Notes
Article history
The research reported in this issue of the journal was funded by PGfAR as project number RP-PG-1209-10099. The contractual start date was in November 2011. The final report began editorial review in February 2018 and was accepted for publication in September 2020. As the funder, the PGfAR programme agreed the research questions and study designs in advance with the investigators. The authors have been wholly responsible for all data collection, analysis and interpretation, and for writing up their work. The PGfAR editors and production house have tried to ensure the accuracy of the authors’ report and would like to thank the reviewers for their constructive comments on the final report document. However, they do not accept liability for damages or losses arising from material published in this report.
Permissions
Copyright statement
Copyright © 2022 Sheikh et al. This work was produced by Sheikh et al. under the terms of a commissioning contract issued by the Secretary of State for Health and Social Care. This is an Open Access publication distributed under the terms of the Creative Commons Attribution CC BY 4.0 licence, which permits unrestricted use, distribution, reproduction and adaption in any medium and for any purpose provided that it is properly attributed. See: https://creativecommons.org/licenses/by/4.0/. For attribution the title, original author(s), the publication source – NIHR Journals Library, and the DOI of the publication must be cited.
2022 Sheikh et al.
SYNOPSIS
Summary of changes to the research programme
The main change from the proposal was that we were unable to employ the stepped-wedge design that was originally planned because of several implementation delays within the hospital sites; instead, we settled on a more pragmatic pre- and post-implementation design. The delays also impaired our ability to assess the longer-term consequences of ePrescribing systems because we were unable to follow developments up over sufficient time periods that were required to understand the longer-term consequences of ePrescribing systems.
One of our original aims was to establish whether or not there is a difference between non-alerting computerised physician order entry (CPOE) systems and more advanced alerting clinical decision support (CDS) systems. However, this proved impossible because both systems implemented some level of CDS within their ePrescribing system. However, given that we measured a fixed set of medication errors, we were able to highlight the different levels of CDS and the impact that this may have had on medication error rates.
Although the original aim of the programme was primarily directed at supporting professionals to implement ePrescribing systems in hospitals, patient and public involvement (PPI) members argued for directly including patients’ views on hospital ePrescribing systems to inform the programme. Therefore, a patient study was introduced into work package (WP)1 that was guided by patient engagement work.
For the health economic work in WP3, an additional step to calculating health gain was used that was not outlined in the original proposal. The health gain was calculated [in quality-adjusted life-years (QALYs)] directly for particular errors chosen by a nominal group method to represent the greatest-impact errors in terms of harm and frequency.
The health economic work was also adapted because we were not able to obtain cost data from the study sites, as hospitals were generally unwilling to provide such information. This resulted in the use of the ‘headroom’ approach, which asks what the maximum price a decision-maker should be willing to pay for a technology on the basis of overall expected benefits. This approach is desirable in this context given that it allows decision-makers to compare the prices that they face with the evidence on effectiveness to make an informed decision.
Development
Prescribing errors are common, costly and often result in considerable harm to patients. 1–3 Work from a handful of predominantly US-based centres of excellence suggests that the risk of patients being inadvertently harmed by erroneous prescribing may be greatly reduced by the introduction of electronic prescribing (ePrescribing) systems. 4–6 Their development, procurement and implementation is, therefore, currently being pursued internationally. 7
Current ePrescribing applications vary significantly in functionality and sophistication. These, in essence, range from basic computerised data entry applications (also sometimes known as CPOE or non-alerting systems) to more sophisticated systems offering advanced CDS systems functionality that generate prescribing and medication administration alerts. 7 CDS systems are active knowledge systems that use real-time patient-specific information to generate individualised prescribing advice. These more sophisticated systems tend to be integrated with existing local electronic health records (EHRs) to produce alerts (e.g. warnings and reminders) tailored to the individual case at hand, for example a prescribing alert suggesting a dose change when a patient’s renal function is deteriorating.
ePrescribing systems are now well established in UK primary care. 7,8 Within secondary care, however, the implementation of ePrescribing systems has been much slower, this in part reflecting the fact that hospital records have until recently tended to be paper based. The widespread adoption of ePrescribing systems had until recently been achieved in very few UK academic hospitals (e.g. the University Hospitals Birmingham NHS Foundation Trust). More recently, there has been an acceleration in the adoption of ePrescribing systems in hospitals, but implementation remains largely unco-ordinated nationally.
Available systems range from the home-grown extensively tailored applications that have been developed over a number of years (as is the case in University Hospitals Birmingham NHS Foundation Trust where the system has been developed for over ≈ 15 years) to the expanding array of ‘commercial off-the-shelf’ (COTS) systems. Although home-grown systems tend to be more easily adopted by hospitals (this reflects the strong sense of local clinical ownership), extensive system customisation over time means that these are not easily transferable to other sites. In addition, very few hospitals have the necessary expertise or budgets to develop their own systems in-house. Moreover, over time, home-grown solutions become very expensive to maintain; as a result, adopters increasingly choose packaged solutions for reasons of cost and dependability. Trusts, therefore, need to decide whether to persist with paper-based prescribing or to move to electronic prescribing. If they choose the latter, as indeed many are, a key decision is to implement a stand-alone basic ePrescribing system or a more integrated packaged solution that is part of a full EHR solution, which often offer additional CDS functionality. This decision is likely to be shaped by a range of factors, including the ease of implementation, training implications, the time and cost investment necessary, and the anticipated benefits, particularly in relation to improving prescribing safety.
Reducing the risk of iatrogenic harm is now firmly established as a priority area throughout much of the economically developed world, including the UK. Given that medication-related harm is associated with a substantial proportion of this potentially avoidable morbidity and mortality, there is now a considerable focus on improving prescribing safety. 9,10
Although real-time prescribing support and electronic alerts/prompts have under some circumstances demonstrated benefit in improving clinicians’ prescribing behaviour and/or reducing error rates, there remains a small, but nonetheless important, risk that the introduction of these systems may also have serious adverse impacts on patient outcomes. 11–15 They can, thus, introduce new areas of clinical risk and unexpected threats to patient safety. Koppel et al. ,13 for example, reported how fragmented CPOE displays prevented a coherent view of patients’ medication and how the separation of functions contributed to accidental double dosing. Another study11 has highlighted how systems’ implementation-generated errors were associated with the process of entering and retrieving information, manifesting as errors in communication and co-ordination processes. Similarly, we have found that many CDS systems can produce clinically spurious or irrelevant alerts that frustrate end users and result in these alerts commonly being over-ridden or ignored. 12 More recent work has highlighted the potentially serious treatment delays that can inadvertently be associated with the introduction of ‘hard stops’ (designed to prevent over-riding of alerts) in the context of potentially serious prescribing errors. 14,15
ePrescribing systems are, therefore, not without associated risks. Unintended consequences of health information technologies (HITs) are strongly linked to poor implementation and adoption strategies. This has been demonstrated not only in the UK, but also in many other parts of the world. 16,17 There is then a need to understand ways of minimising/mitigating the risks associated with the introduction of potentially transformative HITs, such as ePrescribing systems.
We set out to undertake a mixed-methods programme of research involving NHS trusts that were in the process of putting in new systems to study the implementation, adoption, effectiveness and cost-effectiveness of these systems. We also sought to study trusts that had already implemented ePrescribing systems to study more embedded use. Our research team comprised national and international medical and pharmacy experts in ePrescribing/HITs, social scientists, organisational management experts, health economists and PPI experts. Our key aims were to describe the procurement, implementation, adoption and maintenance of basic (i.e. CPOE functionality) and more advanced (i.e. also including CDS) ePrescribing systems; to estimate their effectiveness and cost-effectiveness; and to develop best practice implications for practice and a toolkit for their successful integration into NHS hospitals.
The research was organised into four complementary WPs, namely:
-
WP1 – procurement, implementation, adoption and connectivity
-
WP2 – assessing impact on prescribing safety
-
WP3 – health economics and a value of investment analysis
-
WP4 – integration across WPs to develop a toolkit for the NHS incorporating implications for practice.
These WPs are described in detail in the following sections. Figure 1 illustrates the inter-relationships between the WPs.
FIGURE 1.
Overview of the WP relationships. Reproduced with permission, personal communication, 2021.
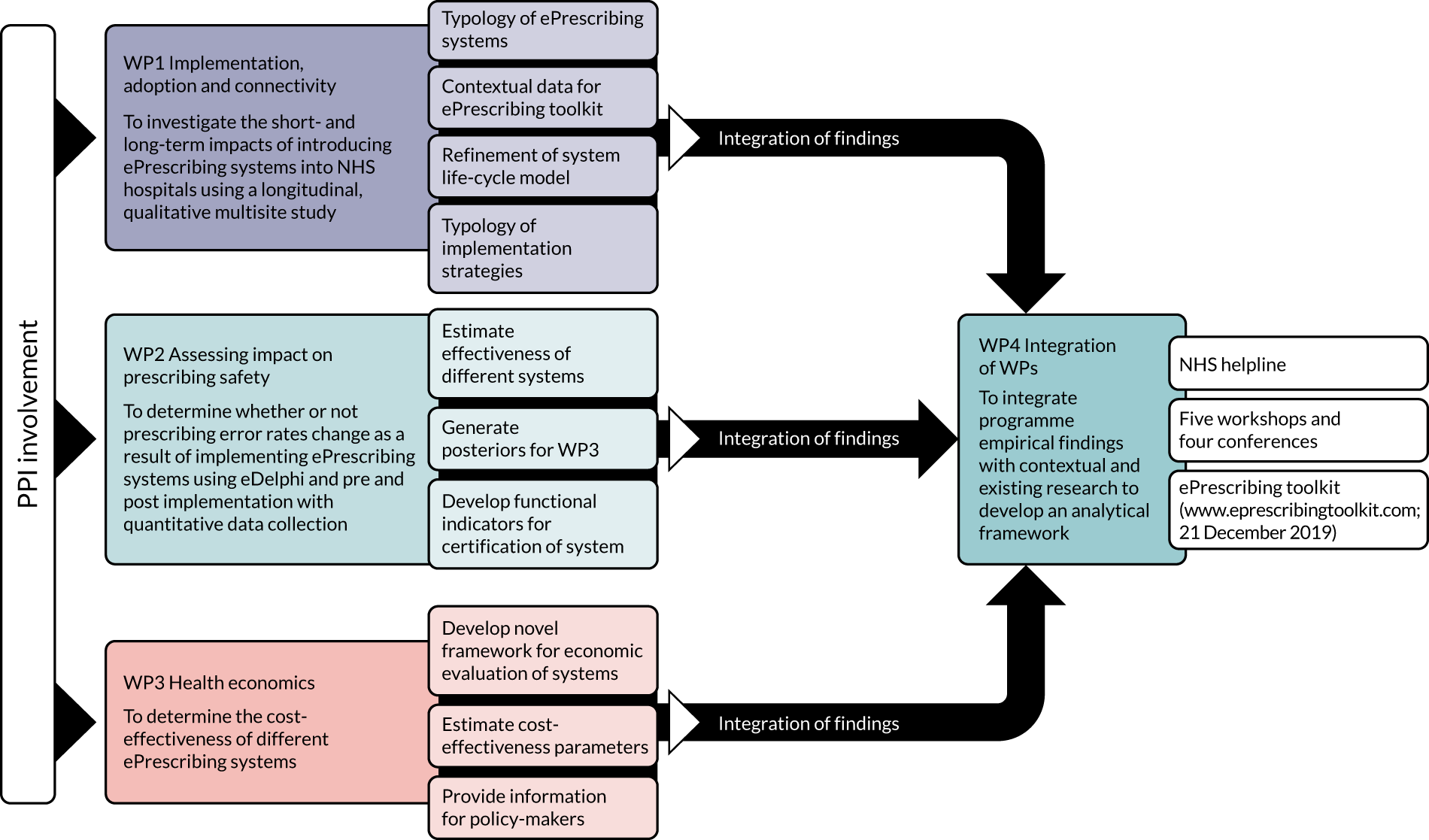
Work package 1: implementation, adoption and connectivity
Background
The implementation of any new HIT within a health-care system is a complex phenomenon. Previous national schemes, such as the National Programme for Information Technology, demonstrated the considerable complexities of the large-scale adoption of enterprise-wide systems. Some of these difficulties lay in having a sufficient information technology (IT) infrastructure in terms of hardware and wireless connectivity; however, there are many other challenges in implementing systems in a health-care organisation. Some of these challenges are common to all IT system implementations, whereas others are more specific to the hospital environment. Organisations need to be ready in terms of both their infrastructure and the people working within the system (e.g. health-care practitioners and managers) for the successful implementation and adoption of systems. The adoption of an ePrescribing system, therefore, needs to recognise the potential for change not only in the technical aspects of the organisational structure and processes, but also in the complex interactions between the people and the new technology in the workplace.
Good communication and information-sharing within a health-care organisation and across organisational boundaries are critical for continuity of patient care. The Department of Health and Social Care (DHSC) document Discharge from Hospital: Pathway, Process and Practice18 called for effective communication between primary, secondary and social care. Research has indicated the impact of communication breakdown on prescribing and on the monitoring of patients following discharge from hospital,19 and a DHSC-commissioned report20 highlighted how the use of ePrescribing systems in hospitals can give staff quicker access to more reliable information and, thus, has the potential to improve patient safety. General practitioners (GPs) may also require timely information from hospitals to support safer care after a patient has been discharged from hospital. 21 This calls for an extension of complex information systems across hospital settings and beyond.
The consequences of adopting new information systems depend on multiple factors. As well as the design and configuration of the computer system, the implementation strategy, training, systems’ maintenance, work reorganisation and development of new work practices are all influential in systems’ deployment, consequences and optimisation. Given the complex interactions between these various sociotechnical factors (the changing inter-relationships between technology and human/organisational factors), introducing new systems into hospitals may result in both positive and negative consequences. For example, in a hospital the workload of individual staff members, team structures, workflows, communication processes (within and beyond the organisation) and patients’ safety and care outcomes may all be influenced.
We conducted longitudinal case studies to explore the evolution of system implementation and use over time, as well as to identify factors determining the short- and long-term impacts of, and transformations brought about by, these systems. The factors explored included the organisational learning achieved, the identifiable impacts on everyday working practices, the issues relating to communication across organisational boundaries, and the safety and risk arising from systems’ utilisation.
This evolutionary perspective also aimed to throw light on the longer-term processes of capacity development, highlighting the extent to which hospitals and trusts have managed to acquire and retain implementation experience and change management capacity (sometimes described as organisational readiness or ‘maturity’), and on an additional dimension that has hitherto received inadequate attention: the extent of knowledge flow, shared understanding and institutional links between suppliers and adopters.
In WP1, we also sought a better understanding of how different hospital ePrescribing systems influence communication and local connectivity both within the hospital and in the wider health community. Here, the focus was particularly on the perspectives of those responsible for prescribing and administering medication to investigate the consequences of the hospital ePrescribing systems for information flow, as well as on patients and their perspectives. 22 We also sought to understand the implications for the prescribing education of health-care professionals. 23
A national digital maturity assessment has shown that NHS hospitals have a very different rate of adoption of inpatient ePrescribing versus discharge ePrescribing (digital maturity) systems. There was only one supplier (JAC Computer Services Limited, Essex, UK) with more than 10 ePrescribing system roll outs. There was also a growing number of Cerner Millennium (Cerner Limited, London UK) implementations in hospitals. More than 10 other systems were also being procured and implemented by NHS England’s hospitals. Therefore, we also sought to identify specific lessons surrounding the selection and utilisation of different kinds of systems and functionalities in different work settings. To achieve this, we examined the product life cycle of the systems, in which packages emerge from development settings and are further refined through implementation in different settings.
Research aims
Our aims were to investigate the shorter- and longer-term impacts of introducing ePrescribing systems into NHS hospitals by:
-
describing the planned and actual implementation and adoption strategies
-
understanding key stakeholders’ perspectives by exploring individuals’ expectations, experiences, practices and perceived needs over time.
During the course of this work, it became clear that addressing the main aims (as described above) required us to investigate how these issues are patterned by the broader context, including patients’ perspectives on ePrescribing, the level of ePrescribing maturity within the NHS and the evolving market of COTS ePrescribing applications and its effects on their uptake and implementation. Therefore, two additional research aims were articulated, namely to investigate the:
-
evolving market of COTS ePrescribing applications and its effects on their uptake and implementation in English hospitals (which hereafter we refer to as the ‘supplier study’)
-
significance of ePrescribing from patients’ perspectives (which hereafter we refer to as the ‘patient study’).
Methods of data collection and analysis
Parts of this text have been reproduced with permission from Mozaffar H, Williams R, Cresswell K, Morrison Z, Bates DW, Sheikh A, The evolution of the market for commercial computerized physician order entry and computerized decision support systems for prescribing, J Am Med Inform Assoc, 2016 vol. 23, pp. 349–55,24 by permission of Oxford University Press.
Analytical approach and conceptual framework
We used a longitudinal, qualitative, multisite case study to investigate the sociotechnical aspects of the implementation and adoption of ePrescribing systems in English hospitals. 25,26 In so doing, we drew insights from current scholarship in information systems and related work in science and technology studies, which are helpful for understanding the challenges of current HIT implementation. Foremost here is the work on information infrastructures (IIs). 27 This concept draws attention to the emergence of (computer-based) ‘systems of systems’ that support an increasingly wide range of tasks across an ever-more extensive base of users. 28 Whereas discrete systems can be analysed in terms of a cycle from design to implementation/use, IIs emerge through multiple cycles of system design and implementation (encompassing the initial design and its further extension and evolution). New conceptual frameworks have emerged to capture the distinctive features of IIs and their implications for policy and practice. 29,30 These IIs may call for different development and implementation strategies, for example to prevent lock-in around existing configurations and to provide flexibility to allow new functionality to be taken on board.
Despite that ePrescribing systems were originally conceived as a discrete pharmacy technology, it has become the occasion to integrate various other kinds of digital information (e.g. laboratory test results) at the point of care and for sharing this information across the care pathway. ePrescribing in the UK has, thus, served as a stepping stone for developing hospital-wide IIs that directly support both diagnosis and care delivery.
The focus on evolving IIs highlighted the need to go beyond single-site snapshot studies of implementation to undertake longitudinal studies of a system’s evolution. 31 One finding from this work was the need to rethink engagement strategies, which in management guidelines are geared towards episodic implementations of discrete technologies to address the extended character of infrastructural change. 32 This focus on evolving IIs also suggested the need to examine the development of the component technologies in tandem with their implementation. Here, the BoAP28 perspective offered an analytical template for developing longitudinal insights into how outcomes were shaped by prior sets of decisions and learning within and between communities of adopters and suppliers of ePrescribing products. 30,31,33 In analysing the findings, we also drew on other pertinent theories, including the systems implementation life-cycle model, theory of workarounds and software product cycle. 34
The initially proposed research design proved well-equipped to address the original aims. The work was supplemented by complementary fieldwork, using similar methods to provide adequate data for (1) the supplier study and (2) the patient study. Appendix 1 shows an outline of the original and complementary studies.
Ethics approval
Our programme of work was reviewed by the NHS Research Ethics Service (NRES) Committee London – City and East Research Ethics Committee and was classed as a service evaluation. We, therefore, obtained Institutional Review Board approval from The University of Edinburgh, Edinburgh. Participating hospitals provided organisational approvals. All places, names and organisations have been anonymised to ensure confidentiality. Individual participants provided written informed consent for participation.
Sampling and recruitment
The protracted and turbulent process of ePrescribing implementation, with frequent delays that were occasionally extended and sometimes resulted in systems being abandoned, required us to sample from a number of potential research sites. We used purposeful sampling to select six case study sites that were planning to implement or had recently implemented two different types of ePrescribing systems. 35,36 Despite that the first generation of ePrescribing systems were bespoke systems, ePrescribing today is procured almost exclusively as COTS-packaged applications within NHS England. A similar trend is seen in other parts of the world. 37–40 Appendix 2 shows a description of each case.
We contacted the director of pharmacy or the lead pharmacist for ePrescribing, and used purposive and snowball sampling to recruit a diverse range of stakeholders for interviews. 35 To address the potential risks of bias caused by snowball sampling, after our first contact with the director of pharmacy or lead pharmacist for ePrescribing we used respondent-driven sampling to maximise the chances of recruiting a maximum variation sample41 to include a range of different respondents (e.g. varying levels of seniority, different professions and different viewpoints). Our sample consisted of implementation team members and users of the system, in particular those users who were involved in implementation.
Data collection
Parts of this section have been reproduced with permission from Mozaffar et al. 42 This is an Open Access article distributed in accordance with the terms of the Creative Commons Attribution (CC BY 4.0) license, which permits others to distribute, remix, adapt and build upon this work, for commercial use, provided the original work is properly cited. See: https://creativecommons.org/licenses/by/4.0/. The text below includes minor additions and formatting changes to the original text.
We used semistructured interviews as the main method of data collection. We supplemented these data with data collected from expert round-table discussions. This was carried out to take into account the perspective of both adopters and suppliers of technology. 30,31 We also observed participants in hospital wards to observe the use of ePrescribing systems in situ. By asking prescribers to talk through each step as they navigated the system, this method contributed to our understanding of how these systems are incorporated into processes of care and hospital workflows. Furthermore, we collected relevant documents, including implementation business cases, roll-out plans and risk logs. Combining different methods enabled us to triangulate the data sources to validate emerging findings. 43
In total, across the six case study sites we conducted 242 interviews and 32.5 hours of observations and collected 55 documents. Interviews were conducted at up to three time points in each organisation. In the three hospitals that implemented the system during our study (i.e. sites C, J and K), we conducted one set of interviews prior to implementation, one set just after the roll out and one set approximately 1 year post implementation. In one site that did not go live during our study (site E), we conducted three rounds of data collection at approximately 1-year intervals. However, given that the system did not go live during our study (because of delays), all three rounds of data collection were in fact pre implementation. In the two hospitals that already had embedded systems (i.e. sites A and D), we conducted two sets of interviews with an approximately 18-month gap between the two rounds. Appendix 3 provides a summary of data collection from each site; the protocols, participant information leaflets, consent forms and interview guides can be found in Report Supplementary Material 1.
The interviews consisted of open-ended questions about the procurement, implementation process and challenges, and the use of the system. Various topics (e.g. project timing and delays, integration and interfacing, and user engagement) emerged spontaneously during the discussions and were followed up as themes. The interview guides (see Report Supplementary Material 1) were, therefore, tailored to the roles and organisations of individuals. Each interview took between 15 minutes and 2 hours. Data were collected between December 2011 and March 2016. We complemented the interview data by observing strategic implementation meetings and system use. In addition, we used data collected in WP4 (round-table discussions and workshops).
With written consent from the participants, interview and round-table meeting data were digitally audio-recorded and transcribed verbatim by a professional transcriber. The researchers also recorded field notes for each meeting and interview.
Data analysis
Data were thematically analysed, initially within cases by lead researchers, to explore the local contexts and changes over time by triangulating different data sources. 44 For each output from WP1, we drew on our previous work in this area as a deductive coding framework, where possible. In addition, we inductively identified emerging themes surrounding the point of focus for each paper (e.g. engagement, delay, integration, safety, workarounds), which served as an analytical lens to examine our data. 44 Themes were developed based on frequency of occurrence across sites and salience among different stakeholder groups. Negative cases, that is those that did not fit within the narrative, were explored in the most detail.
Findings across sites were then compared in analysis meetings of the research team. This involved discussing the commonalities and differences across sites, as well as exploring potential underlying explanations for differences and remaining tensions. Although we observed subtle differences across case study sites, our general analytic findings were comparable, so consensus was achieved through aggregating findings at higher analytic levels.
The supplier study: data collection and analysis
Parts of this section have been reproduced with permission from Mozaffar et al. 37 This is an Open Access article distributed in accordance with the terms of the Creative Commons Attribution (CC BY 4.0) license, which permits others to distribute, remix, adapt and build upon this work, for commercial use, provided the original work is properly cited. See: https://creativecommons.org/licenses/by/4.0/. The text below includes minor additions and formatting changes to the original text.
For the supplier study, our data collection methods consisted of semistructured interviews, ethnographic observation and collection of online documents. Data generation and analysis for this part of the study were performed concurrently in four stages, as described below.
First, we conducted interviews with a network of recognised experts from NHS professional domains, with the purpose of refining a baseline definition for the inclusion of applications as ePrescribing systems. This network consisted of members of the NHS who had closely worked with and studied ePrescribing systems in England. In these interviews, we asked the interviewees about what should be considered as ePrescribing systems.
In the second stage of data collection, we collected documents that were mainly from online sources and health-care conferences. The conferences were either those organised by our research team or those attended by the programme team. Data sources from these conferences included suppliers’ commercial materials, presentation slides, reports and papers publicly distributed at the conferences. These data enabled us to form a provisional picture of the market, which was used as an initiating point for the document search process. In the document search process, we used the search criteria to find any online information available on suppliers of ePrescribing systems in England. This resulted in discovering four main sources of online documents for further examination: suppliers’ commercial websites, NHS websites (including NHS hospitals and NHS Connecting for Health), academic journals and online media (particularly E-Health Insider). These documents were analysed further to gather data on both the state and the progress of adoption of systems in English hospitals. The results were presented as a ‘taxonomy of ePrescribing systems in the UK’. 37
Subsequently in stage three, we performed qualitative interviews to attempt to identify any further data sources, to obtain a ‘respondent validation’ check43 and to evaluate the evolution of the market. We conducted interviews with suppliers and adopter hospitals. In this regard, we carried out a ‘typical case sampling’,35 in which we contacted various suppliers and user hospitals that were found in stage two. Our goal was to interview at least one supplier or user hospital in each of the categories to test our findings about the placement of the system in the defined category and, if necessary, to refine the categories. The interviews were open and semistructured, with the interview guide focusing on four main points: (1) the current status and development trajectory of ePrescribing systems in NHS England; (2) strategies in design, development and adaptation; (3) problems faced during implementation and their possible causes; and (4) the vendor–user relationship throughout the ePrescribing life cycle. The interview guides were tailored to the roles of individuals and were further refined throughout the research based on the findings of prior interviews. We interviewed 11 individuals from 10 organisations (four vendors and six adopter hospitals) and undertook 21 hours of observation of the user group and vendor events. The duration of the interviews ranged from 45 minutes to 2 hours.
In the fourth stage, we observed the user group meetings of two vendors to provide wider context and aid the interpretation of our data. This offered particular opportunities to observe directly how user requirements and concerns were articulated through the user group, and how vendors responded to these. Data from the user group meetings consisted of researcher field notes around three main areas: (1) the technological contents of the discussion, (2) supplier–user relationships and (3) decisions being taken. In addition, we used data collected in WP4 (round-table discussions and workshops) to triangulate and support our findings.
Data were collected from October 2012 to October 2014. The interviews were digitally audio-recorded, transcribed verbatim and analysed using NVivo 10 (QSR International, Warrington, UK).
The patient study: data collection and analysis
Parts of this section have been reproduced with permission from Lee et al. 45 This is an Open Access article distributed in accordance with the terms of the Creative Commons Attribution (CC BY 4.0) license, which permits others to distribute, remix, adapt and build upon this work, for commercial use, provided the original work is properly cited. See: https://creativecommons.org/licenses/by/4.0/. The text below includes minor additions and formatting changes to the original text.
The patient study posed particular methodological challenges. This is because it is difficult to survey patients, who have mainly short-term and sporadic hospital stays, about the adoption and outcomes of long-term technological changes. Interviews were used to collect data for this part of the study. We conducted expert interviews with patient organisations, as well as interviews of inpatients on a renal ward in one of the hospitals studied in the programme. Data were collected in two stages: pre implementation and post implementation.
Ethics approval for the patient study was given by NRES Committee London – London Bridge (reference 13/LO/1202).
Two categories of participants were recruited for the study to provide both breadth and depth of data. For category one, we drew up a sample of 13 patient organisations whose expertise could be used to inform the findings from the interviews with renal patients. This included groups representing renal patients and other conditions from which these patients may concurrently suffer. Eight groups/organisations took part: Diabetes UK (London, UK), British Heart Foundation (London, UK), Anticoagulation Europe (Kent, UK), The Carers’ Trust (London, UK), Neurological Alliance (Watford, UK), Lifeblood (now Thrombosis UK, Llanwrda, UK), National Kidney Federation (Worksop, UK), National Childbirth Trust (London, UK) and Healthcare Quality Improvement Partnership (London, UK). Ten representatives with sufficient expert perspectives on the relevant questions from across the organisations were interviewed: medical directors (n = 2), head of policy (n = 1), head of advocacy (n = 1), senior policy adviser (n = 1), patient network members (n = 2), project development managers (n = 2) and a trustee (n = 1).
For category 2, individual patients were self-selected following a verbal invitation from nursing staff on the renal ward of a large urban hospital in England, both before and after the implementation of an ePrescribing system. This was a specialist renal ward in which patients received care for renal problems. Therefore, the main reason for the patients’ presence on the ward was to receive treatment for renal conditions, although this did not preclude continuation of medication for other existing long-term health issues (e.g. diabetes). Only inpatients were included. A total of 14 patients were invited to participate in the study, of whom 11 were interviewed.
Interview data were collected between January 2014 and February 2015. The interviews were digitally audio-recorded with the participant’s consent, transcribed, fully anonymised, coded by key areas of interest, and then analysed in NVivo 10 to facilitate a systematic and integrated approach.
Key findings
Parts of this text have been reproduced with permission from Mozaffar et al. 46 This article is distributed under the terms of the Creative Commons Attribution 4.0 International License (https://creativecommons.org/licenses/by/4.0/), which permits unrestricted use, distribution, and reproduction in any medium, provided you give appropriate credit to the original author(s) and the source, provide a link to the Creative Commons license, and indicate if changes were made. The Creative Commons Public Domain Dedication waiver (http://creativecommons.org/publicdomain/zero/1.0/) applies to the data made available in this article, unless otherwise stated. The text below includes minor additions and formatting changes to the original text.
Parts of this text have also been reproduced with permission form Mozaffar et al. 47 with permission from BMJ Publishing Group Ltd.
The key deliverables for WP1 were to:
-
develop a typology of ePrescribing systems
-
provide contextual data for the provisional ePrescribing toolkit
-
refine the system life-cycle model for ePrescribing systems
-
develop a typology of implementation strategies.
Early and widespread dissemination of the findings of this WP (together with WP4) was achieved by a strategically managed programme of publication, including 32 articles in peer-refereed journals and two book chapters. These papers are listed in the Acknowledgements. Lay summaries of these outputs are detailed in Appendix 27. These journal articles, together with the information presented in the ePrescribing toolkit, contribute towards achieving WP1 deliverables. Table 1 summarises the project achievements in relation to these key deliverables.
Key deliverable | Progress |
---|---|
Typology of ePrescribing systems | Two papers24,37 have been published to present the typology of systems and their evolution over time:
|
Provisional ePrescribing toolkit | We have collected and produced materials for WP4 to support the development of the online implementation toolkit, which was first launched in December 2013. Details of the toolkit can be found in Work package 4. We have also published two papers48,49 around the findings about the development and use of the toolkit:
|
Refinement of the system life-cycle model | The refined life-cycle model is available on the ePrescribing toolkit website: www.eprescribingtoolkit.com/ (accessed 11 August 2017). It is presented as a planner with details for the following steps: (1) conceptualisation, (2) project initiation, (3) functional specification, (4) system choice, (5) procurement/tendering, (6) drafting a business case, (7) contracting, (8) pre implementation, (9) implementation and (10) system optimisation. In addition, the following paper50 further explains the refined life-cycle model:
|
Typology of implementation strategies | There are a wide range of parameters and strategies involved in implementation strategies. Therefore, we have addressed these issues in several journal articles. These papers include:42,51,52
|
User engagement (explaining the strategies used to involve users from the early outset to implementation and beyond) –
|
In the remainder of this section, we organise our findings around six central themes that emerged from our data analyses. The first four themes focus on the implementation site, the hospital: (1) initiation and procurement, (2) implementation process, (3) organisational consequences and (4) medium- to long-term benefits realisation and system optimisation. The remaining two themes refer to findings taking different perspectives: (5) system knowledge exchange mechanisms in the HIT sector and (6) patient view of ePrescribing use.
Initiation and procurement
Varying definitions
There is no uniform conception of ePrescribing systems. This is reflected in the terminology used across the world: for instance ePrescribing/HEPMA (hospital ePrescribing and medication administration) in the UK and CPOE/CDS in the USA. The fluctuation in the definition is partly a result of the various origins of ePrescribing systems, which have grown out of different systems (e.g. pharmacy stock control, insurance/accounting systems and scheduling systems). There is a wide range of products currently being developed by different national and international suppliers, and the low uptake of these products by English hospitals indicates that the English ePrescribing market is still in its infancy. This market is undergoing rapid cycles of change, with respect to both the number of suppliers and the diversity of offerings. 24,37
In hospitals, the implementation of ePrescribing systems provided an opportunity to integrate a number of previously separate information systems into a broader system of systems or II. Often, implementations of ePrescribing systems are initiated from inside pharmacies, but have implications influencing an ever growing array of health professionals across the hospital and beyond (with important links being made to primary care). Although conceived as discrete solutions, definitions of the technology and its usage are being reshaped as ePrescribing modules are being applied and linked to other applications in diverse clinical settings. This ongoing configuration of complex and, partially, immature systems contributes to rapidly evolving IIs. 37
Types of ePrescribing systems available in the UK
Parts of this section are reproduced with permission from Mozaffar et al. 37 This is an Open Access article distributed in accordance with the terms of the Creative Commons Attribution (CC BY 4.0) license, which permits others to distribute, remix, adapt and build upon this work, for commercial use, provided the original work is properly cited. See: https://creativecommons.org/licenses/by/4.0/. The text below includes minor additions and formatting changes to the original text.
ePrescribing systems can be distinguished according to ‘modes of supply’ (i.e. the way in which suppliers of technology make their products available to the market) and ‘forms of solutions’ (i.e. the position of the ePrescribing systems in relation to other hospital information systems). By analysing these modes, we have developed a typology of ePrescribing systems into two overarching categories, home-grown bespoke products and commercial packaged applications [commercial off the shelf (COTS)], with the latter category further divisible into four subcategories: stand-alone systems, modules within an integrated system, functionalities spread over several modules, and specialty systems. 37
The number of procurements and implementations of COTS is rapidly growing in England. Although bespoke systems promise the best match between functional capabilities and practical requirements of the respective local setting, the development of bespoke systems and their integration with other systems tends to be more time-consuming and requires more effort than packaged systems. Bespoke systems can be more costly because they require in-house development and maintenance teams. These costs rise as systems become more complex. This has led to a move away from bespoke developments to adopting packaged applications. 37,53
Users may seek to share these costs, for example by engaging in the joint development of a system. However, experience suggests that joint HIT programmes can run into even more acute difficulties. The accumulation of user requests without a system in place to align requirements (generification strategy) makes it hard to keep control over systems evolution. Development is likely to run into problems and releases are delayed, which also leads to concerns about sustainability and cost.
Integrated systems demand the highest upfront investment, but offer benefits such as synchronisation between hospital activities, integration of human resources and improved efficiency and productivity. Integrated systems allow the access to hospital-wide information available at any place in the hospital. By contrast, a lack of integration can lead to multiple data entry points, possibly resulting in problems, such as increased potential of error and greater operating costs. 37
Stand-alone applications tend to be cheaper to procure and quicker to implement. These reasons have led to implementation of stand-alone systems by a large number of hospitals. In this manner, these hospitals have perhaps opted to achieve short-term benefits while investing less in the product. Such stand-alone systems tend to be simpler to configure than integrated systems. However, achieving integration and interoperability between multiple stand-alone solutions and wider systems may be more costly and difficult to achieve following initial implementation. 37
ePrescribing products vary significantly in function and there is, to date, little evidence of the emergence of de facto standards or ‘dominant designs’ in the English market. 24 This diversity is a double-edged sword for hospitals that think about procuring ePrescribing systems. On the one hand, hospitals can choose from a variety of systems to find a solution that meets specific organisational requirements and financial constraints. However, on the other hand, the diverse range of options to choose from poses an enormous challenge. Besides the difficulty of selecting a system, legal arrangements between hospitals, vendors and third parties can also hold unanticipated challenges.
Procurement challenges
This work has underlined how procurement is a critical aspect of the process of technological deployment and adoption. Much attention has been given to the economies of scale and the sharing of expertise and knowledge through joint procurement. Our studies of an early attempt at joint procurement through the London Joint Procurement show that joint procurement may paradoxically make it difficult to fulfil the goals that the contract was intended to meet, undermining the claimed benefits of efficiency gains in terms of cost savings and better use of expertise. 54 In particular, this model appears to exacerbate usability issues and difficulties in the customisation process by making it unclear where leadership and control of the project lie. 41
Procurement contracts aim to specify details in advance of the exchange of goods or services to ensure smooth and manageable transactions. However, uncertainties involved in the implementation of ePrescribing systems result in fluctuations of user requirements as the implementation process unfolds. Consequently, evolving user requirements limit the scope for strict enforcement of procurement contracts. 54
Developing a business case for an ePrescribing system requires the consideration of a multitude of issues. For a hospital, this may represent a very considerable challenge. Close discussions with vendors could assist in this process, but this may be inhibited by the requirement in public procurement tendering processes for an arm’s-length relationship between supplier and adopter. 54 Joint contracts limited the opportunity and scope for individual hospitals to exercise influence to change the ePrescribing system according to their specific needs. Joint procurement contexts also entail reputational dependencies between hospitals. Failure in one site could have a negative impact on other members of the alliance, as well as the scale of projects associated with the joint procurement. It is this joint procurement model that leads to end-users’ frustration because they are denied the control of a customisable system, resulting in a perception that the supplier is incompetent and the system is weak. 54
There are significant issues surrounding the capacity to procure and optimise ePrescribing systems among UK hospitals. It may be helpful to strengthen informal and formal channels for collaboration and joint learning between providers and vendors at the site level and across communities of adopters.
