Notes
Article history
The research reported here is the product of an HSDR Rapid Service Evaluation Team, contracted to undertake real time evaluations of innovations and development in health and care services, which will generate evidence of national relevance. Other evaluations by the HSDR Rapid Service Evaluation Teams are available in the HSDR journal.
The research reported in this issue of the journal was funded by the HSDR programme or one of its preceding programmes as project number NIHR134285. The contractual start date was in January 2021. The final report began editorial review in November 2021 and was accepted for publication in February 2022. The authors have been wholly responsible for all data collection, analysis and interpretation, and for writing up their work. The HSDR editors and production house have tried to ensure the accuracy of the authors’ report and would like to thank the reviewers for their constructive comments on the final report document. However, they do not accept liability for damages or losses arising from material published in this report.
Permissions
Copyright statement
Copyright © 2022 Saunders et al. This work was produced by Saunders et al. under the terms of a commissioning contract issued by the Secretary of State for Health and Social Care. This is an Open Access publication distributed under the terms of the Creative Commons Attribution CC BY 4.0 licence, which permits unrestricted use, distribution, reproduction and adaption in any medium and for any purpose provided that it is properly attributed. See: https://creativecommons.org/licenses/by/4.0/. For attribution the title, original author(s), the publication source – NIHR Journals Library, and the DOI of the publication must be cited.
2022 Saunders et al.
Chapter 1 Introduction
Summary
-
The workload for primary care general practices in the UK has been growing rapidly.
-
Telephone triage means that a patient asking to see a general practitioner (GP) or other primary care professional calls usually speaks to a receptionist first, who records a few details. The patient is then telephoned back by a GP or primary care professional. At the end of this return telephone call, either the issue is resolved or a face-to-face appointment is arranged.
-
Before the COVID-19 pandemic, telephone triage was already being introduced in the NHS to help manage demand for GPs’ services and help GPs organise their workload.
-
The use of telephone triage greatly accelerated at the start of the pandemic.
-
People living with multiple long-term health conditions have worse experiences of access to primary care, including telephone access. Improving health services for people with multimorbidity remains a priority.
-
We quantitatively explore the impact of introducing telephone triage on inequalities in primary care access for people living with multiple long-term health conditions.
-
The three evaluation questions (EQs) are:
-
EQ1 – Considering people living with multiple long-term health conditions only, does a telephone triage approach affect how quickly people can see or speak to an appropriate primary care professional?
-
EQ2 – What is the size of that effect relative to the effect on people contacting a general practice who do not have multiple long-term health conditions?
-
EQ3 – Are there any subgroups of the population with multiple long-term health conditions who are particularly affected (either positively or negatively) in terms of how quickly they see or speak to an appropriate primary care professional, both generally and when a telephone triage approach is used?
-
Background
Over the last 20 years, the workload in primary care in the NHS has increased substantially. 1 There has been an increase in general practice consultation rates, the average duration of a consultation and the total patient-facing clinical workload. This increase in workload is driven by a number of factors, including the changing demographics of the UK. Increased life expectancy since 1980 has led to an ageing population2 with increased health needs and an increase in the clinical complexity of the patients managed in primary rather than secondary care. 3 Overall, primary care workforce numbers have increased only a relatively small amount during the same period,4 with inequitable distribution of workforce resources across the country. 5,6
Primary care access remains a health policy priority because of GPs’ role providing some health care and acting as a gatekeeper to other health care, and also because there is evidence that better health-care access leads to cost savings across the health-care system, with reduced use of secondary care. 7–10 Policy initiatives have been introduced to improve patient access to primary care, including extended opening hours for general practices and changes to how patients can access a GP. 11–13 The NHS Long Term Plan included a commitment to new forms of health-care access. 11 The 2019 GP contract, by which the NHS buys GP services for the population, made it a requirement for GPs to offer online consultations by April 2020. 14 Despite these initiatives and plans, patients report that their experiences of trying to make an appointment with a GP worsened between 2011 and 2017, with no evidence of reductions in inequalities between subgroups of patients. 15
The combination of this increased workload and the clinical complexity in primary care, along with the importance of good primary care access for patients and the wider health-care system, is driving the development of new approaches and models in primary care. 16,17 Implemented at general practice level, one of these new models is a telephone triage approach. Before the COVID-19 pandemic, telephone triage was already slowly being introduced in the NHS as a tool to help GPs manage demand for their services. Telephone triage is a health-care service innovation in which every patient asking to see a GP or other primary care professional calls and usually speaks to a receptionist first, who records a few details. The patient is then telephoned back by a GP or primary care professional. At the end of this return telephone call, either the issue is resolved or a face-to-face appointment is arranged.
