relative cost-effectiveness of these treatment strategies.
Finally, the EAG acknowledges that adverse events and the potential benefits associated with surgery were not included in the economic analysis. However, if adverse events were included in the analysis, given that a higher proportion of patients receive biologic treatment in the TD arm, this would have a negative impact on the outcomes in the TD arm of the model. Similarly, although the EAG has not captured the potential benefit of surgery in the economic analysis, it notes that to do so would benefit the SU strategy, as a higher proportion of patients receive surgery in the SU arm than in the TD arm of the model. Therefore, including adverse events and the benefits of surgery in the analysis would contribute further for the dominance of standard care over PredictSURE-IBD.
Suggested research priorities
A high-quality clinical trial that directly compares IBDX and PredictSURE-IBD would facilitate the capture of robust data on the sensitivity and specificity of the tools. The EAG considers that it would be important to prespecify the trial parameters, for example the eligible population, the assessment of disease activity and severity at baseline, the criteria for treatment escalation and the treatment algorithm. In addition, clinical experts would probably need to be consulted to determine which outcome would be the most appropriate measure for prognostic accuracy, for example TTE, development of a complication or need for surgery. An economic evaluation based on the results of the PROFILE RCT would also be warranted.
Acknowledgements
The EAG would like to thank Dr Gordan Moran (Clinical Associate Professor of Gastroenterology, University of Nottingham, Nottingham), Dr John Saunders (Consultant Gastroenterologist, Royal United Hospitals Bath, Bath) and Dr Sami Hoque (Consultant Gastroenterologist, Barts NHS Health Trust, London) for providing clinical advice throughout the project, and Dr Christopher Stinton (Senior Research Fellow, Warwick Medical School, Coventry) for providing advice on the interpretation of prognostic accuracy. The EAG would also like to thank Mr Adil Butt (MSc Candidate, City, University of London, London) for his contribution to the running of searches to retrieve records on health-related quality of life, to the data extraction of economic and health-related quality-of-life studies and to the quality assessment of studies related to cost-effectiveness.
Contributions of authors
Steven J Edwards (https://orcid.org/0000-0002-9049-3421) (Director of Health Technology Assessment) was the project lead and supervised the production of the final report, report writing, critical appraisal of the clinical evidence and critical appraisal of the economic evidence.
Samantha Barton (https://orcid.org/0000-0001-7051-5112) (Principal Health Technology Assessment Analyst) devised and carried out the clinical literature searches and carried out study selection, data extraction, critical appraisal of the clinical evidence and report writing.
Mariana Bacelar (https://orcid.org/0000-0003-2278-0071) (Principal Health Economist) contributed to the study selection, the development of the conceptual economic model and report writing.
Charlotta Karner (https://orcid.org/0000-0003-3855-4853) (Health Technology Assessment Analysis Manager) contributed to the study selection, data extraction, critical appraisal of the clinical evidence and report writing.
Peter Cain (https://orcid.org/0000-0001-8580-9391) (Senior Health Economist) contributed to the development of the economic model and carried out the economic analysis and report writing.
Victoria Wakefield (https://orcid.org/0000-0002-2058-6411) (Principal Health Technology Assessment Analyst) contributed to the study selection, data extraction, critical appraisal of the clinical evidence and report writing.
Gemma Marceniuk (https://orcid.org/0000-0001-9365-0384) (Health Economist) devised and carried out the economic literature searches and carried out study selection, data extraction, critical appraisal of the economic evidence and report writing.
All authors read and commented on draft versions of the report.
Data-sharing statement
Further information and requests for access to the data used in this report can be obtained from the corresponding author.
Disclaimers
This report presents independent research funded by the National Institute for Health Research (NIHR). The views and opinions expressed by authors in this publication are those of the authors and do not necessarily reflect those of the NHS, the NIHR, NETSCC, the HTA programme or the Department of Health and Social Care. If there are verbatim quotations included in this publication the views and opinions expressed by the interviewees are those of the interviewees and do not necessarily reflect those of the authors, those of the NHS, the NIHR, NETSCC, the HTA programme or the Department of Health and Social Care.
References
- Abraham C, Cho JH. Inflammatory bowel disease. N Engl J Med 2009;361:2066-78. https://doi.org/10.1056/NEJMra0804647.
- Hendrickson BA, Gokhale R, Cho JH. Clinical aspects and pathophysiology of inflammatory bowel disease. Clin Microbiol Rev 2002;15:79-94. https://doi.org/10.1128/CMR.15.1.79-94.2002.
- Park JH, Peyrin-Biroulet L, Eisenhut M, Shin JI. IBD immunopathogenesis: a comprehensive review of inflammatory molecules. Autoimmun Rev 2017;16:416-26. https://doi.org/10.1016/j.autrev.2017.02.013.
- Crohn’s and Colitis UK . Crohn’s Disease 2019. www.crohnsandcolitis.org.uk/about-inflammatory-bowel-disease/crohns-disease (accessed 24 July 2019).
- Johns Hopkins Medicine . Crohn’s Disease: Introduction 2001. www.hopkinsmedicine.org/gastroenterology_hepatology/_pdfs/small_large_intestine/crohns_disease.pdf (accessed 5 August 2019).
- Cosnes J, Gower-Rousseau C, Seksik P, Cortot A. Epidemiology and natural history of inflammatory bowel diseases. Gastroenterology 2011;140:1785-94. https://doi.org/10.1053/j.gastro.2011.01.055.
- York Teaching Hospital . Crohn’s Disease 2019. www.yorkhospitals.nhs.uk/our-services/a-z-of-services/inflammatory-bowel-disease-at-york-hospital/crohns-disease/ (accessed 24 July 2019).
- Ananthakrishnan AN. Environmental triggers for inflammatory bowel disease. Curr Gastroenterol Rep 2013;15. https://doi.org/10.1007/s11894-012-0302-4.
- Feagins LA, Iqbal R, Spechler SJ. Case-control study of factors that trigger inflammatory bowel disease flares. World J Gastroenterol 2014;20:4329-34. https://doi.org/10.3748/wjg.v20.i15.4329.
- Wang L, Wang F-S, Gershwin ME. Human autoimmune diseases: a comprehensive update. J Intern Med 2015;278:369-95. https://doi.org/10.1111/joim.12395.
- Langholz E. Current trends in inflammatory bowel disease: the natural history. Therap Adv Gastroenterol 2010;3:77-86. https://doi.org/10.1177/1756283X10361304.
- Loftus EV, Schoenfeld P, Sandborn WJ. The epidemiology and natural history of Crohn’s disease in population-based patient cohorts from North America: a systematic review. Aliment Pharmacol Ther 2002;16:51-60. https://doi.org/10.1046/j.1365-2036.2002.01140.x.
- Munkholm P, Langholz E, Davidsen M, Binder V. Disease activity courses in a regional cohort of Crohn’s disease patients. Scand J Gastroenterol 1995;30:699-706. https://doi.org/10.3109/00365529509096316.
- Lapidus A, Bernell O, Hellers G, Löfberg R. Clinical course of colorectal Crohn’s disease: a 35-year follow-up study of 507 patients. Gastroenterology 1998;114:1151-60. https://doi.org/10.1016/S0016-5085(98)70420-2.
- Regueiro M, Hashash JA. Overview of the Medical Management of Mild (Low Risk) Crohn Disease in Adults 2018. www.uptodate.com/contents/overview-of-the-medical-management-of-mild-low-risk-crohn-disease-in-adults (accessed 18 April 2019).
- Gomollón F, Dignass A, Annese V, Tilg H, Van Assche G, Lindsay JO, et al. 3rd European Evidence-based Consensus on the Diagnosis and Management of Crohn’s Disease 2016: Part 1: diagnosis and medical management. J Crohns Colitis 2017;11:3-25. https://doi.org/10.1093/ecco-jcc/jjw168.
- Duricova D, Burisch J, Jess T, Gower-Rousseau C, Lakatos PL. ECCO-EpiCom . Age-related differences in presentation and course of inflammatory bowel disease: an update on the population-based literature. J Crohns Colitis 2014;8:1351-61. https://doi.org/10.1016/j.crohns.2014.05.006.
- Hovde Ø, Moum BA . Epidemiology and clinical course of Crohn’s disease: results from observational studies. World J Gastroenterol 2012;18:1723-31. https://doi.org/10.3748/wjg.v18.i15.1723.
- García Rodríguez LA, González-Pérez A, Johansson S, Wallander MA. Risk factors for inflammatory bowel disease in the general population. Aliment Pharmacol Ther 2005;22:309-15. https://doi.org/10.1111/j.1365-2036.2005.02564.x.
- Rubin GP, Hungin AP, Kelly PJ, Ling J. Inflammatory bowel disease: epidemiology and management in an English general practice population. Aliment Pharmacol Ther 2000;14:1553-9. https://doi.org/10.1046/j.1365-2036.2000.00886.x.
- Floyd DN, Langham S, Séverac HC, Levesque BG. The economic and quality-of-life burden of Crohn’s disease in Europe and the United States, 2000 to 2013: a systematic review. Dig Dis Sci 2015;60:299-312. https://doi.org/10.1007/s10620-014-3368-z.
- Ghosh N, Premchand P. A UK cost of care model for inflammatory bowel disease. Frontline Gastroenterol 2015;6:169-74. https://doi.org/10.1136/flgastro-2014-100514.
- Shivananda S, Hordijk ML, Pena AS, Mayberry JF. Crohn’s disease: risk of recurrence and reoperation in a defined population. Gut 1989;30:990-5. https://doi.org/10.1136/gut.30.7.990.
- Maaser C, Sturm A, Vavricka SR, Kucharzik T, Fiorino G, Annese V, et al. ECCO-ESGAR Guideline for Diagnostic Assessment in IBD Part 1: initial diagnosis, monitoring of known IBD, detection of complications. J Crohns Colitis 2019;13:144-64. https://doi.org/10.1093/ecco-jcc/jjy113.
- Sturm A, Maaser C, Calabrese E, Annese V, Fiorino G, Kucharzik T, et al. ECCO-ESGAR Guideline for Diagnostic Assessment in IBD Part 2: IBD scores and general principles and technical aspects. J Crohns Colitis 2019;13:273-84. https://doi.org/10.1093/ecco-jcc/jjy114.
- Peyrin-Biroulet L, Panés J, Sandborn WJ, Vermeire S, Danese S, Feagan BG, et al. Defining disease severity in inflammatory bowel diseases: current and future directions. Clin Gastroenterol Hepatol 2016;14:348-54.e17. https://doi.org/10.1016/j.cgh.2015.06.001.
- Walmsley RS, Ayres RC, Pounder RE, Allan RN. A simple clinical colitis activity index. Gut 1998;43:29-32. https://doi.org/10.1136/gut.43.1.29.
- Harvey RF, Bradshaw JM. A simple index of Crohn’s-disease activity. Lancet 1980;1. https://doi.org/10.1016/S0140-6736(80)92767-1.
- Vermeire S, Schreiber S, Sandborn WJ, Dubois C, Rutgeerts P. Correlation between the Crohn’s disease activity and Harvey-Bradshaw indices in assessing Crohn’s disease severity. Clin Gastroenterol Hepatol 2010;8:357-63. https://doi.org/10.1016/j.cgh.2010.01.001.
- Iskandar HN, Ciorba MA. Biomarkers in inflammatory bowel disease: current practices and recent advances. Transl Res 2012;159:313-25. https://doi.org/10.1016/j.trsl.2012.01.001.
- National Institute for Health and Care Excellence (NICE) . Crohn’s Disease: Management 2019. www.nice.org.uk/guidance/ng129/chapter/Recommendations#inducing-remission-in-crohns-disease (accessed 5 August 2019).