Currently, there is a double immaturity in the market: one in relation to the conception of ePrescribing as a result of unco-ordinated and sometimes inconsistent user needs and the other with regard to the uneven growth of systems with differing modes of supply, form of solutions and functionalities. As the market becomes more mature, we would expect to see more standard definitions and interpretations of ePrescribing systems and their functionalities to emerge. Such a market, with larger numbers of live implementations and more mature products, would facilitate decision-making for the procurement of desired products. 37
Implementation process
An overview of ePrescribing implementation challenges
To support smooth and successful implementation, adopters called for increased guidance in relation to implementation strategy, system choice and standards. Furthermore, they pointed out the need for additional financial resources to fund local activities that went beyond what had been provided in the original business plans. Preparations for implementation may need to remain realistic with respect to system capabilities and the speed with which they can be improved. Desired functionalities, including integration with existing local and primary care systems, as well as more sophisticated CDS, may increase the effectiveness of clinical practices, but the attempt to realise desired improvements needs to be balanced against the undesired consequences, such as delays or new safety issues. 55
Tensions between design, usability and implementation
We identified several tensions in the design and implementation of ePrescribing systems. First, the ad hoc management of user modification requests proved problematic. Although hypothetically it would be best for users to spell out their requirements in the initial specification of a system when no or few design decisions have been made, in practice users have limited prior understanding of systems and how they may be used. Users acquire detailed understanding of specific ePrescribing systems and their fit to clinical practices and organisational routines only during the implementation process. As a result, many user requests emerge only after design decisions have been made. These emergent user requests are difficult to deal with for vendors, especially for those that offer COTS products for which products are largely preconfigured. Depending on contractual agreements, users may not even have the option to interact with vendors directly, as can be the case with joint procurement contracts.
Second, many current products have limited configurability in terms of the range of workflows and practices already available by configuration of the packaged solutions rather than writing new software. They may, therefore, need to be customised to suit the needs of the adopters. Particular issues arise with software solutions that were initially developed for non-UK markets. Anglicised versions of foreign developed packages have not yet been stabilised and require a tight collaboration between users and vendors to match requirements in terms of national standards, norms and terminology. Lack of configurability may have resulted in the use of more workarounds owing to international differences in workflows. 56
Third, adopters often have unrealistic demands. For example, adopters have strong expectations with regard to the level of specificity of local practice that packaged applications need to cater for. This is in contrast to how COTS are being developed. Non-bespoke ePrescribing systems have been initially configured to match practices in other clinical settings that are likely to diverge from work practices in hospitals implementing these systems. Owing to the often subtle differences in work routines in different hospitals, ePrescribing systems need to be carefully configured to fit local specifics. Conversely, adopters may need to consider to what extent it would be acceptable to adapt their work processes to the templates in the COTS solutions. 57
Finally, this ties in closely with the struggle by vendors to develop effective generification strategies, that is strategies to address the diversity of customer requirements while maintaining a generic kernel of core functions and applications that cater for a broad set of user settings. We observed a misalignment between the limited functionality offered by generic packages and the diverse requirements requested by adopting organisations. Sustained adopter pressures for customisation left little space for vendors to achieve effective generification strategies. To overcome this, adopters need to better understand vendors’ packages and the associated opportunities and challenges of making use of packaged solutions. 24
Delays in implementation of ePrescribing systems
Implementation delays are caused by a multitude of factors, including unrealistic or unclear business cases, lack of detailed planning, limited knowledge of ePrescribing solutions, human resource capacity, poor user training, weakness of broader IT infrastructure, development of other applications, customisation and modification of applications. To gain a systematic understanding of the diversity of causes for delays, we have developed a taxonomy of delays in implementation of ePrescribing (and HIT) systems.
The taxonomy denotes a two-by-two delay classification matrix: one axis distinguishes tactical versus unintended causes of delay, and the second axis illustrates internal-related (i.e. the adopting hospital) versus external-related (i.e. the suppliers, other hospitals and policy-makers) causes. This highlights that, although there are strategies to mitigate some project delays (e.g. detailed planning, acquiring better knowledge of the market and systems, stepwise implementation strategies), many other delays are unavoidable. In particular, the relative immaturity of the ePrescribing systems in the market adds to the complexity of estimating the time required to implement, and to stick to, the original implementation plans. Finally, we highlight that some delays are deliberately adopted in the course of efforts to nurture a smooth implementation and longer-term usability, as well as improved performance of the system. Long-term planning, starting prior to the procurement of systems and continuing as the system is implemented, may help to minimise the risk of these delays. Whether to delay the go-live date and accept the consequences of deferred adoption or to roll-out the system and disregard the possible longer-term impacts of unplanned delays is a complex decision. 42
Integration and interfacing
ePrescribing systems can be distinguished by their form of solution, that is their technical approach of how they deal with data integration. Stand-alone systems cover a subset of clinical practices, whereas integrated systems aim for a comprehensive solution that incorporates a larger set of applications and clinical processes.
Although integrated systems offer the potential for an improved user experience and facilitate the availability of information in one place, they also limit the potential for customisation and innovation, and often mean that hospitals are ‘locked-in’ to extending their procurement with a single supplier. Integrated systems may be seen as a platform because they cover many different functionalities, of which ePrescribing is just one. 41 Additional interfaces to other systems can be added, but these need to fit in with what already exists. In some ways, resorting to an integrated system to achieve a bundle of functionality may paradoxically pose new kinds of challenges to improving design and usability. Interfaced systems, on the other hand, do offer increased flexibility in procurement, but present issues in relation to incorporation of information and user experience (integration and interfacing).
For both solutions (integrated solutions and interfaced stand-alone systems), we encountered interface integration issues: systems do not yet support workflows of all kinds of health professional throughout the care pathway. Although integrated systems offered somewhat better usability, interfaced systems provided more flexibility and room for innovation. This is particularly salient in relation to interoperability with external systems and to customising these to the needs of different user groups. 51
Pressures for standardisation may be at odds, with tendencies to adapt a system to the local specifics of a particular clinical setting. The highest desirable level of integration could, therefore, consist of an overview of information for all user groups, with the ability for various professions to switch between screens and go into different modules of the system for detailed information (as we observed in integrated systems). 51
Organisational consequences
Medium-term consequences for health professionals
Among the promoted benefits of ePrescribing systems is the opportunity for cost savings incurred through productivity increases. There were some accounts of systems saving time by, for example, health-care workers spending less time trying to decipher handwriting as a result of improved legibility, and quicker generation of discharge summaries because forms were created automatically from inpatient medication lists. At the same time, most users also reported negative consequences for individual workloads, with some tasks perceived as having become more time-consuming across professions. For example, interfacing issues in stand-alone and integrated systems can increase user workloads, open up possibilities for new errors and have adverse impacts on the timeliness of care provided. 51 This contrasted heavily with organisational expectations of time savings for clinical staff. 58
Our data indicate that viewpoints can change from an initial sense of frustration and alienation – in a context in which change is disrupting work routines and creating additional tasks and in which system utilisation and exploitation of system functionality are held back by an initial lack of familiarity with these complex systems – towards an increasing appreciation of more advanced system features over time and more effective integration into work practices. 32
Safety outcomes51
We found, as is generally acknowledged in the literature, that the inadvertent introduction of new, often unanticipated, risks with new health technology is likely. Unintended safety threats were caused by inadequacies in the design of applications, inappropriate use of systems, problems arising from implementation strategies or a combination of the three. 51
From the system point of view, enhancement in system design by suppliers, including the improvement of existing functionalities, the improvement of decision support and alerting tools, better user interface designs, and enhanced integration and transferability of data between modules and systems, were seen as the key enablers of improved patient safety outcomes. 51
Likewise, adopters also implemented strategies (short term, long term and ongoing) to mitigate the potential risks associated with unintended safety threats. These strategies were aimed at improving the culture of use (i.e. through training and user awareness, encouraging incident reporting, and change of work practices), enhancing system implementation strategies (i.e. through better implementation planning and assessment of workarounds) and optimising systems (i.e. through upgrades and system extension). 51
We observed that integration and interfacing problems obstructed effective information transfer in both integrated and stand-alone systems, resulting in threats to patient safety. These threats emerged from the lack of availability of timely information and duplicate data entry (e.g. multiple log-ins). At first sight, integrated systems appeared to obviate such problems. However, given that these were multimodular systems with different modules addressing particular functions (e.g. ePrescribing and laboratory results), there was a perceived lack of data coherence in accessing information across modules. This may reflect their historical evolution through piecemeal addition of modules to integrated systems to support particular functions, as well as the failure to develop interfaces that bring together on a single screen all of the kinds of data needed by health professionals for care delivery at different stages in the care pathway. This requires ‘presentation integration’, which some advanced implementers had begun to address over time. 51
Adopters of ePrescribing systems may wish to consider improving the culture of use through appropriate training, as well as by assessing the processes offered by the systems and redesigning work practices where adopted systems’ processes are more advantageous.
Workarounds
The lack of fit between the work practices and the technical capabilities of the new technology prompted users to develop workarounds. We identified different types of workarounds employed by users. These often involved using paper and other technologies for intermediary storage of information, potentially leading to patient safety risks. Workarounds were most commonly associated with perceived limitations in system design. Our inductive analysis indicated that workarounds could be divided into two categories: ad hoc workarounds, conceptualised as informal practices employed by users that were not approved by management (including the implementation team and senior clinical managers), and formalised workarounds, conceptualised as necessities that were accepted and sometimes actively promoted by management. The distinction between informal and formalised workarounds can be particularly helpful in promoting organisational learning, co-operative working and patient safety. 59–63 It builds on the notion that workarounds can be used as intentional organisational strategies to overcome design and usability limitations of existing offerings and enable users to effectively deploy and gain benefits of using new systems. 56
Workarounds can both introduce new risks to safety (most commonly associated with a lack of up-to-date clinical information) and mitigate risks (resulting from poor system functionality and/or usability). Given that workarounds often involve innovative user behaviours, they may also be used as a source of design enhancement. If workarounds are formalised, it is easier to track and anticipate emerging issues as well as embark on mitigating action to address said issues. This suggests that workarounds become a threat to organisational functioning only if they are not formalised. 56
Medium- to long-term benefit realisation and system optimisation
Long-term user engagement
There is an opportunity for more concerted efforts in benefits realisation in the post-implementation period. Our work indicates that a longer-term model of user engagement may prove helpful. This model could foreground potential concerns of different user groups and pursue different strategies to target these concerns over time. Such a model could reflect issues surrounding longer-term system optimisation and benefits realisation, phases which have largely been overlooked by the episodic view of technologies. 32
Secondary uses of data
We found organisational benefits that were related to secondary uses of data. However, policy-makers may not appreciate the length of time associated with enabling a meaningful utilisation of secondary uses of data. Most of the users reported that, although secondary uses of data were conceivable, they lacked the appropriate skills and tools to extract and process the data accordingly. Employees were sent to gain skills in data analysis and the use of third-party applications. This illustrates the value of more realistic estimates of timelines, costs and returns, as well as the tracking of emerging benefits through longitudinal evaluations of systems and processes. 58
Optimisation and the future of ePrescribing
ePrescribing is not a final stage, but instead serves as an important stepping stone in the integration of health-care systems and services, wherein such software functionality will become a fundamental part of health IIs. 63 To achieve this, there is an urgent need to appreciate the existing complexities and heterogeneities foregrounded by our evaluation. These issues have international applicability as an increasing number of countries strive to stimulate adoption of ePrescribing technologies. 24,64–67
Extending ePrescribing systems to include other clinically important functionalities needed to support care was still an aspiration in most sites, although some advanced integrated systems had begun utilising advanced functionalities. However, integrated systems were seen as being difficult to interface with external systems. 51
Our data suggest that improvements in system design and integration may improve productivity and workflow. This is likely also to depend on the availability of computer terminals and the available support to computing and communication facilities. 58
Given that the ePrescribing market is in an early immature state, with the diverse offerings on the market not yet fully adapted to the circumstances of UK adopters, tried and tested solutions have not yet become established. Some ‘shakeout’ in the market can, therefore, be anticipated, which may well disrupt existing market relationships and offerings. Subsequent withdrawals, mergers and acquisitions may present problems for hospitals locked in to prior procurement decisions. We may anticipate re-implementation of new solutions, in particular for hospitals that initially chose low-cost stand-alone solutions as an expedient stepping stone. A detailed analysis was undertaken of the structure and dynamics of the ePrescribing market. We noted that a large number of suppliers had been attracted to the market, although many offerings had only limited uptake in the UK market and were not well-aligned with adopter contexts and practices. We undertook a comparative analysis of the evolution of this market and patterns previously analysed by the research team in the market for enterprise solutions. This identified strongly homologous developments. On this basis, we were able to make inferences about the probable trajectory of the ePrescribing market. 24,37
Optimising an installed system is not the only route that a hospital has to take post implementation. Given that the ePrescribing market is yet in an early immature state, it is likely that unexpected developments will shake up existing market relationships and offerings. Recent mergers and acquisitions remind of such tendencies. 24 For hospitals, this means that their decision to procure one system can be reversible. Redeployment may be a possible option in the medium to long run, in particular for hospitals that may initially choose a more cost-effective stand-alone solution as a stepping stone to the EHR roll-out.
ePrescribing systems can potentially offer considerable support for many quality initiatives, such as antimicrobial stewardship in hospitals; however, there is currently uncertainty as to how these interventions are optimally conceptualised and deployed. Therefore, there is a need for further studies of optimisation of ePrescribing systems to support medication-related quality improvement initiatives in hospitals.
System knowledge exchange mechanisms in the health information technology sector
A lack of clear user preferences, the existence of varied workflows and the diversity of offerings from vendors exemplifies a market that is still in the emergence and growth stage. Hospitals have been found to lack knowledge about different aspects of the implementation journey. The limited adopter understanding of available options and the heterogeneity of user demands to modify vendors’ products could lead to either ambiguity in characterising the target product or rejection of systems with standardised modules or interfaces. This is particularly noteworthy given that effective ePrescribing requires alignment between user workflows and vendors’ products. However, there are also systemic hindrances that slow down the uptake of the new technology. Across our six case studies, we noted a failure to conserve and redeploy ePrescribing/HIT implementation expertise within the NHS; for example, clinical leads who acquired considerable experience in implementing a technology reverted to their previous clinical role, rather than applying their expertise to implementing these technologies in other settings. We did not find evidence of institutional mechanisms for reusing (and thereby strengthening) this kind of hybrid expertise in the health sector. It would be useful for further research to explore this issue further. 24,37
The patient view of ePrescribing use
Parts of this section have been reproduced with permission from Lee et al. 45 This is an Open Access article distributed in accordance with the terms of the Creative Commons Attribution (CC BY 4.0) license, which permits others to distribute, remix, adapt and build upon this work, for commercial use, provided the original work is properly cited. See: https://creativecommons.org/licenses/by/4.0/. The text below includes minor additions and formatting changes to the original text.
A central theme from the patient perspective of the prescribing process in hospital is a lack of patient involvement. This may become especially pertinent in an ePrescribing environment. Given current health service policies for engaging patients in their care, it is surprising that the studied ePrescribing systems did not allow patients to access further information about their medication. Specifically, by removing the paper chart at the end of their bed, patients had less access than before to information about their medication. It is also clear that an ePrescribing system does not inherently facilitate best practice in self-management. 45
Current health service policy highlights the lack of inpatients’ involvement in prescribing and decision-making, yet the introduction of an ePrescribing system may be doing little to change such cultural norms. Those implementing ePrescribing systems in hospitals may find it helpful to be aware that patients are more concerned with knowing that an opportunity exists to ask questions and provide input for decisions, rather than knowing about the existence of the new ePrescribing system itself. 45
Conclusions
Based on our findings, we offer a set of key lessons on the different aspects affected by the implementation and use of ePrescribing systems. This is followed by concluding remarks on the work of WP1 and a description of inter-relation with other parts of the programme.
Key lessons
Business case
Developing a business case is a necessity for the timely procurement and implementation of a system. In a yet immature and growing ePrescribing market in which understanding of the technology and knowledge about products on the market is limited, extreme efforts are required to align hospital processes and those of the vendor offerings. The business case should ideally be precise, appreciate the complexities of the implementation site and build on previous experiences of implementing HITs. 42
Procurement guidelines
National guidelines based on successful implementation experience may help reduce procurement uncertainties and could avert the procurement of immature solutions. Guidelines could help adopters to make more realistic assumptions and compel vendors to adopt more long-term strategies in the development of their offerings. 24 The ePrescribing toolkit that was developed as an output of this programme provides a starting point for such deliberations. 41
Central guidance
Trusts are facing the choice of various systems with differing capabilities and costs, while lacking central guidance in relation to system standards and implementation strategies. There is, therefore, an apparent opportunity for a central body facilitating the development of systems according to standards that ensure usability and interoperability. 55
Developing and sharing organisational knowledge
Hospitals and policy-makers may wish to improve their knowledge of COTS applications (both benefits and risks), the types of systems available and the interfacing and integration strategies (and their risks and benefits). Experienced adopters could share their insights about functional shortcomings and possible workarounds. 24,51
User engagement
It is important to consider engaging users in all stages of the implementation journey. The realisation of benefits and long-term system optimisation depend on the attentive consideration and evaluation of the diverse and emerging interests of various user groups. 32
Technology development
Supplier offerings are likely to mature over time in terms of basic functionality as well as advanced features, for example clinical decision support, interoperability and secondary use of data. Vendors could pursue generification strategies to ensure standardisation across hospitals while, at the same time, allowing for flexibility to cater for local specifics. 24,37
Culture of use
User organisations could more effectively foster a culture of use of ePrescribing and HIT application by providing appropriate training and revising their use strategies regularly to adapt to the shifting use requirements and the accumulating functionalities offered by the installed ePrescribing system.
Patient involvement
Patients’ views and expressed needs appear to have been largely absent in the development and implementation of the ePrescribing process. Although it may be difficult to involve patients, their participation could be sought as they bring important insights and expertise and can help prioritise further development of health-care services, including the ePrescribing practice. 45
Formalisation of workarounds
Workarounds are necessary in the context of usability shortcomings of immature products; however, a process could be in place to resolve unwanted informal workarounds and to formalise acceptable ones that help promote effective use of systems, organisational learning, co-operative working and patient safety. 56
System optimisation
Implementation of an ePrescribing system is the first step of a journey. System optimisation is marked by periodic major upgrades, continual customisation of the system, re-configuration of clinical practices, secondary uses of data and other post-implementation activities. Implementing ePrescribing offers a long-term learning process.
System knowledge exchange mechanisms
The resolution of the current state of dual immaturity of both the technology and the market calls for engagement and consensus between not only adopters and vendors, but also larger communities, including policy-makers and field experts, and intermediaries, such as industry analysts and implementation consultants. 24 Constant renewal of knowledge on the status of this evolving market can facilitate this, including knowledge regarding the development and adoption of products. 37 Knowledge transfer mechanisms, for example between adopters and suppliers or intraorganisational collaborators, could be established to allow vendors to better understand diverse adopters’ needs and practices, and cater for them in the libraries of workflows and practices available within COTS solutions by local configuration, rather than slow/expensive ad hoc customisation. In the longer term, these mechanisms can help orchestrate the co-evolution of software solutions and health service practices. 42
Concluding remarks
In this WP, we have demonstrated that the implementation of ePrescribing systems is a highly complex and potentially challenging process that needs to be understood in relation to the extended system implementation life cycle. 41 This life cycle begins with plans to procure a new system and continues through a protracted process in which the system is implemented and integrated with care practices, organisational processes and other information systems. Furthermore, this is merely a starting point for further cycles of system enhancement and optimisation.
The longitudinal approach adopted in this programme considered developments at different moments in the implementation journey and at multiple implementation sites. Many hospitals experienced lengthy delays while awaiting system enhancements; some even cancelled or postponed the implementation indefinitely. Hospitals that managed to finish the implementation stage and that overcame the initial set of technological and organisational difficulties eventually managed to achieve stable and effective operation of the new system. However, to realise the benefits promised by the technology, hospitals need to regard the implementation of an ePrescribing system as a continuous learning process. We did not find any established model for the use of ePrescribing systems that embraced this concept and could represent an established best practice approach. Efforts to get the system up and running pave the way for further efforts to optimise the utilisation of the technology that go beyond regular upgrades. There is an opportunity for hospitals to undertake long-term, detailed planning to make the best use of advanced functionalities, such as clinical decision support, secondary use of data and reporting.
Despite this extensive longitudinal investigation, we were unable to observe how the adoption of ePrescribing systems plays out in diverse organisational contexts in the long term. Taking into account that many hospitals that intended to procure and implement ePrescribing systems withdrew or postponed their plans, it would be risky to make predictions about development trajectories and how these systems will perform in the future. There are further substantial challenges in generalising from a selected sample of in-depth case study sites. Therefore, future research into how the practice of ePrescribing becomes embedded in our early adopters sites in the long run and in a wider sample would produce worthwhile insights for hospitals that are currently in the process of implementing or are planning to implement these systems in the near future.
More generally, ePrescribing systems are best viewed as a complex organisational intervention being introduced as part of wider organisational transformation initiatives. If this is not the case, then there is a risk that the implementation may be abandoned by implementing organisations.
Inter-relation with other parts of the programme
This WP has provided important contextual information for WPs 2 and 3, including an understanding of the experiences of system users and implementers, as well as the ‘hidden costs’ of new systems (further discussed in Work package 2, Discussion). It has further provided content for the toolkit (WP4) by developing detailed typologies of ePrescribing systems and suppliers, extracting common lessons across case studies of implementing hospitals and mapping of various aspects of implementation and optimisation strategies.
Work package 2: assessing the impact on prescribing safety
Background
Medications are the most common therapeutic intervention in health care, and over half a million prescriptions are generated in the NHS on a daily basis. Prescribing is a potentially risky process and, although some adverse drug events are unavoidable (e.g. new drug allergies), many adverse events (AEs) are preventable. Preventable medication-related harms are, therefore, classified as medication errors. All health-care practitioners who prescribe have an obligation to be competent to undertake the task, but education and training cannot eliminate all errors. Various strategies have been introduced to tackle medication errors (of which prescriber errors are a subset), one of which is the adoption of ePrescribing systems with CPOE systems plus or minus CDS functionality. The key objective of this WP was to determine whether or not prescribing error rates changed as a result of the implementation of ePrescribing systems.
To evaluate the effectiveness of ePrescribing systems, we focused on COTS systems that are more likely to be implemented in NHS hospitals than ‘bespoke’ systems that have been developed in academic centres. One of the key lessons learnt from previous medication error studies, regardless of the method of prescribing, is that untargeted prescription review can lead to reporting of very large numbers of errors that have minimal clinical impact. Such errors have been shown to be a poor surrogate of more serious errors. 68 Efforts, therefore, need to be directed towards evaluations focusing on errors that are most likely to lead to serious AEs. We, therefore, focused on studying prescribing indicators that are judged by experts to have a significant risk of translating into actual patient harm.
Research aims
The overarching aim of this WP was to estimate the effectiveness and relative effectiveness of different ePrescribing systems in reducing the risk of serious prescribing errors.
We sought to:
-
establish whether or not the introduction of ePrescribing results in a reduction in clinically important errors
-
assess whether or not the more advanced ‘alerting’ CDS-based systems are more effective than simpler CPOE-based (‘non-alerting’) systems.
Methods
Parts of this text have been reproduced with permission from Thomas SK, McDowell SE, Hodson J, Nwulu U, Howard RL, Avery AJ, et al. 69 Developing consensus on hospital prescribing indicators of potential harms amenable to decision support. Br J Clin Pharmacol 2013, vol. 76, pp. 797–809. © 2013 The Authors. British Journal of Clinical Pharmacology © 2013 The British Pharmacological Society.
Development of the key prescribing indicators
To have objective outcome measures for prescribing errors that were amenable to reduction with the introduction of ePrescribing systems, we initially set out to define a list of prescription errors using a consensus technique (electronic Delphi, or eDelphi) to gain agreement among a diverse group of experts using methods that we have previously developed. 70 We provisionally identified and piloted 22 indicators (see Appendix 4) to assess the feasibility of measuring errors using an objective indicator-based approach and to inform our power calculation.
We then undertook a three-round eDelphi exercise with 20 expert pharmacists and physicians across England. Participants were asked to score prescribing errors using a 5-point Likert scale for their likelihood of occurrence and the severity of the most likely outcome. These were combined to produce risk scores, from which median scores were calculated for each indicator across the participants in the study. The degree of consensus between the participants was defined as the proportion who gave a risk score in the same category as the median. The mean consensus across all of the indicators was then calculated and indicators were included if they achieved consensus of ≥ 80%. The indicators (see Appendix 5) were intended to be used to create the validated data collection tool described below.
Development of the data collection methods: the IMPACT tool
There are many different types of error detection systems that are used in medication error systems71 and, although computer monitoring is often feasible after implementation of an ePrescribing system, in a prospective pre- and post-implementation study the detection method needs to remain stable. Therefore, prospective data collection using direct observation was the chosen method. To facilitate the collection of data, we decided to produce a novel, stand-alone data collection tool for this programme of work.
We developed the Investigate Medication Prescribing Accuracy for Critical error Types (IMPACT) tool specifically to ‘Investigate Medication Prescribing Accuracy for Critical error Types’. A clinical/technical development team worked on a specification for this stand-alone Microsoft Windows application (Microsoft Corporation, Redmond, WA, USA), which was developed using C# and .NET Framework technologies. The idea was that the IMPACT tool would collect information for each consecutive patient prescription chart review on a form containing a series of questions to objectively capture data about the patient and the drugs that they are prescribed (see Appendix 6). Its format and content are defined in a clear structure and the program is designed to make the forms highly configurable with the questions presented changing dependent on the answers given.
Selection and recruitment of sites
We sought to undertake detailed case studies in at least two hospitals that were planning to implement basic (i.e. CPOE) and advanced (i.e. CDS) ePrescribing systems, respectively. We identified 29 English trusts that were planning ePrescribing system implementation and contacted all of these sites to determine their initial interest in taking part in our studies. We ultimately selected the first four trusts that replied indicating an interest in the study. In view of the risks of major delays with implementation, we deliberately over-recruited, recruiting four identified hospitals of which three subsequently had go-live dates during the course of the programme. We planned to evaluate the critical prescribing indicators as ePrescribing was rolled out across these hospitals using a pragmatic stepped-wedge design. However, repeated delays resulting from contractual issues and changes to the implementation plans rendered it impossible to pursue this approach and we, therefore, needed to settle on a more pragmatic pre- and post-implementation design.
The power calculations suggested that for each site the study population would need to consist of approximately 1100 patients from six wards (preferably higher-throughput wards, such as acute medical wards). Although many sites have subsequently performed data collection using the IMPACT tool (see Work package 4), we report here the results of three formally recruited sites. In addition, pilot data were captured from a separate hospital site to validate the use of the tool in practice.
We engaged with each case study site to identify the implementation dates of clinical areas. Given that our preliminary work indicated that we would not be able to influence the roll-out plans to randomise the order in which the clinical areas implement ePrescribing, we took the approach of choosing similarly sized clinical areas (blocks of measurement) implementing ePrescribing in an ordered and timely manner. Participants were all inpatients on the selected wards on the days of data collection.
Data collection
Many clinical areas capture medication errors on a routine basis and we, therefore, used experienced clinical staff to undertake the formal data collection at the chosen sites. The CPOE pharmacist at each hospital was trained on the use of the IMPACT tool; therefore, the CPOE pharmacist could train the pharmacist or technician who was allocated to perform the data collection for the study. The pharmacist/technician at each hospital site was asked to visit inpatient medical wards and review patients’ medication charts using the IMPACT tool. This was deemed under the current NHS research governance arrangements as a clinical audit and, therefore, no explicit individual patient consent was required. For analytical purposes, the data were transferred to the central academic statisticians using the secure data transfer capability of the IMPACT data tool. Permission was obtained from the Caldicott Guardian for the use or transfer of information from each organisation, which was anonymised at source.
The data collector visited the participating wards/clinical areas twice per day and noted any new patients with prescriptions in those areas following the standardised data collection process (see Appendix 7). The data collector carried out live observations of medication orders from all patients in that clinical area. All orders (including regular, one-off and as-required drugs, but excluding fluid prescriptions) for each patient were assessed for completeness and clarity. A count of any ambiguous orders was made, together with coded reasons for this (e.g. unclear dosing units). Basic data about the prescriptions were collected (e.g. completeness of drug allergies and missing information) because these represent non-alerting indicators of prescription quality. Sites were asked to keep track of which patients had been reviewed to avoid duplication. Case selection used a convenience sample approach, with sites given flexibility regarding the time that reviews were carried out and the patients chosen for review.
If any of the drugs or drug classes within the alerting prescription indicators were identified, a full review of these specified orders, together with a review of the patient’s records where necessary, was made to identify any of the target indicators. In the post-implementation phase, a review of the on-screen prescription orders (or, where necessary, printed prescription orders) was undertaken to check for the completeness and the elements of the non-alerting indicators.
We also undertook a review of the ePrescribing system (training or copy system) to identify whether or not there were any CDS warnings present for each of the proposed prescription indicators within the system in the post-implementation period. This was essential to identify whether there were any formulary issues or ‘hard-stop’ warnings that may have skewed data collection, as the error would not be permitted by the system and, therefore, would not be measurable. The decision support for each error at each site was categorised into one of five stages of alerting according to set definitions.
Data collection disparities
Feedback from data collectors at the different sites revealed some disparities in the process of information retrieval that had the potential to affect data integrity for two of the 80 indicators, both of which related to the use of low-molecular-weight heparin. Data for a failure to prescribe one of the specific indicators (low-molecular-weight heparin omitted to be prescribed for prophylaxis) were captured in different ways; therefore, this was analysed separately from other error categories. Post hoc investigation also revealed a nuance in the recording of weight data (relevant for the indicator of low-molecular-weight heparin prescribed without the patient’s weight being used to calculate the treatment dose) in one of the sites. The system did not allow this error to occur at this particular site because weight was a mandatory field in the prescribing window. However, this weight recording did not subsequently transfer to the electronic chart that was visible to data collectors. After discussion with the research team of pharmacists and the pharmacologist, it was agreed that this was not technically an error; therefore, this was not included in the error calculations at this particular site. In view of these findings, these two errors were removed from the analysis, leaving 78 errors for consideration.
Data analysis
Statistical methods
Error rates were computed as [number of errors (NErr)/number of opportunities for error (NOpp)], where ‘opportunity’ meant that the medications associated with the relevant IMPACT indicator were prescribed or, in the case of prophylactic medicines, should have been prescribed. Rates were calculated with binomial confidence intervals. The change over time was represented using ratios of error rates with confidence intervals from Poisson regression analyses and two-sided p-values from Fisher’s exact test of proportions. For the purposes of the analysis, an assumption was made that there was statistical independence of errors within patients.
Sample size
The study was powered to detect a reduction in the overall error rate from 5.5% to 4.0%. The baseline rate (5.5%) was informed by the paper-based data in a pilot hospital. To achieve 80% power (with a two-sided p-value of 5%) in each hospital separately, it was calculated that 3156 error opportunities were required under each condition (i.e. paper-based and ePrescribing) at each site. With 9.13 error opportunities per patient (from pilot data), this was expected to translate to at least 346 patients (i.e. 3156/9.3) per hospital, which equals 346 patients.
Results
The eDelphi study identified 80 prescribing errors as being high or extreme risk by consensus. The most common drugs and drug classes named in the indicators were antibiotics (n = 13), antidepressants (n = 8), non-steroidal anti-inflammatory drugs (NSAIDs) (n = 6), opioid analgesics (n = 6), antiplatelets (n = 5), methotrexate (n = 4), low-molecular-weight heparins (n = 4) and benzodiazepine (and like) drugs (n = 4) (see Appendix 5). These prescribing indicators were translated into the IMPACT tool for the collection of data relating to high-risk prescribing errors, in both paper-based and ePrescribing processes. The full details of the consensus methods and the results of prescribing indicators have been published in full, and can be seen in Acknowledgements, Publications.
Drug charts from a total of 2422 patients were reviewed at the three sites: 1244 before and 1178 after the implementation of system A (integrated CPOE and CDS) at sites J and K, and system B (stand-alone CPOE system with low-level CDS switched on) at site S. These drug charts contained a total of 28,526 medication orders: 14,371 before and 14,155 after implementation. Following implementation, the proportion of medication orders that were technically incomplete, or that showed inappropriate dose units or route of delivery, was reduced to zero at all three sites (from 61.80%, 5.06% and 4.94% at sites J, K and S, respectively). The very large number of incomplete orders at site J on paper resulted because of the omission of a bleep number (contact details for their air pager) for the prescriber, which was regarded as an incomplete order according to this hospital’s protocol; this was not the case at sites K and S. In addition, some improvement was noted in the documentation of allergy status and (especially) of patient weight at sites K and S, but not at site J in which the proportions of patients with documented allergy status and recorded weight fell slightly (see Appendix 8).
IMPACT indicators
From the 28,526 individual medicine orders across both periods, 21,138 opportunities for error were identified. In total, 75 out of 78 (96%) of the defined prescribed errors across the three sites had at least one opportunity to occur (Nopp) pre implementation, and 70 out of 78 (90%) had an opportunity post implementation. Over the study period, 44 had > 100 opportunities for error and 28 had > 200 opportunities for error.
Across the three sites as a whole, the proportion of opportunities for error that resulted in an error occurring was found to reduce significantly after the introduction of CPOE, from 5.0% to 4.0% (p < 0.001). When analysed on a site level, similar reductions in error rates were observed after the introduction of CPOE at both site J (4.3% vs. 2.8%; p = 0.002) and site S (7.4% vs. 4.4%; p < 0.001). However, no significant change between periods was detected for site K, with error rates of 4.0% versus 4.4% in the pre-CPOE versus post-CPOE periods (p = 0.294), respectively; see Appendix 9 for details.
Each site tested every indicator through their system (on test patients) to help to identify the extent of CDS actually available to the prescriber at the time of post-implementation data collection, and provided a brief description of the outcome. Five categories of CDS were extracted from these descriptions and are defined in Appendix 10.
All categories of CDS were employed at every site, with just two exceptions: ‘Guided’ was not used at site K and ‘Alert’ was not used at site S. In total, 43 out of 78 (55%) errors had some degree of CDS implemented (i.e. alert to restricted; Table 2) in at least one of the sites, leaving 45% (35/78) of the errors with no CDS at all. The rate of CDS implementation did not differ significantly across the three sites (p = 0.293), with 27 out of 78 (35%) errors triggering some form of CDS at sites K and S, and 19 out of 78 (24%) doing so at site J. The indicator relating to the omission of low-molecular-weight heparin treatment for prevention of venous thromboembolism (VTE) was treated separately in the analysis (see Appendix 11). Uniquely, among the 80 indicators this generated an error opportunity for every single encounter given that every patient was required to have a VTE assessment (a routine assessment for every admitted patient in NHS hospitals). This opportunity for error was not subject to active CDS at any of the sites and there were wide disparities between sites in how the error was assessed.
Site | Pre CPOE | Post CPOE | Relative risk (95% CI) | p-value | ||||||
---|---|---|---|---|---|---|---|---|---|---|
Patient records | N opp | N err | Error rate (%) | Patient records | N opp | N err | Error rate (%) | |||
J | 365 | 3867 | 167 | 4.3 | 297 | 2634 | 74 | 2.8 | 0.65 (0.49 to 0.86) | 0.002 |
K | 615 | 4513 | 179 | 4.0 | 581 | 4527 | 200 | 4.4 | 1.11 (0.91 to 1.36) | 0.294 |
S | 264 | 2932 | 216 | 7.4 | 300 | 2665 | 116 | 4.4 | 0.59 (0.47 to 0.74) | < 0.001 |
Total | 1244 | 11,312 | 562 | 5.0 | 1178 | 9826 | 390 | 4.0 | 0.80 (0.70 to 0.91) | < 0.001 |
At each site, the analysis of pre- and post-implementation error rates revealed a reduction in the observed error rates across all categories of ‘active’ CDS (i.e. restricted, guided, permitted and alert). In particular, the error rate under ‘restricted’ CDS reduced to zero at all three sites (see Appendix 12). Many of these error reductions achieved statistical significance (see Appendix 11). The major differences between the sites concerned the behaviour of errors that were not subject to active support, including the VTE prevention error. At sites K and S, the rate of VTE errors rose by approximately 30%, although the changes were not statistically significant (see Appendix 11). By contrast, the VTE prevention error rate fell at site J to 34% of its pre-implementation level (p < 0.0001). The error rates for the remaining unsupported indicators fell at site J (rate ratio 0.69; p = 0.0226) and at site S (rate ratio 0.55; p < 0.0001), but increased by 50% at site K (rate ratio 1.50; p = 0.0011).
Discussion
The outcome for each key deliverable is shown in Table 3.
Key deliverable | Progress |
---|---|
Estimate the effectiveness of the different systems and provide some indication of changes in effect over the time course of early implementation (i.e. the first 12 months) | We have collected and analysed the data from three sites pre and post implementation of ePrescribing and provide quantitative estimates of the effectiveness of different systems/configurations. These data have been published in Pontefract et al.72 |
Generate posteriors for the Bayesian analysis and, thus, inform the value of investment analysis in WP3 | The quantitative data provide information for the health economic analysis (see Work package, Overall approach) |
A refinement of methods, using defined indicators, to develop functional indicators for certification of systems, which we will test in our case study sites to ensure reproducibility in a variety of systems, and make available in our toolkit | We have undertaken an eDelphi and developed consensus over ley prescribing indicators that indicate potential harms. This work has been published in a journal article in the Br J Clin Pharmacol.69 In addition, we have produced the IMPACT tool as a standardised electronic data capture tool to collect data in the hospitalised setting for prescribing errors: this tool is available via the toolkit |
The implementation of the ePrescribing systems under investigation was associated with a reduction in specific, serious and commonly occurring prescribing errors where CDS was available. Given the before–after design employed in this service-level evaluation, we are not in a position to make clear causal inferences; however, the size of the effects seen and the fact that there were no other major transformations in the hospitals over this time period suggests that the introduction of ePrescribing systems is likely to have been responsible for these effects. Ideally, future studies should select a cluster design with contemporaneous controls, such as the step-wedge cluster approach that we had originally planned.