Early evaluations of telephone triage approaches found that their introduction meant that patients saw or spoke to a clinician more quickly, but that the number of patient contacts increased overall, with no evidence of a reduced GP workload or of cost savings. 18–22 These evaluations also found evidence of strong variation between general practices in the impact of introducing telephone triage on patients’ experience of their practice and on changes in secondary care use. There was also variation in how well practices adapted to the approach, with a substantial minority of practices reverting to non-telephone triage approaches after trialling the innovation.
At the start of 2020, only a minority of general practices had implemented the approach,23,24 and the majority of consultations in primary care were face to face. 25 However, during the first quarter of 2020, much of the work of general practices moved to a remote (almost entirely telephone) triage approach to reduce practice footfall at the start of the pandemic. 26,27 The motivation for introducing telephone triage and remote consultation during the COVID-19 pandemic was to minimise the risk of COVID-19 contact for patients, rather than to support managing demand for GPs. 28
The implementation of telephone triage was also different during the pandemic than previously, with a much more rapid roll-out and often a blurring of the lines between triage and consultation. Hybrid models (i.e. including combinations of online and telephone triage) and online symptom checkers (e.g. eConsult29) for patients to use before contacting their surgery were also introduced during the pandemic, potentially introducing new barriers to access for some patients. In addition, these changes occurred within the wider context of the other health-care challenges at the start of the pandemic, with changes in how primary and secondary care are delivered. 26
The Royal College of General Practitioners has highlighted the need for models of telephone triage in primary care to ensure that roll-out and implementation is equitable and does not increase inequalities in access. 27 The main concerns regarding inequalities in access from new methods of interacting with GPs are differing levels of information technology literacy in the population, language barriers, and physical or sensory disabilities such as deafness. A recent systematic review exploring the (pre-COVID-19) impact of telephone triage and remote primary care consultations on health inequalities found that there was limited available evidence on the subject. 30 Evidence about ethnicity and socioeconomic factors (e.g. income, education or employment) was mixed and none of the studies in the review reported on the impact of remote consultations on quality of care or clinical outcomes, or for people living with multiple long-term health conditions. The authors of the review recommended that use of remote consultations should be treated with caution until the inequalities impact on clinical outcomes and quality of care is known. Research is needed to understand the inequalities impact of the introduction of telephone triage from a health perspective, as the evidence base to date has focused on the overall impact on workload and costs.
Designing and delivering equitable health care for people living with multiple long-term health conditions was a priority for the NHS pre pandemic11,31,32 and remains so, particularly as COVID-19 has had a differentially large impact on this group. 33 Understanding the impact of service innovations for people with multimorbidity is important, not least because previous evaluations have found innovations designed to improve care for people living with multimorbidity have had a mixed or small impact,34 despite evidence of poorer experiences of care and health-care outcomes for this group. 35,36 People living with multiple long-term health conditions have worse experiences of access to primary care, including telephone access. 36,37 In addition, the challenges of managing multiple long-term health conditions often exist alongside other disadvantages, for example material deprivation. 38,39 Ethnic minorities, older or younger patients with multiple health conditions, people in or out of employment, and those living in urban or rural areas may experience further challenges specific to their situations. 40
Robust quantitative evaluation of the effect of introducing technologies, such as telephone triage, on the nature and extent of health inequalities is challenging owing to data (un)availability, required sample size and risk of false-positive findings because of multiple statistical hypothesis tests. High-quality data identifying particular minority or vulnerable patient groups are often unavailable and, if they are available, this may be after a considerable time lag only, which is unhelpful for promptly informing policy-makers. 41 In addition, for an impact evaluation to adequately assess whether or not an intervention has a differential impact for some patients, a sample size at least four times larger is required than the sample size when no subgroups are investigated. 42 Unless testing whether or not an innovation works in a specific group within the population was prespecified, such analyses make little sense if the innovation does not have an overall impact on the outcomes of interest. However, evaluations often find only a small or no impact. 43 False-positive findings are a concern when testing all possible subgroups without prespecified hypotheses a priori,44 and the required sample size would be particularly large when there is only a small impact.