- Rogler G. Top-down or step-up treatment in Crohn’s disease?. Dig Dis 2013;31:83-90. https://doi.org/10.1159/000347190.
- Tsui JJ, Huynh HQ. Is top-down therapy a more effective alternative to conventional step-up therapy for Crohn’s disease?. Ann Gastroenterol 2018;31:413-24.
- Sparrow MP, Melmed GY, Devlin S, Kozuch P, Raffals L, Loftus EV, et al. De-escalating medical therapy in Crohn’s disease patients who are in deep remission: a RAND appropriateness panel. GastroHep 2019;1:108-17. https://doi.org/10.1002/ygh2.337.
- D’Haens G, Baert F, van Assche G, Caenepeel P, Vergauwe P, Tuynman H, et al. Early combined immunosuppression or conventional management in patients with newly diagnosed Crohn’s disease: an open randomised trial. Lancet 2008;371:660-7. https://doi.org/10.1016/S0140-6736(08)60304-9.
- Fan R, Zhong J, Wang ZT, Li SY, Zhou J, Tang YH. Evaluation of ‘top-down’ treatment of early Crohn’s disease by double balloon enteroscopy. World J Gastroenterol 2014;20:14479-87. https://doi.org/10.3748/wjg.v20.i39.14479.
- Khanna R, Bressler B, Levesque BG, Zou G, Stitt LW, Greenberg GR, et al. Early combined immunosuppression for the management of Crohn’s disease (REACT): a cluster randomised controlled trial. Lancet 2015;386:1825-34. https://doi.org/10.1016/S0140-6736(15)00068-9.
- Kaul A, Hutfless S, Liu L, Bayless TM, Marohn MR, Li X. Serum anti-glycan antibody biomarkers for inflammatory bowel disease diagnosis and progression: a systematic review and meta-analysis. Inflamm Bowel Dis 2012;18:1872-84. https://doi.org/10.1002/ibd.22862.
- Segal AW. Making sense of the cause of Crohn’s – a new look at an old disease. F1000Res 2016;5. https://doi.org/10.12688/f1000research.9699.2.
- Mitsuyama K, Niwa M, Takedatsu H, Yamasaki H, Kuwaki K, Yoshioka S, et al. Antibody markers in the diagnosis of inflammatory bowel disease. World J Gastroenterol 2016;22:1304-10. https://doi.org/10.3748/wjg.v22.i3.1304.
- Kamm F, Strauch U, Degenhardt F, Lopez R, Kunst C, Rogler G, et al. Serum anti-glycan-antibodies in relatives of patients with inflammatory bowel disease. PLOS ONE 2018;13. https://doi.org/10.1371/journal.pone.0194222.
- Glycominds . Crohn’s Disease: Prognostic Serological Marker Profile 2016. http://ibdx.net/assets/img/flyers/cd_prognosis.pdf (accessed 8 August 2019).
- Glycominds . IBDX® ALCA IgG ELISA Kit. 2019. www.ibdx.net/assets/img/product/alca_insert.pdf (accessed 8 August 2019).
- Papp M, Foldi I, Altorjay I, Palyu E, Udvardy M, Tumpek J, et al. Anti-microbial antibodies in celiac disease: trick or treat?. World J Gastroenterol 2009;15:3891-900. https://doi.org/10.3748/wjg.15.3891.
- Román AL, Muñoz F. Comorbidity in inflammatory bowel disease. World J Gastroenterol 2011;17:2723-33. https://doi.org/10.3748/wjg.v17.i22.2723.
- Lee JC, Lyons PA, McKinney EF, Sowerby JM, Carr EJ, Bredin F, et al. Gene expression profiling of CD8+ T cells predicts prognosis in patients with Crohn disease and ulcerative colitis. J Clin Invest 2011;121:4170-9. https://doi.org/10.1172/JCI59255.
- McKinney EF, Lee JC, Jayne DR, Lyons PA, Smith KG. T-cell exhaustion, co-stimulation and clinical outcome in autoimmunity and infection. Nature 2015;523:612-16. https://doi.org/10.1038/nature14468.
- McKinney EF, Lyons PA, Carr EJ, Hollis JL, Jayne DR, Willcocks LC, et al. A CD8+ T cell transcription signature predicts prognosis in autoimmune disease. Nat Med 2010;16:586-91. https://doi.org/10.1038/nm.2130.
- Yi JS, Cox MA, Zajac AJ. T-cell exhaustion: characteristics, causes and conversion. Immunology 2010;129:474-81. https://doi.org/10.1111/j.1365-2567.2010.03255.x.
- Biasci D, Lee JC, Noor NM, Pombal DR, Hou M, Lewis N, et al. A blood-based prognostic biomarker in IBD. Gut 2019;68:1386-95. https://doi.org/10.1136/gutjnl-2019-318343.
- Parkes M, Noor NM, Dowling F, Leung H, Bond S, Whitehead L, et al. PRedicting Outcomes For Crohn’s dIsease using a moLecular biomarkEr (PROFILE): protocol for a multicentre, randomised, biomarker-stratified trial. BMJ Open 2018;8. https://doi.org/10.1136/bmjopen-2018-026767.
- National Institute for Health and Care Excellence (NICE) . PredictSURE-IBD and IBDX to Guide Personalised Treatment of Crohn’s Disease. Final Scope 2019. www.nice.org.uk/guidance/gid-dg10029/documents/final-scope (accessed 6 September 2019).
- Glycominds . Non-Invasive Solutions to Gastrointestinal Diseases Detection 2019. www.glycominds.com/ (accessed 20 August 2019).
- PredictImmune . PredictImmune 2019. www.predictimmune.com/ (accessed 20 August 2019).
- Barton S, Edwards SJ, Bacelar M, Karner C, Cain P. PredictSURE-IBD and IBDX to Guide Personalised Treatment of Crohn’s Disease in Adults 2019. www.crd.york.ac.uk/prospero/display_record.php?RecordID=138737 (accessed 20 August 2019).
- Centre for Reviews and Dissemination . Systematic Reviews: CRD’s Guidance for Undertaking Reviews in Healthcare 2009. www.york.ac.uk/media/crd/Systematic_Reviews.pdf (accessed 20 August 2019).
- National Institute for Health and Care Excellence (NICE) . Diagnostics Assessment Programme Manual 2011. www.nice.org.uk/Media/Default/About/what-we-do/NICE-guidance/NICE-diagnostics-guidance/Diagnostics-assessment-programme-manual.pdf (accessed 20 August 2019).
- Cochrane Methods . Screening and Diagnostic Tests. Handbook for DTA Reviews 2018. https://methods.cochrane.org/sdt/handbook-dta-reviews (accessed 20 August 2019).
- Hayden JA, Côté P, Bombardier C. Evaluation of the quality of prognosis studies in systematic reviews. Ann Intern Med 2006;144:427-37. https://doi.org/10.7326/0003-4819-144-6-200603210-00010.
- Hayden JA, van der Windt DA, Cartwright JL, Côté P, Bombardier C. Assessing bias in studies of prognostic factors. Ann Intern Med 2013;158:280-6. https://doi.org/10.7326/0003-4819-158-4-201302190-00009.
- Moons KGM, Wolff RF, Riley RD, Whiting PF, Westwood M, Collins GS, et al. PROBAST: a tool to assess risk of bias and applicability of prediction model studies: explanation and elaboration. Ann Intern Med 2019;170:W1-W33. https://doi.org/10.7326/M18-1377.
- Wolff RF, Moons KGM, Riley RD, Whiting PF, Westwood M, Collins GS, et al. PROBAST: a tool to assess the risk of bias and applicability of prediction model studies. Ann Intern Med 2019;170:51-8. https://doi.org/10.7326/M18-1376.
- Higgins J, Green S. Cochrane Handbook for Systematic Reviews of Interventions 2011. http://handbook-5-1.cochrane.org/ (accessed 18 April 2019).
- Sterne JA, Hernán MA, Reeves BC, Savović J, Berkman ND, Viswanathan M, et al. ROBINS-I: a tool for assessing risk of bias in non-randomised studies of interventions. BMJ 2016;355. https://doi.org/10.1136/bmj.i4919.
- Critical Appraisal Skills Programme . CASP Checklist: 10 Questions to Help You Make Sense of a Qualitative Research 2018. https://casp-uk.net/wp-content/uploads/2018/01/CASP-Qualitative-Checklist-2018.pdf (accessed 9 December 2020).
- Bonneau J, Dumestre-Perard C, Rinaudo-Gaujous M, Genin C, Sparrow M, Roblin X, et al. Systematic review: new serological markers (anti-glycan, anti-GP2, anti-GM-CSF Ab) in the prediction of IBD patient outcomes. Autoimmun Rev 2015;14:231-45. https://doi.org/10.1016/j.autrev.2014.11.004.
- Harrell L, Weyer G, Yarden J, Dotan N, Hanauer S. T1289. Anti-glycan antibodies are associated with disabling disease course and complicated disease behavior in patients with Crohn’s disease. Gastroenterology 2010;138. https://doi.org/10.1016/S0016-5085(10)62443-2.
- Kaul A, Hutfless S, Liu L, Bayless TM, Marohn MR, Li X. Serum anti-glycan antibody biomarkers for inflammatory bowel disease diagnosis and progression: a meta-analysis. Gastroenterology 2011;5. https://doi.org/10.1016/S0016-5085(11)60619-7.
- Paul S, Boschetti G, Rinaudo-Gaujous M, Moreau A, Del Tedesco E, Bonneau J, et al. Association of anti-glycan antibodies and inflammatory bowel disease course. J Crohns Colitis 2015;9:445-51. https://doi.org/10.1093/ecco-jcc/jjv063.
- Prideaux L, De Cruz P, Ng SC, Kamm MA. Serological antibodies in inflammatory bowel disease: a systematic review. Inflamm Bowel Dis 2012;18:1340-55. https://doi.org/10.1002/ibd.21903.
- Rieder F, Hahn P, Finsterhoelzl L, Dirmeier A, Cai H, Shen B, et al. Serum anti-glycan antibodies can contribute to differential diagnosis and disease stratification of pediatric Crohn’s disease patients. Gastroenterology 2010;138:S301-S302. https://doi.org/10.1016/S0016-5085(10)61387-X.
- Rieder F, Hahn P, Finsterhoelzl L, Schleder S, Wolf A, Dirmeier A, et al. Clinical utility of anti-glycan antibodies in pediatric Crohn’s disease in comparison with an adult cohort. Inflamm Bowel Dis 2012;18:1221-31. https://doi.org/10.1002/ibd.21854.
- Rieder F, Hahn P, Finsterholzl L, Dirmeier A, Shen B, Rogler G, et al. Clinical utility of anti-glycan antibodies in pediatric Crohn’s disease in comparison with an adult cohort. J Crohn’s Colitis 2011;5.
- Rieder F, Lopez R, Franke A, Wolf A, Schleder S, Dirmeier A, et al. Characterization of changes in serum anti-glycan antibodies in Crohn’s disease – a longitudinal analysis. PLOS ONE 2011;6. https://doi.org/10.1371/journal.pone.0018172.
- Rieder F, Schleder S, Wolf A, Dirmeier A, Strauch U, Obermeier F, et al. Association of the novel serologic anti-glycan antibodies anti-laminarin and anti-chitin with complicated Crohn’s disease behavior. Inflamm Bowel Dis 2010;16:263-74. https://doi.org/10.1002/ibd.21046.
- Rieder F, Schleder S, Wolf A, Dirmeier A, Strauch U, Obermeier F, et al. Serum anti-glycan antibodies predict complicated Crohn’s disease behavior: a cohort study. Inflamm Bowel Dis 2010;16:1367-75. https://doi.org/10.1002/ibd.21179.