As expected, there were major improvements in procedural errors at all sites, such as improved legibility, completeness, and allergy and weight reporting. There was also an elimination of documentation of dose unit errors. This is typical of improvements seen in any CPOE implementation regardless of whether or not there is active CDS.
Although we initially set out to examine differences in effectiveness between more advanced ‘alerting’ CDS-based systems and simpler ‘non-alerting’ CPOE-based systems, this dichotomisation of system types did not strictly hold true. In fact, when we tested our prescribing indicators on test patients in the two different systems, there was a varying level of CDS in all of the sites. However, the presence of any active CDS did lead to a reduction in prescribing errors. In particular, where sites implemented a ‘restrictive’ level of CDS, associated errors were eliminated, regardless of the error type. Each site had stated that they were concerned about alert fatigue and, therefore, implemented minimal levels of CDS (approximately only 25% of the opportunities for errors being studied). We identified five different levels of CDS and, although two sites implemented the same system, we found that there were differences in local configuration that led to differences in CDS alerting and obvious differences in error rates. The culture of the organisation and, therefore, how they configure their ePrescribing systems is vitally important,73 because implementing a system that has led to improved safety elsewhere does not in itself guarantee a reduction in error rates owing to the configuration differences. The findings from our study suggest that where it is possible to identify specific institutional problems leading to prescribing errors (e.g. common mistakes or inappropriate drug combinations), targeted CDS could prevent these errors from occurring. 74
The errors that were measured were chosen because they were potentially amenable to CDS if implemented within the scope of current systems. However, there are other significant errors or error types for which CDS might improve safety that are dependent on information from other systems (e.g. electronic patient noting of comorbidities or pathology test results). In our research, the sites focused on getting ePrescribing installed and widely used as part of the immediate implementation strategy; therefore, additional integration of other data had not yet been achieved or was not possible. The research team were careful not to influence the decision-making at hospitals and have, therefore, reported on real-world ePrescribing implementation. Additional system integration and optimisation may lead to further improvement in quality and safety, and the hospitals can continue to measure these prescribing errors in this context.
Computerised provider order entry and CDS have been associated with new error types. Identifying these errors is important in the ongoing monitoring and optimisation of systems to minimise the risks of the technology to patient safety. However, in view of our approach to capture data on the occurrence of defined high-risk prescribing errors, investigating the specifics of any new errors was outside the scope of the study.
Implications
Previous studies75,76 have demonstrated that alert fatigue is problematic in practice and that alert over-rides can be inappropriate. This study has shown that varying levels of CDS have different impacts on medication error rates. Previous work that has focused on CDS acceptance and human factors highlighted the differences in acceptance of CDS alerts dependent on the level of acknowledgement required,77 and Phansalkar et al. 78 highlighted the importance of having different levels of alerts relating to the severity of the error. Rather than switching on all possible alerts, sites implemented CDS selectively, focusing on the most serious drug interactions and allergies, which meant that 75% of the targeted errors had no CDS. The errors that were chosen to be on at a ‘restrictive’ level of CDS resulted in a complete elimination of those error types occurring. This strategy may have reduced the number of inappropriate over-rides that the implementers were keen to avoid.
The indicators (and the IMPACT tool developed as a result) used in this study were agreed by an expert panel to be commonly occurring and to be serious errors. The top 25 indicators accounted for 81% of the error opportunities, which suggests that these were indeed the appropriate focus. In addition, using this audit tool provided an objective view of the potential for serious errors in hospitals, as incident-reporting data (including near misses) in hospitals can be unreliable. 79
Conclusions
In this first real-world evaluation of commercial ePrescribing systems on clinically important prescribing errors across three hospitals in NHS England, prescribing error rates post implementation fell significantly, suggesting that CPOE systems when implemented with even relatively low levels of CDS can improve medication safety. We have been unable to specifically determine whether or not increasing the number of CDS warnings has a differing impact on prescribing error rates, but can propose that higher levels of CDS alerting (e.g. restrictive alerts) can eliminate or reduce clinically meaningful errors.
Hospitals considering ePrescribing may find it helpful to consider how their system will interface with existing infrastructure (e.g. laboratory records), as this may have an impact on the types of error that can be targeted. In addition, there may be opportunities arising from the secondary uses of ePrescribing data to improve medication processes to continuously improve patient safety. The implementation of ePrescribing CPOE systems with CDS can reduce medication errors, but with further system optimisation, integration and use of secondary data there is an even greater potential to target adverse drug events and improve patient safety.
Inter-relationship with other parts of the programme
This WP has provided important quantitative data for the health economic WP (WP3). It has further provided content for the toolkit (WP4) by providing access to a bespoke data collection tool that has been made available to other hospitals implementing systems.
Work package 3: health economics
Background
Decision-makers in hospitals need to decide whether or not to invest in new medical technologies. However, the health-care system has limited resources, requiring decision-makers to operate in a framework that maximises the returns produced by the limited inputs. The methods for the economic evaluation of health technologies are well developed and are incorporated into assessments by bodies, such as the National Institute for Health and Care Excellence. However, the evaluation of service delivery interventions, such as ePrescribing systems, is more complicated. Unlike for standard health technology assessment for which the effects of the intervention on the end points relevant to economic evaluation, such as mortality or QALYs, are (or at least can be) measured directly, the evaluation of service delivery interventions requires us to extrapolate from more ‘upstream’ outcomes, such as clinical errors. This is because an important signal (e.g. improved quality of life or fewer deaths because of the ePrescribing system) is likely to be lost in the noise. Consider a scenario in which preventable adverse drug events could be reduced by a worthwhile 25%, a large effect. This would reduce the incidence of these events from approximately 1% to 0.75% in the patient population. Such an absolute reduction of 0.25 percentage points is unlikely to show up in a comparative evaluative study. The logic behind this ‘inconvenient truth’, that worthwhile effects from generic service improvements are likely to be lost in the noise (type II error), is explicated in more detail elsewhere. 80 The cost-effectiveness of large-scale service interventions is further complicated in situations in which there are no fixed costs, but in which costs are locally negotiated as, for example, in the case of an ambulance service or an IT system. Thus, we have to deal with two uncertain aspects of cost-effectiveness: the cost and the effectiveness. We deal with our approach to these challenges below.
Research aims
It is unlikely that an ePrescribing system will have a clinically significant effect on any one patient, on average. However, when measured and aggregated across an entire hospital, the benefits may outweigh the health service costs. This WP, therefore, had the following aims:
-
to further develop the methodology of service delivery intervention evaluation and to develop the methods of evaluating ePrescribing systems
-
to estimate cost-effectiveness parameters based on multiple data sources
-
to estimate the expected net benefits of an ePrescribing system to inform decision-makers.
Overall approach
Previous work and plan
A detailed protocol was published for this WP (see Acknowledgements, Publications). 81 We have also developed further work in conjunction with this programme and others that supports the methodology of service delivery intervention evaluation. 82,83 Only one previous study84 was identified that attempted to estimate the cost-effectiveness of an ePrescribing system. This study used a decision tree approach. However, many of the parameter values were determined through discussion among the authors rather than through empirical observations. They concluded that there was a high probability that an ePrescribing system would have positive net benefits over a 5-year time horizon.
To evaluate ePrescribing, we considered both the estimation of the ‘treatment effect’ on the measured medication errors and the integration of the estimated treatment effect in a larger model that enabled external evidence to be synthesised with evidence from an indirect study, to estimate the overall effect of interest. This approach to service delivery intervention evaluation involved the following stages. First, the specification of a qualitative causal model that linked the intervention to the outcomes of interest via the measured outcomes. Second, the parameterisation of this model and the identification of data to inform estimates of these parameters. Third, the synthesis of these data for estimation of net benefits. 81,82 Fourth, the estimation of net costs. Fifth, a model to reconcile costs, benefits and willingness to pay for a unit of benefit.
The model
The model we chose for this evaluation, which links ePrescribing to costs and QALYs, is conceptually simple. The benefits of the system are mediated by medication errors and consequently adverse drug events. A reduction in adverse drug events both reduces patient costs and improves quality of life. The (direct) costs of the ePrescribing system are a fixed capital cost (amortised over the useful life of the system), along with annual maintenance and training costs. The model structure is shown in Figure 2.
FIGURE 2.
The decision model. a, Assumes all types of error affected equally. b, Assumes proportionality across errors and AEs, and across category of AEs. c, Distributions of quality-of-life loss and life expectancy/duration across category. This figure is reproduced with permission from Yao et al. 85 This is an Open Access article distributed in accordance with the Creative Commons Attribution-Noncommercial (CC BY-NC 3.0) license, which permits others to distribute, remix, adapt and build upon this work, for non-commercial use, provided the original work is properly cited. See: https://creativecommons.org/licenses/by-nc/3.0/. The figure includes minor additions and formatting changes to the original figure.

It might be argued that this model is not sufficiently complex to take into account the necessary contextual variables to understand the impact of an ePrescribing system. Health-care systems are inherently complex systems. They comprise many interacting causal processes across multiple levels. However, it is important to distinguish between the data used to make inferences about the underlying processes and the processes themselves. These processes are constant and stable across different health-care contexts. The effect of an ePrescribing system is targeted towards the same phenomena across all health-care contexts, namely medication errors. Differential use of the system between hospitals may result in heterogeneous treatment effects, which may be reflected in the statistical modelling, but the underlying causal phenomena are the same. Further analysis, whether qualitative or quantitative, would be required to investigate the heterogeneity of treatment effects.
The simple model of ePrescribing effects implies the following estimator of the incremental effect of ePrescribing on patient costs and QALYs arising from averting adverse drug events. The estimated relative risk (RR), which we describe below, serves as an input into the larger model of the effects of an ePrescribing system on patient QALYs in the following way:
The effect on patient costs attributable to reduced adverse drug events (ADEs) is similarly calculated. Further information is, therefore, required to estimate the baseline risk of an ADE and the incremental effect of an ADE on patient costs and QALYs. The health economic evaluation method we describe here takes a health system perspective, including costs incurred by the hospital only and not losses accruing to the patient owing to, for example, lost employment. Although a societal perspective would be preferred, to the best of our knowledge, there is currently no research into the effects of preventable AEs on outcomes occurring outside hospital. This is an important future area of research. We note some key assumptions: first, that there is no other factor that causes a change in the risk that a medication error causes harm and, second, that the implementation of ePrescribing is independent of patient case-mix and risk of medication error.
Estimating health benefits in the form of quality-adjusted life-years
Introduction
The key limitation in the evaluation of service delivery interventions is the lack of evidence on the effects of the intervention on the outcomes relevant to economic evaluation. For choices to be made among different programmes of care, their costs and outcomes need to be compared on the same scale.
A study of ePrescribing systems that aimed to measure changes to QALYs or risk of mortality would require a prohibitively large sample size to detect small average effects. The primary end point measured by this ePrescribing programme was, therefore, medication errors. We described these data in connection with WP2 and the data are given in Work package 2, Results. However, these error rates are ‘upstream’ end points from those relevant to the economic evaluation, such as mortality and additional days in hospital. We measured these ‘upstream’ end points because they are reasonably common among patients and are, therefore, likely to be detectable in a study investigating the effectiveness of ePrescribing systems. The effect of introducing an ePrescribing system on error rates, therefore, needs to be translated into the relevant downstream outcomes for the purposes of health economic analysis (see Figure 2).
We used a Bayesian approach for the statistical modelling. This was for a number of reasons: a Bayesian framework naturally fits into a decision-making framework; prior data can be included in the estimation of model parameters and updated with new study data; probabilistic statements regarding parameter values and outcomes can be made; the uncertainty associated with parameters and hyperparameters can be fully incorporated in any results; and Bayesian methods incorporate value-of-information methods should decision-makers require further evidence.
Bayesian methods are an alternative statistical paradigm to the more commonly used statistical methods in biomedical research, called frequentist statistics. The estimation of parameters, such as the required RR in this study, relies on Bayes’ rule (hence ‘Bayesian’) to ask what is the probability that a parameter has a certain value given the data collected in the study. This estimation requires a model for the data (the likelihood) and the distribution of the parameter of interest before the data are observed (the prior). Both elements require careful consideration.
The key effectiveness parameter was the RR of experiencing a medication error with an ePrescribing system compared with experiencing a medication error without an ePrescribing system. The aim was to estimate both the average effect across all the study hospitals and the posterior predictive distribution of the treatment effect in a new hospital. However, owing to some differences in data collection between sites as described in WP2, the data were not considered to be comparable between study sites. Furthermore, there were not enough sites to estimate the between-site variance in treatment effect. As a result, only individual site RRs were estimated.
Statistical model
The estimation of treatment effects in the Bayesian framework involves the estimation of the unobserved, counterfactual outcomes for each of the patients included in the study. The estimated RR is the ratio of the mean risk of experiencing a medication error with and without an ePrescribing system. We specified a probit model for the risk of experiencing a medication error. The unit of observation within each study was patients. Although information was collected about individual opportunities for error, the harms occur at the patient level. We, therefore, made the simplifying assumption that the severity of the harm resulting from a medication error was independent of the number of errors or opportunities for errors that the patient experienced. As well as having a binary indicator for whether or not the study site had ePrescribing, we controlled for patient age and the number of opportunities for error. Both of these covariates were mean centred to simplify conversion of the elicited RRs to the probit coefficient scale.
Elicitation exercise
The probability in the Bayesian paradigm is interpreted as subjective uncertainty. The prior distribution of the parameter, therefore, represents what is currently known about the parameter. This may be derived from the results of a prior meta-analysis or previous study, should one exist. In the case of ePrescribing, a meta-analysis of the effects of CPOE on the risk of a patient experiencing a medication or adverse drug event was recently conducted. 86 However, the ePrescribing systems that we were evaluating in this study include CPOE as one component only; furthermore, the majority of the studies included in the meta-analysis were based in the USA, where the effects of an ePrescribing system may differ. In addition, we considered that clinical practitioners and pharmacy experts would have important insight into the performance of these systems in NHS England. To construct a prior distribution, we therefore convened an expert workshop.
Expert elicitation methods have been developed to determine prior distributions of parameters. 87 The subjective nature of the process and the aggregation of opinion can arouse doubts in the validity of the results of the elicitation process. Biases can affect the results of the elicitation; for example, the presentation of a particular piece of information can cause participants in the workshop to become ‘anchored’ to that information. We, therefore, developed a protocol specifically for the expert elicitation workshop (see Acknowledgements, Publications). The protocol was designed to maximise the validity of the results from the workshop by minimising potential biases. 88–91 Briefly, the protocol specified that the workshop would consist of seven parts: (1) presentation of key information, (2) training exercise, (3) group discussion and presentation of data, (4) first-round belief elicitation, (5) break, (6) feedback and group discussion and (7) second-round belief elicitation. Each section was designed to minimise the potential biases identified in the scientific literature, including anchoring bias and base rate neglect. The training exercises were designed to familiarise the participants with the elicitation process and to prime the participants to think about the processes involved with an ePrescribing system. The quantile method of the Sheffield Elicitation Framework (SHELF)89 was used to elicit the RR on a log-RR scale. Beta-distributions were fitted to individual elicited densities and then aggregated using linear opinion pooling. 92
The expert workshop was conducted on 24 February 2016 at a session of the conference convened by the ePrescribing programme team. Invitations to the workshop were sent to conference attendees, who were selected on the basis of their knowledge of ePrescribing systems. Eleven participants participated in the workshop. Participants were asked to compare a ‘complete’ ePrescribing system with CDS compared with paper-based prescribing. Appendix 13 shows the individual elicited priors and the aggregated prior. The aggregated prior density for the RR from the expert workshop had a mean value of 0.76 (95% credible interval 0.44 to 1.31). We converted the elicited RR into a prior distribution for the coefficient of the probit model, assuming a baseline risk of medication errors of 5%. For the remaining model parameters, we specified weakly informative normal prior distributions: for the intercept a normal (–1.65, 1) distribution was specified and for the other model parameters a normal (0, 1) distribution was specified. Data from patients at sites J, K and S were included.
Posterior distributions for the effectiveness parameter (change in risk of medication error) across the three study sites
Appendix 14 shows the estimated prior and posterior distributions for the effectiveness parameter of an ePrescribing system on the risk of experiencing a medication error in each of the three study sites. The three posterior mean RRs (95% credible interval) of a medication error with ePrescribing compared with paper-based prescribing at sites K, J and S were 0.97 (0.95 to 0.98), 0.82 (0.66 to 0.82) and 0.81 (0.69 to 0.90), respectively. These are very similar to the observed risks in WP2, indicating that the data have a greater influence on outcome than the rather vague elicited priors.
Converting the medication error risk reduction parameter to a harm (medication adverse event) risk reduction parameter
The analysis described above estimates a RR in terms of medication errors only. We have argued that this is equivalent to the RR that should be expected if adverse drug events were the outcome of interest. This assumption is arguably justified by the serious nature of the errors targeted in our study. To reiterate, the benefits of an ePrescribing system are:
It is, therefore, necessary to estimate the baseline risk of an ADE. For the baseline risk of a medication-related ADE, we derived a figure of 0.6% (p = 0.006). This was determined using information from a previous systematic review93 of the nature of in-hospital AEs. In this review,93 it was reported that the median estimate of the incidence of preventable AEs was 4.5%, of which a median of 15% were medication related.
Converting adverse event risk reductions to a unit of health benefit: a quality-adjusted life-year
The last term in Equation 2 is the incremental effect of an ADE on QALYs and costs. The harm caused by an ADE leads a patient to require more medical attention, which results in increased costs and causes a reduction in health-related quality of life. We conducted a systematic review in which we searched for articles that estimated the incremental effect of an AE on patient length of stay, costs or health outcomes, including QALYs. 83 This systematic review covered all types of AE, but included a separate analysis of ADEs. Seven studies94–100 were identified that reported outcomes for ADEs. None of the studies identified in the review estimated the incremental QALY loss associated with an ADE. However, two studies101,102 reported the proportion of patients experiencing an adverse drug event classified according to the National Coordinating Council for Medication Error Reporting and Prevention’s (NCC-MERP) Index, which we reclassified into minimal harm (category E), temporary harm (category F), permanent harm (categories G and H) and death (category I). Combining the data from the two studies gave respective frequencies of 48%, 42%, 9% and 2% for the different categories.
Our approach was to estimate the incremental QALY losses associated with each of the severity categories and then derive a weighted average QALY loss associated with an ADE. This method was used in a previous study of ours85 and was proposed in the protocol. However, an amendment was made to take into account the uncertainty involved with both the duration and the severity of injury within each severity category, and to take into account patient age in terms of both remaining length of life and baseline quality of life.
To estimate the incremental QALY loss associated with an ADE, both quality-of-life weights and durations were assigned to each of the aggregated NCC-MERP categories. Given that the actual weights and durations over the time horizon of a patient’s life were unknown, probability distributions were specified on the basis of the literature. Counterfactual life expectancies are required to estimate the duration of life lost associated with a fatal, preventable ADE. One study103 does provide a summary table of such estimated values; we are not aware of any other study to do so. On the basis of the aforementioned study,103 we specified an exponential distribution with a rate parameter equal to the reciprocal of the estimated mean life expectancy of patients who experienced a fatal AE. The distribution of remaining life expectancies used to calculate disability-adjusted life-years among people who died from medication error were used to calculate disability-adjusted life-years from those who sustained a permanent disability from medication error. The assumptions in doing so are that those who suffered permanent disability would share the same potential life expectancy as those who died and that the disability weighting remained constant over the remainder of the person’s life. For temporary disability, in the absence of further information, we specified that the duration was distributed according to a gamma distribution, with a median of 3 months and with 95% of patients recovering by 1 year. In addition, for minimal harm we specified that the duration is uniformly distributed between 1 day and 1 month.
The quality-of-life weights associated with mortality were those associated with the years of lost life that the patient experiencing a preventable AE would have lived had they survived. The age groups of patients who died were provided in one previous paper. 22 The relevant quality-of-life weights were, therefore, those associated with individuals at the age at which they died up until the life expectancy that they would have had, had they not experienced a preventable AE. A sample weighted by the aforementioned age groups was taken from the Health Survey for England104 for the years in which the survey-reported, EuroQol-5 Dimensions-derived, health-related quality-of-life weights were reported (1996, 2003–6 and 2008). However, the information on the age categories discussed above provided estimates of the probability of dying from a preventable AE conditional on a patient being in a certain age group; we required the transpose, the probability of being in a certain age group given that the patient experienced a fatal preventable AE. Bayes’ theorem allows one to convert between these two probabilities if further information exists on the marginal probabilities of being in each age group and the overall probability of dying from a preventable AE. These were provided in the same study. The mean (SD) quality-of-life weight was 0.64 (0.25), from which we calculated the parameters of a beta-distribution, which is constrained to between zero and one.
For the disability categories, we used another study that estimated the global burden of AEs and estimated disability weights to estimate the total disability-adjusted life-years. 102 The weights given for temporary and permanent disability ranged from 0.05 to 0.2. We specified uniform distribution over these values for these categories. For the minimal harm category, we considered an exemplar condition: urinary tract infection. We have chosen an upper limit to the quality-of-life weight of 0.04, as reported in a previous study. 105
Calculation of the change in quality-adjusted life-years contingent on the change in relative risk of adverse events by site
Table 4 summarises the QALY loss associated with an AE across all four categories of harm, weighted by the probability of occurrence. The figure of 0.13 QALYs is the average incremental QALY loss for a person suffering from a preventable medication-related AE. We can now fill in all of the terms of our equation for expected benefit:
For example, for site S [see Work package 4, Posterior distributions for the effectiveness parameter (change in risks of medication error) across the three study sites] the QALYs increment is (1 – 0.81) × 0.006 × 0.13 = 0.00015 per inpatient, or 0.15 QALYs per 1000 admissions.
Category | AE | All | |||
---|---|---|---|---|---|
Minimal harm | Temporary disability | Permanent disability | Death | ||
Probability, posterior mean (95% CI) | 0.41 (0.37 to 0.44) | 0.46 (0.43 to 0.49) | 0.10 (0.09 to 0.12) | 0.03 (0.03 to 0.04) | |
Duration (years) | U(0.003, 0.08) | Gam(0.68, 2.34) | exp(0.32) | exp(0.32) | |
Severity (quality of life) | U(0.01, 0.04) | U(0.05, 0.2) | U(0.05, 0.2) | Beta(1.15, 0.34) | |
Incremental QALY loss, mean (95% CI) | 0.00 (0.00 to 0.00) | 0.04 (0.00 to 0.17) | 0.39 (0.01 to 1.60) | 2.34 (0.38 to 9.76) | 0.13 (0.03 to 0.51) |
To recap, we have now described the methods that we used to calculate the health benefit of an ePrescribing system in the form of potential QALY gain (or loss). That is to say, we have described how we estimated the top line in Figure 2. We now need to outline how we calculate net costs (bottom line of Figure 2). Then we can make health economic calculations given willingness to pay for a QALY.
Estimating cost
Net cost
We require net costs. The net costs are calculated as annual amortised system costs plus indirect costs.
System cost of an ePrescribing system (direct costs)
It was not possible to obtain cost information regarding the ePrescribing system at any of the study sites included in this analysis because the trusts were reluctant to divulge this information. Indeed, few reliable cost data were collected during the course of the programme. This problem was further compounded by the different specification systems requested by different hospitals, the different providers supplying the systems and the different prices charged by suppliers for the same product. This rendered it impossible to estimate the costs of a new ePrescribing system at a new hospital. However, we obtained estimated ePrescribing cost data for one NHS foundation trust in 2014. This trust had approximately 60,000 annual inpatient admissions. The total estimated costs per year for 3 years (2013/14 to 2015/16) was estimated at £1.165M, or approximately £6.40 per admission. This included £260,000 for hardware, of which £240,000 was incurred in the first year; £124,000 on software; and approximately £650,000 on labour costs, including new staff and training of existing staff.
Savings from the use of an ePrescribing system (indirect costs)
The effect of AEs on length of stay was based on Hoonhout et al. ’s study. 97 From the studies included in this systematic review, we estimated, using random-effects meta-analysis, a pooled mean increase in length of stay of 3.9 days (95% credible interval –0.3 to 8.7 days) associated with experiencing an ADE. This was 20–60% of the baseline length of stay. To translate this into cost increases, a cost per excess bed-day of £303 was used, which was taken from the NHS Reference Costs 2014/15. 106 These figures give an estimated mean cost increase of £1181 (95% credible interval –£91 to £2636) associated with an ADE. Two studies reported the estimated mean incremental cost associated with a preventable ADE, which we converted to 2015 Great British pounds: £2559 [standard deviation (SD) £932]90 and £2483 (SD £364). 97 These figures were higher than our mean length of stay-derived estimate, but within the 95% credible limit. Arguably, our estimate better reflects costs within the NHS given that bed-days are costed at the NHS tariff price. We opted for the more conservative estimate of costs on the basis that most ADEs result in only minimal or temporary harm. Therefore, the mean cost of AEs across all patients admitted is 0.006 × 1181 = £7.09. The increased risk reduction is 0.19, so cost saving per patient in site S is £1.34. This reduces the system costs cited above from £6.40 to £5.16 per admission.
Health economic analysis: headroom estimation (threshold analysis)
Why we have not used a cost per quality-adjusted life-year approach
A typical cost-effectiveness ratio is calculated by dividing the net cost by a summary measure of health benefit: QALYs in our case. However, in this case this does not make sense because we do not know the cost (among other things because it is subject to local price negotiation) while the decision-maker [the person(s) making the procurement decision] will know the price. Therefore, they will have the information that we do not have. That said, we have calculated a gain of about 0.15 QALYs per 1000 admissions (see Work package 3, Calculation of the change in quality-adjusted life-years contingent on the change in relative risk of adverse events by site). The only reference cost that we have been able to find was £6.40 per admission [see Work package 3, System cost of an ePrescribing system (direct costs)] (yielding a net cost for site S of £5.16 after taking reduced bed-days into account). This amounts to £5160 net per 1000 admissions [see Work package 3, Savings from use of ePrescribing (indirect costs)]. Therefore, an estimate of cost per QALY is £34,400 (5160/0.15). This is above the threshold prices sometimes quoted in England of £20,000 or £30,000.
What has been undertaken before when costs are very variable and why it is applicable here?
Given that we do not have a cost for an ePrescribing system [see Work package 3, System cost of an ePrescribing system (direct costs)], it is possible to ask an alternative question: at what cost would an intervention (in this case an ePrescribing system) be cost-effective? This is applicable here because we do not have the cost and the decision-maker does. It follows that if we can calculate a threshold willingness-to-pay cost, then the decision-maker can immediately see whether or not the cost is justified under the model. In effect, the cost-effectiveness equation has been re-arranged from:
to:
The decision-maker would be neutral (equipoised/indifferent) if:
Interpretation of headroom results
At the point at which system cost equals monetary value of benefits and indirect costs, there is no headroom and an increase in cost would not be justified (see Work package 3, Net cost). However, if the monetary value of the health benefit and indirect costs are higher than amortised system costs, this makes headroom. The greater the headroom, the better buy the system becomes for the purchaser; note that indirect costs are negative in this case because they result in a saving of bed-days. Note also that the more effective the intervention the greater, the benefit and the greater the savings (from a commercial point of view, the more the vendor has underpriced the ‘product’), and that the indirect costs are negative because they represent a saving owing to reduced length of stay.
Sensitivity analysis
Headroom serves as a sensitivity analysis because it allows the decision-maker to see how sensitive the analysis is to changes in cost. For example, the decision-maker might want to see what would happen if they had under-estimated maintenance or training costs, or if they wished to explore the effect of a difference in willingness-to-pay threshold for a QALY.
Limitations
The headroom method suffers from the usual (and extensive) limitation of any health economic model. It is generic because it deals with a generic issue – that which pertains when the decision-maker has better cost information that the analyst. The method has been used before to guide patient safety interventions. 85
Headroom calculation
The intention of this WP was to estimate the expected net benefits of an ePrescribing system. In the absence of cost data for the ePrescribing systems at the study sites, we opted for a ‘headroom’ approach. 81–83,85,107–111 Instead of calculating net costs over expected net benefits, the ‘headroom’ approach estimates the maximum price that a decision-maker should be willing to pay for a technology on the basis of overall expected benefits and a societal willingness-to-pay threshold. This approach is desirable in this context because it allows decision-makers to reconcile the costs that they face with the evidence on effectiveness.
For sites K, J and S, the annual expected headroom per patient was £0.68, £4.08 and £4.31, respectively. If the per-patient amortised cost of the ePrescribing system was lower than these values for the study sites, then the hospitals should invest. As previously discussed, the cost data reported in Work package 3, System cost of an ePrescribing system (Direct costs), are not necessarily generalisable to other hospitals. Those costs also cover only the first 3 years, whereas the useful life of the hardware may be longer. Nevertheless, the reported annual costs for labour and software licenses were approximately £5 per patient. The system whose costs we ascertained would be considered less cost-effective at the £20,000 threshold or even at a system cost of £5 per patient, per annum. However, at £5 per annum per patient it would be more cost-effective at a £30,000 threshold.
As described previously, the level of CDS has an impact on the effectiveness of ePrescribing in preventing an error. Given that restrictive decision support, the most effective kind, is applied preferentially to the most severe errors, the effect of ePrescribing will change over error types. This might suggest that a future direction for research in this area is to model individual error types to identify both the minimal and the optimal configurations for ePrescribing systems to make them more cost-effective.
Conclusions
The outcome for each key deliverable is shown in Table 5.
Key deliverable | Progress |
---|---|
Develop a novel framework for economic evaluations of ePrescribing systems | We developed and published a method to derive the QALY gain from a measurement of error rates.82 Our method is based on a Bayesian network. We have extended the method to calculate the expected net benefit. We used a method, the headroom approach, to deal with the very uncertain and ephemeral cost of ePrescribing systems |
Synthesise findings from WPs 1–3 and the systematic review to inform the cost-effectiveness analysis | We conducted a systematic review for articles that described the effect of AEs on resources and health outcomes. This information enabled us to model the expected costs and benefits contingent on reducing prescribing errors. The prescribing errors were estimated by updating elicited Bayesian priors in the data observed in WP2. We calculated that even a very expensive ePrescribing system would be cost-effective in the centres in which large reductions in error rates were observed in WP2 |
Provide information to decision-makers, thereby informing recommendations on the implementation of ePrescribing systems | Information from the literature was presented to decision-makers to elicit Bayesian priors. Our final synthesis shows that ePrescribing systems, when conscientiously implemented, have been used to inform decision-makers at many levels in the NHS: see Work package 4 |
In this section, we have estimated the RR of a patient experiencing a medication error with an ePrescribing system compared with that of a paper-based prescribing system. The RR is a key parameter for the larger decision model. The next stages incorporate the estimated RR with other sources of data. The RRs that we have estimated are all smaller in magnitude than that previously estimated. A recent meta-analysis comparing CPOE with paper-based prescribing estimated a pooled RR of 0.47 [95% confidence interval (CI) 0.31 to 0.71] compared with the estimates presented here of between 0.81 and 0.97. 83 However, our estimates were supported by both the prior and the data. The structured discussion that took place during the expert elicitation workshop suggested that the meta-analysis estimate may be too large for a number of reasons: the baseline rate of medication errors may have been higher in the predominantly US-based studies included in the review; the ePrescribing system may cause errors as well as prevent them; and the effectiveness of the systems may be lower in the UK. The posterior distribution differed little from the prior distribution, which suggests that the data did not contain much information to inform the estimate compared with the prior. As far as we are aware, this is the first study to use expert elicitation and Bayesian methods to estimate the effects of ePrescribing systems in hospitals.
This WP has provided an estimate of the maximum price that a decision-maker is likely to be willing to pay for an ePrescribing system at three study sites on the basis of the data collected here, previous studies and expert opinion. The costs of an ePrescribing system are unknown and will vary by hospital site on the basis of supplier, required features of the system and any negotiation. However, the model shows that systems would need to cost less than our reference system or be more effective to justify their costs. We utilised a cost per QALY threshold of £20,000 per QALY; however, guidance suggests an upper limit of £30,000 per QALY. Local decision-makers may wish to select an alternative threshold that may be influenced by other budgetary concerns.
Inter-relationship with other parts of the programme
This WP aimed to integrate and synthesise the findings from the other WPs and external findings to support decision-makers. The findings from this WP are not intended to be proscriptive, but to be informative. The key question is whether or not the evidence supports implementation given the costs and the societal willingness to pay for health benefits. The evidence reported by the other WPs cannot be used to directly address this question, hence the requirement for this further step. This WP has not addressed the question of the optimal implementation of an ePrescribing system or the factors explaining treatment effect heterogeneity. The evidence from the other WPs support these questions of implementation.
Work package 4: integration of work packages and implications for future NHS ePrescribing deployments and research
Background
This WP integrates findings from the qualitative and quantitative strands of the programme to identify the overall transformative contributions of ePrescribing systems in terms of procurement, implementation, adoption and use (see Work package 1), as well as of impact and costs (see Work package 2 and Work package 3). 112 Moreover, it integrates our empirical findings with contextual and existing research to develop an analytical framework within which implementation strategies may be evaluated in terms of their impact and transferability to make recommendations for future deployments and research efforts in this area.
Research aims
-
To provide contextual information for WPs 1–3.
-
To provide timely and tangible outputs that may be used by sites implementing and sites yet to implement systems, including a toolkit.
-
To map out potential future scenarios of procurement and implementation of these systems internationally, and make recommendations to ensure maximum returns for all stakeholders.
Methods for data collection and analysis
This integrating WP was informed by a sociotechnical approach, thereby enabling us to interlink the qualitative and quantitative components of our work. 112–116 In doing so, we have drawn on our ‘systems life cycle’ model of the ePrescribing implementation process as a theoretical framework. 22,23
This WP has involved organising a range of conferences, workshops and focus groups with relevant audiences, including those outside case study sites who had an interest in implementing, adopting and optimising ePrescribing systems.
Key findings
The key deliverables for WP4 were to:
-
set up a helpline for NHS trusts that would provide support and answers to implementation-related questions
-
organise stakeholder conferences and annual information-sharing events
-
develop a detailed typology of existing systems and an implementation and adoption toolkit to inform and support trusts implementing or yet to implement ePrescribing systems
-
provide formative feedback to participating sites
-
integrate findings and recommendations for procurement and implementation, and identify future research priorities.
Set-up of a helpline for NHS trusts to provide support and answers to implementation-related questions
An ePrescribing helpdesk was set up at the beginning of the programme that those with an interest in ePrescribing in the NHS could contact: eprescribingresearch@uhb.nhs.uk. The helpdesk received queries relating to specific ePrescribing-related issues. It was run by Professor Coleman and was available until June 2019.
In addition, a programme website was set up at the beginning of the programme as a resource and a platform for networking. The website was available until January 2019.
Organising stakeholder conferences and annual information-sharing events
We held four successful national conferences (see Appendices 15–22 for agendas and evaluation forms):
-
27 March 2012 at the International Convention Centre, Birmingham
-
17 June 2013 at The Royal Society, London
-
27 October 2014 at One Great George Street, London
-
24 February 2016 at the Royal College of General Practitioners, London.
We also organised five participatory workshops. The first workshop was held in September 2012 at the University of Birmingham and explored the development of business cases (paper published in PLOS ONE,117 see Acknowledgements, Publications). The second workshop was held in September 2013 and tackled issues surrounding infrastructure, interfacing and integration (report accessible under www.eprescribingtoolkit.com/wp-content/uploads/2020/06/Roundtable-Discussion-Report.pdf; accessed 31 July 2021). The third workshop was held in April 2014 and discussed the ePrescribing toolkit for NHS hospitals; this helped us to tailor the toolkit towards the needs of the NHS. The fourth workshop was held in September 2014 and tackled issues surrounding system procurement (published in BMJ Open,118 see Acknowledgements, Publications). A final workshop was held in February 2015 and explored the returns on investment from ePrescribing systems; the paper has been published in J Innov Health Inform119 (see Acknowledgements, Publications).
Development of a detailed typology of existing systems and an implementation and adoption toolkit to inform and support trusts implementing, or trusts yet to implement, ePrescribing systems
Parts of this section have been reproduced with permission from Lee et al. 45 This is an Open Access article distributed in accordance with the terms of the Creative Commons Attribution (CC BY 4.0) license, which permits others to distribute, remix, adapt and build upon this work, for commercial use, provided the original work is properly cited. See: https://creativecommons.org/licenses/by/4.0/. The text below includes minor additions and formatting changes to the original text.
We have developed a detailed typology of existing ePrescribing systems and the implementation journey (published in PLOS ONE37 and J R Soc Med;48 see Acknowledgements, Publications) and, based on this, developed an online implementation toolkit. The toolkit was first launched in December 2013 (see Appendix 23 for example screenshots). Feedback and usage to date has been very encouraging, with ≥ 2000 views per month from all over the world. The toolkit is constantly being expanded and is widely used by health-care organisations nationally and internationally. It can be accessed at www.eprescribingtoolkit.com (accessed 11 August 2017). The survey feedback from 49 English NHS trusts (out of 82 different trusts that were e-mailed the survey; 60% response rate) has indicated that 92% of trusts were familiar with the ePrescribing toolkit and 66% reported using it to guide their ongoing implementation efforts. The majority of ePrescribing toolkit users (85%) viewed it as a helpful resource. Implementers particularly valued the case studies describing lessons learnt from hospitals that had already implemented ePrescribing systems. Suggestions for improvement included more information about the progress of hospitals implementing systems, the names of key contacts in these sites, a list of available systems and the contact details of ePrescribing vendors. Respondents also highlighted the need for more information on optimisation and specialist prescribing.