Given the current need to understand the inequalities impact of introducing telephone triage, the ongoing (as of autumn 2021) policy and political debate about primary care access and consultation, and the importance of delivering equitable care for people living with multimorbidity, we have explored the impact of introducing telephone triage on inequalities in primary care access for people living with multiple long-term health conditions. Our methodological approach addresses the challenges that are usually present for inequalities impact evaluations by:
-
using pre- and post-COVID-19 pandemic survey data collections with large sample sizes [the GP Patient Survey (GPPS)45 and Understanding Society (USoc)46]
-
measuring patient characteristics and multimorbidity [in the analysis of both GPPS and USoc, we used a count of self-reported long-term health conditions (zero, one, two, three, or four or more conditions) as a measure of multimorbidity]
-
investigating an intervention that has demonstrated a large impact (in this case, introducing telephone triage has been found to significantly reduce the time taken for a patient to see or speak to a GP19).
The likelihood that telephone triage will remain a key feature of primary care and the fact that the inequalities impact of this approach has not been well described are important context for this research.
In this research, we have sought to answer the following questions: does introducing telephone triage affect access to primary care differently for people living with multiple long-term health conditions from other people, and are there any groups of patients with multiple morbidity who are particularly affected? Box 1 presents our EQs in full.
EQ1: Considering people living with multiple long-term health conditions only, does a telephone triage approach affect how quickly people can see or speak to an appropriate primary care professional?
EQ2: What is the size of that effect relative to the effect on people contacting a general practice who do not have multiple long-term health conditions?
EQ3: Are there any subgroups of the population with multiple long-term health conditions who are particularly affected (either positively or negatively) in terms of how quickly they see or speak to an appropriate primary care professional, both generally and when a telephone triage approach is used?
Chapter 2 Methods
Summary
-
In this chapter, we describe the two data sets used in the analyses presented in this report.
-
We used data from GPPS to explore the inequalities impact of introducing telephone triage on the time taken to see or speak to a GP for people with multiple long-term health conditions pre COVID-19, between 2011 and 2017.
-
In addition, we used data from USoc to explore inequalities in access to primary care between April and November 2020.
-
We describe the included survey questions and constructs from both surveys measuring access to primary care, and respondent characteristics.
-
We also describe how long-term health conditions are measured in each survey, and how responses describing long-term health conditions are combined to create a measure of multiple long-term health conditions or multimorbidity.
-
The five analyses undertaken are described, including how they answer each of the EQs.
-
Details are given of our approach to survey weighting, missing data and patient and public involvement (PPI).
Data
Commissioned by NHS England, GPPS is an annual survey that is sent to about 125 patients in every general practice in England. Eighty-four per cent of the UK population lives in England; because of differences in commissioning arrangements, data are not available for the rest of the UK. 47 The survey collects process measures of care quality, patient characteristics and information on their long-term health conditions. 48,49 A total of 154 practices that had started using a telephone triage approach between 2011 and 2017 were identified by two private telephone triage providers and included as ‘intervention’ practices in this analysis.
Understanding Society is the UK Household Longitudinal Study, which began in 2009 and includes respondents in Northern Ireland, Scotland and Wales as well as England. About 40,000 households were interviewed and the study has annual follow-up. The USoc COVID-19 study is a survey that specifically asks the main study participants about their experiences of the COVID-19 pandemic. It started in April 2020 and this analysis includes data from six waves between April 2020 and November 2020, alongside information on participant characteristics collected from the main study. We used both telephone and online responses to the USoc regarding the COVID-19 waves to minimise non-response bias. 50
Measures of primary care access
From GPPS, we considered responses to the question ‘How long after initially contacting the surgery did you actually see or speak to them?’, which has answer options of ‘on the same day’, ‘on the next working day’, ‘a few days later’ and ‘a week or more later’. We included responses only from people who had had an appointment with their GP in the past 6 months. In preliminary analyses, we compared both linear and binary coding for this question. For the linear coding, we recoded responses on a scale from 0 (longest wait) to 100 (shortest wait), with the middle categories equally spaced at 33 and 67, to allow analyses to interpret changes on this scale as a percentage-point difference. For binary coding, we considered ‘saw or spoke to a GP on the same day’ against all other responses. As the findings were consistent across the two approaches, we continued with linear coding only.