- Rieder F, Schleder S, Wolf A, Schirbel A, Franke A, Dirmeier A, et al. Characterization of changes of serum anti-glycan antibodies in individual patients over time in inflammatory bowel disease (IBD). Gastroenterology 2010;138. https://doi.org/10.1016/S0016-5085(10)62412-2.
- Seow CH, Stempak JM, Xu W, Lan H, Griffiths AM, Greenberg GR, et al. Novel anti-glycan antibodies related to inflammatory bowel disease diagnosis and phenotype. Am J Gastroenterol 2009;104:1426-34. https://doi.org/10.1038/ajg.2009.79.
- Wolfel G, Lopez R, Hosl J, Kunst C, Martina M, Rieder F. The anti-glycan antibodies amca and alca are associated with shorter time to surgical recurrence in Crohn’s disease (CD). Gastroenterology 2017;152. https://doi.org/10.1016/S0016-5085(17)32158-3.
- Halder SL, Stempak JM, Sharaf A, Xu W, Greenberg GR, Steinhart H, et al. Biomarkers associated with progressive behaviour in Crohn’s disease. Gastroenterology 2010;138. https://doi.org/10.1016/S0016-5085(10)60173-4.
- International Standard Randomised Controlled Trials Number Registry . PROFILE – Personalised Medicine in Crohn’s Disease 2019. www.isrctn.com/ISRCTN11808228 (accessed 9 October 2019).
- ClinicalTrials.gov . The PRECIOUS Study: Predicting Crohn’s &Amp; ColitIs Outcomes in the United States 2019. https://clinicaltrials.gov/ct2/show/NCT03952364 (accessed 2 September 2019).
- Lyons P, Noor N, Lee JC, McKinney EF, Parkes M, Smith KGC. P256 Anti-glycan antibody seropositivity at diagnosis does not predict future disease course in patients with Crohn's disease. J Crohns Colitis 2020;14. https://doi.org/10.1093/ecco-jcc/jjz203.385.
- Smids C, Horjus Talabur Horje CS, Drylewicz J, Roosenboom B, Groenen MJM, van Koolwijk E, et al. Intestinal T cell profiling in inflammatory bowel disease: linking T cell subsets to disease activity and disease course. J Crohns Colitis 2018;12:465-75. https://doi.org/10.1093/ecco-jcc/jjx160.
- Canadian Agency for Drugs and Technologies in Health . Strings Attached: CADTH'S Database Search Filters n.d. https://cadth.ca/resources/finding-evidence/strings-attached-cadths-database-search-filters#eco (accessed January 2019).
- Arber M, Garcia S, Veale T, Edwards M, Shaw A, Glanville JM. Performance of Ovid MEDLINE search filters to identify health state utility studies. Int J Technol Assess Health Care 2017;33:472-80. https://doi.org/10.1017/S0266462317000897.
- Drummond M, Sculpher MJ, Claxton K, Stoddart GL, Torrance GW. Methods for the Economic Evaluation of Health Care Programmes. Oxford: Oxford University Press; 1997.
- Marchetti M, Liberato NL, Di Sabatino A, Corazza GR. Cost-effectiveness analysis of top-down versus step-up strategies in patients with newly diagnosed active luminal Crohn’s disease. Eur J Health Econ 2013;14:853-61. https://doi.org/10.1007/s10198-012-0430-7.
- Dretzke J, Edliln R, Round J, Connock M, Hulme C, Czeczot J, et al. A systematic review and economic evaluation of the use of tumour necrosis factor-alpha (TNF-a) inhibitors, adalimumab and infliximab, for Crohn’s disease. Health Technol Assess 2011;15. https://doi.org/10.3310/hta15060.
- Rafia R, Scope A, Harnan S, Stevens JW, Stevenson M, Lobo A. Vedolizumab for treating moderately to severely active Crohn’s disease after prior therapy: an evidence review group perspective of a NICE single technology appraisal. PharmacoEconomics 2016;34:1241-53. https://doi.org/10.1007/s40273-016-0436-6.
- Hodgson R, Walton M, Biswas M, Mebrahtu T, Woolacott N. Ustekinumab for treating moderately to severely active Crohn’s disease after prior therapy: an Evidence Review Group perspective of a NICE single technology appraisal. PharmacoEconomics 2018;36:387-98. https://doi.org/10.1007/s40273-017-0593-2.
- National Institute for Health and Care Excellence (NICE) . Therapeutic Monitoring of TNF-Alpha Inhibitors in Crohn’s Disease (LISA-TRACKER ELISA Kits, IDKmonitor ELISA Kits, and Promonitor ELISA Kits) n.d. www.nice.org.uk/guidance/dg22 (accessed January 2019).
- Bodger K, Kikuchi T, Hughes D. Cost-effectiveness of biological therapy for Crohn’s disease: Markov cohort analyses incorporating United Kingdom patient-level cost data. Aliment Pharmacol Ther 2009;30:265-74. https://doi.org/10.1111/j.1365-2036.2009.04033.x.
- Saito S, Shimizu U, Nan Z, Mandai N, Yokoyama J, Terajima K, et al. Economic impact of combination therapy with infliximab plus azathioprine for drug-refractory Crohn’s disease: a cost-effectiveness analysis. J Crohns Colitis 2013;7:167-74. https://doi.org/10.1016/j.crohns.2012.04.007.
- Mayberry JF, Lobo A, Ford AC, Thomas A. NICE clinical guideline (CG152): the management of Crohn’s disease in adults, children and young people. Aliment Pharmacol Therapeutics 2013;37:195-203. https://doi.org/10.1111/apt.12102.
- Freeman K, Connock M, Auguste P, Taylor-Phillips S, Mistry H, Shyangdan D, et al. Clinical effectiveness and cost-effectiveness of use of therapeutic monitoring of tumour necrosis factor alpha (TNF-α) inhibitors [LISA-TRACKER® enzyme-linked immunosorbent assay (ELISA) kits, TNF-α-Blocker ELISA kits and Promonitor® ELISA kits] versus standard care in patients with Crohn’s disease: systematic reviews and economic modelling. Health Technol Assess 2016;20. https://doi.org/10.3310/hta20830.
- Loftus EV, Johnson SJ, Yu AP, Wu EQ, Chao J, Mulani PM. Cost-effectiveness of adalimumab for the maintenance of remission in patients with Crohn’s disease. Eur J Gastroenterol Hepatol 2009;21:1302-9. https://doi.org/10.1097/MEG.0b013e32832a8d71.
- Lindsay J, Punekar YS, Morris J, Chung-Faye G. Health-economic analysis: cost-effectiveness of scheduled maintenance treatment with infliximab for Crohn’s disease – modelling outcomes in active luminal and fistulizing disease in adults. Aliment Pharmacol Ther 2008;28:76-87. https://doi.org/10.1111/j.1365-2036.2008.03709.x.
- Clark W, Raftery J, Song F, Barton P, Cummins C, Fry-Smith A, et al. Systematic review and economic evaluation of the effectiveness of infliximab for the treatment of Crohn’s disease. Health Technol Assess 2003;7. https://doi.org/10.3310/hta7030.
- Benedini V, Caporaso N, Corazza GR, Rossi Z, Fornaciari G, Cottone M, et al. Burden of Crohn’s disease: economics and quality of life aspects in Italy. Clinicoecon Outcomes Res 2012;4:209-18. https://doi.org/10.2147/CEOR.S31114.
- Mozzi A, Meregaglia M, Lazzaro C, Tornatore V, Belfiglio M, Fattore G. A comparison of EuroQol 5-Dimension health-related utilities using Italian, UK, and US preference weights in a patient sample. Clinicoecon Outcomes Res 2016;8:267-74. https://doi.org/10.2147/CEOR.S98226.
- Stark RG, Reitmeir P, Leidl R, König HH. Validity, reliability, and responsiveness of the EQ-5D in inflammatory bowel disease in Germany. Inflamm Bowel Dis 2010;16:42-51. https://doi.org/10.1002/ibd.20989.
- Rencz F, Lakatos PL, Gulacsi L, Brodszky V, Kurti Z, Lovas S, et al. Validity of the EQ-5D-5L and EQ-5D-3L in patients with Crohn’s disease. Qual Life Res 2019;28:141-52. https://doi.org/10.1007/s11136-018-2003-4.
- Dolan P, Gudex C, Kind P, Williams A. A Social Tariff for EuroQol: Results from a UK General Population Survey. Discussion Paper 138. York: Centre of Health Economics, University of York; 1995.
- Badia X, Roset M, Herdman M, Kind P. A comparison of United Kingdom and Spanish general population time trade-off values for EQ-5D health states. Med Decis Making 2001;21:7-16. https://doi.org/10.1177/0272989X0102100102.
- Dolan P, Gudex C, Kind P, Williams A. The time trade-off method: results from a general population study. Health Econ 1996;5:141-54. https://doi.org/10.1002/(SICI)1099-1050(199603)5:2<141::AID-HEC189>3.0.CO;2-N.
- Dolan P. Modeling valuations for EuroQol health states. Med Care 1997;35:1095-108. https://doi.org/10.1097/00005650-199711000-00002.
- Badia X, Roset M, Montserrat S, Herdman M, Segura A. The Spanish version of EuroQol: a description and its applications. European Quality of Life scale. Med Clin 1999;112:79-85.
- Casellas F, Vivancos JL, Sampedro M, Malagelada JR. Relevance of the phenotypic characteristics of Crohn’s disease in patient perception of health-related quality of life. Am J Gastroenterol 2005;100:2737-42. https://doi.org/10.1111/j.1572-0241.2005.00360.x.
- Casellas F, Rodrigo L, Niño P, Pantiga C, Riestra S, Malagelada JR. Sustained improvement of health-related quality of life in Crohn’s disease patients treated with infliximab and azathioprine for 4 years. Inflamm Bowel Dis 2007;13:1395-400. https://doi.org/10.1002/ibd.20205.
- Huamán JW, Casellas F, Borruel N, Peláez A, Torrejón A, Castells I, et al. Cutoff values of the Inflammatory Bowel Disease Questionnaire to predict a normal health related quality of life. J Crohns Colitis 2010;4:637-41. https://doi.org/10.1016/j.crohns.2010.07.006.
- Rue M, Badia X, Badia X, Herdman M, Segura A. EuroQol, Plenary Meeting. Barcelona: Institut Universitari de Salut Publiea de Catalunya; 1996.
- Casellas F, López-Vivancos J, Badia X, Vilaseca J, Malagelada JR. Impact of surgery for Crohn’s disease on health-related quality of life. Am J Gastroenterol 2000;95:177-82. https://doi.org/10.1111/j.1572-0241.2000.01681.x.
- Casellas F, Arenas JI, Baudet JS, Fábregas S, García N, Gelabert J, et al. Impairment of health-related quality of life in patients with inflammatory bowel disease: a Spanish multicenter study. Inflamm Bowel Dis 2005;11:488-96. https://doi.org/10.1097/01.MIB.0000159661.55028.56.
- Saro C, Ceballos D, Muñoz F, de la Coba C, Aguilar MD, Lázaro P, et al. Clinical status, quality of life, and work productivity in Crohn’s disease patients after one year of treatment with adalimumab. Rev Esp Enferm Dig 2017;109:122-9. https://doi.org/10.17235/reed.2016.4600/2016.
- Holko P, Kawalec P, Mossakowska M, Pilc A. Health-related quality of life impairment and indirect cost of Crohn’s disease: a self-report study in Poland. PLOS ONE 2016;11. https://doi.org/10.1371/journal.pone.0168586.
- Golicki D, Jakubczyk M, Niewada M, Wrona W, Busschbach JJ. Valuation of EQ-5D health states in Poland: first TTO-based social value set in Central and Eastern Europe. Value Health 2010;13:289-97. https://doi.org/10.1111/j.1524-4733.2009.00596.x.