Provide formative feedback to participating sites
Throughout our work, we have provided formative feedback to participating sites after each round of data collection (time 1, time 2 and, where applicable, time 3). This has taken the format of giving an informal presentation to implementation teams, including the preliminary anonymised findings.
Conclusions
Integration of findings, recommendations for procurement and implementation, and identification of future research priorities
Overall, it is important to keep in mind that quantitative and qualitative WPs have somewhat different foci and each have their own limitations (e.g. depth vs. breadth, controlled vs. naturalistic, causal vs. descriptive). Although the qualitative work investigated implementation, adoption and use of systems in a relatively broad sense, quantitative WPs had a much narrower focus on specific impacts (cost and safety) of systems. This allowed a broad picture of different issues, but also complicated the triangulation of findings.
Integration of findings across work packages
Overall, we found that all ePrescribing solutions have the potential to improve safety, and insights from studying implementation experiences (including stand-alone and paper systems) may still have utility, at least in relation to informing system design.
The quantitative work in WP2 has indicated that systems brought benefits relating to legibility, completeness and availability of information in all sites. The IMPACT tool measured a reduction of approximately 50% in most errors.
There was, however, no elimination of errors. The qualitative work in WP1 and WP4 gave some insights into potential reasons why this may be the case. First, systems are designed to encourage the ‘right’ behaviours in complex and unpredictable organisational environments; therefore, they are not foolproof. 116 Second, there are likely to be a range of sociotechnical reasons underlying the performance of systems and contributing to the remaining risk for error. These include technical features (e.g. usability and infrastructures), organisational implementation strategies (e.g. training and engagement), user-related factors (e.g. perceived benefits and risks, and integration with work practices) and wider strategic considerations (e.g. incentives and strategic directions). Behavioural issues, in particular, mean that there may always be a risk for errors, even with the ‘best’ technological system. Our work has also shown that the sheer scale of implementing meant that sites may have had limited time for optimisation activities and enhancing practice, at least initially. The effect of the way that data were collected, and the design of the IMPACT tool and the way information was recorded, may also have contributed to differences in reporting rates (e.g. in the VTE indicator).
Future research priorities emerging from this work
This work has identified important future research priorities that could now be taken forward to ensure that systems are realising their full potential and become part and parcel of wider digitisation of care. Our findings highlight the importance of re-conceptualising evaluation approaches of large transformative HIT initiatives, for which outcomes are difficult to identify and attribute, especially over short time frames. As this work has illustrated, quantitative approaches, such as randomised controlled trials (including stepped-wedge designs), may not be suitable because they lack flexibility and attention to context in which developments take place. Formative and summative evaluations of efforts will be central to promote learning across settings. Other priorities emerging from this work include the possibility of learning from international experiences and the commercial sector; the development of national strategies associated with stimulating implementation, adoption and system optimisation; and the need to analyse detailed system functionalities, implementation activities and associated costs.
This programme has provided significant breadth in terms of the areas and settings covered, but there is now an opportunity for more in-depth follow-on investigations. Important areas to focus on include further work into potentially adverse consequences of HIT, particularly in the early stages of implementation/adoption (e.g. parallel paper and electronic systems and the persistence of paper); work surrounding CDS functionality, particularly on achieving a balance between the number of helpful alerts and alert fatigue, and exploring the uses of local configuration and inbuilt functionality; exploring how to integrate and interface systems with existing and new applications; and ways of improving usability by tailoring systems to different contexts and uses (e.g. intensive care, accident and emergency, and paediatrics).
Implications for policy
Parts of this section have been reproduced with permission from Lee et al. 45 This is an Open Access article distributed in accordance with the terms of the Creative Commons Attribution (CC BY 4.0) license, which permits others to distribute, remix, adapt and build upon this work, for commercial use, provided the original work is properly cited. See: https://creativecommons.org/licenses/by/4.0/. The text below includes minor additions and formatting changes to the original text.
The findings of our programme have already been widely disseminated to policy-makers and have contributed significantly to the Wachter Review120 through the involvement of key programme members (AS, Asl and RL). Our findings may provide a useful context for the ongoing work surrounding the Digital Maturity Index on a national basis, as well as feed into the NHS 5-year plan121 regarding digitisation of the NHS. Given that the digital maturity work focuses mainly on issues surrounding capacity, our work surrounding practice and use of systems could complement ongoing efforts and help to explore how system optimisation activity may close the existing digital maturity gap.
Overall, our work has supported the view that a more coherent national approach to realising benefits from ePrescribing systems could be considered as implementation progresses and the range of tools to collect information will lead to exponential data growth. The move towards more sophisticated closed-loop systems that integrate prescribing, administration and dispensing, as well as increasingly empowered patients accessing their data through portals and portable devices, will accelerate these developments.
In keeping with evidence-based clinical practice, our work may helpfully feed into policy-making related to ePrescribing and is highly relevant for and transferable to other HIT implementations.
This programme shows that system optimisation and capacity-building surrounding optimisation nationally (and effective sharing of this resource) can be strategic enablers for HIT transformation. The increasing creation of data scientist posts in health care can facilitate this, and academic institutions (e.g. within the university sector) may wish to support the development of an appropriate career track for data scientists. Health-care institutions could maximise their current HIT capacity by ensuring that existing technology works more effectively through interfacing/interrogation and through innovative approaches to design/implementation/optimisation, particularly because the financial resources available to buy new systems are likely to be limited. Our work shows that investigating new ways to aggregate data from ePrescribing systems nationally may facilitate these developments.
The evidence presented as part of this research programme indicates that finding a balance between strategic direction and local involvement in decision-making is difficult to achieve, but can be facilitated through establishing national bodies, setting interoperability standards and agreeing on a limited set of national priorities that ePrescribing systems can help to address and that can then service as exemplar areas (e.g. antimicrobial stewardship).
Inter-relation with other parts of the programme
This WP has provided the overall summative element of the programme, integrating findings across qualitative and quantitative WPs, engaging a wide range of stakeholders and distilling overall implications for policy-making and implications for future research.
Patient and public involvement
Background
Our PPI strategy was designed to be an important, cross-cutting component of our work. We had lay representation at every level of the research to contribute views, questions and priorities from the perspective of health service users and the wider public. Our lead lay member, Antony Chuter, was a co-applicant on the funding application. His early input contributed to formulating and refining the research proposal and to developing the PPI strategy. He was instrumental in recruiting the members of the six-strong PPI group (patients living with long-term conditions and carers) who worked with us to deliver the programme. He remained a key PPI member throughout, having primary responsibility for managing the PPI budget, in addition to attending the programme’s steering group and programme management group meetings and contributing to the lay advisory group’s work days.
Of the recruited volunteers, two experienced lay representatives had a programme oversight role on the independent Programme Steering Committee (iPSC), which convened twice per year. In our original PPI strategy (see Appendix 24), each of the remaining four volunteers was attached to one of the four inter-related WPs that made up the programme. In response to feedback in the first evaluation of the PPI strategy in 2012, we amended the processes to give our lay representatives greater involvement in the programme and more opportunity to work collaboratively with each other as a lay advisory group. The amended processes aimed to address some lay feedback of sometimes feeling isolated as the single lay representative attached to one WP and the challenge of appreciating the work of the programme as a whole beyond that particular WP. We also found that some researchers reported a level of uncertainty among the WPs’ academic teams as to how best to make full use of their individual lay representative. Subsequently, it was agreed that the PPI volunteers would participate in the programme’s quarterly steering group meetings, usually in pairs, and also all meet as a lay advisory group twice per year to work together on specific tasks for the programme.
For example, working as a lay advisory group, PPI representatives contributed significantly to the development of the protocol and documents for a patient study that was undertaken as part of WP1. Although the main work of the programme was primarily aimed at supporting professionals to implement ePrescribing systems in hospitals, PPI members argued for directly including patients’ views on hospital ePrescribing systems to inform the programme. This PPI input led the academic research team to embed a new patient study within WP1. The study included interviews with hospital ward patients and with several patient organisations (i.e. Diabetes UK, British Heart Foundation, Anticoagulation Europe, Carers Trust, Neurological Alliance, Lifeblood, National Kidney Federation, National Childbirth Trust and Healthcare Quality Improvement). Key outputs from the lay advisory group over the course of the programme also included producing a ‘jargon buster’ or lay glossary of ePrescribing-related terms and a list of top 10 tips for researchers for successful PPI in HIT research (see Appendices 25 and 26). In addition, the lay advisory group requested and then worked on a set of lay summaries of all of the programme’s academic publications (see Appendix 27). These lay summaries were then circulated to the patient organisations of the hospital trusts that had been participating in the programme and, along with the lay glossary and PPI tips, they are publicly available at www.ed.ac.uk/usher/eprescribing-antimicrobial/publications-and-research-outputs/publications-from-previous-eprescribing-programme (accessed 28 January 2019).
A retained component of the original PPI strategy was to have a designated, part-time staff member who had the role of supporting the lay co-applicant and the PPI volunteers; liaising between lay and staff team members; and, throughout the programme, undertaking a series of interviews and reviews of meetings’ minutes to continually assess the PPI processes and outcomes. Evaluation of the programme’s PPI was considered an important element of the PPI strategy (see Report Supplementary Material 1).
In 2013, a second round of PPI evaluation interviews found that the revised PPI processes were deemed more successful by both staff and lay team members. Therefore, we continued to have lay participation in steering group meetings and lay advisory group workdays between 2013 and the close of the programme.
We enjoyed a high level of commitment from and retention of our lay volunteers, despite the programme lasting for 5 years. Only one of the recruited PPI members left (owing to ill health) and was replaced in that time. We believe that this retention record was, among other factors, in large part attributable to strong commitment to PPI from the principal investigators heading up the programme.
All of the programme’s PPI members were invited and funded to attend the conferences and workshops that were organised by the programme. Hence, PPI was integrated with all of the programme’s activities, at every level, from the inception of the research to its completion.
Aims
The aims of the PPI strategy, which remained unchanged for the duration of the programme, were to:
-
Conduct our academic research in the NHS with awareness of what is important from a lay perspective – whether or not what we talk about and plan to do makes sense, what is missing and whether or not the way that we present/disseminate what we have undertaken and why is comprehensible and meaningful to health service users and members of the public. This might relate in particular to the patient-/carer-facing aspects of our work, but not exclusively.
-
Undertake an ongoing evaluation of the PPI strategy to (1) address any problems and suggestions at an early stage to enhance the potential benefits of co-operation between the public and the researchers in our programme; and (2) enable us to share what we learn about PPI in health-related research and thereby contribute to current debates about PPI.
Methods
The initial PPI co-investigator was an experienced lay representative in health-related research, with whom we had worked previously. We invited him to be a co-applicant on the funding application and, thereby, have input in the development of the research proposal. The subsequent recruitment of additional PPI members drew on our lay co-applicant’s network of patient and carer contacts. Following an induction day for all of the programme’s PPI recruits (see Appendices 28 and 29), we integrated PPI in the day-to-day work of the programme by including lay representation at the grant holder and management level (n = 1), iPSC level (n = 2) and steering group level (n = 4), with, from 2013 onward, additional separate meetings of the lay advisory group that consisted of all of the lay representatives other than the iPSC lay members. We evaluated the PPI strategy by a staff member reviewing the minutes of the programme meetings and by conducting repeated rounds of semistructured, thematically analysed, audio-taped interviews between 2012 and 2016. These interviews were conducted with both lay and staff team members to assess how well or otherwise the PPI strategy was perceived to be working to pinpoint and address any problems with the strategy and to identify outputs of the programme’s PPI.
Patient and public involvement outputs
Patient and public involvement representatives saw their principal role in attending steering group meetings to be asking questions and continually bringing the academic team members’ focus back to what they perceived to be the most important reasons for doing the research, that is patient safety and the welfare of individuals who were or would become NHS patients or carers. In addition to such ‘soft’ benefits of PPI in terms of having lay perspectives constantly brought to the programme table, we were also able to identify specific outputs derived from the PPI strategy and from the lay advisory group’s work days. The key specific outcomes of PPI are summarised below:
-
instigating a patient study to be embedded in WP1 –
-
input in the design of the study protocol and documents
-
reviewing the findings of the patient study
-
-
lay summaries of the National Institute for Health and Care Research (NIHR) ePrescribing programme’s written academic outputs
-
‘10 top tips’ for researchers for successful PPI in HIT-related research
-
a ‘jargon buster’ (lay glossary) of ePrescribing-related terms
-
two submissions to the 2016 conference of the Society of Academic Primary Care, which was held in Dublin –
-
an award-winning ‘Dangerous Ideas’ submission – ‘Reorganising community pharmacy services to meet the patients’ needs’
-
poster presentation – ‘Getting PPI right: Who’s a lay patient?’
-
-
a PPI plenary, ‘Maximising the opportunity for partnership with patients, carers and members of the public’, at the NIHR ePrescribing programme’s 2016 London conference
-
an ePrescribing programme PPI-led workshop on PPI, in collaboration with the Greater Manchester Patient Safety Translational Research Centre, for delegates at the programme’s 2014 London conference
-
oral presentation – ‘The Why(s) and How(s) of PPI in the NHS’ (Wellcome Trust Symposium for the NHS, Edinburgh, 2013)
-
oral presentation – ‘Health Research PPI. Comparing Some Different Approaches’ (Society of Academic Primary Care, 2013)
-
poster presentation – ‘A Lay Partner’s Experience of Patient and Public Involvement in Health Research’ (ePrescribing Symposium, London, 2013)
-
PPI input to the development of the ePrescribing implementation toolkit and of the programme’s website –
-
overall ‘sense checking’ of the implementation toolkit
-
preparation of a PPI section in the toolkit
-
review of the frequently asked questions section
-
-
preparation of a journal article drawing on lessons learned from this Programme – ‘How to do HIT-related PPI’ (for submission to BMJ Open)
-
preparation of a journal article – ‘What is lay?’ (for submission to Res Involv Engagem).
Evaluation
Two major reviews of the available evidence on PPI in research122,123 reported that evaluating PPI was methodologically challenging and hampered by the complexity and wide variation in what constituted PPI. It has been argued that any evaluation of PPI could take account of the particular context and processes of that PPI activity. 124,125 The PPI literature indicates that, although PPI has become strongly advocated by the government and major research funding bodies, its evaluation often relies on anecdotal evidence of perceived benefits. 126–128 However, there is relatively scant reporting of health research PPI in the literature, and this is particularly so for HIT-related research. Our proposed approach was to base the evaluation of our programme’s PPI on PPI purpose + process + context = outcome(s) (Box 1).
Purpose: to conduct our programme with awareness of what is important from a lay perspective – what is missing, whether or not what we talk about and plan to do makes sense, and whether or not how we present/disseminate what we are doing and why is meaningful and comprehensible. This might particularly relate to the patient-/carer-facing aspects of our work, but not exclusively.
Process: we have three streams in the lay representation: (1) a collaborator/grant holder, (2) two ‘independent’ members contributing to iPSC meetings and (3) four PPI members working as a lay advisory group and taking part in steering meetings.
Context: the context is one of learning, in a time when PPI is increasingly widely advocated, about positive and negative consequences of PPI in academic research and how these might be maximised and minimised, respectively. In addition, we are fulfilling our commitment to the PPI strategy in the context of having received substantial public funding for this innovative aspect of our programme.
Outcome: evaluation relates to both process and outcome(s). An example of an outcome would be a specific instance where PPI input led to an identifiable consequence, for example changing the PPI process itself, influencing a research-related decision or re-wording the content of a programme-related document.
Key findings
The first semistructured evaluation interviews (see Report Supplementary Material 1) in 2012 (lay interviewees, n = 5; staff, n = 5) provided very useful feedback on aspects of the processes of the original PPI strategy and indicated areas in which some improvements could be made (Box 2). Specifically, the initial evaluation highlighted opportunities for modifying PPI processes to help clarify the lay representatives’ roles for lay and staff team members alike.
I have been made to feel very welcome and I do feel very supported, but I am still struggling to find a place, you know, a niche, where I feel that I am actually being helpful.
Lay interviewee, 2012
I suppose one of the most fundamental problems is that we don’t really know what we want, what exactly we want, from them because we’re so used to not having them.
Staff interviewee, 2012, bold emphasis added by authors
The initial evaluation also showed early, perceived outcomes of the PPI strategy, with the interesting dimension that those outcomes were identified by programme staff rather than by PPI members. Instances where PPI input led to reported outcomes, that is specific positive or negative consequences of PPI, were identified under the headings of ‘helping staff to reflect and reconsider’ (e.g. changing document wording), ‘raising staff team members’ awareness of the way they communicate’ (e.g. modification of academics’ language in meetings) and ‘opening researchers’ eyes to a lay perspective’ (e.g. challenging staff perspectives). These might all be viewed as positive outcomes. Some staff interviewees were also able to identify specific consequences with respect to PPI creating more work for them, which was viewed as a negative outcome. The key findings then were:
-
Getting the right processes in place for optimum PPI in a large-scale, mixed-methods investigation into health-related technology takes time and requires flexibility in the PPI strategy.
-
The programme’s lay volunteers and staff team members recognise that PPI is a learning process and remain actively committed to making PPI as beneficial to the ongoing work as possible.
-
Academic investigators perceive more benefits from having PPI to date than the lay representatives. Currently, the ‘reward’ for the PPI members lies in personally finding it interesting to learn about the programme, but what they want is to know that they are being genuinely useful.
-
The reported PPI benefits can come at a personal cost to academics in terms of additional work and effort from them.
The following round of evaluation interviews that took place in 2013 (lay interviewees, n = 5; staff, n = 5) found that, overall, the revised PPI strategy was working far better for both staff and lay team members (Box 3). Based on the higher levels of satisfaction reported in 2013 and in subsequent years, we made no further changes to the PPI processes during the programme. Lay members increasingly felt that they were a useful part of the team, and staff members increasingly valued the influence and input of the PPI members at steering meetings and at the programme’s conferences and workshops.
When I was first involved with this project I couldn’t really see what role I could play. Then the first conference we had, we had that talk from someone from the Patient Safety Agency about how many people die and are made worse and whatever through prescribing errors, and then I realised what the PPI involvement was. Once you realise how much ePrescribing could change that, it’s really important.
Lay interviewee, 2013
Certainly in the patient study just some of the organisations they suggested in terms of the charities to contact and so on, and sort of making sure that when we’re thinking about the patient we’re not just thinking about the patient but we’re also thinking about the carer, so there were those sorts of things and that’s been really, really helpful.
Staff interviewee, 2013
By the time of the final round of evaluation interviews in 2016 (lay interviewees, n = 5; staff, n = 6), we were able to trace attitude shifts in some but not all of the interviewees. Changes in team members meant that it was not always possible to re-interview the same individuals throughout the whole programme, but we did so as far as was possible. Among the repeatedly interviewed research staff, some started and remained enthusiastically committed to PPI and to learning from this programme to ‘do’ PPI even better in the future. Others were somewhat ambivalent about the usefulness of PPI at the beginning and clearly shifted towards greater recognition of its worth as the programme progressed. A few members of the staff team appeared to start out and to remain somewhat ambivalent about PPI.
The lay representatives, by contrast, became universally convinced of the importance of involving patients and carers in HIT-related research after some initial, personal uncertainty about their usefulness on the ePrescribing team. This was because they saw beyond the technology itself to the potential impacts of using HIT systems in hospitals. They envisaged implementation of the ePrescribing system leading to improved medication safety for patients, to more convenient and efficient care processes for patients, their carers and hospital staff, and to cost savings to the NHS, all of which were deemed highly relevant to NHS patients, carers and taxpayers.
Evaluation interviewees in 2015 were asked to identify the most notable impact of PPI over the course of the programme. Staff interviewees repeatedly referred to the ‘soft’ but valued influence of the lay members in keeping the researchers ‘grounded’ and aware of NHS patients’ perspectives (Box 4) and, more specifically, to the introduction of an additional patient study to the original programme’s scope. However, it was acknowledged that, although our PPI strategy was deemed successful and well resourced, PPI had sometimes felt challenging. Interviewees suggested that this was because of the nature of the research topic, which was not specific to any particular health condition and, therefore, not closely aligned to a clearly identifiable patient group. Other perceived PPI challenges reported by interviewees related to the very different types of work involved in a complex, long research programme and, particularly, to the large size of the academic team and its geographical dispersal.
As a carer and patient, I can see a future where every person will have a secure app on their phone or tablet that holds all the information about their medical and drugs history. When a person goes into hospital, anywhere in the country, the medical staff will be able to have instant access to the information . . . This idea may be a long way down the road but ePrescribing in hospitals is, I feel, on the way to starting to achieve this vision.
Lay interviewee 1, 2015
ePrescribing matters to carers like me because it offers more control over the supply of medicines.
Lay interviewee 2, 2015
Electronic links to community pharmacy would make life much easier for patients and carers. It should be safer for patients and it could help speed up discharge from hospital.
Lay interviewee 3, 2015
My experience at the moment is that paper-based information is slow to move between departments, gets mislaid, lost or doesn’t turn up on time. I hope ePrescribing will lead to instant and shared access. Then people seeing different consultants for different conditions, or different elements of one condition, don’t have to be totally responsible for passing information from one person to another. Eventually, all that information will be able to be accessed electronically by individual consultants and the GP.
Lay interviewee 4, 2015
A further challenge highlighted in the final interviews was how well the university sector in general was geared up to deal with the bureaucratic administration necessary to support research PPI, for instance organisations’ processes for making honoraria payments to PPI representatives attached to academic research teams.
Staff and lay interviewees cited good interpersonal communication and genuine commitment to PPI from the very top of the research team as the most critical factors underpinning successful PPI; overall, this programme’s PPI was considered successful (Box 5). An improvement for the future would be to increase the emphasis on the PPI inductions and to include the academics, not only the PPI recruits. A joint induction would allow staff and lay team members to meet face to face and jointly to discuss expectations and hopes, and to refine roles at the start of a project, and might also help foster open, constructive dialogue for its duration.
If research funders and universities are genuine about PPI involvement then there are certain kind of organisational things that they might need to look at, just to make sure it works out with the kind of research and PPI member relationship.
Staff interviewee 5, 2016
I think probably a bit more inductive work up front and maybe sort of a bit more quality time together where issues could be raised and discussed . . . Overall all I found it fun, I valued the input and sometimes it is a bit of a juggling act but I’ve certainly valued it.
Staff interviewee 6, 2016
Discussion
Other authors have noted the importance of attending to process and context in attempting to evaluate PPI. 122 Previous experiences, personal attitudes and individual academics’ skills and capabilities with respect to engaging with PPI form an important part of the context in which research PPI can function well or less well. Beyond individual factors, organisational structures and processes contribute to the context. The various contextual factors will influence a range of PPI activities taking place within a given institution over time, and potentially negatively if problems are not identified and addressed in a timely manner. We have highlighted two areas in the context of our PPI that might perhaps be improved in future PPI undertakings. When the academic research team is large and often physically working long distances apart, greater thought could be given to creating an induction at the start of the work to include both staff and lay representatives. Both may have misunderstandings of what is and what is not possible for PPI to achieve, and both could benefit from candid debate, greater support and possibly some training if wanted. At the institutional level, universities in general could consider reviewing their PPI-related processes. Embedding simpler and streamlined channels for servicing the needs of PPI representatives recruited to their organisations would help to support a positive context for PPI work.
We also identified some problems with the processes of our early PPI strategy, which we addressed in response to evaluation feedback at the end of the first year. This highlighted the need to approach planned PPI strategies with flexibility and for evaluation if reasonably nimble adjustments are to be made if the need arises.
We found that HIT-related PPI in most respects proved very similar to other health-related PPI. Lay representatives for HIT research do not need to be technology experts: they understand that HIT systems, and how these are deployed by the NHS, will effect patients and carers and, for this reason, clearly see themselves as stakeholders in HIT-related research. On the other hand, researchers may be slower to see patients and carers as HIT stakeholders, for example, compared with patients with a particular health condition who are more readily recognised as stakeholders in research programmes related to that condition. Nonetheless, it was our experience in a large and complex programme that aspects of the work were more amenable to PPI than others. For instance, the patient study benefited more obviously from PPI input than the health economics work. With hindsight, we could perhaps have increased the potential benefits of having PPI by ensuring that all of the study protocols within the programme included PPI from the very beginning; however, practical implications in terms of finding additional time to do so and of not overburdening part-time volunteers needs to be considered.
The other main findings from evaluating the programme’s PPI strategy included the importance to lay representatives of enthusiastic engagement with PPI by the team leader, the value of recruiting a mix of more experienced and less experienced PPI members (who bring different perspectives and learn from each other), the need to bring in PPI right at the start and the usefulness of having a named staff member specifically for PPI. These all are common to PPI findings reported in other areas. 123 For this reason, and given the very different contexts and processes that are happening under the umbrella of PPI, HIT-related PPI does not appear to be clearly distinguishable.
Patient and public involvement was found to be a valuable asset in this instance of HIT-related research.
What was successful and not successful in the programme?
The success of the programme can be demonstrated through the meeting of its deliverables, the publication of 32 papers and the demonstrable impact of our results. These successes were achieved despite a number of challenges being encountered during the programme.
The key limitation relates to several implementation delays within the hospital sites, which meant that we were unable to employ the stepped-wedge design as originally planned. The delays also impaired our ability to assess the longer-term consequences of ePrescribing systems. Therefore, although WP1 was designed to collect data at different moments in time, given the time limitations of the programme we were unable to follow-up developments over sufficient time periods required to understand the longer-term consequences of ePrescribing systems. Such extended study could potentially have provided insights into ways that sites addressed some of the challenges and ways in which systems were optimised.
We set out to identify the complexity of implementing an ePrescribing solution in a small number of contrasting environments. Although we provided some assessments of the impact on errors, including its financial implications, the small number of sites, the extensive limitations and the lack of existing information to support better understanding meant that the findings need to be interpreted with caution.
As with all qualitative research, WPs 1 and 4 were based on perceptions, perspectives and experiences, and the insights obtained are based on participant experiences. Therefore, some of the findings are subjective, such as the definition and constitution of workarounds, changes to work routines of individuals or the emergence of new safety threats. To address issues of the reliability and validity of our findings, we triangulated our findings across multiple sets of data from different sites. Returning to the same settings at different times in WP1 allowed us to test and validate our findings with our interview partners. By adopting established approaches in social sciences, we sought to ensure that our findings could be applied beyond the sites under investigation.
The need for a patient study was identified in the course of the research, and encouraged and guided by our patient engagement work. Methodologically, directly assessing patient perceptions of long-term changes in technology and working practices required surveying patients with chronic conditions: renal patients were identified as a suitable group and were available for study. It is possible that other disease groups had different perceptions. This could be assessed by further study encompassing a range of conditions.
This work was grounded in the context of NHS England. Hence, careful considerations are especially required to generalise these findings to other UK and non-UK settings and contexts, as our work has highlighted particular issues arising when systems that originated in one national context are applied elsewhere. More systematic cross-national research is needed into increasingly globalised system development and implementation to give insight into market adaptation and systems adoption.
A further important limitation of this study was that we have reported on the young and still yet growing ePrescribing market, which means that, as the systems mature, new impinging factors may unfold. For example, other currently unknown factors may emerge to influence the growth of these systems. We note the continued dynamism of HIT applications that may further shape the field, including extension in technical functionalities and increasing integration between applications. This highlights the need to continue to independently evaluate this rapidly evolving landscape. We have provided a starting point for this work.
We used a novel tool to facilitate the approach to data collection and to allow for a comparison of error rates between sites. Despite the fact that the tool was tested for usability by an independent pharmacist to the study team, the IMPACT tool is not fully independently validated for sensitivity and specificity in identifying opportunities for medication errors. We also used a convenience sampling approach and, therefore, none of the specific sites, wards or patients may be fully representative of the general hospital inpatient population in every setting. It would have been preferable to standardise patients selected for inclusion, which was not possible owing to the variation in time of CPOE implementation across sites. Finally, we concentrated only on defined high-risk prescribing errors and, therefore, the investigation of any new errors (introduced as part of system implementation) or other error types (rare or non-standard errors) was not carried out. This would have required analysis of all prescription data generated, along with comprehensive care note review and monitoring of local patient safety incidents. Our approach, which aimed to be both feasible and objective, could be supplemented in future pre-/post-implementation studies if a more comprehensive overview of benefits and harms of system implementation is required. Although several studies have measured the benefits of ePrescribing as a reduction in medication errors,42,56 to our knowledge this is the first study of its kind to compare ePrescribing systems with a standardised set of measures of serious and commonly occurring medication errors in inpatient settings. Systematic reviews have reported wide variability in reductions in error rates, between 13% and 99%, but with wide and variable medication error types. 55 Measuring a fixed set of medication errors has enabled CPOE systems and the extent of CDS to be measured and compared directly. Thus, our study has been able to highlight the different levels of CDS and the impact that this has had on medication error rates. Our original aim to establish whether or not there is a difference between non-alerting CPOE systems and more advanced alerting CDS systems proved impossible because both systems implemented some level of CDS within their ePrescribing systems.
The three hospitals implemented two different ePrescribing systems. Differences between the systems and their local implementation may have had differing impacts on local hospital practices and error rates. 42 However, the tool that was used provided systematic objective data. Moreover, the data analysis was specifically able to explore the effect of levels of decision support and other factors potentially moderating the impacts of CDS across the three sites. Although sites K and S had different ePrescribing systems, the study highlighted similarities in the specific CDS support that was switched on. Wider aspects of the configuration, implementation and culture of each hospital may have influenced the outcomes. Sites J and K implemented the same system, but with very different results. This was largely a result of an increase in the frequency of errors for which no CDS had been put in place by site K. The increased error rate may be as a result of suboptimal or insufficient training provided to staff post implementation of CPOE and for newer staff inducted at the hospital, the provision of which is essential for minimising unintended effects of the technology. Errors relating to the prescribing of insulin in particular were identified as problematic post CPOE. This may have reflected an overdependence on the capability of the system, with prescribers having a false expectation that the system will alert them to all types of error and, for this reason, inadvertently generating prescriptions that are inaccurate or suboptimal.
A further limitation was that a convenience sampling approach was used to select patents for inclusion in the study. As a result, the selection of patients may not be fully representative of the overall general medicine inpatient population in each setting and may have led to differences in error rates between sites pre implementation. Ideally, it would have been preferable to standardise patient selection across the sites based on the day of the week and time of day, and by randomising the patients selected for inclusion. However, this was not logistically possible in this study owing to the variation in the time of CPOE implementation across sites. Information relating to the ward and prescriber were not recorded in impact. This ensured that any patient data collected (i.e. age) would remain non-identifiable and adhered to the information governance processes at each hospital site. In view of this, it was not possible to account for the impact of clustering by these factors in the analysis. Finally, we made an assumption that there was statistical independence of errors within patients, which may not be true.
The health economic analyses conducted in this study were limited by the quantity and quality of the data. Cost data were also not obtained from the study sites; hospitals were generally unwilling to provide such information. The headroom method that we have employed in this study proved useful in such a situation and we recommend it as a useful tool in future research. Future evaluations may also try to negotiate collection of anonymised cost data from participating study sites. A further limitation of our economic models arises from the possibility that the ePrescribing systems may have introduced hazards not picked up in the quantitative data. Furthermore, there is evidence of such risks in the qualitative data (WP1). It is possible that the need for ‘workarounds’ (reported in Work package 1, Key findings) distracted staff and, hence, that errors, for example diagnostic errors, may arise. Such an error may have serious consequences if not picked up in the model. It would be possible to specify and elicit a ‘prior’ probability distribution for putative harms, but such a distribution is likely to have a very wide variance. One point that we can make is that any likely negative effects of ePrescribing systems are not fixed because HIT systems are subject to repeated upgrading/refinement. One may, therefore, expect that the hazards introduced when systems are first implemented are likely to attenuate over time.
Although we aimed to reach the widest audience possible within the constraints of the programme, some stakeholder groups may have been under-represented. This is because we necessarily had to limit the number of active workshop participants to maintain a focused exchange of experiences. This risk could have been mitigated, however, by allowing feedback through our conferences (which were open to the public) and to invite feedback through our programme website and toolkit. Other limitations within individual workshops relate to the nature of qualitative research (which guided data collection and analysis activity), including the fact that the in-depth nature of insights obtained may have compromised breadth, and that participants’ accounts were necessarily shaped by our own assumptions and preconceptions as researchers. We attempted to mitigate this risk by feeding back results to individual participants before wider dissemination. We acknowledge that the toolkit may be seen as lacking theoretical foundations because it is focused on a particular stakeholder perspective and audience (i.e. implementers in NHS organisations). It, therefore, needs to be viewed as a specific output of the work that is, although based on theoretically informed data collection (WP1), intended to have primarily practical relevance and real-world applicability. We recognise that the toolkit could be tailored more specifically to various stakeholder groups, but this was outside the scope of our work and could usefully inform future efforts in refining the toolkit.
The programme ultimately involved research staff from eight universities, one NHS hospital trust and one overseas partner, with seven patient and public representatives, seven professors, 15 research staff, four administrators and one programme manager. Given the complexity of the programme and the number of people and institutions involved, care was required to ensure that progress and deliverables were kept on track. Operational control of the programme was, therefore, managed through monthly meetings to which all staff and PPI members were invited. These were supplemented by WP-specific meetings that fed into the monthly meetings. To manage the strategic direction of the programme and to ensure good cross-fertilisation between the WPs, quarterly day-long meetings were also held, rotating between Birmingham and Edinburgh.
The programme ran for 66 months and there was inevitably movement among the research staff, such that only one of the original researchers was still involved in the programme at the end and a further five researchers were appointed during that time. This was particularly challenging given the importance of the relationships that had to be built and maintained with the study sites over the course of 5 years. The impact of staff turnover was mitigated through the development of common job descriptions to ensure that, for example, all WP1 staff could cover for one another in the case of absence and the development of rigorous study documentation and an induction system whereby new staff could rapidly pick up studies that had already started. Despite this, the inevitable hiatus caused by staff turnover, particularly when dealing with complex qualitative data, should not be underestimated.
A key challenge in running this programme was the amount and variety of work in ensuring the regulatory sign-off for each element of each WP. We were advised early in the development of the programme that, as this was an evaluation, NHS ethics approval would not be required. However, given that protocols had not yet been written for each WP, advice was sought from the scientific advisor of the South East Scotland Ethics Committee for each protocol written. For all but one protocol (the WP1 patient study see Work package 1, The patient study: data collection and analysis), we were advised to seek university rather than NHS approval. In most cases, this institutional approval was relatively straightforward. However, we did initially encounter difficulty in getting approval for the observational work in WP1. In the interests of expediency, we, therefore, developed two protocols for this work: one for the interviews that we were able to proceed with and one for the observational work that required further work to satisfy the institutional approval panel. By contrast, the NHS approval for the WP1 patient study proceeded without an issue. An associated issue was NHS sign-off of each of the protocols within each site. Given that we did not require NHS approval for most of the protocols, there was no associated application to submit to the relevant NHS research and development department for sign-off. For each of the sites, we were then advised to contact local quality improvement teams for the relevant governance procedures within each of the trusts. In some trusts, this was effected relatively quickly within the space of 2 weeks, but, in most, there did not seem to be a clear pathway to gaining this approval, which occasionally required sending the protocol and associated paperwork to four different staff members before adequate sign-off was received. Related to this was the varying approach to transferring anonymised data from sites J, K and S to the University Hospitals Birmingham NHS Trust for analysis. Each of these sites had a completely different mechanism for agreeing sign-off of this data transfer, which ranged from a simple one-page data request form from one trust to a negotiated agreement on a University Hospitals Birmingham template from one trust, which took 4 months to sign-off the data-sharing agreement.
The impact of these challenges were planned for and minimised using project management methodology, including a rigorous risk management scheme that involved a monthly assessment of the risks and escalation to the co-directors as necessary. We were also fortunate that, as a result of savings in researcher salaries, we were able to appoint an experienced 0.4 whole-time equivalent research manager who was able to manage many of these complexities on behalf of the applicants.
Recommendations for future research
There are many new questions that are raised by our research, as well as clear areas that require further explanations/investigation. In general, we can divide areas for future research into the following areas: implementation and effectiveness research, system optimisation issues, tacking specific health-care challenges, secondary use of data to improve population use of medicines, and other (including ePrescribing and patient involvement).
Implementation and effectiveness research
There are many areas of research that are within the scope of our programme of work, but that we have been unable to explore in sufficient detail:
-
We discovered that there is limited knowledge transfer not just within England, but more widely within the UK and internationally. We would, therefore, suggest that more systematic cross-national research is required into increasingly globalised system development and implementation to give insight into market adaptation and systems adoption. This could, for instance, include comparative work relating to particular ePrescribing systems.
-
In terms of prescribing error research, wider-scale use of error reporting tools, such as IMPACT, is recommended. IMPACT has recently been updated to include newer errors commonly found in hospital settings to reflect the changing nature of drug use, and it can provide a useful benchmark for hospitals implementing ePrescribing and wider HIT interventions. We are keen to make IMPACT available via our toolkit for large-scale real-world comparative error data collection.
-
The design of future research programmes for service delivery interventions are recommended to ensure a sampling design that (1) is consistent between study sites, (2) identifies admission type and ward for each patient and (3) includes enough hospitals to reliably estimate between hospital variance in treatment effects.
System optimisation issues
Our research has proposed a system life-cycle approach to ePrescribing adoption, with a clear steer that implementation is only the starting point for further cycles of system enhancement and optimisation. Even our analysis of embedded sites has been limited; therefore, we would propose careful longitudinal study of system optimisation issues:
-
In view of our observation that there is a continued dynamism of HIT applications, including extension in technical functionalities and increasing integration between applications, there is a need to continue to independently evaluate this rapidly evolving landscape that may further shape the field. These evaluations are ideally longitudinal in nature and responsive to changing environments.