In USoc, we considered the responses to the question ‘Thinking about your situation now, have you been able to access the NHS services [GP or primary care practice staff] you need to help manage your condition(s) over the last 4 weeks?’, which has response options of ‘yes, in person’, ‘yes, online or by phone only’, ‘no, not able to access’, ‘no, decided not to seek help at this time’ and ‘not required’. For analysis, we recoded responses into four separate measures that identified whether or not:
-
someone had needed to access their GP
-
they had tried to contact their GP if they needed access
-
they were able to access their GP if they tried to contact them
-
this access was online/by telephone or face to face.
From April to September 2020, these questions were asked of only those respondents who reported current treatment for a health problem or at least one long-term health condition; in November 2020, these questions were asked of all survey respondents.
Long-term health conditions
In analysis of both GPPS and USoc, we used a count of self-reported long-term health conditions (zero, one, two, three or four or more conditions) as a measure of multimorbidity. In analyses comparing people living with multimorbidity with those without, we included people who reported two or more long-term health conditions as living with multimorbidity. This is an established approach in health services research and, although weighted approaches allow consideration of differences in the strength of the association between specific morbidities and a given outcome,51 increasing multimorbidity measured in terms of the number of long-term health conditions is associated with mortality,52 prescribing,53 worse health-related quality of life35 and greater health-care utilisation. 54 Similarly, alternative measures such as frailty indices are primarily based on weighted and unweighted counts of long-term health conditions, and would be likely to measure similar constructs to the unweighted count used here. 55
The number of people living with multimorbidity was not expected to be the same across the two data sets because the definition of multimorbidity depends on the number and prevalence of conditions, and the long-term health conditions differ between the two surveys, as does the geographical coverage. 56,57 However, because the approaches to measuring long-term health conditions (a single survey question with multiple response options) and multimorbidity (count of conditions) are consistent across the two surveys, we expect the directions of relationships between multimorbidity and primary care access to be consistent.
In GPPS, respondents were asked ‘Which, if any, of the following medical conditions do you have?’, with response options including 13 long-term physical health conditions, plus additional responses for a long-term mental health problem and ‘another long-term condition’. In USoc, respondents were asked ‘Has a doctor or other health professional ever told you that you have any of these conditions?’, with response options including 21 long-term physical health conditions, plus additional responses for a long-term mental health problem and ‘other long standing/chronic condition’. Full answer options for both surveys are given in Report Supplementary Material 1.
The long-term health condition measure in GPPS was previously validated against external nationally representative data for England, with acceptable concordance. 35 Further details of the long-term health condition questions in USoc are described in Report Supplementary Material 2.
Sociodemographic characteristics
In GPPS, we used survey measures of age (in 10-year age groups), sex, ethnicity (grouped into five categories: white, Asian, black, mixed and other), working status (defined as in employment or not) and small-area postcode-level data for measures of rurality and deprivation.
In USoc, we used survey measures of included characteristics, grouping age into 20-year age groups because of the overall smaller sample size for these data than for GPPS, and also included responses to a question asking whether or not someone was shielding during 2020. Net household income was taken from the most recent measure available from the last three waves pre COVID-19, and divided by a coefficient provided by the Organisation for Economic Co-operation and Development to account for household sizes. Then, equivalised household income was split into quintiles and used for analysis.
Analyses
We carried out a series of five linked analyses to explore the relationship between multimorbidity and access to primary care when a telephone triage approach is in place. In terms of data, we included survey respondents to both GPPS and USoc with complete data collection for long-term health conditions, age, sex, ethnicity, and income (USoc) or deprivation (GPPS). For missing data concerning employment, rurality (GPPS and USoc) and shielding (USoc only), exclusions were made on an analysis-by-analysis basis. For both GPPS and USoc, the analysis sample changed across analyses depending on whether an analysis considered one point in time or looked at changes over time. For each analysis, we described the specific analysis question, the data set and included periods.
In our first analysis (analysis 1), we described the characteristics of respondents in both data sets, overall and stratified by number of long-term health conditions, to understand the characteristics of people living with long-term health conditions in England (GPPS) or the UK (USoc).