- Devlin NJ, Shah KK, Feng Y, Mulhern B, van Hout B. Valuing health-related quality of life: an EQ-5D-5L value set for England. Health Econ 2018;27:7-22. https://doi.org/10.1002/hec.3564.
- National Institute for Health and Care Excellence (NICE) . Ustekinumab for Moderately to Severely Active Crohn’s Disease After Previous Treatment 2017. www.nice.org.uk/guidance/ta456 (accessed July 2019).
- Hoekman D, Stibbe J, Baert F, Caenepeel P, Vergauwe P, De Vos M, et al. Long-term outcome of early combined immunosuppression versus conventional management in newly diagnosed Crohn’s disease. J Crohns Colitis 2018;12:517-24. https://doi.org/10.1093/ecco-jcc/jjy014.
- Latimer N. NICE DSU Technical Support Document 14. Undertaking Survival Analysis for Economic Evaluations Alongside Clinical Trials – Extrapolation With Patient-Level Data 2013. http://nicedsu.org.uk/wp-content/uploads/2016/03/NICE-DSU-TSD-Survival-analysis.updated-March-2013.v2.pdf (accessed March 2021).
- Guyot P, Ades AE, Ouwens MJ, Welton NJ. Enhanced secondary analysis of survival data: reconstructing the data from published Kaplan-Meier survival curves. BMC Med Res Methodol 2012;12. https://doi.org/10.1186/1471-2288-12-9.
- Tsui JJ, Huynh HQ. Is top-down therapy a more effective alternative to conventional step-up therapy for Crohn’s disease?. Ann Gastroenterol 2018;31:413-24.
- National Institute for Health and Care Excellence (NICE) . British National Formulary 2019. https://bnf.nice.org.uk/ (accessed 30 October 2019).
- National Institute for Health and Care Excellence (NICE) . Vedolizumab for Treating Moderately to Severely Active Crohn’s Disease After Prior Therapy 2015. www.nice.org.uk/guidance/ta352 (accessed July 2019).
- Hanauer SB, Sandborn WJ, Rutgeerts P, Fedorak RN, Lukas M, MacIntosh D, et al. Human anti-tumor necrosis factor monoclonal antibody (adalimumab) in Crohn’s disease: the CLASSIC-I trial. Gastroenterology 2006;130:323-33. https://doi.org/10.1053/j.gastro.2005.11.030.
- Watanabe M, Hibi T, Lomax KG, Paulson SK, Chao J, Alam MS, et al. Adalimumab for the induction and maintenance of clinical remission in Japanese patients with Crohn’s disease. J Crohns Colitis 2012;6:160-73. https://doi.org/10.1016/j.crohns.2011.07.013.
- Feagan BG, Sandborn WJ, Gasink C, Jacobstein D, Lang Y, Friedman JR, et al. Ustekinumab as induction and maintenance therapy for Crohn’s disease. N Engl J Med 2016;375:1946-60. https://doi.org/10.1056/NEJMoa1602773.
- Sandborn WJ, Feagan BG, Rutgeerts P, Hanauer S, Colombel JF, Sands BE, et al. Vedolizumab as induction and maintenance therapy for Crohn’s disease. N Engl J Med 2013;369:711-21. https://doi.org/10.1056/NEJMoa1215739.
- Sandborn WJ, Gasink C, Gao LL, Blank MA, Johanns J, Guzzo C, et al. Ustekinumab induction and maintenance therapy in refractory Crohn’s disease. N Engl J Med 2012;367:1519-28. https://doi.org/10.1056/NEJMoa1203572.
- Sands BE, Feagan BG, Rutgeerts P, Colombel JF, Sandborn WJ, Sy R, et al. Effects of vedolizumab induction therapy for patients with Crohn’s disease in whom tumor necrosis factor antagonist treatment failed. Gastroenterology 2014;147:618-27.e3. https://doi.org/10.1053/j.gastro.2014.05.008.
- Hanauer SB, Feagan BG, Lichtenstein GR, Mayer LF, Schreiber S, Colombel JF, et al. Maintenance infliximab for Crohn’s disease: the ACCENT I randomised trial. Lancet 2002;359:1541-9. https://doi.org/10.1016/S0140-6736(02)08512-4.
- Office for National Statistics . National Life Tables: England and Wales. 2015–17 n.d. www.ons.gov.uk/peoplepopulationandcommunity/birthsdeathsandmarriages/lifeexpectancies/datasets/nationallifetablesenglandandwalesreferencetables (accessed October 2019).
- Silverstein MD, Loftus EV, Sandborn WJ, Tremaine WJ, Feagan BG, Nietert PJ, et al. Clinical course and costs of care for Crohn’s disease: Markov model analysis of a population-based cohort. Gastroenterology 1999;117:49-57. https://doi.org/10.1016/S0016-5085(99)70549-4.
- Ara R, Brazier JE. Populating an economic model with health state utility values: moving toward better practice. Value Health 2010;13:509-18. https://doi.org/10.1111/j.1524-4733.2010.00700.x.
- Office for National Statistics . Consumer Price Inflation Index for Medical Services (DKC3) 2018. www.ons.gov.uk/economy/inflationandpriceindices/timeseries/dkc3/mm23 (accessed 12 December 2018).
- Department of Health and Social Care (NHS Improvement) . NHS National Schedule of Reference Costs 2017 to 2018 n.d. https://improvement.nhs.uk/resources/reference-costs/ (accessed November 2019).
- Curtis L, Burns A. Unit Costs of Health and Social Care 2018. Canterbury: Personal Social Services Research Unit, University of Kent; 2018.
- Wailoo A, Tosh J. Use of Tumour Necrosis Factor Alpha (TNF A) Inhibitors (Adalimumab and Infliximab) for Crohn’s Disease: Report by the Decision Support Unit 2009.
- Martin AD, Quinn KM, Park JH. MCMCpack: Markov Chain Monte Carlo in R. J Stat Software 2011;42:1-21. https://doi.org/10.18637/jss.v042.i09.
- Venables WN, Ripley BD. Modern Applied Statistics with S. New York, NY: Springer; 2002.
- National Institute for Health and Care Excellence (NICE) . Guide to the Methods of Technology Appraisal 2013 2013. www.nice.org.uk/process/pmg9/chapter/foreword (accessed 18 December 2018).
- Baert FJ, Moortgat L, Van Assche GA, Caenepeel P, Vergauwe PL, De Vos M, et al. Mucosal healing predicts sustained clinical remission in early Crohn’s disease. Gastroenterology 2010;138:463-8. https://doi.org/10.1053/j.gastro.2009.09.056.
- Chau NA, Romero D, Toolsie P, Jimmy J, Bleidt BA. Assessment of biological agents in the treatment regimen of moderate to severe Crohn’s disease. Pharmacotherapy 2015;35:e299-300.
- Colombel JF, Ungaro R, Aggarwal S, Topaloglu O, Skup M, Lee WJ. Efficacy and safety of early biologic treatment of Crohn’s disease in adult and paediatric patients: a systematic review. J Crohns Colitis 2018;12. https://doi.org/10.1093/ecco-jcc/jjx180.819.
- Hirschmann S, Neurath MF. Top-down approach to biological therapy of Crohn’s disease. Expert Opinion Biological Ther 2017;17:285-93. https://doi.org/10.1080/14712598.2017.1287170.
- Hommes DW. Step-up versus top-down therapy in the treatment of Crohn’s disease. Gastroenterol Hepatol 2006;2:546-7.
- Hutfless S, Lau BD, Wilson LM, Lazarev M, Bass EB. Pharmacological Management of Crohn’s Disease: Future Research Needs. Rockville, MD: Agency for Healthcare Research and Quality; 2014.
- Katz JA. Postoperative endoscopic surveillance in Crohn’s disease: bottom up or top down?. Gastrointest Endosc 2007;66:541-3. https://doi.org/10.1016/j.gie.2007.02.060.
- Kuznar W, Writer M. Step-up therapy program for anti-inflammatory biologic agents does not increase cost nor adversely affect patient outcomes n.d. https://ahdbonline.com/issues/2013/may-june-2013-vol-6-no-4/1419-article-1419 (accessed June 2019).
- Lee J, Biasci D, Noor N, McKinney E, Ahmad T, Lewis N, et al. PROFILE trial: predicting outcomes for Crohn’s disease using a molecular biomarker. J Crohns Colitis 2017;11. https://doi.org/10.1093/ecco-jcc/jjx002.085.
- Meier J, Sturm A. Top-down versus step-up: new strategies in the treatment of Crohn’s disease. Z Gastroenterol 2009;47:240-2. https://doi.org/10.1055/s-0028-1109073.
- Peyrin-Biroulet L, Bigard MA, Malesci A, Danese S. Step-up and top-down approaches to the treatment of Crohn’s disease: early may already be too late. Gastroenterology 2008;135:1420-2. https://doi.org/10.1053/j.gastro.2008.08.017.
- Sucong L, Baili C, Yinglian X, Kang C, Yao H, Zhirong Z, et al. Endoscopic and clinical follow-up of step-up and top-down infliximab therapy in Crohn’s disease. J Gastroenterol Hepatol 2013;28.
- Xiao Y, Chen B, He Y, Gao X, Huang M, Hu P, et al. The Clinical and Endoscopic Efficacy of Step-up and Top-down Infliximab Therapy in Crohn’s Disease 2012. www.cochranelibrary.com/central/doi/10.1002/central/CN-00914623/full (accessed 2019).
- Colombel JF, Sandborn WJ, Rutgeerts P, Enns R, Hanauer SB, Panaccione R, et al. Adalimumab for maintenance of clinical response and remission in patients with Crohn’s disease: the CHARM trial. Gastroenterology 2007;132:52-65. https://doi.org/10.1053/j.gastro.2006.11.041.
- Sandborn WJ, Hanauer SB, Rutgeerts P, Fedorak RN, Lukas M, MacIntosh DG, et al. Adalimumab for maintenance treatment of Crohn’s disease: results of the CLASSIC II trial. Gut 2007;56:1232-9. https://doi.org/10.1136/gut.2006.106781.
- Rutgeerts P, Van Assche G, Sandborn WJ, Wolf DC, Geboes K, Colombel JF, et al. Adalimumab induces and maintains mucosal healing in patients with Crohn’s disease: data from the EXTEND trial. Gastroenterology 2012;142:1102-11.e2. https://doi.org/10.1053/j.gastro.2012.01.035.
- Sandborn WJ, Rutgeerts P, Enns R, Hanauer SB, Colombel JF, Panaccione R, et al. Adalimumab induction therapy for Crohn disease previously treated with infliximab: a randomized trial. Ann Intern Med 2007;146:829-38. https://doi.org/10.7326/0003-4819-146-12-200706190-00159.
- Targan SR, Hanauer SB, van Deventer SJ, Mayer L, Present DH, Braakman T, et al. A short-term study of chimeric monoclonal antibody cA2 to tumor necrosis factor alpha for Crohn’s disease. Crohn’s Disease cA2 Study Group. N Engl J Med 1997;337:1029-35. https://doi.org/10.1056/NEJM199710093371502.
- National Institute for Health and Care Excellence (NICE) . Briefing Paper for Methods Review Working Party on Companion Diagnostics 2011. http://nicedsu.org.uk/wp-content/uploads/2016/03/DSU_TAMethodsGuideReviewSupportingDocuments.pdf (accessed 26 January 2021).