-
We are aware that effective system configuration and optimisation is important in achieving benefits in terms of quality, safety and efficiency of medicines use. It remains unclear what is the best strategy towards systems optimisation to deliver the digital maturity of systems and medicines optimisation and related activities within the NHS. A systematic evaluation of ePrescribing system optimisation approaches (including those that are looking at the usability and safety of systems in practice) is required. This could begin with systematic review activities and most usefully focus on secondary uses of data.
-
Given the differences in effectiveness that we and others have observed even when the same system is implemented in different sites, comparative analysis of systems use in practice is warranted. We would promote the use of tools similar to Leapfrog (The Leapfrog Group, Washington, DC, USA) (used in the USA) as a simulation tool to ensure that systems are optimally configured to address known usability and safety issues in ePrescribing.
Tackling specific health-care challenges
We have taken a general approach to our research, focusing in particular on adult inpatient hospital care; however, there are many patient groups, situations or health-care challenges that demand further study. In particular:
-
We would like to extend the scope of ePrescribing research to involved specialist systems. Extending research to study the effectiveness and cost-effectiveness of niche clinical areas implementing ePrescribing, such as critical care, paediatrics and obstetrics, may show a different effect to that which we have described.
-
Specific health-care challenges may be amenable to specific interventions that utilise ePrescribing, together with behavioural change to improve processes of care (e.g. we have published a systematic review on antimicrobial stewardship interventions in electronic systems). We commend further evaluation of interventions that may drive benefits to the NHS from specific challenges that relate to specific challenges and/or specialties (e.g. cancer and diabetes mellitus).
Secondary use of data to improve population use of medicines
The secondary use of data to drive quality improvements is in some ways part of systems optimisation; however, this is such an important issue that we believe that it would benefit from a research stream in its own right:
-
We noted the early impacts of trust’s secondary uses of ePrescribing data to drive quality and safety initiatives at an institutional level, particularly within our embedded case study sites. The evidence behind effectiveness of medicines optimisation approaches at a population level using hospital ePrescribing data deserves further evaluation. Particular areas that are relevant in the current climate are strategies to improve efficiency and reduce waste in medicines use in hospitals.
-
In addition, we are keen to explore the key data science strategies in terms of medicines (getting information out and using it) in more detail to drive evidence-based informatics approaches. This needs to include exploring artificial intelligence and machine learning based approaches and can be promoted by closer collaboration with industry.
Other issues
Inevitably, there are a few areas that do not fall neatly within the above areas, but are nevertheless important potential areas for future research:
-
We noted from our patient study that the use of systems has the potential to change the way that we care for patients and how patients interact with their medication-related data. Further work may focus on how patients access information about their medication and how systems can not only provide CDS for practitioners but also allow patients to provide input for their decisions alongside the technical infrastructure of the ePrescribing systems. In the era of person-centred care, such study is important to avoid cutting the patient out of decisions about their medicines.
-
We found it very difficult to extract data on the costs of these systems from hospitals owing to commercial sensitivities. We considered resorting to freedom-of-information requests, but were concerned that this was likely to have alienated our case study sites. Now that this work has been completed, there is scope to see if information on costs can be extracted in this way.
-
It was clear that there was reluctance to deploy or fully deploy the computer decision support functions on ePrescribing systems. Such error-trapping solutions are reported to be effective in the literature and have the potential to reduce medication error, arguably to a greater extent than any other particular intervention; the barrier to use seems to lie in a fear of information overload and alert fatigue. More research is, thus, urgently required in how to optimise feedback in real time from IT systems.
-
There is a need to formally evaluate the impact of the ePrescribing toolkit that we have produced and, if necessary, to improve this. It also needs to continue to be populated as systems are further evolved (e.g. with the anticipated move towards closed-loop prescribing systems).
-
Finally, our research has recognised that ePrescribing and CDS systems are only a relatively small part of a much larger technical infrastructure that involve medication use in the hospital environment. As the NHS moves towards a more integrated view of digital medicines management, it is imperative that the integration of ePrescribing with other supporting technologies (e.g. smart infusion pumps) and data processes (e.g. clinical dashboards and personal health records) is also subject to further investigation.
Implications for practice
Our research findings have multiple implications directly and indirectly for the health service. The improved understanding yielded by our conceptual and methodological advances may have translational relevance for health-care practice, as well as their contribution to academic debates. The practice orientation of our applied health research generates insights around numerous avenues of ways to enhance IT delivery and related policy in the NHS. We have summarised the implications in terms of health-care professionals/personnel, hardware, software, policy/practice and data science.
Health-care professionals/personnel
Health-care managers often underestimate the effect of system implementation on short-, medium- and long-term care processes. Our work shows that, although the introduction of systems can enhance care co-ordination and improve safety, ePrescribing system implementation can change workflows, redistribute roles and have unintended and undesired outcomes. The unintended and undesired outcomes include local elaboration of new informal processes (workarounds) to manage constraints in the implemented system. Actively addressing such usability issues with clinical and system staff to eliminate any novel errors and identify opportunities for system improvement can facilitate implementation and improve safety.
We found that hospitals lacked knowledge about different aspects of the implementation journey, often including overambitious expectations of system deliverables leading to delays with procurement and challenges with implementation. Small groups of informatics specialists and seconded clinical staff played a key role in system procurement and roll-out. Staff turnover owing, for example, to their employment on contracts/secondments that were often shorter than the lengthy time scales of system implementation impeded progress. There were also longer-term consequences because system optimisation and secondary uses often rely heavily on initial implementation knowledge. At the end of a project, experienced staff often reverted to their clinical roles or general informatics functions.
In the past, doctors, nurses and pharmacists spent their education using paper-based systems to later work with EHRs, which led to consequences for their understanding of, confidence in using and acceptance of ePrescribing systems. This work has shown that the education of health-care professionals using the electronic systems that are used in clinical practice will enhance the quality of training and ultimately the safety of their future patients. Prior training in electronic systems could enhance the acceptance of incoming cohorts of health professionals and allow them to effectively exploit the full functionality of IT systems.
We noted the particular influence of senior clinical managers in the successful implementation strategies in some of the case study sites. By contrast, we saw that the scale and long time frames of implementation decisions, especially with the joint procurement, made the chain of ownership and engagement too attenuated to achieve effective engagement of senior clinical and other staff. Increasing skills to align IT with business and clinical needs to use systems to drive safety, quality and efficiency was found to be key.
Hardware
We noted difficulties in the availability and deployment of hardware and wireless connectivity in hospital practice. Although this seemed to be one of the more apparently remediable challenges, we found it to be a prerequisite of system implementation. This included enabling capabilities in terms of hardware and wireless infrastructure to cope with the demands of system use during peak times of clinical activity. As well as capital investment, we have identified the importance of revenue funding streams to provide for the upkeep, maintenance and planned replacement of hardware, as well as additional IT support.
Other considerations, such as business continuity, downtime procedures and planned maintenance, were also found to be important. Novel issues, such as cyber security, were only beginning to be discussed during our research programme, but are likely to become more important.
Software
The implementation and adoption of ePrescribing was extremely challenging and time-consuming. This was, to a large part, reflected by the relative immaturity of currently available ePrescribing system offerings, and the lack of local expertise and experience within individual hospitals in their implementation and exploitation. We have supported hospitals with system deployment through the use of the ePrescribing toolkit, but sharing of existing expertise for the deployment and optimisation of systems in tandem with the establishment of Global Digital Exemplar sites in the NHS may support the national digital agenda.
The programme has illustrated that promoting centralised support for procurement and optimisation strategies (e.g. interoperability standards, transferring data between primary care and hospitals) is key.
Given that the ePrescribing system market in the UK is still immature (with a limited number of systems and established vendors), with hospitals having limited choice of systems that are tailored to the needs of the NHS, our work has shown that hospitals need to be aware that packaged solutions may vary in maturity and are not likely to fulfil all demands of different specialties. Investing in local expertise that allows creating innovative approaches and interfacing solutions, as well as relationship building with vendors, may mitigate this risk.
We found the principle of procuring technology based on an identified need within the NHS is important throughout, and that an adequate base of suppliers with implementation experience before promoting wide adoption of new generations of technology could facilitate wider rollout. Pursuing generification strategies to create economic and robust generic solutions for the UK market, while incorporating the flexibility to cater for local specific functional requirements, has been shown to enable vendors to match the expectations of current health-care providers. We also found that packages need to incorporate a variety of care processes made available by configuring the software rather than by slow and expensive processes of user customisation requests. This is in concord with the next steps on the NHS Five Year Forward View,24,121 which suggests that hospitals will not choose an IT vendor in the future, they will choose a hospital that they want to partner with and implement the same system, keeping the IT 80% the same and making only the 20% of changes that are absolutely necessary to meet local needs.
We note that, at a hospital level, increasing financial support, efforts to study existing paper-based processes before the introduction of systems to inform whole-system (re-)design, continued stakeholder engagement, and evaluation efforts throughout the system life cycle can act as important enablers. Although desired efficiency savings may not materialise within specified time frames, we have found that in case study sites organisational vision was an important driver for change. The programme indicates that factors that are particularly important to consider going forward include the role of developing business cases considering strategic contexts, cases for change and objectives; future service requirements and options appraisal; capital and revenue implications; time scales and deliverability; and risk analysis and management.
Throughout implementation, we note that it is important to acknowledge and accept that an initial decline in organisational performance is likely, and that costs may exceed anticipated benefits. Our work shows that to ensure realisation of benefits, tracking of potentially undesired workaround and unanticipated risks is a crucial step that organisations need to take. This may include wide dissemination of risks, particularly across hospitals that are implementing the same system and also nationally.
Evidence suggests that delays in implementation timelines are par for the course. These can be owing to a range of tactical, unintended, internal (i.e. the adopting hospital) and external (i.e. suppliers, other hospitals, policy-makers) factors. User engagement can be promoted through tactically introducing clinical benefits as early in the implementation process as possible.
Our work also shows that system optimisation activity is likely to be ongoing. It consists of resolving continuity usability issues, risk mitigation strategy and refining systems for more effective use/re-uses of information. At a national level, it is likely to also include integration of care settings and refinement/updating of standards.
We have further found that integration of ePrescribing with ancillary information (contextualised demographic data) and specialty systems, and across settings (hospital, primary and social care), is an activity that is likely to result in significant benefits.
Within organisations, this work indicates that leadership is important in ensuring that continued resources are available to optimisation activities and that a dedicated optimisation team drives development forward.
Evidence from case studies illustrates that unrealistic user expectations about ease of adoption and the ability of complex packaged software to match particular organisational processes can inspire a sense of disengagement with systems that are not yet adopted. Improving the culture of use by organising appropriate training, as well as by assessing processes offered by the systems and redesigning work practices where adopted systems’ processes are more advantageous, can facilitate these developments. Our research found that the implementation of ePrescribing systems (stand-alone CPOE or integrated CDS) was associated with overall reductions in the risk of clinically important prescribing errors. We have also found that effective system configuration and optimisation is important in achieving these benefits. Given that we also found no clear advantage for the more advanced and expensive alerting system over-and-above the more basic ePrescribing system, there may be implications for how hospitals consider starting the journey to ePrescribing even if fiscal constraints do not allow for a full EHR implementation.
Policy/practice
We have shown that hospitals find that wider support in the procurement implementation and optimisation of ePrescribing systems is beneficial. The ePrescribing toolkit and the associated frameworks and tools that we have produced to help overcome some of the challenges, maximise the benefits of ePrescribing systems and minimise potential harms and associated costs may have benefits beyond the programme.
Data science
This work has illustrated that effective transfer of data within and between systems is vital for the efficient and effective operation of HIT systems. We identified that a lack of availability of timely information and duplicate data entry compounded these problems, even in integrated systems in which there was often a lack of data coherence in accessing information across modules. We note that interoperability is a key enabler of data sharing within the NHS to support the life sciences research strategy and encourage the full-scale adoption of common standards, such as Dictionary of Medicines and Devices129 and SNOMED-CT (SNOMED International, London, UK) within systems.
Data on medicines use are particularly important in relation to efficiency of health-care delivery and reduction in variation within the health service. Chronological analysis and content analysis of 207 interviews conducted in six sites over the period 2011–16130 suggests that policy-makers often failed to appreciate the length of time associated with enabling a meaningful utilisation of secondary uses of data. Most of the users reported that, although secondary uses of data were conceivable, they lacked appropriate skills and tools to extract and process data accordingly. This may suggest that there is a need to continue to track emerging benefits through longitudinal evaluations of systems and processes, as well as upskill data processing skills within the NHS workforce (including health, clinical and pharmacy informaticists).
Cross-cutting themes
Infrastructure, digital maturity and constantly evolving markets are factors that are likely to influence local and national developments over the coming years. Our work shows that anticipating to what extent effects of systems on the organisation and delivery of care can be anticipated and potential risks can be mitigated can facilitate these developments. This may include monitoring new sources of error, developing system resilience, being alert to cybersecurity threats and developing capacity in the NHS (monetary, analytic, change management) to maintain and optimise systems.
An overall shared vision of a learning health system at individual, organisational and national levels may facilitate such efforts and ensure that different stakeholders are working towards a common goal.
Concluding comments
The overarching implications that we highlight are that there is now an increasing experience around the implementation of ePrescribing and an accumulating knowledge base within sites that is captured within the toolkit. However, moving systems and organisations beyond the implementation stage towards ePrescribing system maturity and optimisation strategies will be key to maximising the benefits in terms of quality, safety and efficiency of medicines optimisation and related activities in the NHS.
Acknowledgements
The authors would like to thank staff and patients at the participating hospital sites, representatives from patient organisations, and all other informants for providing data for this programme. We would also like to thank the (anonymous) clinical staff at the WP2 sites for collecting medication error data. Our PPI panel gave essential feedback on the design of the research carried out, the tools used and the dissemination of the findings. The PPI panel included Jillian Beggs, Jon Dunster, Paul Henry, Susan Howe and Ember Vincent. We are indebted to the members of our iPSC who provided clear and supportive guidance during the 5-year programme. The iPSC was chaired by Professor Denis Protti, Professor Emeritus, University of Victoria, Canada, and included Professor Bryony Dean Franklin, Professor of Medical Safety, University College London; Ailsa Donnelly, lay member; Rosemary Humphreys, lay member; and Professor Sir Munir Pirmohamed, Professor of Molecular and Clinical Pharmacology, The University of Liverpool. We would like to thank Dawn Cattanach for creating the infographic in Figure 1 of this report. Finally, the success of the programme would not have been possible without the excellent administrative co-ordination provided by Rosemary Porteous, with additional support from Keely Dudley, Theresa Kirkpatrick, Karen MacNaughton, June Seaton and Anna Wierzoch.
Contributions of authors
Professor Aziz Sheikh (https://orcid.org/0000-0001-7022-3056) (Chairperson of Primary Care Research and Development) contributed to the overall design of the programme and specific design of individual WPs and protocols; contributed to writing the Scientific summary and Abstract; and contributed to writing the sections What was successful and not successful in the programme?, Recommendations for future research and Implications for practice.
Professor Jamie Coleman (https://orcid.org/0000-0002-7512-5153) (Professor in Clinical Pharmacology and Medical Education) contributed to the overall design of the programme and specific design of individual WPs and protocols; contributed to writing the Scientific summary and Abstract; contributed to writing Work package 2; and contributed to writing the sections What was successful and not successful in the programme?, Recommendations for future research and Implications for practice.
Mr Antony Chuter (https://orcid.org/0000-0003-0751-6758) (Lay Member) contributed to the overall design of the programme and specific design of individual WPs and protocols; contributed to writing the Plain English summary; and contributed to writing the Patient and public involvement section.
Professor Robin Williams (https://orcid.org/0000-0002-9044-4611) (Director, Institute for the Study of Science, Technology and Innovation Studies) contributed to the overall design of the programme and specific design of individual WPs and protocols; contributed to writing the Scientific summary and Abstract; contributed to writing Work package 1; and contributed to writing the sections What was successful and not successful in the programme?, Recommendations for future research and Implications for practice.
Professor Richard Lilford (https://orcid.org/0000-0002-0634-984X) (Pro-Dean, Research) contributed to the overall design of the programme and specific design of individual WPs and protocols; contributed to writing the Scientific summary and Abstract; contributed to writing Work package 3; and contributed to writing the sections What was successful and not successful in the programme?, Recommendations for future research and Implications for practice.
Ms Ann Slee (https://orcid.org/0000-0002-9731-7041) (ePrescribing Lead) contributed to the overall design of the programme and specific design of individual WPs and protocols; and contributed to writing the sections What was successful and not successful in the programme?, Recommendations for future research and Implications for practice.
Dr Zoe Morrison (https://orcid.org/0000-0003-4655-2268) (Senior Lecturer) contributed to the overall design of the programme and specific design of individual WPs and protocols; and contributed to data collection and analysis.
Dr Kathrin Cresswell (https://orcid.org/0000-0001-6634-9537) (Senior Research Fellow) contributed to the overall design of the programme and specific design of individual WPs and protocols; contributed to data collection and analysis; contributed to writing Work package 1; and wrote Work package 4.
Dr Ann Robertson (https://orcid.org/0000-0003-1077-0343) (Research Fellow) contributed to the overall design of the programme and specific design of individual WPs and protocols, and wrote the Patient and public involvement section.
Dr Sarah Slight (https://orcid.org/0000-0002-0339-846X) (Reader in Pharmacy Practice) contributed to the overall design of the programme and the specific design of the individual WPs and protocols.
Dr Hajar Mozaffar (https://orcid.org/0000-0002-6400-5260) (Lecturer in Innovation) contributed to data collection and analysis, and contributed to writing Work package 1.
Dr Lisa Lee (https://orcid.org/0000-0002-9735-9989) (Research Fellow) contributed to data collection and analysis.
Ms Sonal Shah (https://orcid.org/0000-0003-2465-9531) (Research Fellow) contributed to data collection and analysis, and contributed to writing Work package 2.
Dr Sarah Pontefract (https://orcid.org/0000-0002-0344-4075) (NIHR Doctoral Research Fellow) contributed to data collection and analysis, and contributed to writing Work package 2.
Ms Abby King (https://orcid.org/0000-0002-2212-5221) (Research Fellow) contributed to data collection and analysis, and contributed to writing Work package 1.
Dr Valeri Wiegel (https://orcid.org/0000-0002-9597-0645) (Research Fellow) contributed to data collection and analysis, and contributed to writing Work package 1.
Dr Samuel Watson (https://orcid.org/0000-0002-8972-769X) (Research Fellow) contributed to data collection and analysis, and contributed to writing Work package 3.
Dr Nde-Eshimuni Salema (https://orcid.org/0000-0002-0981-7661) (Senior Research Fellow) contributed to data collection and analysis.
Dr David Bates (https://orcid.org/0000-0001-6268-1540) (Chief Innovation Officer) contributed to the overall design of the programme.
Professor Anthony Avery (https://orcid.org/0000-0001-7591-4438) (Professor of Primary Health Care) contributed to the overall design of the programme.
Mr Alan Girling (https://orcid.org/0000-0003-2022-045X) (Reader in Medical Statistics) contributed to data collection and analysis, and contributed to writing Work package 2.
Dr Lucy McCloughan (https://orcid.org/0000-0003-4099-6183) (Research Manager) contributed to writing the Plain English summary.
Mr Neil Watson (https://orcid.org/0000-0002-5024-8456) (Director of Pharmacy) contributed to the interpretation of the results.
Publications
Yao GL, Novielli N, Manaseki-Holland S, Chen YF, van der Klink M, Barach P, et al. Evaluation of a predevelopment service delivery intervention: an application to improve clinical handovers. BMJ Qual Saf 2012;21(Suppl. 1):i29–38. https://doi.org/10.1136/bmjqs-2012-001210
Coleman JJ, van der Sijs H, Haefeli WE, Slight SP, McDowell SE, Seidling HM, et al. On the alert: future priorities for alerts in clinical decision support for computerized physician order entry identified from a European workshop. BMC Med Inform Decis Mak 2013;13:111. https://doi.org/10.1186/1472-6947-13-111
Cresswell K, Coleman J, Slee A, Williams R, Sheikh A, ePrescribing Programme Team. Investigating and learning lessons from early experiences of implementing ePrescribing systems into NHS hospitals: a questionnaire study. PLOS ONE 2013;8:e53369. https://doi.org/10.1371/journal.pone.0053369
Cresswell KM, Bates DW, Sheikh A. Ten key considerations for the successful implementation and adoption of large-scale health information technology. J Am Med Inform Assoc 2013;20:e9–e13. https://doi.org/10.1136/amiajnl-2013-001684
Cresswell KM, Slee A, Coleman J, Williams R, Bates DW, Sheikh A. Qualitative analysis of round-table discussions on the business case and procurement challenges for hospital electronic prescribing systems. PLOS ONE 2013;8:e79394. https://doi.org/10.1371/journal.pone.0079394
Thomas SK, McDowell SE, Hodson J, Nwulu U, Howard RL, Avery AJ, et al. Developing consensus on hospital prescribing indicators of potential harms amenable to decision support. Br J Clin Pharmacol 2013;76:797–809. https://doi.org/10.1111/bcp.12087
Cresswell K, Coleman J, Slee A, Morrison Z, Sheikh A. A toolkit to support the implementation of electronic prescribing systems into UK hospitals: preliminary recommendations. J R Soc Med 2014;107:8–13. https://doi.org/10.1177/0141076813502955
Cresswell KM, Bates DW, Williams R, Morrison Z, Slee A, Coleman JJ, et al. Evaluation of medium-term consequences of implementing commercial computerized physician order entry and clinical decision support prescribing systems in two ‘early adopter’ hospitals. J Am Med Inform Assoc 2014;21:e194–202. https://doi.org/10.14236/jhi.v23i2.178
Cresswell KM, Sheikh A. Undertaking sociotechnical evaluations of health information technologies. Inform Prim Care 2014;21:78–83. https://doi.org/10.14236/jhi.v21i2.54
Lee L, Cresswell K, Slee A, Slight SP, Coleman J, Sheikh A. Using stakeholder perspectives to develop an ePrescribing toolkit for NHS Hospitals: a questionnaire study. JRSM Open 2014;5:2054270414551658. https://doi.org/10.1177/2054270414551658
Lilford RJ, Girling AJ, Sheikh A, Coleman JJ, Chilton PJ, Burn SL, et al. Protocol for evaluation of the cost-effectiveness of ePrescribing systems and candidate prototype for other related health information technologies. BMC Health Serv Res 2014;14:314. https://doi.org/10.1186/1472-6963-14-314
Mozaffar H, Williams R, Cresswell K, Morison Z, Slee A, Sheikh A, ePrescribing Programme. Product diversity and spectrum of choice in hospital ePrescribing Systems in England. PLOS ONE 2014;9:e92516. https://doi.org/10.1371/journal.pone.0092516
Cresswell KM, Lee L, Slee A, Coleman J, Bates DW, Sheikh A. Qualitative analysis of vendor discussions on the procurement of Computerised Physician Order Entry and Clinical Decision Support systems in hospitals. BMJ Open 2015;5:e008313. https://doi.org/10.1136/bmjopen-2015-008313
Cresswell KM, Sheikh A. Health information technology in hospitals: current issues and future trends. Future Hosp J 2015;2:50–6. https://doi.org/10.7861/futurehosp.2-1-50
Lee L, Williams R, Sheikh A. What does ePrescribing mean for patients? A case study of the perspectives of hospital renal patients. J Innov Health Inform 2015;22:391–8. https://doi.org/10.14236/jhi.v22i4.176
Schofield B, Cresswell K, Westbrook J, Slee A, Girling A, Shah S, et al. The impact of electronic prescribing systems on pharmacists’ time and workflow: protocol for a time-and-motion study in English NHS hospitals. BMJ Open 2015;5:e008785. https://doi.org/10.1136/bmjopen-2015-008785
Cresswell K. Evaluation of implementation of health IT. Stud Health Technol Inform 2016;222:206–19.
Cresswell K, Bates DW, Sheikh A. Six ways for governments to get value from health IT. Lancet 2016;387:2074–5. https://doi.org/10.1016/S0140-6736(16)30519-0
Cresswell K, Coleman J, Smith P, Swainson C, Slee A, Sheikh A. Qualitative analysis of multi-disciplinary round-table discussions on the acceleration of benefits and data analytics through hospital electronic prescribing (ePrescribing) systems. J Innov Health Inform 2016;23:178. https://doi.org/10.14236/jhi.v23i2.178
Lee L, Sheikh A. Understanding stakeholder interests and perspectives in evaluations of health IT. Stud Health Technol Inform 2016;222:53–62.
Lee L, Williams R, Sheikh A. How does joint procurement affect the design, customisation and usability of a hospital ePrescribing system? Health Inform J 2016;22:828–38. https://doi.org/10.1177/1460458215592915
Mozaffar H, Cresswell KM, Lee L, Williams R, Sheikh A, NIHR ePrescribing Programme Team. Taxonomy of delays in the implementation of hospital computerized physician order entry and clinical decision support systems for prescribing: a longitudinal qualitative study. BMC Med Inform Decis Mak 2016;16:25. https://doi.org/10.1186/s12911-016-0263-x
Mozaffar H, Williams R, Cresswell K, Morrison Z, Bates DW, Sheikh A. The evolution of the market for commercial computerized physician order entry and computerized decision support systems for prescribing. J Am Med Inform Assoc 2016;23:349–55. https://doi.org/10.1093/jamia/ocv095
Cresswell KM, Bates DW, Sheikh A. Ten key considerations for the successful optimization of large-scale health information technology. J Am Med Inform Assoc 2017;24:182–7. https://doi.org/10.1093/jamia/ocw037
Cresswell K, Mozaffar H, Shah S, Sheikh A. Approaches to promoting the appropriate use of antibiotics through hospital electronic prescribing systems: a scoping review. Int J Pharm Pract 2017;25:5–17. https://doi.org/10.1111/ijpp.12274
Cresswell K, Blandford A, Sheikh A. Drawing on human factors engineering to reconsider paradigms for evaluating the effectiveness of health information technology. J R Soc Med 2017;110:309–15. https://doi.org/10.1177/0141076817712252
Cresswell KM, Lee L, Mozaffar H, Williams R, Sheikh A, NIHR ePrescribing Programme Team. Sustained user engagement in health information technology: the long road from implementation to system optimization of computerized physician order entry and clinical decision support systems for prescribing in hospitals in England. Health Serv Res 2017;52:1928–57. https://doi.org/10.1111/1475-6773.12581
Cresswell KM, Mozaffar H, Lee L, Williams R, Sheikh A. Safety risks associated with the lack of integration and interfacing of hospital health information technologies: a qualitative study of hospital electronic prescribing systems in England. BMJ Qual Saf 2017;26:530–41. https://doi.org/10.1136/bmjqs-2015-004925
Cresswell KM, Mozaffar H, Lee L, Williams R, Sheikh A. Workarounds to hospital electronic prescribing systems: a qualitative study in English hospitals. BMJ Qual Saf 2017;26:542–51. https://doi.org/10.1136/bmjqs-2015-005149
Cresswell K, Slee A, Sheikh A. Five key recommendations for the implementation of Hospital Electronic Prescribing and Medicines Administration systems in Scotland. J Innov Health Inform 2017;23:904. https://doi.org/10.14236/jhi.v23i4.904
Mozaffar H, Cresswell KM, Williams R, Bates DW, Sheikh A. Exploring the roots of unintended safety threats associated with the introduction of hospital ePrescribing systems and candidate avoidance and/or mitigation strategies: a qualitative study. BMJ Qual Saf 2017;26:722–33. https://doi.org/10.1136/bmjqs-2016-005879
Mozaffar H, Williams R, Cresswell K, Sheikh A. Anglicization of hospital information systems: managing diversity alongside particularity. Int J Med Inform 2018;119:88–93. https://doi.org/10.1016/j.ijmedinf.2018.09.014
Wiegel V, King A, Mozaffar H, Cresswell K, Williams R, Sheikh A. A systematic analysis of the optimization of computerized physician order entry and clinical decision support systems: a qualitative study in English hospitals. Health Inform J 2019;26:1118–32. https://doi.org/10.1177/1460458219868650
Data-sharing statement
All data requests should be submitted to the corresponding author for consideration. Access to anonymised data may be granted following review.
Patient data
This work uses data provided by patients and collected by the NHS as part of their care and support. Using patient data is vital to improve health and care for everyone. There is huge potential to make better use of information from people’s patient records, to understand more about disease, develop new treatments, monitor safety, and plan NHS services. Patient data should be kept safe and secure, to protect everyone’s privacy, and it’s important that there are safeguards to make sure that it is stored and used responsibly. Everyone should be able to find out about how patient data are used. #datasaveslives You can find out more about the background to this citation here: https://understandingpatientdata.org.uk/data-citation.
Disclaimers
This report presents independent research funded by the National Institute for Health and Care Research (NIHR). The views and opinions expressed by authors in this publication are those of the authors and do not necessarily reflect those of the NHS, the NIHR, CCF, PGfAR or the Department of Health and Social Care. If there are verbatim quotations included in this publication the views and opinions expressed by the interviewees are those of the interviewees and do not necessarily reflect those of the authors, those of the NHS, the NIHR, the PGfAR programme or the Department of Health and Social Care.
References
- Muehlberger N, Schneeweiss S, Hasford J. Adverse drug reaction monitoring – cost and benefit considerations. Part I: frequency of adverse drug reactions causing hospital admissions. Pharmacoepidemiol Drug Saf 1997;6:71-7.
- Pirmohamed M, James S, Meakin S, Green C, Scott AK, Walley TJ, et al. Adverse drug reactions as cause of admission to hospital: prospective analysis of 18 820 patients. BMJ 2004;329:15-9. https://doi.org/10.1136/bmj.329.7456.15.
- Roughead EE, Gilbert AL, Primrose JG, Sansom LN. Drug-related hospital admissions: a review of Australian studies published 1988–1996. Med J Aust 1998;168:405-8. https://doi.org/10.5694/j.1326-5377.1998.tb138996.x.
- Huckvale C, Car J, Akiyama M, Jaafar S, Khoja T, Khalid AB, et al. Information technology for patient safety. Qual Saf Health Care 2010;19:i25-i33. https://doi.org/10.1136/qshc.2009.038497.
- Kaushal R, Shojania KG, Bates DW. Effects of computerized physician order entry and clinical decision support systems on medication safety: a systematic review. Arch Intern Med 2003;163:1409-16. https://doi.org/10.1001/archinte.163.12.1409.
- Smith DH, Perrin N, Feldstein A, Yang X, Kuang D, Simon SR, et al. The impact of prescribing safety alerts for elderly persons in an electronic medical record. Arch Intern Med 2006;166:1098-104. https://doi.org/10.1001/archinte.166.10.1098.
- Black AD, Car J, Pagliari C, Anandan C, Cresswell K, Bokun T, et al. The impact of eHealth on the quality and safety of health care: a systematic overview. PLOS Med 2011;8. https://doi.org/10.1371/journal.pmed.1000387.
- Schade CP, Sullivan FM, de Lusignan S, Madeley J. e-Prescribing, efficiency, quality: lessons from the computerization of UK family practice. J Am Med Inform Assoc 2006;13:470-5. https://doi.org/10.1197/jamia.M2041.
- Department of Health and Social Care . An Organisation With a Memory: Report of an Expert Group on Learning From Adverse Events in the NHS 2000.
- Department of Health and Social Care (DHSC) . Building a Safer NHS for Patients: Improving Medication Safety 2004.
- Ash JS, Berg M, Coiera E. Some unintended consequences of information technology in health care: the nature of patient care information system-related errors. J Am Med Inform Assoc 2004;11:104-12. https://doi.org/10.1197/jamia.M1471.
- Fernando B, Savelyich BS, Avery AJ, Sheikh A, Bainbridge M, Horsfield P, et al. Prescribing safety features of general practice computer systems: evaluation using simulated test cases. BMJ 2004;328:1171-2. https://doi.org/10.1136/bmj.328.7449.1171.
- Koppel R, Metlay JP, Cohen A, Abaluck B, Localio AR, Kimmel SE, et al. Role of computerized physician order entry systems in facilitating medication errors. JAMA 2005;293:1197-203. https://doi.org/10.1001/jama.293.10.1197.
- Bates DW. CPOE and clinical decision support in hospitals: getting the benefits. Arch Intern Med 2010;170:1583-4. https://doi.org/10.1001/archinternmed.2010.325.
- Strom BL, Schinnar R, Aberra F, Bilker W, Hennessy S, Leonard CE, et al. Unintended effects of a computerized physician order entry nearly hard-stop alert to prevent a drug interaction: a randomized controlled trial. Arch Intern Med 2010;170:1578-83. https://doi.org/10.1001/archinternmed.2010.324.
- Harrison MI, Koppel R, Bar-Lev S. Unintended consequences of information technologies in health care – an interactive sociotechnical analysis. J Am Med Inform Assoc 2007;14:542-9. https://doi.org/10.1197/jamia.M2384.
- Ludwick DA, Doucette J. Adopting electronic medical records in primary care: lessons learned from health information systems implementation experience in seven countries. Int J Med Inform 2009;78:22-31. https://doi.org/10.1016/j.ijmedinf.2008.06.005.
- Department of Health and Social Care (DHSC) . Discharge from Hospital: Pathway, Process and Practice 2003.
- Moore C, Wisnivesky J, Williams S, McGinn T. Medical errors related to discontinuity of care from an inpatient to an outpatient setting. J Gen Intern Med 2003;18:646-51. https://doi.org/10.1046/j.1525-1497.2003.20722.x.
- NHS Connecting for Health . Electronic Prescribing in Hospitals – Challenges and Lessons Learned 2009.
- Crowe S, Cantrill JA, Tully MP. Shared care arrangements for specialist drugs in the UK: the challenges facing GP adherence. Qual Saf Health Care 2010;19. https://doi.org/10.1136/qshc.2009.035857.
- Crowe S, Tully MP, Cantrill JA. The prescribing of specialist medicines: what factors influence GPs’ decision making?. Fam Pract 2009;26:301-8. https://doi.org/10.1093/fampra/cmp030.
- NHS National Patient Safety Agency . Safety in Doses: Improving the Use of Medicines in the NHS 2009.
- Mozaffar H, Williams R, Cresswell K, Morrison Z, Bates DW, Sheikh A. The evolution of the market for commercial computerized physician order entry and computerized decision support systems for prescribing. J Am Med Inform Assoc 2016;23:349-55. https://doi.org/10.1093/jamia/ocv095.
- Cresswell KM, Sheikh A. Undertaking sociotechnical evaluations of health information technologies. Inform Prim Care 2014;21:78-83. https://doi.org/10.14236/jhi.v21i2.54.
- Crowe S, Cresswell K, Robertson A, Huby G, Avery AJ, Sheikh A. The case study approach. BMC Med Res Methodol 2011;11. https://doi.org/10.1186/1471-2288-11-100.
- Grisot M, Hanseth O, Thorseng A. Innovation of, in, on infrastructures: articulating the role of architecture in information infrastructure evolution. J Assoc Inf Syst 2014;15:197-219. https://doi.org/10.17705/1jais.00357.
- Hanseth O, Monteiro E, Hatling M. Developing information infrastructure: the tension between standardization and flexibility. Sci Technol Human Values 1996;21:407-26. https://doi.org/10.1177/016224399602100402.
- Monteiro E, Pollock N, Williams R. Innovation in Information Infrastructures: Introduction to the Special Issue n.d. https://doi.org/10.17705/1jais.00359.
- Monteiro E, Pollock N, Hanseth O, Williams R. From artefacts to infrastructures. Comput Support Coop Work 2013;22:575-607. https://doi.org/10.1007/s10606-012-9167-1.
- Pollock N, Williams R. E-infrastructures: How do we know and understand them? Strategic ethnography and the biography of artefacts. Comput Support Coop Work 2010;19:521-56. https://doi.org/10.1007/s10606-010-9129-4.
- Cresswell K, Mozaffar H, Lee L, Williams R, Sheikh A. Sustaining user engagement in health information technology initiatives: the long road from implementation to system optimization of computerized physician order entry and clinical decision support systems for prescribing in hospitals in England. Health Serv Res 2017;52:1928-57. https://doi.org/10.1111/1475-6773.12581.
- Pollock N, Williams R. Software and Organizations. New York, NY: Routledge; 2008.
- Abernethy WJ, Utterback JM. Patterns of industrial innovation. Technol Rev 1978;80:40-7.
- Patton MQ. Qualitative Evaluation and Research Methods. Thousand Oaks, CA: SAGE Publications Ltd; 2001.
- Coyne IT. Sampling in qualitative research. Purposeful and theoretical sampling; merging or clear boundaries?. J Adv Nurs 1997;26:623-30. https://doi.org/10.1046/j.1365-2648.1997.t01-25-00999.x.
- Mozaffar H, Williams R, Cresswell K, Morison Z, Slee A, Sheikh A, et al. ePrescribing Programme . Product diversity and spectrum of choice in hospital ePrescribing Systems in England. PLOS ONE 2014;9. https://doi.org/10.1371/journal.pone.0092516.
- Brennan S. The biggest computer programme in the world ever! How’s it going?. J Inf Tech 2007;22:202-11. https://doi.org/10.1057/palgrave.jit.2000104.
- Blumenthal D, Tavenner M. The ‘Meaningful Use’ regulation for electronic health records. N Engl J Med 2010;363:501-4. https://doi.org/10.1056/NEJMp1006114.
- Connor M, Day GE, Meston D. Successful linking of patient records between hospital services and general practice to facilitate integrated care in a hospital and health service in south-east Queensland. Aust Health Rev 2016;40:78-81. https://doi.org/10.1071/AH15048.