In our second analysis (analysis 2), using USoc only, we explored the percentage of all respondents each month who had needed to access their GP and the percentage who had tried to contact their GP, and explored if they were able to access their GP and whether or not this access was face to face. Analyses were stratified by month during 2020 and weighted to be representative of the population of the UK using survey weights developed by the USoc team to account for sampling and non-response. 58,59
In our third analysis (analysis 3), we used survey responses to GPPS from 2011 to 2017 to estimate the impact of introducing telephone triage on the time taken to see or speak to a GP among people with multimorbidity. This was an adjusted analysis. An adjusted analysis analytically accounts for differences between intervention and control practices, and changes in primary care access overall that occur over time. We used a difference-in-difference analysis framework, looking at whether or not the change from introducing telephone triage was the same for people with and without multimorbidity. We used a model structure developed as part of earlier work and described in full elsewhere,20 and adjusted for age, sex, ethnicity, deprivation, survey year and general practice, and we also allowed the impact of switching to a telephone triage approach to vary between practices. We included GPPS responses from 154 practices, identified by two commercial telephone triage providers, that had implemented telephone triage before 2017, looking at changes before and after the date on which telephone triage was implemented in each practice. We used a random sample of 10% of all other general practices in England as a control in these analyses and excluded people who had not attended a GP in the previous 6 months.
General practices implemented telephone triage at different times, and so the number of practices in which it had been implemented increased across the survey time series:
-
2012 – six practices
-
2013 – 44 practices
-
2014 – 79 practices
-
2015 – 122 practices
-
2016 – 145 practices
-
2017 – 154 practices.
For the analysis sample, we excluded people who could not remember how long it had taken them to see or speak to a GP, or who were missing responses to this question; people with missing data on the number of long-term health conditions they had; and people who had not seen a GP within 6 months of completing the survey. We then split the surveys into three groups: non-telephone triage practices, telephone triage practices before implementation and telephone triage practices after implementation.
We investigated the change in the time taken to speak to a clinician after switching to telephone triage for all those consulting a GP and then for those with multiple long-term health conditions. This addressed the first EQ: considering people living with multiple long-term health conditions only, does a telephone triage approach affect how quickly people can see or speak to an appropriate primary care professional?60 We then investigated the effect of the number of long-term health conditions on the change in time taken to speak to a clinician. This answered the second EQ: what is the size of that effect relative to the effect on people contacting a general practice who do not have multiple long-term health conditions?
In our fourth analysis (analysis 4), we explored the relationship between a person’s number of long-term health conditions and whether or not they had needed to access their GP, whether or not they had tried to contact their GP if they needed access, whether or not they had been able to access their GP and whether or not this access was face to face. We used logistic regression with cluster standard errors to account for the fact that some respondents may have more than one response from different months, and adjusted for age, sex, ethnicity, household income and survey wave, using data from USoc during 2020.
In our final analysis (analysis 5), to answer the EQ ‘Are there any subgroups of the population with multiple long-term health conditions who are particularly affected (either positively or negatively) in terms of how quickly they see or speak to an appropriate primary care professional, both generally and when a telephone triage approach is used?’, we used both GPPS and USoc. For GPPS, we carried out a cross-sectional analysis of responses from the 2015/16 and 2017 survey waves. We used linear regression to explore the relationship between multimorbidity and time taken to see or speak to an appropriate professional. We then added interaction terms to the analysis to explore whether or not this relationship changed for subgroups of the population (age, sex, ethnicity, deprivation, rurality and employment). In USoc, we explored whether or not the relationships described in analysis 4 (the relationship between a person’s number of long-term health conditions and whether or not they had needed to access their GP, whether or not they had tried to contact their GP if they needed access, whether or not they were able to access their GP and whether or not this access was face to face) varied between groups. We used logistic regression to explore the interaction between the effect of multimorbidity and age, sex, ethnicity, household income, employment, rurality and whether or not someone was shielding. In both analyses, we applied the Bonferroni correction to account for multiple testing.
Details of how these five analyses map to the three EQs for this project are presented in Table 1.