Appendix 1 Risk of developing a complication or need for surgery based on number of positive biomarkers in the IBDX tool
Outcome | n | Population | Result | p-value |
---|---|---|---|---|
aComplication75 | Unclear | CD | OR 1.5 (95% CI 1.3 to 1.9) | < 0.001 |
bComplication76 (subgroup of people experiencing a complication) | 20 | CD but no prior complication or surgery | ||
|
HR 1.8 (95% CI 0.61 to 5.4) | 0.29 | ||
|
HR 2.5 (95% CI 1.03 to 6.1) | 0.043 | ||
|
HR 2.6 (95% CI 0.92 to 7.2) | 0.072 |
Outcome | n | Population | Result | p-value |
---|---|---|---|---|
aSurgery75 | Unclear | People with CD | OR 1.5 (95% CI 1.3 to 1.8) | < 0.001 |
bSurgery76 (subgroup of people undergoing surgery) | 14 | CD but no prior complication or surgery | ||
|
HR 2.6 (95% CI 0.58 to 12.0) | 0.21 | ||
|
HR 3.6 (95% CI 1.2 to 11.0) | 0.023 | ||
|
HR 2.8 (95% CI 0.80 to 9.6) | 0.11 | ||
cSurgery78 (abdominal) | 517 | People with CD | ||
|
103 | 51.64% | < 0.0001 | |
|
130 | 54.62% | ||
|
77 | 63.64% | ||
|
36 | 57.89% | ||
|
36 | 76.67% |
Appendix 2 Measure-of-fit statistics
Distribution | AIC | BIC |
---|---|---|
Exponential | 150.95 | 152.09 |
Weibull | 152.11 | 154.38 |
Gompertz | 150.13 | 152.40 |
Log-normal | 149.17 | 151.44 |
Log-logistic | 149.99 | 152.26 |
Gamma | 149.98 | 153.39 |
Distribution | AIC | BIC |
---|---|---|
Exponential | 48.79 | 49.62 |
Weibull | 50.60 | 52.27 |
Gompertz | 49.08 | 50.75 |
Log-normal | 49.47 | 51.14 |
Log-logistic | 50.17 | 51.83 |
Gamma | 46.68 | 49.18 |
Distribution | AIC | BIC |
---|---|---|
Exponential | 326.49 | 330.75 |
Weibull | 325.43 | 331.81 |
Gompertz | 328.43 | 334.81 |
Log-normal | 315.92 | 322.30 |
Log-logistic | 318.59 | 324.97 |
Gamma | 299.47 | 307.97 |
Distribution | AIC | BIC |
---|---|---|
Exponential | 305.93 | 310.18 |
Weibull | 306.88 | 313.26 |
Gompertz | 301.24 | 307.62 |
Log-normal | 301.18 | 307.56 |
Log-logistic | 303.08 | 309.46 |
Gamma | 301.15 | 309.66 |
Distribution | AIC | BIC |
---|---|---|
Exponential | 220.04 | 222.50 |
Weibull | 220.68 | 225.61 |
Gompertz | 216.82 | 221.76 |
Log-normal | 217.81 | 222.74 |
Log-logistic | 219.72 | 224.65 |
Gamma | 216.25 | 223.65 |
Distribution | AIC | BIC |
---|---|---|
Exponential | 278.12 | 283.67 |
Weibull | 279.84 | 288.18 |
Gompertz | 280.00 | 288.34 |
Log-normal | 279.29 | 287.63 |
Log-logistic | 279.72 | 288.05 |
Gamma | 281.24 | 292.36 |
Appendix 3 Time to relapse truncated curves
FIGURE 25.
Step-up time to relapse curves fitted with Gompertz, log-normal and gamma (truncated data).
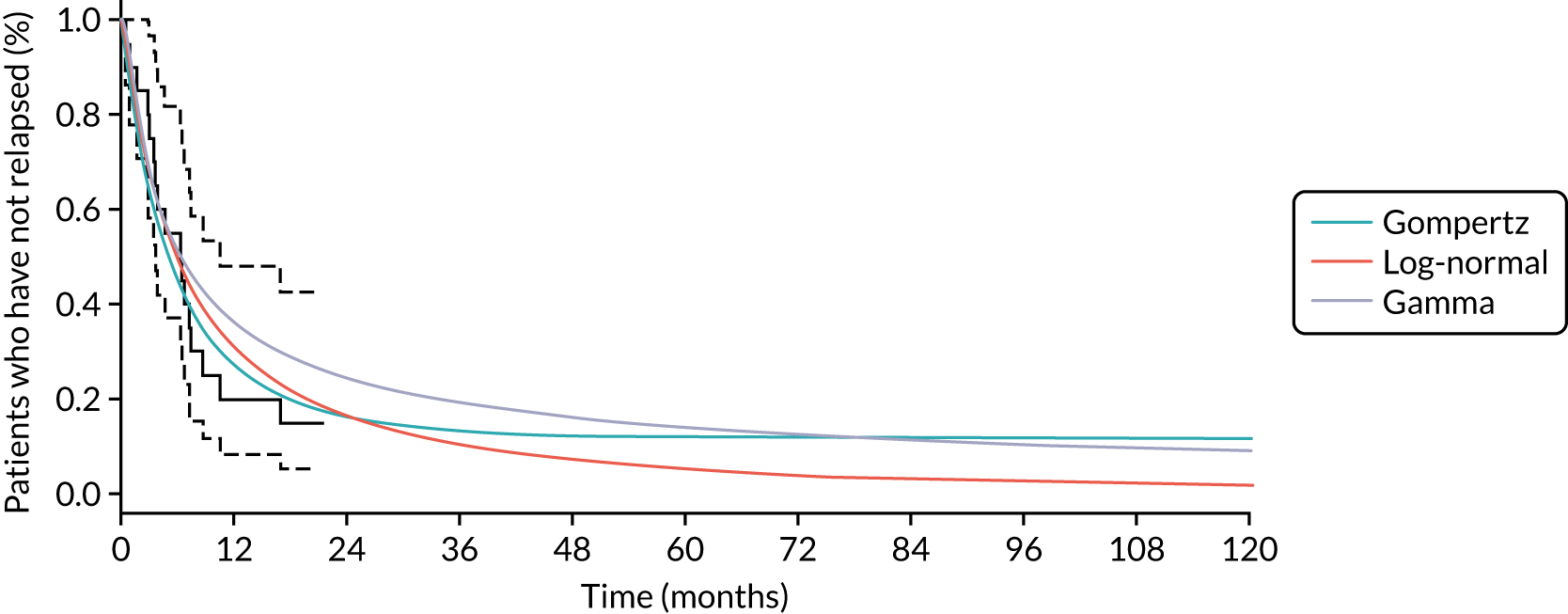
FIGURE 26.
Top-down time to relapse curves fitted with Gompertz, log-normal and gamma (truncated data).
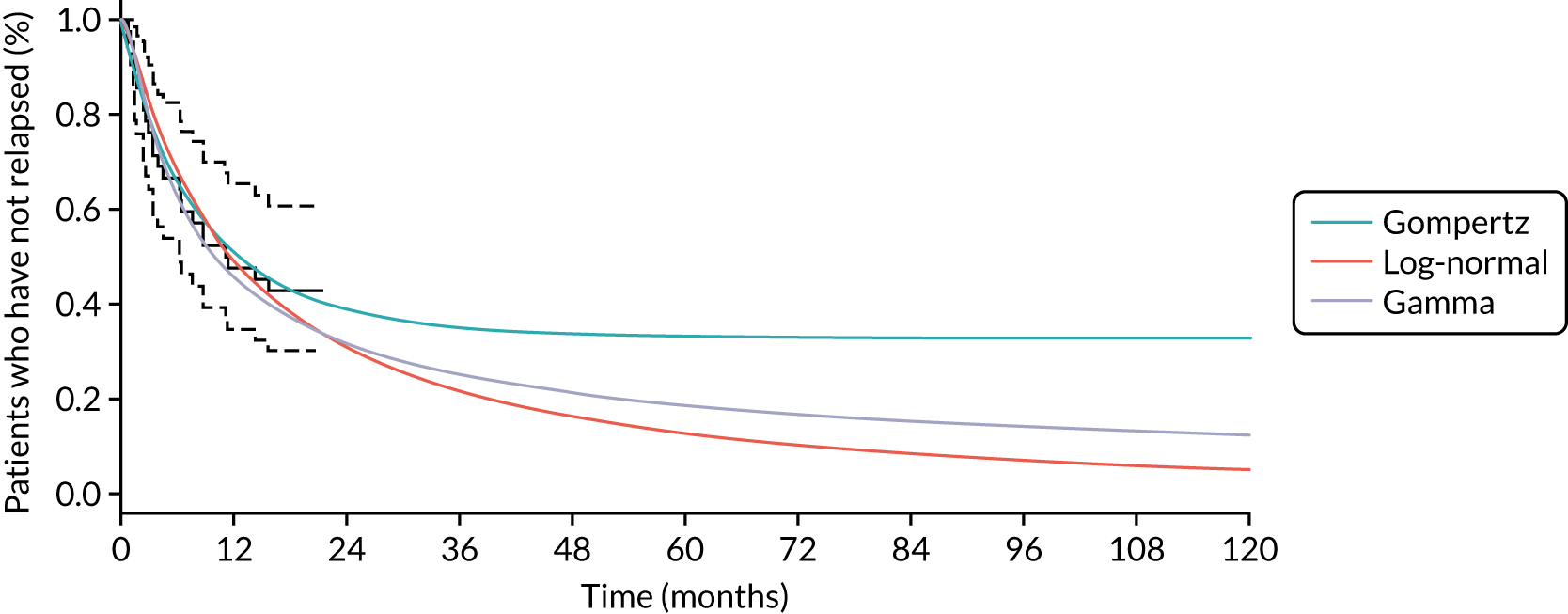
Appendix 4 Comparison of time to treatment escalation curves
FIGURE 27.
Comparison of TTE curves across treatment strategies for high-risk patients (base case). (a) Anti-TNF vs. IM (first step); (b) first biologic vs. anti-TNF (second step); and (c) second biologic vs. first biologic (third step).