- Atkinson R, Flint J. Accessing hidden and hard-to-reach populations: snowball research strategies. Soc Res Update 2001;33:1-4.
- Mozaffar H, Cresswell KM, Lee L, Williams R, Sheikh A. NIHR ePrescribing Programme Team . Taxonomy of delays in the implementation of hospital computerized physician order entry and clinical decision support systems for prescribing: a longitudinal qualitative study. BMC Med Inform Decis Mak 2016;16. https://doi.org/10.1186/s12911-016-0263-x.
- Mays N, Pope C. Qualitative research in health care. Assessing quality in qualitative research. BMJ 2000;320:50-2. https://doi.org/10.1136/bmj.320.7226.50.
- Denzin NK, Lincoln YS. The Sage Handbook of Qualitative Research. London: SAGE Publications Ltd; 2005.
- Lee L, Williams R, Sheikh A. What does ePrescribing mean for patients? A case study of the perspectives of hospital renal patients. J Innov Health Inform 2015;22:391-8. https://doi.org/10.14236/jhi.v22i4.176.
- Mozaffar H, Cresswell KM, Lee L, Williams R, Sheikh A. NIHR ePrescribing Programme Team . Taxonomy of delays in the implementation of hospital computerized physician order entry and clinical decision support systems for prescribing: a longitudinal qualitative study. BMC Med Inform Decis Mak 2016;16. https://doi.org/10.1186/s12911-016-0263-x.
- Mozaffar H, Cresswell KM, Williams R, Bates DW, Sheikh A. Exploring the roots of unintended safety threats associated with the introduction of hospital ePrescribing systems and candidate avoidance and/or mitigation strategies: a qualitative study. BMJ Qual Saf 2017;26:722-33. https://doi.org/10.1136/bmjqs-2016-005879.
- Cresswell K, Coleman J, Slee A, Morrison Z, Sheikh A. A toolkit to support the implementation of electronic prescribing systems into UK hospitals: preliminary recommendations. J R Soc Med 2014;107:8-13. https://doi.org/10.1177/0141076813502955.
- Lee L, Cresswell K, Slee A, Slight SP, Coleman JJ, Sheikh A. Using stakeholder perspectives to develop an ePrescribing toolkit for NHS hospitals: a questionnaire study. JRSM Open 2014;5. https://doi.org/10.1177/2054270414551658.
- Cresswell KM, Bates DW, Sheikh A. Ten key considerations for the successful implementation and adoption of large-scale health information technology. J Am Med Inform Assoc 2013;20:e9-e13. https://doi.org/10.1136/amiajnl-2013-001684.
- Cresswell K, Mozaffar H, Lee L, Williams R, Sheikh A. Safety risks associated with the lack of integration and interfacing of hospital health information technologies: a qualitative study of hospital electronic prescribing systems in England. BMJ Qual Saf 2017;26:530-41. https://doi.org/10.1136/bmjqs-2015-004925.
- Cresswell KM, Lee L, Mozaffar H, Williams R, Sheikh A. NIHR ePrescribing Programme Team . Sustained user engagement in Health Information Technology: the long road from implementation to system optimization of computerized physician order entry and clinical decision support systems for prescribing in hospitals in England. Health Serv Res 2017;52:1928-57. https://doi.org/10.1111/1475-6773.12581.
- Crowe S, Cresswell K, Avery AJ, Slee A, Coleman JJ, Sheikh A. Planned implementations of ePrescribing systems in NHS hospitals in England: a questionnaire study. JRSM Short Rep 2010;1. https://doi.org/10.1258/shorts.2010.010040.
- Lee L, Williams R, Sheikh A. How does joint procurement affect the design, customisation and usability of a hospital ePrescribing system?. Health Informatics J 2016;22:828-38. https://doi.org/10.1177/1460458215592915.
- Cresswell K, Coleman J, Slee A, Williams R, Sheikh A. ePrescribing Programme Team. Investigating and learning lessons from early experiences of implementing ePrescribing systems into NHS hospitals: a questionnaire study. PLOS ONE 2013;8. https://doi.org/10.1371/journal.pone.0053369.
- Cresswell K, Mozaffar H, Lee L, Williams R, Sheikh A. Workarounds to hospital electronic prescribing systems: a qualitative study in English hospitals. BMJ Qual Saf 2017;26:542-51. https://doi.org/10.1136/bmjqs-2015-005149.
- Mozaffar H, Williams R, Cresswell K, Sheikh A. Anglicization of hospital information systems: Managing diversity alongside particularity. Int J Med Inform 2018;119:88-93. https://doi.org/10.1016/j.ijmedinf.2018.09.014.
- Cresswell KM, Bates DW, Williams R, Morrison Z, Slee A, Coleman J, et al. Evaluation of medium-term consequences of implementing commercial computerized physician order entry and clinical decision support prescribing systems in two ‘early adopter’ hospitals. J Am Med Inform Assoc 2014;21:e194-202. https://doi.org/10.1136/amiajnl-2013-002252.
- Tucker AL, Edmondson AC, Spear S. When problem solving prevents organizational learning. J Organ Chang Manag 2002;15:122-37. https://doi.org/10.1108/09534810210423008.
- Tucker AL, Edmondson AC. Why hospitals don’t learn from failures: organizational and psychological dynamics that inhibit system change. Calif Manage Rev 2003;45:55-72. https://doi.org/10.2307/41166165.
- Hewitt TA, Chreim S. Fix and forget or fix and report: a qualitative study of tensions at the front line of incident reporting. BMJ Qual Saf 2015;24:303-10. https://doi.org/10.1136/bmjqs-2014-003279.
- Vogus TJ, Sutcliffe KM, Weick KE. Doing no harm: enabling, enacting and elaborating a culture of safety in health care. Acad Manag Perspect 2010;24:60-77. https://doi.org/10.5465/amp.2010.24.4.3652485.a.
- Vogus TJ, Hilligoss B. The underappreciated role of habit in highly reliable healthcare. BMJ Qual Saf 2016;25:141-6. https://doi.org/10.1136/bmjqs-2015-004512.
- Aarts J, Koppel R. Implementation of computerized physician order entry in seven countries. Health Aff 2009;28:404-14. https://doi.org/10.1377/hlthaff.28.2.404.
- Mäkinen M, Rautava P, Forsström J, Aärimaa M. Electronic prescriptions are slowly spreading in the European Union. Telemed J E Health 2011;17:217-22. https://doi.org/10.1089/tmj.2010.0111.
- Van Dijk L, De Vries H, Bell D. Electronic Prescribing in the United Kingdom and in the Netherlands. Rockville, MD: Agency for Healthcare Research and Quality US Department of Health and Human Services; 2011.
- Schoen C, Davis K, How SK, Schoenbaum SC. US health system performance: a national scorecard. Health Aff 2006;25:w457-75. https://doi.org/10.1377/hlthaff.25.w457.
- Avery T, Barber N, Ghaleb M, Franklin BD, Armstrong S, Crowe S, et al. Investigating the Prevalence and Causes of Prescribing Errors in General Practice. London: General Medical Council; 2012.
- Thomas SK, McDowell SE, Hodson J, Nwulu U, Howard RL, Avery AJ, et al. Developing consensus on hospital prescribing indicators of potential harms amenable to decision support. Br J Clin Pharmacol 2013;76:797-809. https://doi.org/10.1111/bcp.12087.
- Avery AJ, Savelyich BS, Sheikh A, Cantrill J, Morris CJ, Fernando B, et al. Identifying and establishing consensus on the most important safety features of GP computer systems: e-Delphi study. Inform Prim Care 2005;13:3-12. https://doi.org/10.14236/jhi.v13i1.575.
- Manias E. Detection of medication related problems in hospital practice: a review. Br J Clin Pharmacol 2013;76:7-20. https://doi.org/10.1111/bcp.12049.
- Pontefract SK, Hodson J, Slee A, Shah S, Girling AJ, Williams R, et al. Impact of a commercial order entry system on prescribing errors amenable to computerised decision support in the hospital setting: a prospective pre-post study. BMJ Qual Saf 2018;27:725-36. https://doi.org/10.1136/bmjqs-2017-007135.
- Lanham HJ, Leykum LK, McDaniel RR. Same organization, same electronic health records (EHRs) system, different use: exploring the linkage between practice member communication patterns and EHR use patterns in an ambulatory care setting. J Am Med Inform Assoc 2012;19:382-91. https://doi.org/10.1136/amiajnl-2011-000263.
- Roshanov PS, Fernandes N, Wilczynski JM, Hemens BJ, You JJ, Handler SM, et al. Features of effective computerised clinical decision support systems: meta-regression of 162 randomised trials. BMJ 2013;346. https://doi.org/10.1136/bmj.f657.
- Wong A, Amato MG, Seger DL, Slight SP, Beeler PE, Dykes PC, et al. Evaluation of medication-related clinical decision support alert overrides in the intensive care unit. J Crit Care 2017;39:156-61. https://doi.org/10.1016/j.jcrc.2017.02.027.
- Jani YH, Barber N, Wong IC. Characteristics of clinical decision support alert overrides in an electronic prescribing system at a tertiary care paediatric hospital. Int J Pharm Pract 2011;19:363-6. https://doi.org/10.1111/j.2042-7174.2011.00132.x.
- Seidling HM, Phansalkar S, Seger DL, Paterno MD, Shaykevich S, Haefeli WE, et al. Factors influencing alert acceptance: a novel approach for predicting the success of clinical decision support. J Am Med Inform Assoc 2011;18:479-84. https://doi.org/10.1136/amiajnl-2010-000039.
- Phansalkar S, Edworthy J, Hellier E, Seger DL, Schedlbauer A, Avery AJ, et al. A review of human factors principles for the design and implementation of medication safety alerts in clinical information systems. J Am Med Inform Assoc 2010;17:493-501. https://doi.org/10.1136/jamia.2010.005264.
- Westbrook JI, Li L, Lehnbom EC, Baysari MT, Braithwaite J, Burke R, et al. What are incident reports telling us? A comparative study at two Australian hospitals of medication errors identified at audit, detected by staff and reported to an incident system. Int J Qual Health Care 2015;27:1-9. https://doi.org/10.1093/intqhc/mzu098.
- Lilford RJ, Chilton PJ, Hemming K, Girling AJ, Taylor CA, Barach P. Evaluating policy and service interventions: framework to guide selection and interpretation of study end points. BMJ 2010;341. https://doi.org/10.1136/bmj.c4413.
- Lilford RJ, Girling AJ, Sheikh A, Coleman JJ, Chilton PJ, Burn SL, et al. Protocol for evaluation of the cost-effectiveness of ePrescribing systems and candidate prototype for other related health information technologies. BMC Health Serv Res 2014;14. https://doi.org/10.1186/1472-6963-14-314.
- Watson S, Lilford RJ. Integrating multiple sources of evidence: a Bayesian perspective. Health Serv Deliv Res 2016;4.
- Sutton M, Garfield-Birkbeck S, Martin G, Meacock R, Morris S, Sculpher M, et al. Economic analysis of service and delivery interventions in health care. Health Serv Deliv Res 2018;6. https://doi.org/10.3310/hsdr06050.
- Karnon J, McIntosh A, Dean J, Bath P, Hutchinson A, Oakley J, et al. Modelling the expected net benefits of interventions to reduce the burden of medication errors. J Health Serv Res Policy 2008;13:85-91. https://doi.org/10.1258/jhsrp.2007.007011.
- Yao GL, Novielli N, Manaseki-Holland S, Chen YF, van der Klink M, Barach P, et al. Evaluation of a predevelopment service delivery intervention: an application to improve clinical handovers. BMJ Qual Saf 2012;21:i29-38. https://doi.org/10.1136/bmjqs-2012-001210.
- Nuckols TK, Smith-Spangler C, Morton SC, Asch SM, Patel VM, Anderson LJ, et al. The effectiveness of computerized order entry at reducing preventable adverse drug events and medication errors in hospital settings: a systematic review and meta-analysis. Syst Rev 2014;3. https://doi.org/10.1186/2046-4053-3-56.
- O’Hagan A, Buck CE, Daneshkhah A, Eiser JR, Garthwaite PH, Jenkinson DJ, et al. Uncertain Judgements: Eliciting Experts’ Probabilities. New York, NY: John Wiley & Sons; 2006.
- Morgan MG. Use (and abuse) of expert elicitation in support of decision making for public policy. Proc Natl Acad Sci U S A 2014;111:7176-84. https://doi.org/10.1073/pnas.1319946111.
- O’Hagan A. Eliciting expert beliefs in substantial practical applications. Statistician 1998;47:21-35. https://doi.org/10.1111/1467-9884.00114.
- Johnson SR, Tomlinson GA, Hawker GA, Granton JT, Feldman BM. Methods to elicit beliefs for Bayesian priors: a systematic review. J Clin Epidemiol 2010;63:355-69. https://doi.org/10.1016/j.jclinepi.2009.06.003.
- O’Hagan T. SHELF: The Sheffield Elicitation Framework. 2014. www.tonyohagan.co.uk/shelf/ (accessed 11 August 2017).
- French S. Aggregating expert judgement. Revista De La Real Academia De Ciencias Exactas, Fisicas Y Naturales 2011;105:181-206. https://doi.org/10.1007/s13398-011-0018-6.
- de Vries EN, Ramrattan MA, Smorenburg SM, Gouma DJ, Boermeester MA. The incidence and nature of in-hospital adverse events: a systematic review. Qual Saf Health Care 2008;17:216-23. https://doi.org/10.1136/qshc.2007.023622.
- Bates DW, Spell N, Cullen DJ, Burdick E, Laird N, Petersen LA, et al. The costs of adverse drug events in hospitalized patients. J Am Med Assoc 1997;277:307-11. https://doi.org/10.1001/jama.1997.03540280045032.
- Encinosa WE, Bae J. Will meaningful use electronic medical records reduce hospital costs?. Am J Manag Care 2013;19:eSP19-eSP25.
- Evans RS, Classen DC, Stevens LE, Pestotnik SL, Gardner RM, Lloyd JF, et al. Using a hospital information system to assess the effects of adverse drug events. Proc Annu Symp Comput Appl Med Care 1993:161-5.
- Hoonhout LH, de Bruijne MC, Wagner C, Asscheman H, van der Wal G, van Tulder MW. Nature, occurrence and consequences of medication-related adverse events during hospitalization: a retrospective chart review in the Netherlands. Drug Saf 2010;33:853-64. https://doi.org/10.2165/11536800-000000000-00000.
- Hug BL, Keohane C, Seger DL, Yoon C, Bates DW. The costs of adverse drug events in community hospitals. Jt Comm J Qual Patient Saf 2012;38:120-6. https://doi.org/10.1016/S1553-7250(12)38016-1.
- Rottenkolber D, Hasford J, Stausberg J. Costs of adverse drug events in German hospitals – a microcosting study. Value Health 2012;15:868-75. https://doi.org/10.1016/j.jval.2012.05.007.
- Senst BL, Achusim LE, Genest RP, Cosentino LA, Ford CC, Little JA, et al. Practical approach to determining costs and frequency of adverse drug events in a health care network. Am J Health Syst Pharm 2001;58:1126-32. https://doi.org/10.1093/ajhp/58.12.1126.
- Classen D, Pestotnik S, Evans S, Lloyd J, Burke J. Adverse drug events in hospitalized patients. JAMA 1997;277:1352-3. https://doi.org/10.1001/jama.277.17.1352.
- Landrigan CP, Parry GJ, Bones CB, Hackbarth AD, Goldmann DA, Sharek PJ. Temporal trends in rates of patient harm resulting from medical care. N Engl J Med 2010;363:2124-34. https://doi.org/10.1056/NEJMsa1004404.
- Zegers M, de Bruijne MC, Wagner C, Hoonhout LH, Waaijman R, Smits M, et al. Adverse events and potentially preventable deaths in Dutch hospitals: results of a retrospective patient record review study. Qual Saf Health Care 2009;18:297-302. https://doi.org/10.1136/qshc.2007.025924.
- NatCen Social Research . Health Survey for England n.d. http://natcen.ac.uk/our-research/research/health-survey-for-england/ (accessed 7 October 2018).
- Bermingham SL, Ashe J. Systematic review of the impact of urinary tract infections on health related quality of life. BJU Int 2012;110:830-6. https://doi.org/10.1111/j.1464-410X.2012.11337.x.
- Department of Health and Social Care . NHS Reference Costs 2014 to 2015 n.d. www.gov.uk/government/publications/nhs-reference-costs-2014-to-2015 (accessed August 2021).
- Girling A, Lilford R, Cole A, Young T. Headroom approach to device development: current and future directions. Int J Technol Assess Health Care 2015;31:331-8. https://doi.org/10.1017/S0266462315000501.
- Girling AJ, Freeman G, Gordan JP, Poole-Wilson P, Scott DA, Lilford RJ. Modelling payback from research into the efficacy of left-ventricular assist devices as destination therapy. Int J Technol Assess Health Care 2007;23:269-77. https://doi.org/10.1017/S0266462307070365.
- McAteer H, Cosh E, Freeman G, Pandit A, Wood P, Lilford R. Cost-effectiveness analysis at the development phase of a potential health technology: examples based on tissue engineering of bladder and urethra. J Tissue Eng Regen Med 2007;1:343-9. https://doi.org/10.1002/term.36.
- Cosh E, Girling A, Lilford R, McAteer HL, Young T. Investing in new medical technologies: a decision framework. J Comm Biotechnol 2007;13:236-71. https://doi.org/10.1057/palgrave.jcb.3050062.
- Vallejo-Torres L, Steuten LM, Buxton MJ, Girling AJ, Lilford RJ, Young T. Integrating health economics modelling in the product development cycle of medical devices: a Bayesian approach. Int J Technol Assess Health Care 2008;24:459-64. https://doi.org/10.1017/S0266462308080604.
- Aarts J, Doorewaard H, Berg M. Understanding implementation: the case of a computerized physician order entry system in a large Dutch university medical center. J Am Med Inform Assoc 2004;11:207-16. https://doi.org/10.1197/jamia.M1372.
- Keshavjee K, Bosomworth J, Copen J, Lai J, Kucukyazici B, Lilani R, et al. Best practices in EMR implementation: a systematic review. AMIA Annu Symp Proc 2006;2006.
- Paré G. Implementing clinical information systems: a multiple-case study within a US hospital. Health Serv Manage Res 2002;15:71-92. https://doi.org/10.1258/0951484021912851.
- Yusof MM, Kuljis J, Papazafeiropoulou A, Stergioulas LK. An evaluation framework for Health Information Systems: human, organization and technology-fit factors (HOT-fit). Int J Med Inform 2008;77:386-98. https://doi.org/10.1016/j.ijmedinf.2007.08.011.
- O’Cathain A, Murphy E, Nicholl J. Three techniques for integrating data in mixed methods studies. BMJ 2010;341. https://doi.org/10.1136/bmj.c4587.
- Cresswell KM, Slee A, Coleman J, Williams R, Bates DW, Sheikh A. Qualitative analysis of round-table discussions on the business case and procurement challenges for hospital electronic prescribing systems. PLOS ONE 2013;8. https://doi.org/10.1371/journal.pone.0079394.
- Cresswell KM, Lee L, Slee A, Coleman J, Bates DW, Sheikh A. Qualitative analysis of vendor discussions on the procurement of Computerised Physician Order Entry and Clinical Decision Support systems in hospitals. BMJ Open 2015;5. https://doi.org/10.1136/bmjopen-2015-008313.
- Cresswell K, Coleman J, Smith P, Swainson C, Slee A, Sheikh A. Qualitative analysis of multi-disciplinary round-table discussions on the acceleration of benefits and data analytics through hospital electronic prescribing (ePrescribing) systems. J Innov Health Inform 2016;23. https://doi.org/10.14236/jhi.v23i2.178.
- Robert M, Wachter MD. Making IT Work: Harnessing the Power of Health Information Technology to Improve Care in England. n.d. www.gov.uk/government/uploads/system/uploads/attachment_data/file/550866/Wachter_Review_Accessible.pdf (accessed 12 May 2017).
- NHS . NHS Five Year Forward View n.d. www.england.nhs.uk/five-year-forward-view/ (accessed 31 July 2021).
- Staley K. Exploring Impact: Public Involvement in NHS, Public Health and Social Care Research. Eastleigh: INVOLVE; 2009.
- Brett J, Staniszewska S, Mockford C, Seers K, Herron-Marx SB, Bayliss HR. The PIRICOM Study: A Systematic Review of the Conceptualisation, Measurement, Impact and Outcomes of Patients and Public Involvement in Health and Social Care Research. Coventry: University of Warwick; 2010.
- Staniszewska S, Brett J, Mockford C, Barber R. The GRIPP checklist: strengthening the quality of patient and public involvement reporting in research. Int J Technol Assess Health Care 2011;27:391-9. https://doi.org/10.1017/S0266462311000481.
- Staley K, Buckland SA, Hayes H, Tarpey M. ‘The Missing Links’: understanding how context and mechanism influence the impact of public involvement in research. Health Expect 2014;17:755-64. https://doi.org/10.1111/hex.12017.
- INVOLVE . Briefing Notes for Researchers: Involving the Public in NHS, Public Health and Social Research 2012.
- Department of Health and Social Care (DHSC) . Equity and Excellence: Liberating the NHS 2010.
- Cartwright J, Crowe S. Patient and Public Involvement Toolkit. Chichester: Wiley–Blackwell; 2011.
- NHS . Dictionary of Medicines and Devices n.d. www.nhsbsa.nhs.uk/pharmacies-gp-practices-and-appliance-contractors/dictionary-medicines-and-devices-dmd (accessed 31 July 2021).
- Wiegel V, King A, Mozaffar H, Cresswell K, Williams R, Sheik A. A systematic analysis of the optimization of computerized physician order entry and clinical decision support systems: a qualitative study in English hospitals. J Health Inform 2019;26:1118-32. https://doi.org/10.1177/1460458219868650.
- Great Britain . The Misuse of Drugs Regulations 2001 n.d. www.legislation.gov.uk/uksi/2001/3998/contents/made (accessed 31 July 2021).
- International Statistical Classification of Diseases and Related Health Problems 10th Revision n.d. https://icd.who.int/browse10/2010/en (accessed 31 July 2021).
- SNOMED International . Use SNOMED CT n.d. www.snomed.org/snomed-ct/Use-SNOMED-CT (accessed 31 July 2021).
- Coleman JJ, van der Sijs H, Haefeli WE, Slight SP, McDowell SE, Seidling HM, et al. On the alert: future priorities for alerts in clinical decision support for computerised physician order entry identified from a European workshop. BMC Med Inform Decis Mak 2013;13. https://doi.org/10.1186/1472-6947-13-111.
- Schofield B, Cresswell K, Westbrook J, Slee A, Girling A, Shah S, et al. The impact of electronic prescribing systems on pharmacists’ time and workflow: protocol for a time-and-motion study in English NHS hospitals. BMJ Open 2015;5. https://doi.org/10.1136/bmjopen-2015-008785.
- Cresswell KM, Sheikh A. Health information technology in hospitals: current issues and future trends. Future Hosp J 2015;2:50-6. https://doi.org/10.7861/futurehosp.2-1-50.
- Cresswell K, Mozaffar H, Shah S, Sheikh A. A systematic assessment of review to promoting the appropriate use of antibiotics through hospital electronic prescribing systems. Int J Pharm Pract 2017;25:5-17. https://doi.org/10.1111/ijpp.12274.
- Cresswell K. Evaluation of implementation of health IT. Stud Health Technol Inform 2016;222:206-19.
- Cresswell K, Bates DW, Sheikh A. Six ways for governments to get value from health IT. Lancet 2016;387:2074-5. https://doi.org/10.1016/S0140-6736(16)30519-0.
Appendix 1 Outline of work package 1 research design
WP1: main study |
|
|
Patient study |
|
|
Supplier study |
|
|
Appendix 2 Summary of the case study sites
Site | Site characteristics | System description | Implementation start date; roll-out date |
---|---|---|---|
A | Urban, acute care, university hospital, 330,000 patients per year |
|
2010 roll-out |
C | Urban, acute care, university hospital, 750,000 patients per year |
|
2011; 2013 roll-out in most wards |
D | Urban, acute care, university hospital, 3,500,000 patients per year |
|
2013 roll-out |
E | Urban, acute care, 550,000 patients per year |
|
2011; implementation has been paused (system not rolled out) |
J | Urban, acute care, university hospital, 3,000,000 patients per year |
|
2009; 2014 for 44% of the hospital. Final 54% planned to roll-out in 2016 |
K | Urban, acute care, university hospital, 540,000 patients per year |
|
2013 roll-out |
Appendix 3 Summary of work package 1 data collection
Site | Time 1 | Time 2 | Time 3 | Observations; documents |
---|---|---|---|---|
A |
|
|
|
|
C |
|
|
|
|
D |
|
|
|
|
E |
|
|
|
|
J |
|
|
|
|
K |
|
|
|
Appendix 4 Work package 2 pilot work on the frequency of alerts for prescription indicators at University Hospitals Birmingham NHS Foundation Trust
Category | Prescription indicator | Estimated frequency of indicator generation | Heeding of warning/revoked Rx in University Hospitals Birmingham NHS Foundation Trust |
---|---|---|---|
Hazardous drug–drug interactions | Warfarin with macrolide antibiotics (without appropriate dose reduction or annotation re monitoring) | 6 | 0 |
Warfarin with amiodarone | 98 | 22 | |
Lithium and NSAIDs/diuretics | 1 | 0 | |
Verapamil and beta-blocker | 0 | 0 | |
Aspirin and anticoagulants (warfarin, enoxaparin) | 409 | 66 | |
Clopidogrel and NSAIDs | 234 | 60 | |
Warfarin and NSAIDs | 105 | 17 | |
Contraindicated prescribing | NSAIDs in severe renal impairment | 18 | 9 |
Beta-blocker (non-cardioselective) in asthma | 10 | 8 | |
Enoxaparin in renal impairment | 19 | 5 | |
Warfarin in peptic ulcer disease | 113 | 20 | |
Inappropriate drug dosing | Gentamicin at a dose of > 7 mg/day | Disallowed in the system: therefore not evaluable | |
Low-molecular-weight heparin given without reference to latest weight (treatment doses) | Disallowed in the system: therefore not evaluable | ||
Methotrexate more than once weekly | 0 (note that default dosing makes this error extremely unlikely in current system) | 0 | |
Paracetamol (> 4 g in 24 hours) | 287 | 52 | |
Drug doubling/therapeutic duplication | Paracetamol-containing compounds (more than one concurrent) | 51 | 14 |
NSAIDs (more than one concurrent) | 57 | 12 | |
Opioid duplication (codeine, tramadol and compound analgesics with opioids) | 632 | 125 | |
Laboratory drug interaction | Potassium supplements to patients with supranormal potassium levels | Disallowed in the system: therefore not evaluable | 0 |
Warfarin prescribing with baseline INR of > 5 | Unknown | N/A | |
Drug allergy checking | Cephalosporins given to penicillin-allergic patient | 3 | 0 |
Any penicillin-containing compound prescribed for a penicillin-allergic patient | Disallowed in the system: therefore not evaluable | ||
Total | 2043 warnings | (Average heeding rate of 23%) |
Appendix 5 Work package 2 final prescription indicators
Prescribing safety indicator title | Group | Error type |
---|---|---|
Low-molecular-weight heparin prescribed without the patient’s weight being used to calculate the treatment dose (risk of subtherapeutic or supratherapeutic dosing) | Cardiovascular | Dosing |
Low-molecular-weight heparin prescribed at a dose exceeding the maximum as stated in the product literature (risk of bleeding increased) | Cardiovascular | Dosing |
Digoxin prescribed at a dose of > 125 µg daily to a patient with renal impairment (increased risk of digoxin toxicity) | Cardiovascular | Dosing |
Digoxin prescribed at a dose of > 125 µg daily to a patient with heart failure who is in sinus rhythm (increased risk of digoxin toxicity) | Cardiovascular | Dosing |
Amiodarone prescribed to a patient with abnormal thyroid function tests (increased risk of thyroid disorders) | Cardiovascular | Clinical contraindication |
Non-cardioselective beta-adrenoceptor-blocking drug prescribed to a patient with COPD (increased risk of bronchospasm) | Cardiovascular | Clinical contraindication |
ACE inhibitor or angiotensin-II-receptor antagonist prescribed to a patient with a potassium level of ≥ 5.0 mmol/l (can cause or exacerbate hyperkalaemia) | Cardiovascular | Clinical contraindication |
Verapamil prescribed to a patient with NYHA Class III or IV heart failure (risk of precipitating heart failure, exacerbating conduction disorders and causing significant deterioration) | Cardiovascular | Clinical contraindication |
Low-molecular-weight heparin prescribed to a patient with renal impairment without dose adjustment (increased risk of bleeding) | Cardiovascular | Dosing |
Warfarin prescribed to a patient with a concurrent bleeding disorder (increased risk of bleeding) | Cardiovascular | Clinical contraindication |
Aspirin prescribed to a patient with a past medical history of peptic ulcer disease without antisecretory drugs or mucosal protectants (increased risk of peptic ulceration and risk of bleeding) | Cardiovascular | Clinical contraindication |
Antiplatelet prescribed to a patient with a concurrent bleeding disorder (increased risk of bleeding) | Cardiovascular | Clinical contraindication |
Digoxin prescribed concomitantly with a diuretic (risk of hypokalaemia and subsequent digoxin toxicity) | Cardiovascular | Drug–drug interaction |
Statin prescribed concomitantly with a macrolide antibiotic (increased risk of myopathy) | Cardiovascular | Drug–drug interaction |
Potassium-sparing diuretic (excluding aldosterone antagonists) prescribed to a patient also receiving an ACE inhibitor or angiotensin-II receptor antagonist (increased risk of severe hyperkalaemia) | Cardiovascular | Drug–drug interaction |
Amiodarone prescribed concomitantly with simvastatin at 40 mg or above (increased risk of myopathy) | Cardiovascular | Drug–drug interaction |
Verapamil prescribed to a patient concomitantly a with beta-adrenoceptor blocking drug (increased risk of adverse cardiovascular effects) | Cardiovascular | Drug–drug interaction |
Warfarin prescribed concomitantly with a NSAID (increased risk of bleeding) | Cardiovascular | Drug–drug interaction |
Clopidogrel prescribed to a patient concomitantly with a NSAID (increased risk of bleeding) | Cardiovascular | Drug–drug interaction |
Clopidogrel prescribed to a patient concomitantly with omeprazole or esomeprazole (antiplatelet effect of clopidogrel potentially reduced) | Cardiovascular | Drug–drug interaction |
Macrolide antibiotic prescribed concomitantly with warfarin without appropriate dose adjustment or increased INR monitoring (increased risk of bleeding) | Cardiovascular | Drug–drug interaction |
Low-molecular-weight heparin omitted to be prescribed for prophylaxis (increased risk of thrombosis) | Cardiovascular | Omission of prophylactic treatment |
Lithium dose not adjusted or omitted in a patient with a lithium concentration above the therapeutic range (> 1.0 mmol/l) (risk of lithium toxicity) | Central nervous system | Dosing |
Paracetamol prescribed at a dose of 4 g over 24 hours to a patient under 50 kg (risk of hepatocellular toxicity) | Central nervous system | Dosing |
Benzodiazepine or benzodiazepine-like drug prescribed to a patient with COPD (risk of respiratory depression) | Central nervous system | Clinical contraindication |
Antipsychotic, other than risperidone, prescribed to a patient for the management of the behavioural and psychological symptoms of dementia (increased risk of stroke) | Central nervous system | Clinical contraindication |
Tricyclic antidepressant prescribed to a patient with dementia (increased risk of worsening cognitive impairment) | Central nervous system | Clinical contraindication |
Selective serotonin re-uptake inhibitor prescribed to a patient with epilepsy (increased risk of seizure threshold being reduced) | Central nervous system | Clinical contraindication |
Selective serotonin re-uptake inhibitor prescribed to a patient with a history of clinically significant hyponatraemia (non-iatrogenic, sodium < 130 mmol/l in the previous 2 months) (increased risk of hyponatraemia) | Central nervous system | Clinical contraindication |
Prochlorperazine prescribed to a patient with Parkinsonism (risk of exacerbating Parkinsonism symptoms) | Central nervous system | Clinical contraindication |
NSAID prescribed to a patient with chronic renal failure (increased risk of deteriorating renal function) | Central nervous system | Clinical contraindication |
NSAID prescribed to a patient with a history of peptic ulcer disease or gastrointestinal bleeding without antisecretory drugs or mucosal protectants (increased risk of peptic ulceration and bleeding) | Central nervous system | Clinical contraindication |
NSAID prescribed to a patient with a history of heart failure (risk of exacerbation of heart failure) | Central nervous system | Clinical contraindication |
Lithium prescribed in conjunction with newly prescribed NSAIDs without dose adjustment or increased monitoring (increased risk of toxicity) | Central nervous system | Drug–drug interaction |
Lithium therapy prescribed in conjunction with newly prescribed loop or thiazide diuretics without dose adjustment or increased monitoring (increased risk of toxicity) | Central nervous system | Drug–drug interaction |
Tricyclic antidepressant prescribed at the same time as a monoamine oxidase inhibitor (increased risk of serotonin syndrome) | Central nervous system | Drug–drug interaction |
Tramadol prescribed concomitantly with a monoamine oxidase inhibitor (increased risk of serotonin syndrome) | Central nervous system | Drug–drug interaction |
Selective serotonin re-uptake inhibitor prescribed concomitantly with tramadol (increased risk of serotonin syndrome) | Central nervous system | Drug–drug interaction |
Selective serotonin re-uptake inhibitor prescribed concomitantly with aspirin without appropriate prophylaxis with antisecretory drugs or mucosal protectant (increased risk of gastrointestinal bleeding) | Central nervous system | Drug–drug interaction |
Citalopram prescribed concomitantly with other QT-prolonging drugs (increased risk of arrhythmias) | Central nervous system | Drug–drug interaction |
Tramadol prescribed concomitantly with antiepileptics (increased risk of seizures in patients with uncontrolled epilepsy) | Central nervous system | Drug–drug interaction |
Nefopam prescribed concomitantly with antiepileptics (increased risk of seizures in patients with uncontrolled epilepsy) | Central nervous system | Drug–drug interaction |
Phenytoin and enteral feeds prescribed to a patient concomitantly (reduced absorption of phenytoin) | Central nervous system | Drug–food interaction |
More than one paracetamol-containing product prescribed to a patient at a time (maximum dose exceeded) | Central nervous system | Duplicate therapy |
Benzodiazepines prescribed long term (i.e. more than 2–4 weeks) (risk of dependence and withdrawal reactions) | Central nervous system | Duration |
Benzodiazepine or benzodiazepine-like drug prescribed long term to a patient with depression (risk of dependence and withdrawal reactions) | Central nervous system | Duration |
Benzodiazepine-like drugs (e.g. zopiclone) prescribed long term (i.e. more than 2–4 weeks) (risk of dependence reactions) | Central nervous system | Duration |
Antipsychotic prescribed long term (i.e. > 1 month) to a patient with Parkinsonism (increased risk of worsening of extra-pyramidal side effects) | Central nervous system | Duration |
Regular opiates prescribed without concurrent use of laxatives (risk of severe constipation) | Central nervous system | Omission of prophylactic treatment |
Prescribing of incorrect or inequivalent morphine (opiate) dose via multiple routes (risk of toxicity) | Central nervous system | Route |
Glibenclamide prescribed to an older adult with type 2 diabetes mellitus (increased risk of hypoglycaemia) | Endocrine | Clinical contraindication |
Metformin prescribed to a patient with eGFR of < 30 ml/minute/1.73m2 (increased risk of lactic acidosis) | Endocrine | Clinical contraindication |
Pioglitazone prescribed to a patient with heart failure (risk of exacerbation of heart failure) | Endocrine | Clinical contraindication |
Soluble insulin prescribed to a patient when required (increased risk of serious episodes of hypoglycaemia and nocturnal hypoglycaemia post dose) | Endocrine | Frequency |
Insulin prescribed to a patient at an inappropriate time, allowing for an administration without food (except once-daily long-acting insulins) (increased risk of hypoglycaemia) | Endocrine | Timing of dose |
Domperidone prescribed at a total daily dose exceeding 30 mg/day in adults aged > 60 years (increased risk of QTc prolongation, serious ventricular arrhythmia and sudden cardiac death) | Gastrointestinal | Dosing |
Diphenoxylate, loperamide, codeine phosphate prescribed as antidiarrhoeal agents for treatment of severe infective gastroenteritis (e.g. bloody diarrhoea, high fever, or severe systemic toxicity) (increased risk of exacerbation or protraction of infection) | Gastrointestinal | Clinical contraindication |
Metoclopramide prescribed to a patient with Parkinsonism (risk of exacerbating Parkinsonism symptoms) | Gastrointestinal | Clinical contraindication |
Colestyramine prescribed to a patient at the same time as any other oral medication (risk of poor clinical effect owing to reduced absorption of medications) | Gastrointestinal | Drug–drug interaction |
Orlistat prescribed at the same time of day as oral antiepileptics (orlistat can reduce the absorption of antiepileptics, leading to loss of seizure control) | Gastrointestinal | Drug–drug interaction |
Diphenoxylate, loperamide or codeine phosphate prescribed as antidiarrhoeal agents for treatment of diarrhoea of unknown cause (increased risk of exacerbating constipation with overflow diarrhoea) | Gastrointestinal | Indication |
Penicillin-containing compound prescribed to a penicillin-allergic patient without reasoning (e.g. a mild or non-allergy, such as diarrhoea or vomiting, entered as an allergy where the indication for penicillin is compelling) (risk of hypersensitivity reactions) | Infection | Allergy |
Gentamicin dose calculated based on actual body weight rather than ideal body weight in an obese patient (BMI > 30 kg/m2) (risk of excessive dosing and toxicity) | Infection | Dosing |
Amphotericin B prescribed without stating the brand name and the dose in mg/kg (risk of fatal overdose owing to confusion between lipid-based and non-lipid formulations) | Infection | Drug name |
Cephalosporin antibiotic prescribed to an older adult (except under the direction of microbiology or for suspected meningitis) (increased risk of antibiotic-associated infections) | Infection | Clinical contraindication |
Gentamicin prescribed to a patient with renal impairment without dose adjustment (increased risk of toxicity) | Infection | Dosing |
Gentamicin prescribed to an adult patient with normal renal function in a dose exceeding 7 mg/kg/day (increased risk of toxicity) | Infection | Dosing |
Vancomycin prescribed intravenously to a patient with renal impairment without dose adjustment (increased risk of toxicity) | Infection | Clinical contraindication |
Quinolone antibiotic prescribed to a patient with epilepsy (increased risk of seizure threshold being reduced) | Infection | Clinical contraindication |
Nitrofurantoin prescribed to a patient with eGFR < 60 ml/minute/1.73m2 (risk of peripheral neuropathy and inadequate concentration in urine) | Infection | Clinical contraindication |
Quinolone prescribed to a patient who is also receiving theophylline (possible increased risk of convulsions) | Infection | Drug–drug interaction |
Atazanavir prescribed concomitantly with a proton-pump inhibitor (concentration of atazanavir potentially reduced, reducing therapeutic effect) | Infection | Drug–drug interaction |
Vancomycin prescribed intravenously over less than 60 minutes (rapid infusion of vancomycin can cause severe reactions) | Infection | Intravenous rate |
Brand specific prescribing of tacrolimus preparations (brands vary in their dosing and pharmacokinetics) | Miscellaneous | Drug name |
Methotrexate prescribed to a patient with a clinically significant drop in white cell count or platelet count (risk of bone marrow suppression) | Miscellaneous | Clinical contraindication |
Methotrexate prescribed to a patient with abnormal liver function tests (risk of liver toxicity) | Miscellaneous | Clinical contraindication |
Potassium chloride supplements continued for longer than is required (reference range 3.5–5.3 mmol/l) (increased risk of hyperkalaemia) | Miscellaneous | Clinical contraindication |
Methotrexate prescribed concomitantly with trimethoprim (increased risk of haematological toxicity) | Miscellaneous | Drug–drug interaction |
Weekly dose of an oral bisphosphonate prescribed daily (risk of hypocalcaemia) | Miscellaneous | Frequency |
Oral methotrexate prescribed to a patient with an inappropriate frequency (increased risk of toxicity) | Miscellaneous | Frequency |
Appendix 6 Information about the IMPACT tool
The IMPACT tool is a stand-alone Windows application, developed using C# and .NET Framework technologies. It presents the user with a form containing a series of questions to capture data about the patient and the drugs that they are prescribed (Figures 3 and 4). Its format and content is defined in an XML file and is highly configurable, with the questions presented changing dependent on the answers given.