Analysis | EQ |
---|---|
Analysis 1: descriptive analysis of the characteristics of survey respondents with multiple long-term health conditions | Relevant to EQs 1–3: preliminary analyses to describe the analysis samples and data sets, with a focus on understanding the characteristics of respondents with multimorbidity |
Analysis 2: descriptive analysis of primary care access (whether or not people needed to access care, whether or not they decided to try to access care if they had a health-care need, whether or not they were able to access care and, if they were, whether this access was face to face or remote) between April and November 2020 using USoc | Preliminary analysis relevant for EQs 1 and 2 (post COVID-19). The main analysis in this report is based on data from GPPS pre COVID-19, which includes a measure of how quickly people can see or speak to an appropriate primary care professional. Because USoc does not collect this measure of primary care access, we instead described four related measures of primary care access from USoc |
Analysis 3: inequalities analysis of introducing telephone triage for people with multimorbidity, exploring the impact on time taken to see or speak to a GP (pre the COVID-19 pandemic) using GPPS | Analysis 3 is the main analysis that answers EQs 1 and 2 |
Analysis 4: analysis of inequalities in access to primary care for people with multiple long-term health conditions between April and November 2020 using USoc | Analysis 4 provides additional evidence relevant for EQs 1 and 2 on primary care access for people with multimorbidity post COVID-19 |
Analysis 5: analysis before and during the COVID-19 pandemic using both GPPS and USoc exploring whether there is any variation in primary care access among people with multimorbidity based on age, sex, ethnicity, household income/deprivation, employment, rurality or whether or not someone was shielding (USoc only) | Relevant to EQ3 (GPPS), plus an analysis answering EQ3 (USoc) exploring alternative measures of primary care access post COVID-19 |
Weighting
In survey research, weighting is an important tool to account for the possible non-representativeness of respondents by analytically assigning more or less ‘weight’ to some responses, depending on how likely the person who responded was to have been sampled or returned their questionnaire. In this evaluation, one researcher (EG) attended a 2-day survey workshop run by the USoc team and asked the team for advice on the best weighting approach for these analyses. 59 For GPPS, we used the single survey weight provided with the survey data set in unadjusted analysis. However, for multivariable analyses of GPPS, we did not weight the analysis, as the weights are not appropriate for data sets combined across years. Nevertheless, we did include the important variables that were used in deriving the weights in our analysis, so will have accounted for issues of possible non-representativeness in that way. We know that weighting is important because the response rate to GPPS across years is usually between 36% and 38%48,49 and, although USoc has a more complex design, both non-response at recruitment and attrition over time are concerns addressed through weighting. 61
For analysis 1, through descriptive analysis of data from GPPS (sociodemographic characteristics stratified by the number of long-term health conditions), we estimated the percentage of respondents with each characteristic among people with and without multimorbidity from the 2017 survey wave. We used the survey weights that account for sampling and non-response, and weighted the analysis to the age and sex profile of each included general practice, which resulted in percentages that are weighted to be representative of the population of England. 49 Multivariable analyses were unweighted. Descriptive analyses of data from USoc (sociodemographic characteristics stratified by the number of long-term health conditions; analysis 1) used data from the April 2020 wave, using the cross-sectional weight to weight to the population of the UK.
Analysis 2 looked at the percentage of people who had needed access to their GP, tried to contact their GP if they needed access and were able to access their GP, and looked at whether or not this access was face to face, stratified by month during 2020. Data from each monthly COVID-19 wave of USoc were included separately, with the appropriate cross-sectional weight for that month. This means that the calculated percentages are nationally representative for each included month. In multivariable analyses using USoc, exploring the relationship between multimorbidity and primary care access, all six of the monthly waves were included (with an adjustment for wave), and the analysis was weighted using the appropriate longitudinal survey weight for the most recent included month (November 2020). This weighting accounts for both survey non-response and sampling, and means that the results are weighted to be representative of the UK. 59
Sample size considerations (evaluation questions 1 and 2)
After exclusions, we split the survey responses into three groups: non-telephone triage practices, telephone triage practices before implementation and telephone practices after implementation. We looked at the number of survey responses available for analysis in each group:
-
non-telephone triage practices – 2,945,553 responses in total
-
telephone triage practices before implementation – 27,758 responses in total
-
telephone triage practices after implementation – 31,538 responses in total.