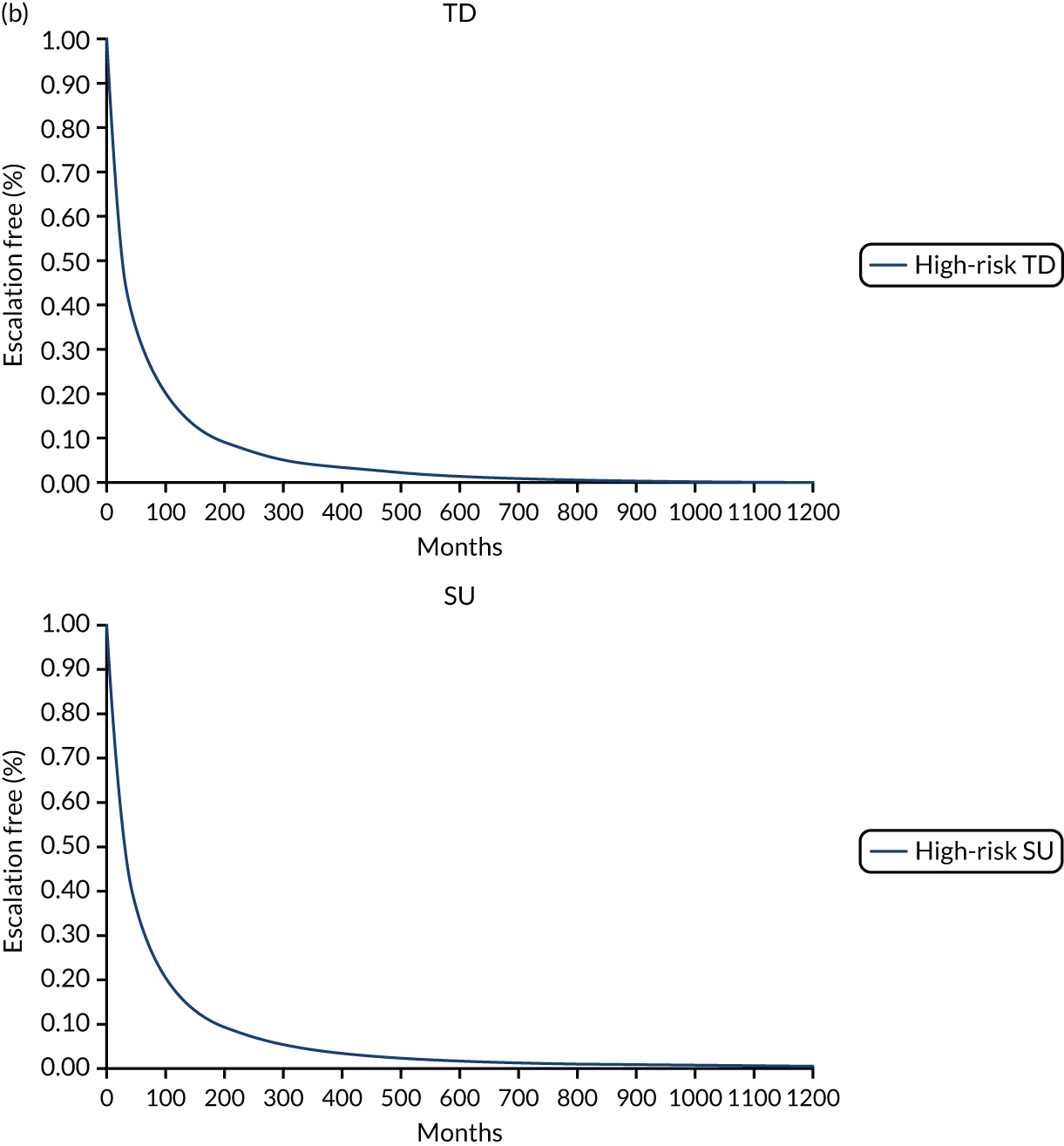

FIGURE 28.
Comparison of TTE curves across treatment strategies for high-risk patients (scenario analysis 1 with SU time to escalation from step 2 estimated with half of the base-case relative hazard). (a) Anti-TNF vs. IM (first step); (b) first biologic vs. anti-TNF (second step); and (c) second biologic vs. first biologic (third step).


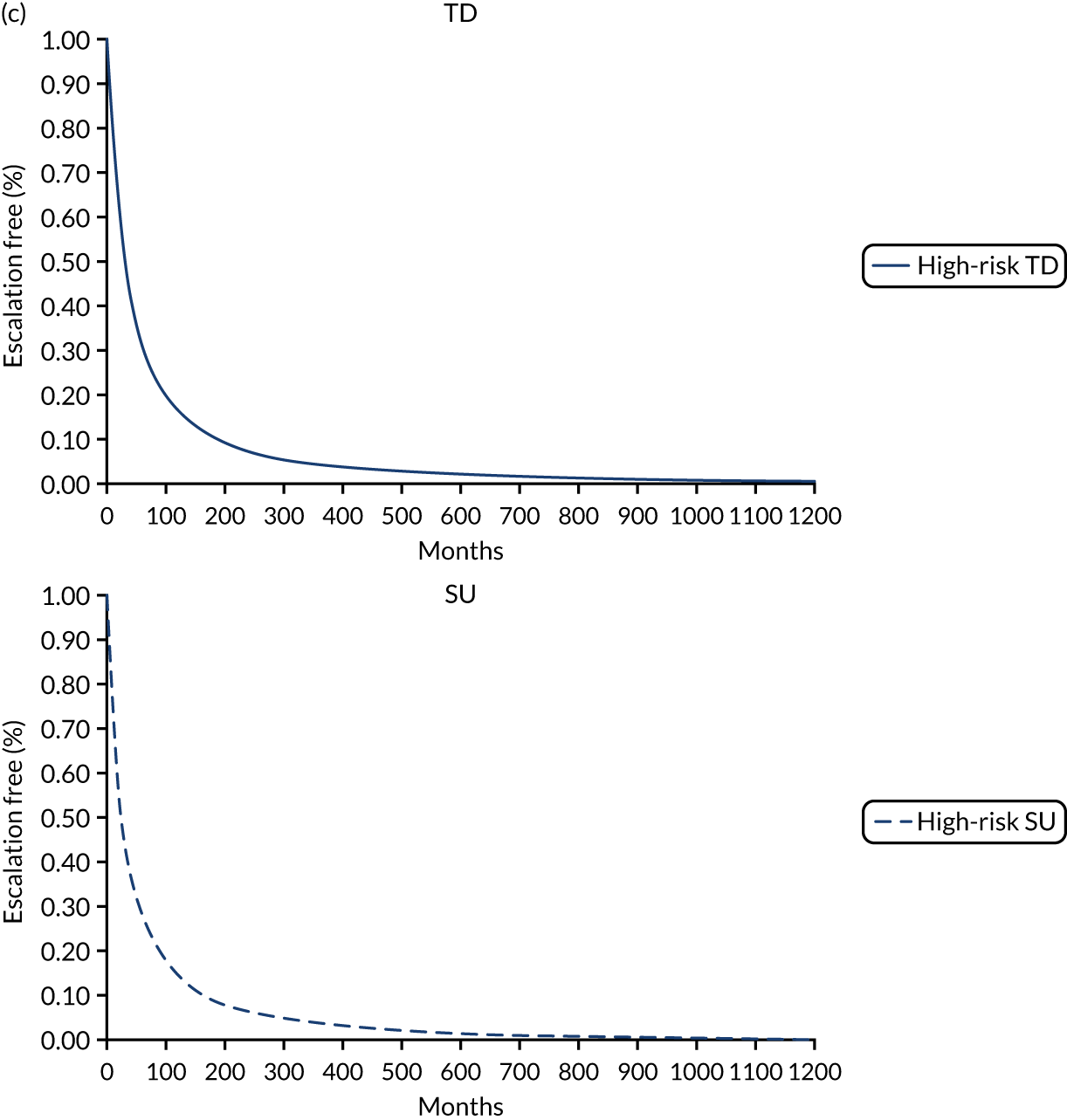
FIGURE 29.
Comparison of TTE curves across treatment strategies for high-risk patients (scenario analysis 2 with SU and TD time to escalation from step 2 estimated with half of the base-case relative hazard). (a) Anti-TNF vs. IM (first step); (b) first biologic vs. anti-TNF (second step); and (c) second biologic vs. first biologic (third step).



Appendix 5 Search strategies and list of excluded studies for literature review to inform estimates of clinical effectiveness of induction (step up and top down) and maintenance treatment
Step up and top down
Ovid MEDLINE(R) and Epub Ahead of Print, In-Process & Other Non-Indexed Citations and Daily and Versions(R): database searched from inception to 14 June 2019 | ||
---|---|---|
# | Terms | Hits |
1 | Crohn Disease/ | 37,169 |
2 | Crohn*.mp | 53,162 |
3 | ((Crohn$adj2 (disease or syndrome)) or regional enteritis).tw. | 42,992 |
4 | Inflammatory bowel diseases/ | 20,151 |
5 | IBD.mp. | 22,462 |
6 | Inflammatory bowel disease*.mp. | 48,138 |
7 | or/1-6 | 84,595 |
8 | (top-down or top down or step-up or step up).ti,ab. | 15,774 |
9 | 7 and 8 | 191 |
EMBASE: database searched from inception to 14 June 2019 | ||
---|---|---|
# | Terms | Hits |
1 | Exp Crohn Disease/ | 83,531 |
2 | Crohn*.mp | 94,568 |
3 | ((Crohn$adj2 (disease or syndrome)) or regional enteritis).tw. | 68,633 |
4 | Exp Inflammatory bowel disease/ | 134,801 |
5 | IBD.mp. | 46,227 |
6 | Inflammatory bowel disease*.mp. | 79,562 |
7 | or/1-6 | 168,160 |
8 | (top-down or top down or step-up or step up).ti,ab. | 18,369 |
9 | 7 and 8 | 472 |
CENTRAL and CDSR: database searched from inception to 14 June 2019 | ||
---|---|---|
# | Terms | Hits |
1 | Crohn:ti,ab,kw | 4482 |
2 | MeSH: [Inflammatory bowel diseases] explode all trees | 2889 |
3 | IBD:ti,ab,kw | 1738 |
4 | ‘Inflammatory bowel disease’:ti,ab,kw | 2650 |
5 | #1 or #2 or #3 or #4 | 7295 |
6 | ‘top-down’ or ‘top down’ or ‘step-up’ or ‘step up’:ti,ab,kw | 1194 |
7 | #5 or #6 | 43 |
Study (first author and year) | Reason for exclusion |
---|---|
Chau 2015144 | Focuses on treatment with biological therapy rather than SU vs. TD |
Colombel 2018145 | Focuses on treatment with biological therapy rather than SU vs. TD |
Fan 201436 | RCT included in chosen SR |
Hirschmann 2017146 | Not SR |
Hommes 2006147 | Not SR |
Hutfless 2014148 | Book chapter |
Katz 2007149 | Not SR |
Kuznar 2013150 | Not SR |
Lee 2017151 | Not SR |
Meier 2009152 | Not SR |
Parkes 201851 | Not SR |
Peyrin-Biroulet 2018153 | Not SR |
Sucong 2013154 | Not SR |
Xiao 2012155 | Not SR |
Effectiveness of induction and maintenance therapies
The reasons for excluding studies identified from TA352125 (vedolizumab) and TA456119 (ustekinumab) from the EAG’s analyses are presented in Table 38. The EAG notes that the key differences between the analyses carried out by the EAG and those presented in TA352 and TA456 are exclusion by the EAG of the study carried out by Targan et al. 160 (single dose of 5 mg of infliximab administered) and inclusion of subgroup data from the anti-TNF-naive subgroup of the study reported by Watanabe et al. 127 (see Table 38). In addition, the EAG notes that studies of ustekinumab were not included in TA352, whereas they were included in both TA456 and the EAG analyses.