FIGURE 3.
The IMPACT front page. Reproduced with permission, personal communication, 2021.

FIGURE 4.
The prescription review page. Reproduced with permission, personal communication, 2021.

The data captured are held locally in a Structured Query Language server database and are encrypted before secure data transfer for collation and further analysis. The secure data transfer for the IMPACT data capture tool uses a combination of symmetric and asymmetric (public-key) encryption. Prior to being exported from the database, every form has a new and random symmetric encryption key generated. This encryption key together with a generated random initial block of data are used to encrypt the data in the form using symmetric encryption. The form’s symmetric encryption key is then encrypted using asymmetric encryption (using the public encryption key) and written together with the initial block of data and the encrypted form data to a file that can then be securely transferred from the hospital site to University Hospitals Birmingham NHS Foundation Trust. Once at University Hospitals Birmingham NHS Foundation Trust, the symmetric encryption key can be decrypted (using the private asymmetric decryption key), allowing the form data to be decrypted and processed. In this method, only the keys required for asymmetric encryption and decryption of each form’s symmetric encryption key needs to be held, and only the public encryption key needs to be stored in the software used in the hospital site. Given that University Hospitals Birmingham NHS Foundation Trust controls the software both to capture the data on the hospital site and to process the transferred data at University Hospitals Birmingham NHS Foundation Trust, these asymmetric keys can be held securely within the software, and the private decryption key never needs to be stored outside University Hospitals Birmingham NHS Foundation Trust. Both encryption methods are industry-standard methods provided by Microsoft (Microsoft Corporation, Redmond, WA, USA) and the longest key length possible has been used to maximise security of the data.
Appendix 7 Work package 2 standardised data collection process

Appendix 8 Work package 2: documentation errors under paper-based (pre) and electronic (post) ePrescribing system implementation
Site | Patient records (n) | Error, n (%) | Total medication orders (n) | Error, n (%) | ||
---|---|---|---|---|---|---|
Allergy status not recorded | Weight not recorded | Incomplete orders | Orders with inappropriate dose units | |||
Paper | ||||||
J | 365 | 3 (0.8) | 28 (7.7) | 4471 | 2763 (61.8) | 67 (1.5) |
K | 615 | 60 (9.8) | 242 (39.3) | 5932 | 300 (5.1) | 79 (1.3) |
S | 264 | 26 (9.8) | 185 (70.1) | 3968 | 196 (4.9) | 65 (1.6) |
Total | 1244 | 89 (7.2) | 455 (36.6) | 14,371 | 3259 (22.7) | 211 (1.5) |
Electronic | ||||||
J | 297 | 4 (1.3) | 37 (12.5) | 4071 | 0 (0.0) | 0 (0.0) |
K | 581 | 11 (1.9) | 62 (10.7) | 6052 | 0 (0.0) | 0 (0.0) |
S | 300 | 2 (0.7) | 155 (51.7) | 4032 | 0 (0.0) | 0 (0.0) |
Total | 1178 | 17 (1.4) | 254 (21.6) | 14,155 | 0 (0.0) | 0 (0.0) |
Appendix 9 Work package 2: summary of indicator errors
Site | Pre CPOE | Post CPOE | RR (95% CI) | p-value | ||||||
---|---|---|---|---|---|---|---|---|---|---|
Patient records | N opp | N err | Error rate (%) | Patient records | N opp | N err | Error rate (%) | |||
J | 365 | 3867 | 167 | 4.3 | 297 | 2634 | 74 | 2.8 | 0.65 (0.49 to 0.86) | 0.002 |
K | 615 | 4513 | 179 | 4.0 | 581 | 4527 | 200 | 4.4 | 1.11 (0.91 to 1.36) | 0.294 |
S | 264 | 2932 | 216 | 7.4 | 300 | 2665 | 116 | 4.4 | 0.59 (0.47 to 0.74) | < 0.001 |
Total | 1244 | 11,312 | 562 | 5.0 | 1178 | 9826 | 390 | 4.0 | 0.80 (0.70 to 0.91) | < 0.001 |
Appendix 10 Level of clinical decision support
Level of CDS | Definition |
---|---|
Restricted | Error was prevented by the system as prescribers could not proceed |
Guided | Default fields pre populated to encourage the prescriber to accept and continue |
Permitted | An alert for which a reason needs to be given to over-ride |
Alert | An alert for which no reason needs to be given for over-ride |
None | No interruptive CDS present |
Appendix 11 Comparison of paper- and ePrescribing-based systems
Level of support | Paper-based systems | ePrescribing-based systems | Rate ratio (95% CI) | p-value | |||||
---|---|---|---|---|---|---|---|---|---|
N ind | N opp | N err | Error rate (%) ( = 100Nerr/Nopp) | N opp | N err | Error rate (%) ( = 100Nerr/Nopp) | |||
Site J | |||||||||
Restricted | 6 | 190 | 9 | 4.74 | 182 | 0 | 0.00 | 0.00 | 0.0036 |
Guided | 5 | 288 | 32 | 11.11 | 143 | 15 | 10.49 | 0.94 (0.51 to 1.74) | 1.0000 |
Permitted | 8 | 878 | 22 | 2.51 | 488 | 2 | 0.41 | 0.16 (0.04 to 0.70) | 0.0041 |
Alert | 1 | 4 | 1 | 25.00 | 3 | 0 | 0.00 | 0.00 | 1.0000 |
None (excluding VTE) | 59 | 2742 | 117 | 4.27 | 1924 | 57 | 2.96 | 0.69 (0.51 to 0.95) | 0.0226 |
Subtotal | 79 | 4102 | 181 | 4.41 | 2740 | 74 | 2.70 | 0.61 (0.47 to 0.80) | 0.0003 |
VTE (none) | 1 | 365 | 86 | 23.56 | 297 | 24 | 8.08 | 0.34 (0.22 to 0.54) | 0.0000 |
Total | 80 | 4467 | 267 | 5.98 | 3037 | 98 | 3.23 | 0.54 (0.43 to 0.68) | 0.0000 |
Site K | |||||||||
Restricted | 5 | 342 | 17 | 4.97 | 235 | 0 | 0.00 | 0.00 | 0.0002 |
Guided | |||||||||
Permitted | 20 | 1138 | 66 | 5.80 | 1084 | 36 | 3.32 | 0.57 (0.38 to 0.86) | 0.0060 |
Alert | 3 | 174 | 5 | 2.87 | 190 | 4 | 2.11 | 0.73 (0.20 to 2.73) | 0.7420 |
None (excluding VTE) | 51 | 3177 | 106 | 3.34 | 3207 | 160 | 4.99 | 1.50 (1.17 to 1.91) | 0.0011 |
Subtotal | 79 | 4831 | 194 | 4.02 | 4716 | 200 | 4.52 | 1.06 (0.87 to 1.29) | 0.6069 |
VTE (none) | 1 | 615 | 68 | 11.06 | 581 | 84 | 14.46 | 1.31 (0.95 to 1.80) | 0.0827 |
Total | 80 | 5446 | 262 | 4.81 | 5297 | 284 | 5.36 | 1.11 (0.94 to 1.32) | 0.2027 |
Site S | |||||||||
Restricted | 3 | 17 | 7 | 41.18 | 4 | 0 | 0.00 | 0.00 | 0.2550 |
Guided | 1 | 133 | 8 | 6.02 | 129 | 0 | 0.00 | 0.00 | 0.0070 |
Permitted | 23 | 1083 | 82 | 7.57 | 1003 | 57 | 5.68 | 0.75 (0.54 to 1.05) | 0.0949 |
Alert | |||||||||
None (excluding VTE) | 52 | 1858 | 129 | 6.94 | 1677 | 64 | 3.82 | 0.55 (0.41 to 0.74) | 0.0000 |
Subtotal | 79 | 3091 | 226 | 7.31 | 2813 | 121 | 4.30 | 0.59 (0.47 to 0.73) | 0.0000 |
VTE (none) | 1 | 264 | 13 | 4.92 | 300 | 19 | 6.33 | 1.29 (0.64 to 2.60) | 0.5850 |
Total | 80 | 3355 | 239 | 7.12 | 3113 | 140 | 4.50 | 0.63 (0.51 to 0.78) | 0.0000 |
Appendix 12 Comparison of error rates on pre and post implementation grouped by level of clinical decision support

Appendix 13 Aggregated prior density for the relative risk of a mediation error with an ePrescribing system compared with paper-based prescribing from the expert elicitation work shop and individual expert priors densities


Appendix 14 Plot showing prior density (dashed line) and posterior density (solid line) for the relative risk of experiencing a medication error with an ePrescribing system compared with paper-based prescribing

Appendix 15 Conference 1 programme
Appendix 16 Evaluation summary from conference 1
Appendix 17 Conference 2 programme
Appendix 18 Evaluation summary from conference 2
Appendix 19 Conference 3 programme
Appendix 20 Evaluation summary from conference 3
Appendix 21 Conference 4 programme
Appendix 22 Evaluation summary from conference 4
Appendix 23 Example screenshots from the ePrescribing toolkit
Reproduced with permission, personal communication, 2021.
Appendix 24 The original (later amended) patient and public involvement strategy for the ePrescribing programme
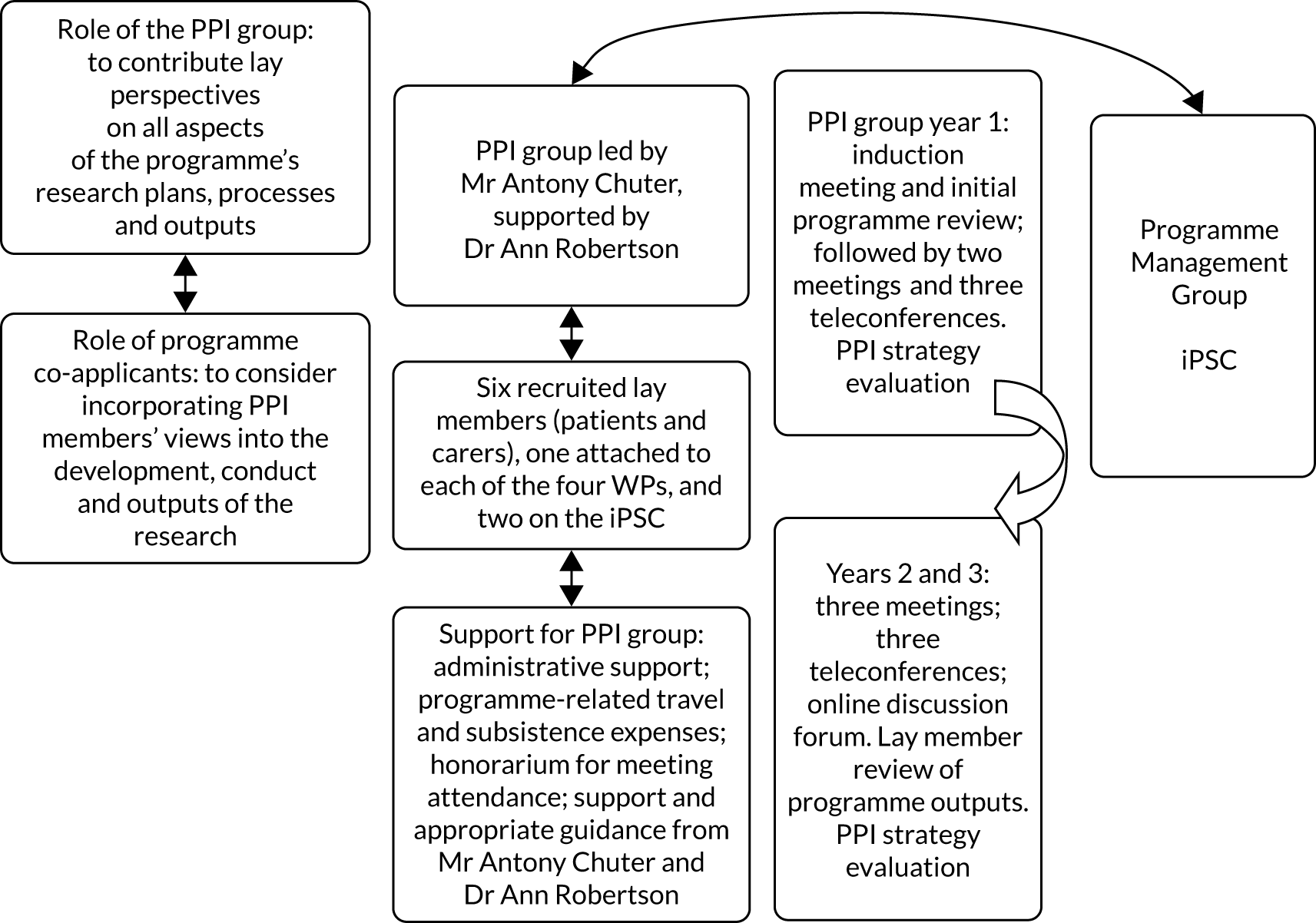
Appendix 25 Patient and public involvement ePrescribing jargon buster
Term | Abbreviation/acronym | Definition |
---|---|---|
Acute (medicines) | A medicine for managing an acute symptom/condition. An acute symptom/condition is of recent onset and limited duration (usually less than a few weeks) | |
Administration | The giving of a dose of a medicine to a patient in a managed care environment | |
Administration history | The timing and other details of a patient’s past medicine administrations | |
Administration instructions | Instructions that detail a prescriber’s directions as to how medicine is to be given/taken | |
Administration plan | The schedule and other details of a patient’s further medicine administrations | |
Adoption | Adoption of an electronic prescribing system is when using that system has become routine and embedded in everyday practice | |
Advanced alerting | A notification that is part of a decision support system within an electronic prescribing system. A basic or ‘non-alerting’ system does not have built-in decision support to aid prescribers | |
Adverse drug reaction | ADR | An unintended response to a medicine |
Alert | A notification that comes up on the screen of the system’s user | |
Allied health-care professional | AHP | A health-care professional, other than a medical doctor, nurse or psychologist, who works as part of a clinical team |
Authorisation | The act of a prescriber giving permission to administer or supply a medicine other than by a verbal order | |
Benchmark studies | Initial studies to define the criteria by which something else will be measured | |
Benefits realisation | Achievement of positive outcomes | |
British National Formulary | BNF | A reference publication that provides information about the use of medicines in the UK |
Care setting | The environment in which a patient receives care | |
Computer/clinical decision support | CDS | See ‘Computer/clinical decision support system’ |
Computer/clinical decision support system | CDSS | Clinical decision support systems are active knowledge systems that use patient data to generate patient-specific advice for the health-care professional who is prescribing |
Clinical effect | The effect of a medicine or treatment on a patient, which includes the beneficial effect(s) and may include side effects or allergies | |
Clinical trial | A scientific study to evaluate the clinical effect of a medicine or treatment on patients or volunteers | |
Clinician | A health-care professional who is involved in the clinical care of a patient | |
Compliance | The extent to which a patient or a health-care professional follows prescribing advice | |
Computerised order entry | A patient-specific request made on an electronic prescribing system | |
Computerised physician order entry | CPOE | Computerised physician order entry (by a doctor or other qualified prescriber) – see also ‘Computerised order entry’ |
Condition (patient) | Illness, diagnosis or health state of a patient | |
Confirmation (medicines) | The action of electronically reviewing and authorising a course of medicine to be dispensed or administered, and committing this to a patient records system | |
Connectivity | The means by which individual terminals, computers and mobile devices are connected to the central computer system | |
Context-rich enquiry | A study that considers a range of different factors that are outside the topic being investigated but are relevant to the topic | |
Contraindication | A clinical reason not to give a medicine | |
Controlled drug | CD | A medicine that may not be prescribed, supplied or administered other than within the restrictions of the Misuse of Drugs Regulations 2001131 |
Countersign | The act of adding a second ‘signature’ to indicate that an action has been witnessed or authorised | |
Course | A period of treatment when medicine is given over a defined time | |
Course definition | The details of a course of treatment | |
Course status | The current state of a course of medicine within its overall time period | |
Current medicines | A medicine or course of a medicine that a patient is receiving | |
Cycle | A repeated pattern of a course | |
Day case | A patient admitted electively with the intention of receiving care who does not require the use of a hospital bed overnight | |
Decision support system | See ‘Computer/clinical decision support system’ (CDSS) | |
Discharge medicines | Medicines that are prescribed and authorised for the patient to take home from hospital following an inpatient or day case stay | |
Discharge prescriptions | A clinician’s order for the preparation, supply and administration of a medicine or other treatment for the patient after leaving hospital | |
Discontinued medicines | Treatments that have been withdrawn from patient care for reason(s) that should have been specified | |
Dispense | The preparing and issuing of medicine(s) by pharmacy staff | |
Dispensing history | A record of past dispensing of a single medicine for a patient | |
Dosage or dose | A specified quantity of a therapeutic agent, such as a medicine, prescribed to be taken at one time or at stated intervals | |
Dose range | The minimum and maximum range of a dose of medicine | |
Drug (or medicine) classification | The listing of medicines according to different attributes (e.g. according to their effect on the body) | |
Drug name | The text agreed as an unambiguous name | |
Elective | Planned or scheduled | |
Electronic prescribing | eP | The action of ordering a prescription on a computer |
End date | The date for the end of a course of medicine/treatment | |
End point | One of the target outcomes of a research study | |
ePrescribing system | A computer-based system that enables electronic prescribing supporting all medical and non-medical prescribers to order patients’ medicines using computers | |
Expiry date | Date after which a preparation should not be used | |
Fluids | Liquids given to a patient with the aim of maintaining or improving the level of hydration or circulatory volume | |
Form (medicine) | A description of the presentation of a medicine (e.g. tablet or injection) | |
Formulary | A limited list of selected medicines available to be prescribed | |
Formulation | The compounding of a medicinal product into a form suitable for administration to a patient | |
Framework for cost categories | The itemisation and classification into categories of the various costs involved in changing from a paper-based to an electronic prescribing system | |
Functionality | What a given electronic prescribing system is capable of doing | |
Generic drug name | The chemical or approved name of a product instead of a brand name | |
Health issue (for a patient) | A health condition that a patient experiences, which impacts or has the potential to impact on their day-to-day life or future health | |
Health Technology Assessment | HTA | The evaluation of the impact of health-related technologies |
Health-care professional | HCP | A person involved in the provision of health care or social care who is regulated by a professional body |
Hospital Electronic Prescribing and Medicines Administration | HEPMA or EPMA | The same as or similar to an ePrescribing systems but including the recording of the giving of medicines to a patient |
Iatrogenic harm | Unintended injury to a patient as a result of medical treatment | |
Implementation (electronic prescribing system) | The process of putting an electronic prescribing system into a hospital and using it | |
Indication | The condition a patient has for which a medicine is being prescribed | |
Indicators (screen display) | Symbols that are associated with a medicine, device or course of a medicine, which are designed to quickly notify a system user of key items of information | |
Infrastructure | The basic facilities, services and installations needed for the functioning of an electronic prescribing system | |
Infusion | A medicine that is given at a rate controlled by a mechanical device | |
Inpatient | A patient when they are admitted to hospital | |
Integration | The bringing together of different computer systems to work together | |
Interface | The device used to access an electronic prescribing system (e.g. a computer, tablet or smart phone) | |
International Statistical Classification of Diseases and Related Health Problems codes | ICD | A medical classification coding system for diseases, signs and symptoms (currently Tenth Revision – ICD-10132) |
Interoperability | The ability of computers or computer software to ‘talk’ to each other without being specially configured to do so | |
Intervention | Any measure with a purpose to improve health or alter the course of a disease | |
Knowledge support | When knowledge is delivered to clinicians at the point of knowledge need either in the form of facts or in guidelines or protocols | |
Longitudinal case study | A case study for which data are gathered over a period of time (e.g. a hospital that is studied over months or years) | |
Medical device | A medical device is not a drug that achieves its purpose through chemical reaction within or on the body; it is as instrument, apparatus or implant used to diagnose, prevent or treat disease or other conditions | |
Medication or medicine | A substance given to cure, heal, prevent or relieve the symptoms of a disease | |
Morbidity | Rate of incidence of a disease | |
Mortality | Death | |
Multidisciplinary collaboration | A research team combining different individuals each with their own specialised skills and expertise | |
Multifaceted programme | A research study made up of several different but related research projects | |
Naturalistic evaluation | A research method that involves observing study participants in their natural environment | |
Non-compliance | Failure to take a medicine as directed | |
Opportunity costs | A benefit that must be given up to acquire or achieve an alternative | |
Order (for a patient) | A request from an authorised clinician for the supply or administration of medicine(s) | |
Order set | A predefined list of orders normally containing complete information to allow the authorisation of the supply or administration of a medicine | |
Outcome | An observed consequence | |
Outpatient | A patient who goes to a hospital for a consultation | |
Patient | An individual receiving or registered to receive medical treatment | |
Patient and public involvement | PPI | Having members of the public actively involved in bringing their lay perspectives to academic/medical research |
Patient instructions | Information telling a patient how much of a medicine to take, how often and for how long and by what method, including any warnings | |
Patient record | An electronic record of a patient’s care and treatment | |
Patient safety | Freedom from health-care-associated, preventable harm | |
Patient’s own drugs | PODs | Medicines brought into a hospital by a patient that have been previously prescribed and dispensed for the patient in either primary or secondary care, or purchased over the counter |
Preparation | The act of preparing medicines for administration | |
Prescription | Rx | An order, created by a clinician, for the preparation, supply and administration of a medicine or other treatment for a patient |
Prescription-only medicines | POM | Medicines that may be sold or supplied from pharmacies only in accordance with a prescription from an appropriate clinician |
Primary care | Goods and/or services provided by general practitioner (family doctor) doctors, dentists, pharmacists, optometrists and ophthalmic medical practitioners, together with district nurses, health visitors and community AHPs | |
p.r.n. medicines | A medicine to be taken on an ‘as-required’ basis, as determined by a patient within specific guidelines to treat specific symptoms | |
Procurement | The act of obtaining or buying goods and/or services | |
Protocol | A predefined list of treatments or plans | |
Qualitative research | A research methodology that investigates and analyses experiences and perceptions | |
Quantitative research | A research methodology that collects numerical data to produce statistical results | |
Referral | The process by which one professional refers a patient or service user to another | |
Regimen | A set of rules outlining the treatment that is to be ordered for a patient | |
Repeat prescription | A prescription that is authorised so that the patient may at intervals obtain another copy without a doctor consultation | |
Review date | The latest date at which a patient’s prescribed medicine(s) should next be reviewed | |
Schedule | The specific time(s) or frequency at which a medicine should be administered | |
Script | Shortened term for prescription (see ‘Prescription’) | |
Secondary care | Specialist care traditionally provided in a hospital setting | |
133SNOMED Clinical Terms® | SNOMED-CT® | A clinical terminology that enables clinical documentation and reporting, and can be used to code, retrieve and analyse clinical data |
Special medicine | See ‘Unlicensed medicine’ | |
Strength | The quantity of an active pharmaceutical product contained within a measured volume or dose | |
Systems analyst | A person who uses analysis and design techniques to solve problems using information technology | |
Tendering | Making a formal written offer for supply of goods or services | |
The NHS dictionary of medicines and devices | dm + d | A dictionary of descriptions and codes that represent medicines and devices in use across the NHS |
Theoretically informed (research) | Research designed and carried out based on some existing thinking | |
Therapeutic effect | The degree of healing, relief or cure achieved | |
To take away | TTA | See ‘Discharge prescriptions’ |
To take home | TTH | See ‘Discharge prescriptions’ |
To take out | TTO | See ‘Discharge prescriptions’ |
Toolkit | A series of processes, models and documents available online to guide NHS organisations in implementing electronic prescribing systems | |
Typology | A classification by sort | |
Unlicensed medicine | A medicine that is not covered by a product licence issued by the Medicines and Healthcare products Regulatory Agency | |
Verified | An order or prescription that has been confirmed as safe to supply or administer |
Appendix 26 Patient and public involvement in health information technology research: top 10 tips for researchers
-
Include PPI at the very beginning of your research project.
-
Think it through to be clear about your PPI expectations, aims and strategy, then be prepared to be flexible.
-
Have genuine support for PPI from the top of the research team. Top leadership will probably be crucial to the success of PPI.
-
Appoint a designated research team member who is the ‘go-to’ member of staff for PPI matters, for both the academic and the lay team members.
-
Choose your PPI representatives wisely. The minimum recommended number is two, one of whom could be an experienced PPI representative and a co-applicant and the other a more novice lay representative.
-
Lay representatives for HIT research do not need to be IT enthusiasts or knowledgeable about HIT systems. (The HIT expertise, along with any other academic expertise needed to carry out the research, should be with the staff members.)
-
Take PPI evaluation seriously. Evaluation is important to keep checking how your PPI strategy is working in practice and to track the outcomes from PPI.
-
Lay representatives may want training in research, but they may not. They might simply want support to carry out their PPI role well. Staff members can need support/training too, to make the best use of the resource that PPI brings.
-
Patient and public involvement members can support each other, learn from each other and bounce ideas off one another, and inviting two or more lay representatives to team meetings can give them more confidence to speak out.
-
The context for PPI is one of learning. HIT PPI will be different every time: different individuals, team sizes and dynamics, and different research topics and funding availability. Be prepared for everyone, staff and lay team members alike, to learn from the experience.
Appendix 27 Unpublished lay summaries
Reproduced with permission, personal communication, 2021.
Cresswell et al.55
Electronic prescribing (ePrescribing) systems show considerable promise for making health care more safe, efficient and cost-effective. ePrescribing systems have been shown to lower prescribing errors and costs. While these systems are not yet in many English hospitals, there are plans to introduce them into most hospitals over the next 5 years.
Choosing an ePrescribing system is not straightforward. There are many systems available, coming with different capabilities and at varying costs. While one system might work better for one hospital, a different system could work better for another. Currently, there are no standardised guidelines to help hospitals decide which ePrescribing system might be best for them.
This paper looks at the perspectives of health professionals who have a prescribing role in hospitals that already use an ePrescribing system or in organisations that are planning to do so in the near future. We asked such health professionals from all over England to fill out a questionnaire to provide us with their views.
We found that the majority of our study participants felt unclear about which ePrescribing system should be chosen. They wanted guidance on how to choose a system, as well as guidance on setting up the systems in their hospitals. They also wanted good communication between an ePrescribing system and the other, local health-care computer systems.
We conclude that hospitals in England need more guidance on how to choose and to set up a new ePrescribing system. Lessons that have been learned from hospitals that have already set up systems should be shared with hospitals that are soon to go through this process. More research is needed to investigate the outcomes for hospitals of using ePrescribing systems.
Cresswell et al.50
Health-care environments are complex. Trying to integrate a new technology, such as an electronic prescribing (ePrescribing) system, is difficult and often comes with many frustrations. Health-care workers and health-care organisations need time to adapt to using a new technology. The benefits of having the new system may not be seen until after quite some time.
In this paper we offer important points for hospitals to consider before and during the process of setting up a new health-care technology. We use our experiences of studying various health-related technologies to give 10 pointers that should help health-care professionals and organisations to set up these new technologies.
-
Before selecting a technology:
-
Agree on the goals for setting up the technology. In other words, what problems should the new technology fix and what improvements should the technology support?
-
Will the technology affect the whole organisation or will it target a specific task? Agreement on this point needs input from management teams, as well as from the people who will be using the technology on a day-to-day basis (such as doctors and nurses).
-
Consider all of the different options available. It is helpful to observe other health-care organisations that have already set up new technologies. It is important to consider how well a system will work in your own setting and how much the new technology will cost.
-
-
Choosing a system:
-
Choose a system that works well for the organisation as a whole, as well as for individual patients and health-care workers. It is difficult to find a system that pleases everyone so, usually, compromises are needed.
-
-
Setting up the system:
-
Aim to prioritise getting benefits for patients and health-care workers from the new technology as early as possible. The setting up process should support communication between those managing the setting up process and those using the technology.
-
Consider the hardware that supports the new technology. For example, the system will need a strong wireless connection to work properly.
-
Train staff just before the new technology is introduced. Training should be hands-on and simulate how the technology would actually work day to day. Some individuals will need more training than others and different types of training modules should be available for different people.
-
-
Once the technology is in use:
-
Keep evaluating the technology and get feedback from the users. This feedback can help organisations use the technology in better ways in the future.
-
Allow for the technology to change as the organisation changes its goals.
-
Leave enough time for the organisation to see benefits from a new technology. Once these benefits are noticed, they should be assessed to make sure the technology is being used in the best way.
-
These 10 pointers will, it is hoped help health-care organisations to avoid common mistakes in the setting up of new technologies.
Thomas et al.69
Prescription errors made in hospitals can cause unnecessary inconvenience, illnesses and deaths. Monitoring the types, frequency and severity of these errors is crucial to preventing such errors. ‘Prescribing indicators’ – viewable effects of prescription errors – are generally seen by medical professionals as a sound way to record, measure and help stop errors being made. However, prescribing indicators are rarely very exact and they point more to matters of quality rather than safety. A standard opinion on the importance of different prescription errors does not exist. Therefore, as yet, there is no validated list of important prescribing errors to help prescribers in hospitals to work more safely. This study aimed to provide such a list.
We carried out two rounds of surveys of hospital pharmacists and doctors, who reported severe and/or highly frequent prescribing errors from both paper-based and electronic prescription systems. In the first round of the survey, participants identified 210 prescribing indicators. For the second round of the survey, the full list of reported indicators was returned to study participants in the hope of creating a more focused list. This second round produced a new and smaller list of 89 prescribing indicators, but with an additional 71 new indicators being suggested by our participants. The authors of the study then selected 20 of those new indicators for inclusion in the revised list, which brought the list total to 109. Of the prescribing errors that our survey had now identified, 80 of the 109 were assessed to be of high or extreme risk when the scores for likelihood and severity were considered.
Compiling and standardising prescribing indicators can be a very useful tool for understanding and preventing prescription errors in hospitals. It is hoped that our new validated list of important prescription indicators will help to refine and tailor the alerts issued in ePrescribing systems with clinical decision support, and so help hospital doctors and pharmacists to prescribe more safely for patients.
Yao et al.85
This study aimed to understand the cost-effectiveness of a service intervention to improve the transfer of patient care from hospitals to community organisations (clinical handovers).
Health professionals are undecided whether or not new services for understanding emergency preparedness (i.e. the ability to respond to health-care emergencies) represent a sound investment. In this paper, the authors describe creating a way to estimate the expected cost-effectiveness of proposed new services, which they tested by evaluating a potential service intervention to improve clinical handovers.
The new method focuses on economic evaluation at the pre-implementation stage before a new service or intervention has actually been introduced. Applying the method to a service intervention to improve clinical handovers required the authors to guess the number of potential adverse events and which adverse events were potentially preventable. Adverse events were classified by severity and duration, but not frequency. Afterwards, the authors estimated the level of success of the proposed intervention, by asking experts their opinion on the effectiveness of the intervention. After incorporating the experts’ opinions into the evaluation, the authors then estimated the costs associated with categories of adverse events and the savings resulting from the intervention.
Health policy reforms for emergency preparedness and response are being hampered by poor understanding of the health economics of proposed service interventions. This study offers a method that could be applied to evaluations of service interventions in general, while the interventions are still at the design stage. For this reason, the study might help with projected health economic evaluations of health service emergency preparedness interventions.
Cresswell et al.48
Electronic prescribing (ePrescribing) may help to improve the quality, cost and efficiency of health care. There is much interest in setting up computer systems across the UK for hospital prescribing, but progress with getting ePrescribing systems into hospitals has been very slow. We are working to develop a toolkit as an online resource for hospitals to provide them with help and advice for setting up such systems.
This paper shares our work so far in putting together this toolkit, which we are developing using information from the literature on ePrescribing, our own experiences and findings from early studies in this area. Importantly, we encourage users of ePrescribing systems to view setting up their system as a journey because much work is required before and after the initial phase of getting a new system.
In the toolkit, we give recommendations for each stage of implementing an ePrescribing system. Recommendations for hospitals include, but are not limited to:
-
identify a hospital’s needs for a system
-
put together a team to help with the setting up process
-
look to other hospitals for help in understanding what different types of systems can offer
-
involve as many of the people who will be using the system, from as many different professions as possible, when choosing a system
-
build strong relationships with the people who supply the system
-
have different types of training for the different types of people who will be using the system
-
put all necessary hardware and software in place before setting up the system so it can run efficiently and effectively
-
recognise that the process is ongoing and will continually change.
The toolkit recommendations will, we hope, be useful to hospitals as they judge their needs for an ePrescribing system, choose a system, plan the process of setting it up, set up the system and maintain the system over time.
Coleman et al.134
Clinical decision support for computerised provider order entry is an online system that helps health professionals to prescribe medicines in the right amounts at the right times for the right patient. Medicines can have damaging effects if they are administered wrongly. For this reason, systems can issue ‘alerts’ to health professionals who are prescribing for patients. Prescribing is often complex. Therefore, a clinical decision support system might issue many alerts, which can distract the prescriber and can lead to ‘alert fatigue’, where they the alerts start to be ignored.
Some clinical decision support systems might also issue too few alerts, which could result in a doctor or pharmacist making a prescribing error and causing an ‘adverse drug event’.
Today, there is no agreement or standard for the alerts in clinical decision support systems. The purpose of this study is to explore how clinical decision support alerts should look. The study authors wanted to understand what experts thought about the alerts in clinical decision support and, further, wanted to come to some agreement on the gaps in current clinical decision support capabilities. European experts in clinical decision support and computerised provider order entry were invited to participate in the study, and their contributions were vital to the study’s findings.
The authors list what they believe to be the most important knowledge gaps in clinical decision support alerts. A perfect system would be ‘100% sensitive and 100% specific’. Current systems can be very sensitive, but they are often lacking in specificity. To correct this, clinical decision support systems need to be made more sensitive and more specific than they are today. The authors also found that allowing prescribers to personalise the screens on their clinical decision support systems, as a person would personalise their smart phone, could be a good improvement. They thought that if doctors and pharmacists could customise their alert systems, they would be more likely to pay attention to them. The authors said that there were differences of opinion on the best timing and frequency of prescribing alerts. The authors, in conjunction with the experts, suggested that an alert should be displayed as early as possible in the prescription process and, ideally, the alert should be shown only once.
While the authors identified a number of problems with current clinical decision support, they agreed that much more research is needed to better understand the best ways to set up clinical decision support alerts.
They conclude that the use of clinical decision support systems within computerised provider order entry is increasing, especially in the USA and, therefore, this study is an important reminder to medical professionals in the UK and Europe of the need to assess prescribing alerts within computerised provider order entry systems.
Cresswell et al.117
Electronic prescribing (ePrescribing) systems could help to improve the quality of health care by making it more safe, efficient and cost-effective. However, setting up an ePrescribing system in a health-care organisation comes with challenges. ePrescribing systems can make big changes to the day-to-day work of the people who use the system. In addition, the benefits of using an ePrescribing system might take a while to be seen. Organisations that want to set up an ePrescribing system need to make a strong business case to show why it is worthwhile investing in the new system.