The number of survey respondents stratified by the number of long-term health conditions people reported are presented in Table 2. Full details of the study sample size calculations are given in the published protocol. 60
Number of long-term health conditions | Telephone triage practices before implementation (n) | Telephone triage practices after implementation (n) | Non-telephone triage practices (n) | Total (n) |
---|---|---|---|---|
Zero | 9535 | 11,202 | 1,047,315 | 1,068,052 |
One | 9143 | 10,120 | 956,831 | 976,094 |
Two | 4951 | 5436 | 512,785 | 523,172 |
Three | 2434 | 2770 | 253,869 | 259,073 |
Four or more | 1695 | 2010 | 174,753 | 178,458 |
Supplementary analyses
The main sensitivity analyses for the statistical models exploring the impact of introducing telephone triage have been reported previously and we did not repeat them in this evaluation. 20 These previous sensitivity analyses included analyses considering only those general practices that continued to use a telephone triage approach after introducing it and did not revert to a non-telephone triage approach, and an analysis exploring a binary outcome variable (whether or not someone was able to see or speak to a GP on the same day).
In the main analysis, in addition to exploring whether or not the impact of telephone triage was different for people with multimorbidity using GPPS, we also considered whether or not there were any differential effects based on other respondent characteristics.
In addition, we explored whether the relationship between multimorbidity and two other measures of primary care access in GPPS (overall experience of access and telephone access) were similar to or different from the measures considered in the main analysis among survey respondents from 2015/16 and 2017. Details of the full question wording for these additional questions are given in Report Supplementary Material 3.
In USoc, the main sensitivity analyses explored two further measures of access to health-care services in the community from April to November 2020. We repeated all the main multivariable analyses that had considered access to a GP for outcome measures that explored access to NHS 111 and to prescription medications. Details of the full question wording for these are given in Report Supplementary Material 4.
Computer software
All analyses were carried out using Stata®, version 14.2 (StataCorp LP, College Station, TX, USA).
Included responses
For descriptive analysis in GPPS, after excluding missing data, we included 1,231,568 responses (analysis 1). In multivariable analysis of the same data set (analysis 5) exploring whether or not the relationship between the time taken to see and speak to a GP and multimorbidity varied by age, sex, ethnicity, deprivation, employment or rurality, we included 1,002,396 responses (for details, see Report Supplementary Material 5). For the analysis exploring the impact of telephone triage in 154 intervention practices and a 10% sample of control practices (analysis 3), we included 333,618 responses (for details, see Report Supplementary Material 6). We included responses from 15,775 participants in the USoc COVID-19 survey (analyses 1, 2, 4 and 5) with one to six responses included per person (for details, see Report Supplementary Material 7).
Patient and public involvement
During the course of the research, we met five members of the Brimingham, RAND and Cambridge Evaluation Centre (BRACE) PPI panel, all of whom live with long-term health conditions, in two online workshops. After our first meeting, during protocol development, we critically revised our analysis plan in response to PPI feedback; in particular, this lead to including hearing problems (4.2% of respondents; 56,013 responses in total) separately in the analysis.
At our second meeting, which was to review preliminary findings, we discussed how the PPI panel participants would have responded to the USoc survey questions to make sure that we were interpreting responses appropriately. We also discussed whether or not to include a separate analysis of people with mental health problems. The panel suggested that this would not be appropriate, as there may well be some situations when people with mental health problems would find telephone triage easier or harder, and that it would not be possible to incorporate this distinction into the analysis framework; therefore, we did not take this analysis further. Last, we discussed our interpretation of the results of the analyses with the panel and discussed their reflections. In particular, comments about experiences of access to primary care during the COVID-19 pandemic informed the discussion and implications of this work that are presented in Chapter 4.
In addition, the Plain English summary of the report was reviewed by five PPI advisers. Project updates were also shared with members of the BRACE Steering Group for the duration of the project. The BRACE Steering Group includes BRACE co-investigator Charlotte Augst (Chief Executive of National Voices; London, UK). Updates on the project were given to the BRACE Health and Care Panel in regular e-bulletins.
Changes from protocol
The analyses described in the published protocol based on analysis of data from GPPS are included in this report. 60 In the protocol, we also stated that we would carry out a ‘data review’ to explore whether or not other publicly available data sets were available to support analyses to answer the three EQs. Although we identified USoc as one possible data source in the published protocol, the full analyses using USoc presented here were developed after the protocol was written and data review completed. These analyses are integrated into the report, which allows the report to present data on the inequalities impact of telephone triage collected after the start of the COVID-19 pandemic.