Study name | Intervention | Induction EAG analysis | Notes | Maintenance EAG analysis | Notes |
---|---|---|---|---|---|
Studies from TA352125 | |||||
ACCENT I132 | Infliximab | N/A | – | Included | Data available on use of infliximab in anti-TNF-naive patients at the start of induction therapy |
CHARM156 | Adalimumab | N/A | – | Excluded | 47.7% patients in the study had received anti-TNF before the induction study. Subgroup data were not available for maintenance treatment of those who were anti-TNF naive at induction |
CLASSIC-I126 | Adalimumab | Included | Data extracted for anti-TNF-naive subgroup for 160/80 mg dose of adalimumab | N/A | – |
CLASSIC-II157 | Adalimumab | N/A | – | Excluded | All patients were required to be in remission at start of maintenance treatment rather than to have achieved a set level of response to induction therapy; other studies specify a cut-off point for response |
EXTEND158 | Adalimumab | Excluded | 46.9% of patients had prior anti-TNF exposure and subgroup data were not available for the anti-TNF-naive patients. It was noted that prior exposure did not include patients with primary non-response | Excluded | Maintenance adalimumab arm includes patients with non-response from induction (CDAI did not decrease by ≥ 70). In addition, 46.9% of people had received prior anti-TNF, but it is acknowledged that they were not classed as ‘primary non-response’ |
GAIN159 | Adalimumab | Excluded | Prior failure of or intolerance to infliximab was required; therefore, the patients were not anti-TNF naive | N/A | – |
Watanabe 2012127 | Adalimumab | Included | Data extracted for 160/80 mg dose of adalimumab from the anti-TNF naive subgroup | Excluded | 52% of patients in the study had received anti-TNF before entering the induction study. Data were not available for maintenance therapy in the subgroup of anti-TNF-naive patients |
Targan 1997160 | Infliximab | Excluded | Single dose of infliximab, which is not standard protocol or in keeping with other drugs in the analysis; typically, more than one dose would be expected for induction therapy | N/A | – |
GEMINI II129 | Vedolizumab | Included | Data on vedolizumab | Included | Data on vedolizumab |
GEMINI III131 | Vedolizumab | Included | Data on vedolizumab | N/A | – |
Additional studies from TA456119 | |||||
CERTIFI130 | Ustekinumab | Included | Data were available for ustekinumab from the prior anti-TNF failure subgroup. Note that data from the 6 mg/kg arm have been used, as this dose was deemed to be the most similar to the licensed dose | N/A | The study had some maintenance end points but these were assessed at 22 weeks and not 52 weeks, as in other studies, and were therefore excluded from analyses of maintenance |
UNITI-1119,128 | Ustekinumab | Included | Data were extracted on ustekinumab for the subgroup of those failing prior anti-TNF. Note that data from the 6 mg/kg arm have been used, as this dose was deemed to be the most similar to the licensed dose | N/A | – |
UNITI-2119,130 | Ustekinumab | Excluded | Less than 40% of patients had a history of anti-TNF treatment, and the study inclusion criteria restricted the patients who had previously received one or more TNF antagonists to those who had not had unacceptable side effects and had not met the criteria for primary or secondary non-response to treatment | N/A | – |
IM-UNITI128 | Ustekinumab | N/A | – | Included | Data were extracted on ustekinumab for the subgroup of those failing prior anti-TNF |
Appendix 6 Clinical estimates informing the model
Clinical outcome | Induction (%) | Maintenance (%) | ||
---|---|---|---|---|
Response | Remission | Response | Remission | |
TD | ||||
Biologics | – | – | – | – |
Anti-TNF | – | 66 | – | – |
SU | ||||
Biologics | 30 | 15 | – | 28 |
Anti-TNF | 26 | 37 | 10 | 33 |
IM | – | 26 | – | – |
Annual transition | First step (annual probabilities) | First step (2-week probabilities) |
---|---|---|
TD | ||
Anti-TNF | 7.27 × 10–11 | 0.0630 |
First- and second-line biologics | 3.09 × 10–5 | 0.0072 |
SU | ||
IM | 2.85 × 10–10 | 0.0034 |
Anti-TNF | 7.27 × 10–11 | 0.0630 |
First- and second-line biologics | 3.09 × 10–5 | 0.0072 |
Clinical outcome | Induction (%) | |||
---|---|---|---|---|
Remission | Mild | Moderate/severe | No response | |
TD | ||||
Biologics | 13 | 25 | 7 | 55 |
Anti-TNF | 32 | 23 | 6 | 38 |
SU | ||||
Biologics | 13 | 25 | 7 | 55 |
Anti-TNF | 32 | 23 | 6 | 38 |
IM | 16 | 18 | 5 | 62 |
Clinical outcome | Maintenance (%) | |||
---|---|---|---|---|
Remission | Mild | Moderate/severe | No response | |
TD | ||||
Biologics | 28 | 1 | 0 | 70 |
Anti-TNF | 48 | 9 | 3 | 41 |
SU | ||||
Biologics | 28 | 1 | 0 | 70 |
Anti-TNF | 48 | 9 | 3 | 41 |
IM | 25 | 12 | 3 | 60 |
Variable | Value/assumption in EAG model | Measurement of uncertainty/distribution in EAG’s model | Source |
---|---|---|---|
Model settings | |||
Time horizon (years) | 65 | Fixed | Assumption |
Discount rate for costs and benefits | 3.5% | Fixed | NICE guidelines161 |
Days in a cycle | 14.00 | Fixed | Assumption |
Patients’ characteristics | |||
Age (years) | 35 | Gamma | Biasci et al.50 IPD |
Patients’ weight (kg) | 71.4 | Gamma | Assumption |
Proportion of males | 0.38 | Beta | Biasci et al.50 IPD |
Probability of high-risk disease course | 0.58 | Beta | Biasci et al.50 IPD |
Diagnostic test accuracy | |||
Probability of PredictSURE-IBD identifying high risk correctly | 1.00 | Beta | See Methods for assessing cost-effectiveness |
Probability of IBDX identifying low risk correctly | 1.00 | Beta | See Methods for assessing cost-effectiveness |
Treatment bundles | |||
Proportion on infliximab in anti-TNF biologics bundle | 0.40 | Beta | Clinical expert opinion |
Proportion on adalimumab in anti-TNF biologics bundle | 0.60 | 1− Proportion on infliximab in anti-TNF biologics bundle | Clinical expert opinion |
Proportion on vedolizumab in non-anti-TNF biologics bundle | 0.50 | Beta | Clinical expert opinion |
Proportion on ustekinumab in non-anti-TNF biologics bundle | 0.50 | 1− Proportion on vedolizumab in non-anti-TNF biologics bundle | Clinical expert opinion |
Proportion on azathioprine in IM bundle | 0.80 | Beta | Clinical expert opinion |
Proportion of 6-mercaptopurine in IM bundle | 0.10 | Beta | Clinical expert opinion |
Proportion of methotrexate in IM bundle | 0.10 | 1− (Proportion of 6-mercaptopurine in IM bundle + proportion of methotrexate in IM bundle) | Clinical expert opinion |
Proportion of patients receiving IM in anti-TNF bundle | 0.30 | Gamma | Clinical expert opinion |
Proportion of patients receiving IM in non-anti-TNF biologic bundle | 0.20 | Gamma | Clinical expert opinion |
Induction period | |||
Time spent in induction state with IMs (weeks) | 8 | Gamma | BNF124/clinical expert opinion |
Time spent in induction state with anti-TNF (weeks) | 4 | Gamma | BNF124/clinical expert opinion |
Time spent in induction state with biologics (weeks) | 8 | Gamma | BNF124/clinical expert opinion |
Mortality | |||
Probability of death following surgery | 0.0015 | Beta | Marchetti et al.88 |
Diagnostic test cost | |||
PredictSURE cost | £1250 | Fixed | Company’s reply to request for information |
IBDX cost | £347 | Uniform | Company’s reply to request for information and EAG’s assumptions |
Health state costs per cycle | |||
Remission | £17 | Gamma | Clinical expert opinion |
Mild | £27 | Gamma | Clinical expert opinion |
Moderate/severe | £122 | Gamma | Clinical expert opinion |
No response | £122 | Gamma | Clinical expert opinion |
Surgery | £8813 | Gamma | NHS Reference Costs 2017–18 137 |
Treatment costs | |||
Induction: anti-TNF | £1525 | Gamma | BNF124/clinical expert opinion |
Induction: biologic | £1545 | Gamma | BNF124/clinical expert opinion |
Induction: IM | £4.43 | Gamma | BNF124/clinical expert opinion |
Maintenance: anti-TNF | £536.46 | Gamma | BNF124/clinical expert opinion |
Maintenance: biologic | £656.47 | Gamma | BNF124/clinical expert opinion |
Maintenance: IM | £12.10 | Gamma | BNF124/clinical expert opinion |
i.v. administration: first attendance | £199 | Gamma | NHS Reference Costs 2017–18 137 |
i.v. administration: follow-up | £212 | Gamma | NHS Reference Costs 2017–18 137 |
Utility | |||
Remission | 0.82 | Beta | TA352125 |
Mild | 0.73 | Beta | TA352125 |
Moderate to severe | 0.57 | Beta | TA352125 |
No response | 0.57 | Beta | Assumption |
Appendix 7 Time to surgery curves
FIGURE 30.
Step-up TTS curves (with CIs) fitted with log-normal, log-logistic and exponential models.

FIGURE 31.
Top-down TTS curves (with CIs) fitted with log-normal, log-logistic and exponential models.

Appendix 8 General population survival in the model
FIGURE 32.
General population survival in the model.
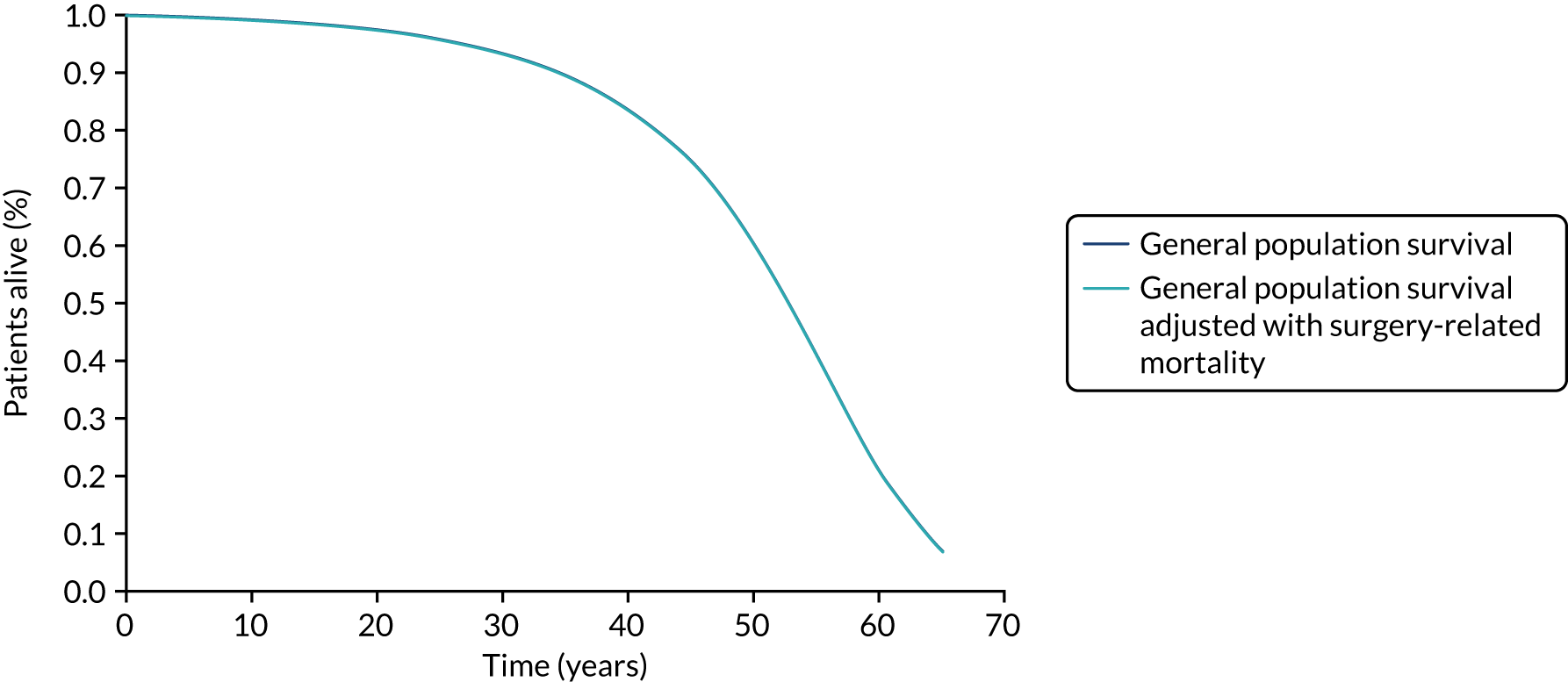
Appendix 9 Cost-effectiveness acceptability curve
FIGURE 33.
Cost-effectiveness acceptability curve. SoC, standard of care.