Business cases describe the investment that is needed, the expected benefits and the time frames for setting up an ePrescribing system, and that developing a business case is usually one of the first steps in the process of getting an ePrescribing system. Given that there are a lot of unknowns before the new system is set up, it can often be difficult to write a strong business case.
This paper looks at ways to deal with the difficulties of writing a business case. We did this by discussing the matter of business cases with groups of people from all over England who are interested in or who have experience of setting up ePrescribing systems.
We found that business cases should recognise that an ePrescribing system would introduce changes to the whole organisation, not to only some parts of the organisation. To make a good business case, it was important for hospitals to create strong relationships with the supplier of the ePrescribing system. Hospitals should also look to other hospitals with more experience of ePrescribing systems for help. As the setting up process went on, there would probably be changes in the finances of the health-care organisation, so a business case should not be ‘set in stone’. In addition, health-care organisations should distinguish between what they really needed from their ePrescribing system and what they would like from it.
We conclude that it is important to have a business case before introducing an ePrescribing system. Given that many English hospitals are thinking about setting up these systems, how to write good business cases is an important topic to discuss more. Hospitals in England should share lessons with each other, as well as looking to learn from experiences in other countries, when developing business cases for implementing ePrescribing systems.
Cresswell et al.58
Electronic prescribing (ePrescribing) systems may help to improve the quality and cost of health care. Many studies have investigated the short-term outcomes of setting up ePrescribing systems in hospitals, but there is a lack of research looking into the longer-term effects of ePrescribing systems.
This paper looks at differences and similarities between two English hospitals in which ePrescribing systems have been used for at least 2 years. The two hospitals are using different systems. One set up an ePrescribing system to help with ordering patients’ medications, called a computerised provider order entry system. The other set up an ePrescribing system that also includes clinical decision support. This second system is more integrated with that hospital’s other computer systems and it provides more advice for health professionals while they are prescribing. We used a combination of interviews, observations and analysis of ePrescribing system documents to carry out our study.
We found that there were not many differences between the two different types of ePrescribing systems that we looked at. We found that both of the hospitals reported benefits as well as negative effects from setting up an ePrescribing system. The reported benefits mostly came from using the data collected from the systems to improve quality of care. Staff members in both hospitals were frustrated that not enough computers were available for accessing the ePrescribing systems. In both hospitals, staff members were also frustrated by the amount of time it took to log in whenever they wanted to look at a patient’s medications or to write a prescription.
We conclude that these two different types of systems are similar in the sorts of challenges and benefits that they had produced in a 2-year period after being set up. We believe that 2 years is too short a time fully to understand all the benefits that an ePrescribing system could bring and that the information held in these systems is not yet being used as much or as well as it could be to benefit the hospitals. We recommend that hospital staff and policy-makers have more realistic expectations about the costs, benefits and timelines for benefits from ePrescribing systems. We also recommend that future research tracks the benefits from ePrescribing systems after a longer period of the systems being in place. It is important that future research into the longer-term effects of having ePrescribing systems should study a wider range of hospitals than we looked at in this study.
Mozaffar et al.37
More and more hospitals in England are moving from paper-based to computer-based systems for prescribing and managing the administration of medicines to improve patient safety. At a basic level, these computer systems, often referred to as ePrescribing systems, are replacing the old paper-based drug charts.
This paper investigated the different ePrescribing systems that are available. We searched published articles and data available online (such as news articles, hospitals’ own websites, suppliers’ websites and NHS England data sources) to identify the various ePrescribing systems in use or in the process of being set up. We also spoke to experts to ask about additional systems and their status in UK hospitals.
We found that there is a wide range of ePrescribing systems available to English hospitals, including 13 hospital-wide applications and a range of specialty systems (e.g. for chemotherapy). These were either stand-alone systems or integrated into the hospital’s other computer systems. More than half of the ePrescribing systems were from international suppliers. We found very few hospitals were using the same systems.
We conclude that the market for ePrescribing systems in England is still in its early days, and ongoing review of system suppliers and their products is required to assist hospitals going digital.
Lee et al.49
Electronic prescribing (ePrescribing) systems have the potential to increase the safety and efficiency of health care. Currently, there is limited experience or knowledge of how best to set up these systems in the UK. Our team is developing an online toolkit that will support hospitals to go through the process of setting up an ePrescribing system.
This paper looks at how the toolkit might provide support for those setting up ePrescribing systems in UK hospitals. Creating a user-friendly technology and a user-friendly toolkit require input from the users themselves. Therefore, we designed a questionnaire and gave it out to pharmacists, NHS managers, information technology staff, doctors and suppliers of ePrescribing systems who were at a national conference on ePrescribing. The questionnaire asked what people needed at different stages in the process of setting up an ePrescribing system. We used the answers to help determine how useful it might be to have an ePrescribing toolkit for hospitals and what content it should have to make it most helpful.
We found that there was great interest in having a toolkit that could give more help with setting up ePrescribing systems. We found that the toolkit would be most useful if it provided guidance for each stage of setting up a system. It should have online tools for planning and overseeing the system, as well as useful information from hospitals that had already been through the process. People were aware of the challenges of developing a toolkit that was general enough to help different hospitals across the country but at the same time could be specific enough to help particular groups of people in particular settings.
We conclude that there is clearly a need for more support for hospitals setting up and using ePrescribing systems. An online toolkit can offer reliable and up-to-date help. A toolkit also allows its users to share their experiences and ideas. We realise that the value of a toolkit will become more evident only once it is being widely used and people can share their knowledge and experience. This questionnaire study was only the first step in creating an ePrescribing toolkit. Future research should seek views from a wider range of users and especially from hospital patients whose care will be affected by ePrescribing systems.
Lilford et al.81
Health information technology is an area of computer technology concerned with the design and development of systems to generate and store health-care-related information and make use of that information. Health-care organisations that use health information technology, for instance, such as an ePrescribing system, are expected to have lower costs, improved efficiency and reduced errors while also providing better services and patient care.
To know if health information technology systems are really as good as might be expected, we need methods to study the effects, or end points, of using health information technology systems in health-care organisations. Here, the authors seek to develop a protocol for evaluating the cost-effectiveness of ePrescribing systems in four hospitals in the UK. In two of the hospitals, ePrescribing systems are to include extensive clinical decision support. In addition to ordering medications, systems with clinical decision support offer health professionals advice and onscreen alerts to aid decision-making while they are prescribing. In the other two hospitals, the ePrescribing systems are more basic systems.
The method that the authors describe for evaluating the ePrescribing systems is a statistical method called Bayesian probability logic. This is a type of statistic that uses knowledge of prior events to help to predict future events. For a cost-effectiveness evaluation of ePrescribing systems, we need to consider the costs and the benefits of limiting the number of adverse drug events. To do that requires identifying adverse events, the expected health benefits, the cost of the health information technology system and the cost consequences of avoided adverse events. No single event can totally capture the effectiveness of an ePrescribing system, and these authors describe observing changes in error rates and adverse events in four error categories (i.e. of errors resulting in death, permanent disability, moderate disability and minimal effect). The authors suggest using both quantitative and qualitative data from different sources to find evidence for or against the cost-effectiveness of ePrescribing systems.
This protocol is designed to help address the criticism that evaluations of health information technology systems do not capture all of the relevant end points. The authors argue that evaluations of ePrescribing systems cannot ignore multiple end points merely because they are difficult to observe and to analyse. This protocol seeks to include as many variables as possible in the cost-effectiveness analysis. The authors conclude that there is still room to better understand the full impact of ePrescribing systems on preventing adverse events in hospitals. The protocol they describe offers a method for evaluating the cost-effectiveness of ePrescribing systems, which could also be useful for evaluating other health information technology systems.
Mozaffar et al.24
Electronic prescribing (ePrescribing) systems could improve the quality, efficiency and costs of health care. Standardised ePrescribing systems are popular because they can communicate more easily with other computer systems in hospitals. They are also more affordable than a system that is especially designed for one health-care setting. Although many ePrescribing systems are available to buy now, and setting up these systems is a top priority for the NHS, so far, their uptake in English hospitals has been very slow.
This paper looks at why the uptake of ePrescribing systems has been so slow. To do this, we carried out interviews with companies that sell these systems, the vendors, and with people who are using ePrescribing systems. We wanted to understand the viewpoints of these two different groups. We also observed meetings between vendors and users of ePrescribing systems to help us understand the relationship between these two groups. In addition, we hoped to understand how the market for ePrescribing systems had developed over time.
We found that there were big differences in the range of capabilities offered by standardised ePrescribing systems and in the particular requests made to vendors by hospitals that had taken standardised systems. Most ePrescribing systems had been designed in other countries, usually the USA. This caused some difficulties because English hospitals differ greatly from American hospitals. Vendors and buyers went through many phases of redeveloping a system after it had been installed to try to make it fit the hospital better. That process was time-consuming. In addition, if the hospital requested changes that were too specific to that hospital, the vendor could not make the changes that the hospital wanted.
We conclude that the development of standardised ePrescribing systems for English hospitals is still at an early stage. We recommend that vendors take more time to decide on how to respond to change requests from hospitals before trying to set up ePrescribing systems throughout the UK. We also recommend that these systems are evaluated continually. The issues brought up in this paper will be important for other countries too, as they begin to adopt ePrescribing systems.
Lee et al.54
In England, the former National Programme for Information Technology was introduced with the aim of providing an electronic health record for every NHS patient in the country. At the time, the National Programme for Information Technology was the largest, public, health sector health information technology programme ever undertaken. Post National Programme for Information Technology, the UK government now wants the NHS to go paperless by 2018. However, some worry that the goal of going paperless is setting too difficult a task too soon.
This qualitative study aims to explore how the joint procurement model that was in place during the National Programme for Information Technology has affected the design, customisation and usability of electronic prescribing (ePrescribing) systems in use today. To explore the impact of the National Programme for Information Technology procurement process, we studied two sites in which an ePrescribing system had been jointly procured within one of the National Programme for Information Technology’s geographical areas.
We found that the procurement process, relationships with the system supplier and the strict contractual arrangements under the National Programme for Information Technology had contributed to reported usability problems with the ePrescribing system and to difficulties with implementing and customising the ePrescribing system. The National Programme for Information Technology arrangements had limited the sites’ ability to make changes to the ePrescribing system, which affected the system’s usability and the users’ perception of its usability.
This study illustrates how important the early stage of procurement is in the process of implementing an ePrescribing system. The joint procurement model, as used during the National Programme for Information Technology, appeared to make setting up an ePrescribing system more difficult and less efficient in the two sites we studied.
Cresswell et al.25
Health-care workers now argue that any assessment of HIT needs to account for its technical uses and its social uses. International observers of health care are also interested in this ‘sociotechnical’ perspective, a view that assumes that both organisational and human (socio) factors and information technology systems (technical) factors will influence the success of HIT systems. Sociotechnical evaluations of HIT, therefore, attempt to study the processes associated with using a new technology in its social and organisational setting. This approach to HIT evaluations could lead to learning lessons relevant for assessing information technology systems in other industries too.
Sociotechnical evaluations that use multiple methods are becoming more popular and are likely to offer a new research approach for some scholars. There have been clear benefits of sociotechnical analyses of the efficiency of HIT. However, there are issues with this approach, mainly that a sociotechnical analysis offers subjective qualifications rather than objective answers. There are, therefore, opportunities for scholars to decide on the role and scope of the sociotechnical investigation and analysis of HIT, and to understand if it demands further research in its own right.
The authors hope that there will be more sociotechnical evaluations of HIT systems in the future. Given the interests of financial and policy groups in developing HIT to improve health outcomes (and to reduce health-care costs), the authors argue that greater use of sociotechnical evaluations will help to illustrate the big idea of the relationships between technology, health-care organisations and patients. They believe that such understanding will improve patient well-being, reduce health-care costs and offer lessons for other industries.
Schofield et al.135
NHS hospitals in England are putting ePrescribing systems into place. ePrescribing systems have been successfully used in primary care settings.
Introducing such systems in hospitals can be problematic because they force pharmacists to change the way that they work. We do not know yet if such changes make hospital pharmacists work more or less efficiently or more or less safely. To understand such changes and the effects they bring, we need to develop a protocol to measure and analyse the impact of ePrescribing in hospitals. It is very important to identify and assess the impact if we wish to design HIT systems that aid (rather than hinder) the work of pharmacists.
We conducted a time-and-motion study in six wards at one NHS trust in England, with two periods of observations (one before and one after introducing the ePrescribing system). Researchers followed the hospital pharmacists, and recorded and time-stamped the details of all the work tasks that they carried out. They made a record of all the tasks that the pharmacists completed, how often they completed each task and the time that they took for each task. Then the researchers analysed changes in these measures before and after getting an ePrescribing system.
We also interviewed pharmacists to ask their opinions of the impact of ePrescribing on their work. Pharmacists’ expectations of the likely impact of ePrescribing were also explored.
This study has limitations. The results of the study relate to two English NHS hospitals. The pharmacists who took part may have altered their behaviour during the study, perhaps because of fears of being seen as ineffective. However, this is the first and only time-and-motion study of hospital pharmacists before and after using an ePrescribing system and, for this reason, it provides a useful baseline and guide for future studies.
Cresswell et al.136
Doctors, pharmacists and others are interested in improving current health care. For example, many hope to move from a doctor-centred model of care to a more patient-centred approach. Using HIT is seen as a very important part of making these changes happen in our health-care services. However, English hospitals are being slower to take up HIT than might be expected, and the authors of this paper seek to explore why this is so.
The authors review a number of factors that have contributed to the successes of HIT in English hospitals in recent years, and point out the complex challenges to hospitals of setting up HIT systems. They review possible future developments from HIT, such as increased patient involvement; the sharing of medical records; more patient-centred and integrated care for patients; using smart devices; sharing of health-related data sets; and new uses for those data. They argue that HIT systems should be viewed as aids to doctors and also as an important source of data to support re-designing health-care services. This is known as a Learning Health Systems approach. In the Learning Health Systems approach, different computer systems are linked with each other and share databases of information to make improvements to medical practice and to patient care. According to the authors, implementing HIT is a ‘work in progress’, which will aim to tackle new challenges as well as possible through taking a Learning Health Systems approach.
The authors conclude that health-care professionals need to understand that HIT systems are not final products but rather they are tools to help with a never-ending task of improving health outcomes.
Cresswell et al.118
Electronic prescribing (ePrescribing) systems may increase the safety, efficiency and quality of health care. There are many different types of these systems available and hospitals are responsible for choosing the system that will work best for them. There is a great need to collect knowledge and experience to help hospitals to choose a new system and to set it up. The companies that sell these systems, the vendors, have the most experience of setting up ePrescribing systems and could be an important resource for other hospitals, but the vendors’ knowledge is rarely used more widely.
This paper looks at some of the challenges of setting up ePrescribing systems from the point of view of the vendors. It also considers how those challenges might be addressed, using insights gained from vendors. We held group discussions with nine participants, who represented six different vendors.
We found that there were currently no guidelines for hospitals that were choosing and setting up an ePrescribing system. Vendors recommended that hospitals should employ people with a lot of experience in that particular hospital to help out with decision-making and setting up the new system. Vendors suggested that hospitals often moved too quickly through the process of setting up an ePrescribing system. They recommended that hospitals should begin by setting up a simple system and give the staff time to get used to those changes first. Vendors also mentioned the importance of strong relationships between vendors and hospitals. Good relationships would help hospitals to choose the system that would best meet their needs.
We conclude that vendors of ePrescribing systems can provide important insights to hospitals on how best to choose and set up a system. Guidelines for hospitals are needed to support them through the decision-making and setting up phases. These findings may be useful to English hospitals introducing ePrescribing systems, as well to health-care organisations in other countries.
Lee et al.45
Parts of this section have been reproduced with permission from Lee et al. 45 This is an Open Access article distributed in accordance with the terms of the Creative Commons Attribution (CC BY 4.0) license, which permits others to distribute, remix, adapt and build upon this work, for commercial use, provided the original work is properly cited. See: https://creativecommons.org/licenses/by/4.0/. The text below includes minor additions and formatting changes to the original text.
Electronic prescribing (ePrescribing) in hospitals is expected to improve the quality of patient care. Patients themselves, however, have not had the opportunity to voice their views on ePrescribing systems. Anecdotal evidence suggests that many patients may understand the potential benefits of electronic health-care systems. For instance, it is thought that patients recognise that ePrescribing could improve information-sharing between health professionals and patients. This study looked at the significance of ePrescribing systems to patients.
We interviewed representatives from national patient groups as well as hospital patients in a renal ward in which an ePrescribing system was in use. We also spent time on that ward and took notes about what we observed. We organised the information collected in these three ways by keeping a focus on perceived benefits of ePrescribing; patients’ awareness and understanding of their prescribed medications; and patients’ views on medications prescribed (or advised) on admission and on discharge from hospital.
We found that, overall, patients seemed to view ePrescribing in a positive light. However, the ePrescribing system in the site we studied did not seem to have improved patients’ involvement in the prescribing process or to have improved medication counselling at discharge. We found that ePrescribing systems did not make patients feel that they had more information about their medications. Indeed, by removing the paper charts at the end of hospital beds, patients seemed to have less access to information about their medications and care than before. We did not identify technical reasons to do with the ePrescribing system itself to explain why patients’ involvement in hospital prescribing had not apparently changed for the better.
We conclude that ePrescribing could bring new opportunities for patients and health-care professionals to improve patient involvement. Having an ePrescribing system is not enough on its own to promote better patient involvement. To achieve that also needs other changes to take place, such as policy changes and changes in health-care professionals’ approach to prescribing for hospital patients.
Cresswell et al.52
This section has been reproduced with permission from Cresswell et al. 52 Published by John Wiley & Sons. © 2016 Health Research and Educational Trust.
The implementation of health information technologies, including electronic health records, computerised provider order entry and clinical decision support systems, promises health organisations to achieve a range of benefits. However, these benefits can be achieved only if the systems are used as intended. Implementing health information technologies is not without challenges and risks, and not all obstacles can be resolved during implementation. To overcome challenges and to realise benefits in the long run, implementers need to pay more attention to the needs of prescribers and other users of these systems after implementation. However, given that most research focuses on the challenges prior and during implementation, we have little understanding of issues that users experience in later periods of system use. For this reason, this study aims to explore a long-term perspective on user engagement through investigating the range of ways in which health-care workers and organisations familiarise themselves with new technology and how they adopt them in their workplaces over time.
To begin, we define that user engagement is a process with three elements: (1) the need to involve different types of users in the selection and design of a technology, for example by giving them the opportunity to be heard; (2) the need to make sure that the workforce supports using a new technology; and (3) the need to give users the opportunity to change the technology as they discover its features and capabilities over time.
During the period of 2011 and 2015, we approached six hospitals in England to conduct 173 interviews, but also to collect 17 relevant documents and to observe health-care professionals in practice on 24 different occasions. Observations in the field were noted down on paper and the interviews were audio-recorded and later transcribed. We analysed the data by looking for recurring themes related to our interest on user engagement. As a result of analysing and discussing preliminary results among researchers in the team, we identified three dominant themes and a set of subthemes within each.
The first theme indicates that users were more willing to support a new technology if they perceived it as beneficial in terms of improving patient safety or everyday work. The second theme identifies a variety of negative responses, including resistance and workarounds, to the introduction of a new technology. Finally, the third theme points to an increase of sustained use over time as users’ competence improved and changes in software eased the application of the new technology.
Our study shows that new computerised provider order entry and clinical decision support systems were eventually successfully adopted in our case study hospitals. However, our work indicates that the adoption process could be smoother and quicker if more attention would have been paid to user concerns to sustain user engagement in contrast to system installation. In conclusion, we suggest hospitals take a long-term perspective when considering the implementation of new technologies and computerised provider order entry/clinical decision support systems in particular.
Cresswell et al.119
Electronic systems that facilitate prescribing, administration and dispensing of medicines (ePrescribing systems) are at the heart of international efforts to improve the safety, quality and efficiency of medicine management. Considering the initial costs of procuring and maintaining ePrescribing systems, there is a need to better understand how to generate more financial benefits and how to generate them sooner.
In this study, we sought to find out what representatives of different sectors think and what experiences they made about the return of investment from ePrescribing systems in UK hospitals and what lessons can be learned for future developments and implementation strategies within health-care settings.
We conducted international round-table discussions with 21 participants from different backgrounds, including policy-makers, health-care organisations, academic researchers, vendors and patient representatives. We audio-recorded the discussions and transcribed them for a thematic analysis. For this, we identified recurring and emerging themes addressed during these discussions using the qualitative analysis software NVivo10.
There was an over-riding concern that it is difficult to realise direct financial benefits from ePrescribing systems. The underlying reasons included substantial fixed costs of care provision, the difficulties in radically changing the medicines management process and the lack of capacity within NHS hospitals to analyse and exploit the digital data being generated. Any future data strategy should take into account the need to collect and analyse local and national data (i.e. within and across hospitals), agreeing on qualities for comparison to measure progress (i.e. baseline measurements) and clear standards guiding data management so that data are comparable across different health-care organisations.
Cresswell et al.56
Electronic prescribing systems in hospitals might be able to make health care safer and more efficient. However, the health-care professionals in hospital are still trying to find the best ways to use these systems. Sometimes, the people who use these systems (doctors, pharmacists and nurses) find it difficult to use the system the way that it was designed to be used. In these cases, health-care professionals might create a ‘workaround’. Workarounds are new behaviours or new ways of using the electronic prescribing system that allow someone to accomplish their work in a way that was not planned for by the designers of the system. It is important to be aware of the different types of workarounds that may exist to decrease any safety risks and redesign any systems or hospital procedures to better fit the system and the health-care professionals who use it.
This research had three goals: (1) to identify the workarounds that were created and used by health-care professionals when an electronic prescribing system was set up in hospital; (2) to understand why the workarounds happened; and (3) to understand how workarounds might create safety risks. To accomplish these goals, this research studied workarounds at six hospitals in England that had recently set up electronic prescribing systems. To study these workarounds, this research involved interviews with health-care professionals who used the systems, as well as observation of the systems being used in hospital.
This research identified different types of workarounds: informal workarounds (practices that had not been approved by hospital management) and formal workarounds (practices that were understood by leaders in the hospital to be necessary to use the system). The main reason for creating and using a workaround was that certain practices within a hospital are very complicated and diverse. This makes it difficult for an IT system to control all aspects and all possible situations in the prescription process. Sometimes, doctors, pharmacists and nurses would use another electronic system or paper and pen to record information that should have been entered into the electronic prescribing system instead.
This work found that workarounds can cause new safety risks and, at the same time, decrease other risks to safety that may have been introduced by electronic prescribing systems. Instead of seeing workarounds as a bad thing, workarounds should be seen as helpful, for example helpful in the design of future systems that will fit better with the work of the people who use them. This paper emphasises that workarounds might change over time. Understanding the difference between informal and formal workarounds is important in planning for potential risks causes by the use of these systems.
Overall, workarounds should not be seen as a bad thing. They need to be better understood to make sure that the quality of health care does not decrease when an electronic prescribing system is set up in hospital.
Cresswell et al.137
Resistance to antibiotics is a huge threat to health across the world. Resistance to antibiotics means that the medicines used to treat infections (antibiotics) are no longer strong enough to fight against the germs that cause infections. This results in infections that last longer and are more dangerous to patients. As the resistance to antibiotics keeps increasing, it may be unsafe in the future to get routine surgeries. For example, caesarean sections or hip replacements might become very risky procedures.
One way to prevent the increase in resistance is for doctors, pharmacists and nurses to make sure that the antibiotics that they prescribe and give to patients are the most effective antibiotics for the particular patient. This means that the antibiotic targets the specific germ that is causing a patient’s infection. It also means that the antibiotic is prescribed at the correct dose and for the correct length of time. However, choosing the most effective antibiotic is difficult and not straight forward. To help select the most effective antibiotic, some hospitals are turning to information technology systems to provide support and guidance for doctors, pharmacists and nurses.
This paper looked at research from 1997 to 2015 to understand how a certain type of information technology system, electronic prescribing systems, can help doctors, pharmacists and nurses to use antibiotics correctly and effectively in hospital. Electronic prescribing systems help to bring together all of the information available about a particular patient to guide decisions about which medicines to prescribe. These systems also connect doctors, pharmacists and nurses with guidelines for prescribing antibiotics and information about medicines.
This paper provides an overview of electronic prescribing systems that help doctors, pharmacists and nurses to prescribe and give the correct antibiotic. This paper found that many of the electronic prescribing systems that helped prescribe the correct antibiotic are used on particular, specialty wards (such as intensive care), rather than in an entire hospital. Most of the systems were very specific and designed to work in one particular hospital, rather than designed more generally to be able to work in many different hospitals. This paper also provides ideas about how information technology systems can help prescribe correct antibiotics in ways that have not yet been used. This paper suggests that the designers of these electronic prescribing systems will need to be more active in changing the systems to help with the correct prescribing of antibiotics. In addition, hospitals will have to help to tailor their system to meet local antibiotic prescribing guidelines.
There still is a lot that is unknown about how information technology can best support antibiotic prescribing in hospital. This paper calls for more research into understanding how electronic prescribing systems can help antibiotic prescribing. This paper also recommends that, alongside the technical aspects of electronic prescribing systems, people working in the hospital also need to help encourage antibiotics to be prescribed correctly.
Cresswell138
Across the world, there are large investments in health information technologies to try to decrease the number of medical errors and costs, and to increase the quality of care. However, there is still a lot of difficulty when setting up health information technology systems and getting them to work in hospitals. These difficulties can come from the technical aspects of the systems but also from the interactions between the system and the people who use the systems (such as doctors, pharmacists and nurses).
Health information technology evaluations follow the process of a system being set up in hospital and can help to better understand what works when setting up a health information technology system. A good understanding of what works when setting up these systems is helpful for other hospitals that are trying to set up these systems, so they can know what to do and what to avoid when getting their system up and running. Given that evaluations can provide hospitals with information about how to best set up an information technology system, evaluations can also help to avoid any safety risks.
Although these evaluations are really important, they are also complex. This paper describes the different types of evaluations that could be used to better understand how health information technology systems are set up. By describing the different types of evaluation, this paper outlines the necessary steps of an evaluation and also provides advice to people who carry out these evaluations.
During an evaluation is it important to pay attention to these different aspects:
-
the characteristics of the health information technology system
-
the characteristics of the hospital that the system is being set up in
-
how the health information technology system is first brought into the hospital and introduced to the people who use it, as well as what happens when it is being set up
-
any changes that have happened as a result of setting up the system.
It is important that evaluations should always happen during the same period of time that the health information technology system is being set up. Evaluations that happen only after an information technology system has been set up will miss everything that happened during the set-up of the system. Given that there are a lot of challenges during the system set-up, it is important to understand these challenges as well as any challenges or changes after the set-up of the system. Therefore, evaluations should span the entire process of the system set up, rather than just a short period of time at the end when a set-up is complete.
Evaluations should also include different types of understandings, such as overarching statistical understandings as well as more specific and detailed understandings from people in the hospitals who use the system. Evaluations can focus on different parts of the set-up of information technology systems. The most common focus for evaluations are the technical parts of the system and the interaction between these technical parts and the people who use them. Another focus of evaluations is the hospital as whole and understanding how the set-up of a system makes changes on a larger scale, for the whole hospital. It is important for evaluations to focus on a few different aspects, from the technical components of a system to the people who use the system and to how the whole hospital is affected by the system. Evaluations also help to learn about what happens during the set-up of a system within one hospital and then to compare what happens within other hospitals that are also setting up systems.
Mozaffar et al.47
Parts of this text have also been reproduced with permission from Mozaffar et al. 47 with permission from BMJ Publishing Group Ltd.
Hospital ePrescribing systems offer a wide range of benefits to improve patient safety. However, like other attempts to implement new hospital health information technology, they may also introduce new areas of risk. Despite efforts made by many other researchers in identifying these risks, the development and use of ePrescribing systems is still leading to numerous unintended consequences, which may undermine improvement and threaten patient safety. These negative consequences need to be analysed in the many stages a system goes through including design, implementation and use. This study, therefore, aimed to understand the roots of these reported threats and identify possible approaches to avoid or mitigate such threats.
We analysed six English hospitals over an extended period of time to learn about the implementation and adoption of electronic prescribing systems. To this end, we collected data that included interviews with people affected by the system, observations of implementation meetings and system use, and a collection of relevant documents. Our data set included 214 interviews, 24 observations and 18 documents. After analysing each hospital individually, we compared the findings across hospitals to find recurring patterns.
Based on our data, we found a range of reasons that can cause unintended safety threats. We built a taxonomy of factors, which means that we sorted these reasons into groups of different classes, underlying these safety threats. The first class of factors encompasses poor system design, including lack of support for complex medication administration regimens (such as infusions, lack of effective sharing of data between different systems, and lack of effective automated decision support tools, such as automated alerts for drug intolerances). Another class of factors covers the inappropriate use of systems, including too much reliance on the system and introduction of workarounds. Finally, a third class of factors causing unintended safety threats include inadequate implementation strategies that result from partial roll-outs, simultaneously running new electronic systems and old paper-based systems, and a lack of appropriate training. We have identified a number of system and organisational strategies that could potentially avoid or reduce these risks.
Imperfections in the design, implementation and use of ePrescribing systems can give rise to unintended consequences, including safety threats. Hospitals and suppliers need to implement short- and long-term strategies in terms of the technology and organisation to minimise the unintended safety risks.
Cresswell et al.139
Electronic health records and other health information technologies are used across the world in hospital care and primary care. However, it is still unclear how these information technology systems directly help patient care and the health of populations. It is important to outline strategies for the future about how these systems will be used in health care and, in outlining these strategies, is it important for national governments to be involved. This paper offers six suggestions for governments to draw the most value and financial benefit from these systems.
Appendix 28 Patient and public involvement induction day documents role guide
Role guide: National Institute for Health and Care Research ePrescribing patient public involvement, updated: January 2014
Introduction
The PPI strategy for the NIHR ePrescribing evaluation was conceived as a cross-cutting component of this research programme. It was envisaged that PPI group members and members of the academic research team would work closely together throughout the programme and that, individually and collectively, members of the PPI group might identify different viewpoints, questions and priorities from those of the academic and NHS members of the team.
The PPI group leader, Antony Chuter, was a co-applicant on the funding application and his early input contributed to the development of the ePrescribing research proposal, including the PPI strategy.
1. Role description for patient and public involvement group members
Work package lay representatives are asked to commit to attending up to four face-to-face meetings in 1 year and to taking part in up to six telephone contacts (up to four WP related, initiated by your WP contact, and up to four informal chats with the PPI group lay lead to monitor the PPI strategy), in addition to co-operating with an annual evaluation of the PPI strategy (one audio-taped interview).
Specifically, PPI members attached to a programme WP are asked to:
-
have attended an induction day to learn about the ePrescribing programme and the PPI strategy, and identify any support/training requirements for participating
-
attend and contribute to subsequent twice-yearly meetings of the whole PPI group, in which you may be asked to work on PPI-specific tasks or take part in a specific, PPI-related ‘focus group’ for the programme
-
attend and contribute to two programme steering group meetings in the course of 1 year (held in Birmingham or Edinburgh)
-
participate in up to four WP-specific telephone calls in the course of 1 year
-
assist the ePrescribing team to understand some lay views and questions relevant to the ePrescribing programme overall and to your WP in particular
-
raise any concerns or problems that may arise with the WP leader and/or with a PPI group leader (lay: Antony Chuter; staff: Ann Robertson)
-
participate in activities to evaluate the PPI strategy (i.e. informal reviews with the group’s lay leader and an annual interview)
-
notify a PPI group leader of any intention to withdraw from participation in the programme.
Patient and public involvement members attached to the iPSC are asked to:
-
have attended an induction day to learn about the ePrescribing programme and PPI strategy, and identify any support/training requirements for participating
-
participate in iPSC meetings twice per year
-
assist the iPSC to maintain awareness and understanding of the relevance of PPI in its oversight of the whole programme
-
raise any concerns or problems that may arise with the iPSC chairperson and/or with a PPI group leader (lay: Antony Chuter; staff: Ann Robertson)
-
participate in activities to evaluate the PPI strategy
-
notify a PPI group leader of any intention to withdraw from participation in the programme.
2. Requirements of the role
To fulfil the role, all PPI group members are required to:
-
have experience of the NHS or research as a patient or carer, or as an advocate/representative of patients, carers or members of the public
-
be able to contribute relevant personal knowledge, experience and views to inform the work of the programme
-
be able to represent a broader range of lay perspectives to the programme work, beyond the directly personal, where relevant
-
be willing to accept a context of learning about PPI for both the lay and the staff members of the programme team to develop PPI in NHS research
-
be able to work effectively and appropriately as a team member.
3. Commitment
Members of the PPI group are invited to participate for 3 years in the first instance, with the possibility for continued involvement in the programme for a further 2 years. The programme will finish in October 2016. Participation is voluntary and PPI members are free to withdraw at any time. It would be helpful to the team to know the reason(s) if a PPI member chooses to leave but members are not required to help in this way.
4. Confidentiality
Patient and public involvement volunteers must not share any confidential information (personal or commercial) that they learn from being part of the programme with anyone outside the programme team. If a PPI member is not sure whether or not any information is confidential, please seek guidance from your WP lead or committee chairperson.
5. Finances
Reasonable expenses will be covered by the PPI budget within the programme budget, such that PPI members are not ‘out of pocket’ for taking part in PPI-related activities. In addition, lay members will be paid a medical volunteer honorarium of £100 per face-to-face meeting.
6. Other
All members of the PPI group are entitled to expect to be treated with consideration and courtesy at all times by others in the programme. E-mails or telephone calls to the PPI group lay and staff leads should receive a response in a reasonable time (i.e. within a few days); all e-mails and telephone calls by PPI members to their WP contacts should always receive a response in a similarly timely manner.
In the event of a volunteer having a complaint about their involvement in the programme, please inform either of the PPI group leaders in the first instance or, if you prefer, you may contact one of the programmes co-principal investigators (Aziz Sheikh, Jamie Coleman) if the complaint or concern relates to Antony or Ann.
Appendix 29 Patient and public involvement induction day documents expenses guide
National Institute for Health and Care Research ePrescribing patient and public involvement group: general guidance for expenses claims
Updated: March 2014
By now, everyone should have an electronic copy of an expenses claim form, which gets sent to Rosemary Porteous (whose contact details are on your contacts sheet, and are given again below). Stamped addressed envelopes are being sent out by Rosemary Porteous, so if you have not already given Rosemary your home address, and would like some stamped address envelopes for posting your expenses claims, please e-mail or ring her with your address details.
Rosemary Porteous, XXXX.
The first time that you submit an expenses claim, please complete and return the bank details form to Rosemary also. After that, you will only ever need to send in a completed claims form; this has a space on it that you can use to notify Rosemary should your bank details change in the future.
The general idea of expenses is that you should not be out of pocket. Therefore, we will refund the cost of your travel to a NIHR ePrescribing-related meeting, and the cost of drinks – non-alcoholic – and food that you have to buy on your travels (because you are not at home or in your usual workplace to make your own coffee or snack). Please keep and submit all your receipts.
Travel by public transport: the policy is to book standard (second-class) fares and, where possible, the least expensive flights. Very occasionally you may find, as I have done, that booking a train fare online in advance has given the option of 1st class tickets that are cheaper or as cheap as a standard fare, in which case, please use your judgement and don’t deprive yourself of a 1st class ticket that is actually more economical! In general, booking well ahead gives better ticket prices so, whenever possible, please do make your travel plans earlier rather than later.
If you are using a private car, we will refund mileage at 45 pence per mile. If your proposed journey is more than a 100-mile round trip, please consider whether or not public transport would be a more economical option.
Please feel free to request paper copies of documents from the programme’s senior research secretary (Rosemary Porteous) for steering group or iPSC meetings. These are usually available at the meeting on the day for people attending in person. However, if you find you are printing out large documents for your NIHR ePrescribing work at home, do remember that we don’t want you to be out of pocket; if a claim for printer paper or ink is justified, please don’t hesitate to include reasonable, additional costs on your claims form (again, please include receipts).
For meetings that you are attending in person for NIHR ePrescribing-related work, please also claim an honorarium of £100 for the day. The maximum total honorarium payment in a single financial year (April to March) is £500. Expenses claims will be reimbursed promptly throughout the year. Honorarium claims will be filed by Rosemary and the total due to you paid in a single payment at the end of each financial year.
Teleconferences will be on a free phone number.
If you have a question not answered here, you are probably not the only one so please do ask Ann or Antony and we will be pleased to try to help.
List of abbreviations
- ADE
- adverse drug event
- AE
- adverse event
- CDS
- clinical decision support
- CI
- confidence interval
- COTS
- commercial off the shelf
- CPOE
- computerised provider order entry
- DHSC
- Department of Health and Social Care
- EHR
- electronic health record
- GP
- general practitioner
- HIT
- health information technology
- II
- information infrastructure
- IMPACT
- Investigate Medication Prescribing Accuracy for Critical error Types
- INR
- international normalised ratio
- iPSC
- independent Programme Steering Committee
- IT
- information technology
- NCC-MERP
- National Coordinating Council for Medication Error Reporting and Prevention
- NIHR
- National Institute for Health and Care Research
- NRES
- NHS Research Ethics Service
- NSAID
- non-steroidal anti-inflammatory drug
- PPI
- patient and public involvement
- QALY
- quality-adjusted life-year
- RR
- relative risk
- SD
- standard deviation
- VTE
- venous thromboembolism
- WP
- work package
Notes
Supplementary material can be found on the NIHR Journals Library report page (https://doi.org/10.3310/KSRS2009).
Supplementary material has been provided by the authors to support the report and any files provided at submission will have been seen by peer reviewers, but not extensively reviewed. Any supplementary material provided at a later stage in the process may not have been peer reviewed.