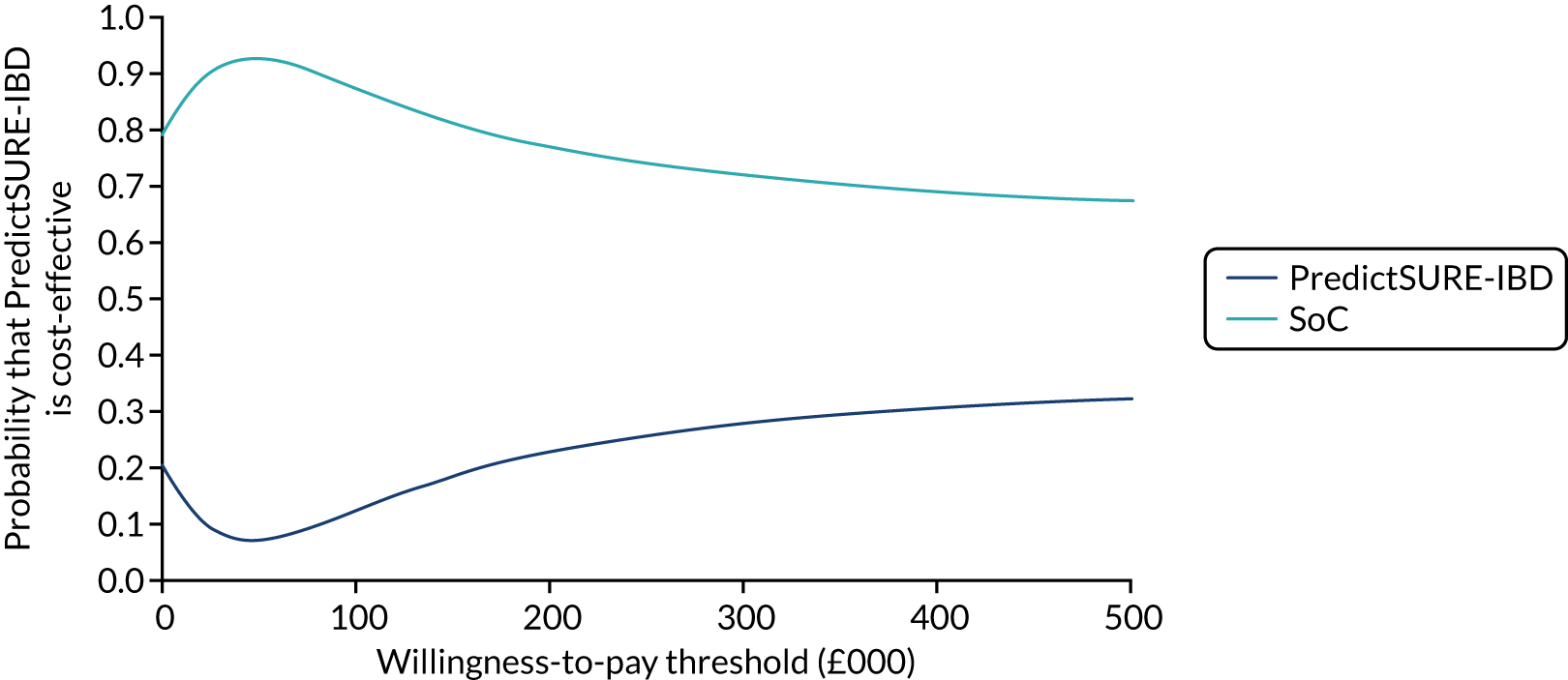
FIGURE 34.
Scatterplot of the 10,000 PSA samples of costs and QALYs. SoC, standard of care.

Appendix 10 Drug price discount scenarios
Intervention | Total costs (£) | Total QALYs | Incremental costs (£) | Incremental QALYs | ICER |
---|---|---|---|---|---|
Biologic discount: 25% | |||||
Standard of care | 190,628 | 15.96 | – | – | – |
PredictSURE-IBD | 196,974 | 15.85 | 6346 | –0.10 | Dominated |
Biologic discount: 50% | |||||
Standard of care | 173,399 | 15.96 | – | – | – |
PredictSURE-IBD | 178,454 | 15.85 | 5055 | –0.10 | Dominated |
Biologic discount: 75% | |||||
Standard of care | 156,169 | 15.96 | – | – | – |
PredictSURE-IBD | 159,935 | 15.85 | 3765 | –0.10 | Dominated |
Anti-TNF discount: 25% | |||||
Standard of care | 199,028 | 15.96 | – | – | – |
PredictSURE-IBD | 206,898 | 15.85 | 7870 | –0.10 | Dominated |
Anti-TNF discount: 50% | |||||
Standard of care | 190,198 | 15.96 | – | – | – |
PredictSURE-IBD | 198,302 | 15.85 | 8104 | –0.10 | Dominated |
Anti-TNF discount: 75% | |||||
Standard of care | 181,369 | 15.96 | – | – | – |
PredictSURE-IBD | 189,707 | 15.85 | 8338 | –0.10 | Dominated |
Biologic and anti-TNF discount: 25% | |||||
Standard of care | 181,798 | 15.96 | – | – | – |
PredictSURE-IBD | 188,378 | 15.85 | 6580 | –0.10 | Dominated |
Biologic and anti-TNF discount: 50% | |||||
Standard of care | 155,740 | 15.96 | – | – | – |
PredictSURE-IBD | 161,263 | 15.85 | 5523 | –0.10 | Dominated |
Biologic and anti-TNF discount: 75% | |||||
Standard of care | 129,682 | 15.96 | – | – | – |
PredictSURE-IBD | 134,149 | 15.85 | 4467 | –0.10 | Dominated |
Steps in the model | Base case | Scenario a | Scenario b |
---|---|---|---|
Anti-TNF (TD) vs. IM (SU) | Relative benefit for anti-TNF (D’Haens et al.35) | Relative benefit for anti-TNF (D’Haens et al.35) | Relative benefit for anti-TNF (D’Haens et al.35) |
Anti-TNF (TD) vs. anti-TNF (SU) | No relative benefit |
Relative benefit for TD:a ai) Half of D’Haens et al. 35 aii) Same as D’Haens et al. 35 |
Relative benefit for TD:a bi) Half of D’Haens et al. 35 bii) Same as D’Haens et al. 35 |
Second- and third-line biologic (TD) vs. second- and third-line biologic (SU) | No relative benefit |
Relative benefit for TD:a ai) Half of D’Haens et al. 35 aii) Same as D’Haens et al. 35 |
No relative benefit |
Second- and third-line biologic (TD) vs. anti-TNF (TD) | No relative benefit | No relative benefit |
Relative benefit for anti-TNF:a bi) Half of D’Haens et al. 35 bii) Same as D’Haens et al. 35 |
Second- and third-line biologic (SU) vs. anti-TNF (SU) | No relative benefit | No relative benefit | No relative benefit |
Glossary
- Accuracy
- The ability of a test to identify positive and negative cases correctly. Calculated as the proportion of true positives and true negatives in all evaluated cases.
- Cost-effectiveness analysis
- An economic methodology that converts effects into health terms and describes the costs per additional health gain.
- False negative
- An incorrect negative test result for an affected individual.
- False positive
- An incorrect positive test result for an unaffected individual.
- Incremental cost-effectiveness ratio
- The difference in the mean costs of two interventions in the population of interest divided by the difference in the mean outcomes in the population of interest.
- Markov model
- An analytical method particularly suited to modelling repeated events or the progression of a chronic disease over time.
- Meta-analysis
- A statistical technique used to combine the results of two or more studies and obtain a combined estimate of effect.
- Negative predictive value
- The probability that people with a negative test result truly do not have the target condition.
- Opportunity costs
- The cost of forgone outcomes that could have been achieved through alternative investments.
- Positive predictive value
- The probability that people with a positive test result truly have the target condition.
- Probabilistic sensitivity analysis
- A method of quantifying uncertainty in a mathematical model, such as a cost-effectiveness model.
- Reference standard
- The best currently available test against which the index test is compared.
- Sensitivity
- The proportion of people with the target condition who test positive.
- Specificity
- The proportion of people without the target condition who test negative.
- True negative
- A correct negative test result for an unaffected individual.
- True positive
- A correct positive test result for an affected individual.
List of abbreviations
- 5-ASA
- 5-aminosalicylate
- ACCA
- anti-chitobioside antibodies
- AIC
- Akaike information criterion
- ALCA
- anti-laminaribioside antibodies
- AMCA
- anti-mannobioside antibodies
- anti-C
- anti-chitin antibody
- anti-L
- anti-laminarin antibody
- ASCA
- anti-Saccharomyces cerevisiae antibodies
- BIC
- Bayesian information criterion
- CD
- Crohn’s disease
- CD8+
- cluster of differentiation 8
- CDAI
- Crohn’s Disease Activity Index
- cDNA
- complementary deoxyribonucleic acid
- CDSR
- Cochrane Database of Systematic Reviews
- CENTRAL
- Cochrane Central Register of Controlled Trials
- CI
- confidence interval
- CRD
- Centre for Reviews and Dissemination
- DAP
- Diagnostic Assessment Programme
- DAR
- Diagnostics Assessment Review
- DNA
- deoxyribonucleic acid
- EAG
- External Assessment Group
- ELISA
- enzyme-linked immunosorbent assay
- EQ-5D
- EuroQol-5 Dimensions
- EQ-5D-3L
- EuroQol-5 Dimensions, three-level version
- EQ-5D-5L
- EuroQol-5 Dimensions, five-level version
- gASCA
- anti-Saccharomyces cerevisiae antibodies
- HBI
- Harvey–Bradshaw Index
- HR
- hazard ratio
- IBD
- inflammatory bowel disease
- IBDX
- Crohn’s disease Prognosis Test
- ICER
- incremental cost-effectiveness ratio
- IM
- immunomodulator
- IPD
- individual patient data
- MeSH
- medical subject heading
- mRNA
- messenger ribonucleic acid
- NICE
- National Institute for Health and Care Excellence
- OR
- odds ratio
- PRISMA
- Preferred Reporting Items for Systematic Reviews and Meta-Analyses
- PROFILE
- PRedicting Outcomes For Crohn’s dIsease using a moLecular biomarkEr
- PSA
- probabilistic sensitivity analysis
- QALY
- quality-adjusted life-year
- qPCR
- quantitative polymerase chain reaction
- QUIPS
- Quality In Prognosis Studies
- RCT
- randomised controlled trial
- RT-qPCR
- reverse transcription-quantitative polymerase chain reaction
- SLR
- systematic literature review
- SU
- step-up
- TA
- technology appraisal
- TD
- top-down
- TNF
- tumour necrosis factor
- TTE
- time to treatment escalation
- TTS
- time to surgery
This monograph is based on the Diagnostic Assessment Report produced for NICE. The full report contained a considerable number of data that were deemed confidential and were used by the Diagnostics Advisory Committee at NICE in their deliberations. The full version of the report with the confidential information removed is available on the NICE website: www.nice.org.uk.
The present monograph presents as full a version of the report as is possible while retaining readability, but some sections, sentences, tables and figures have been removed. Readers should bear in mind that the discussion, conclusions and implications for practice and research are based on all the data considered in the original full NICE report.
Notes
-
The PRISMA-DTA checklist and PRISMA-DTA for abstracts checklist
-
Search strategies for electronic databases to retrieve records on studies evaluating prognostic accuracy and the impact of using the tools on the management of CD
-
List of full-text publications screened but subsequently excluded (with reasons for exclusion) from the review
Supplementary material can be found on the NIHR Journals Library report page (https://doi.org/10.3310/hta25230).
Supplementary material has been provided by the authors to support the report and any files provided at submission will have been seen by peer reviewers, but not extensively reviewed. Any supplementary material provided at a later stage in the process may not have been peer reviewed.