In the protocol, we also stated that we would consider the inequalities experience of respondents with caregiving responsibilities and experience based on sexual orientation. For these analyses, data were available for GPPS only, not USoc; therefore, the results are presented separately in Report Supplementary Material 8–10.
Chapter 3 Results
Summary
-
In this chapter, we report the findings from the five linked analyses carried out to understand the impact of introducing telephone triage on inequalities in access to primary care for people living with multiple long-term health conditions.
-
In analysis 1, we found that people living with multimorbidity are older, live in more deprived areas, are less likely to be in employment and are more likely to have been shielding during 2020.
-
In analysis 2, we found that overall people (with and without multimorbidity) were less likely to try to see their GP if they had a problem requiring medical attention at the start of the COVID-19 pandemic. However, by September 2020, > 90% of people with a problem did try to get in touch with a GP. Of people who did try to access primary care, > 90% were able to make an appointment, and this was the case at any time between April and November 2020. In April 2020, only 20% of all appointments were face to face; this had increased to about 40% by November 2020.
-
In analysis 3, we found that before the COVID-19 pandemic there were differences in the time taken to see or speak to an appropriate primary care professional between people with multimorbidity and those without, both before and after the introduction of telephone triage. However, these differences were small compared with the overall improvement for all patients when a general practice switched to a telephone triage approach.
-
In analysis 4, we found that during the COVID-19 pandemic people living with multimorbidity were more likely to have a problem that meant they needed to see a GP than people with no long-term health conditions or a single condition. However, we found no evidence that there was any difference between people with or without multimorbidity regarding whether or not they tried to access a GP if they did have a problem; whether or not they were able to make an appointment; or whether the appointment was face to face, by telephone or online.
-
In analysis 5, we found little evidence across most measures of access to primary care, both before and during the COVID-19 pandemic, that particular groups of people living with multimorbidity had differentially better or worse experiences of primary care access when considering age, sex, ethnicity, deprivation, rurality, employment or shielding status. We did find variation in the relationship between multimorbidity and time taken to see or speak to a GP or other appropriate primary care professional when looking at age. In particular, among people aged ≥ 85 years, the speed with which it was possible to see or speak to a GP increased with increasing numbers of long-term health conditions.
Analysis 1: survey respondents with multiple long-term health conditions
In our first analysis, we described the characteristics of included respondents to both GPPS (Table 3) and USoc (Table 4); characteristics are weighted to be representative of the population of England (GPPS) or the UK (USoc). Multimorbidity increases with age and is more common among people living in more deprived areas or households.
Characteristic | All respondents, n (weighted %) (N = 1,231,568) | Number of long-term health conditions, n (weighted %) | ||||
---|---|---|---|---|---|---|
Zero (N = 395,614) | One (N = 414,000) | Two (N = 224,978) | Three (N = 112,368) | Four or more (N = 84,608) | ||
Age (years) | ||||||
18–24 | 43,995 (8.1) | 29,985 (13.2) | 10,977 (6.7) | 2322 (3.1) | 514 (1.6) | 197 (0.8) |
25–34 | 107,474 (16.2) | 72,785 (26.6) | 26,532 (12.7) | 5957 (6.4) | 1526 (3.6) | 674 (2.3) |
35–44 | 146,253 (16.4) | 85,228 (23.4) | 43,492 (15.1) | 12,050 (9.2) | 3607 (5.9) | 1876 (4.3) |
45–54 | 205,214 (18.0) | 85,426 (18.6) | 74,081 (20.1) | 28,127 (16.3) | 10,528 (13.2) | 7052 (12.1) |
55–64 | 244,862 (15.7) | 63,995 (10.4) | 93,766 (18.5) | 48,852 (20.5) | 22,152 (19.6) | 16,097 (19.8) |
65–74 | 270,651 (14.0) | 42,150 (5.6) | 99,302 (15.9) | 68,723 (23.2) | 35,317 (25.8) | 25,159 (24.8) |
75–84 | 160,977 (8.2) | 13,379 (1.7) | 51,513 (8.2) | 44,656 (15.2) | 28,036 (20.7) | 23,393 (23.1) |
≥ 85 | 52,142 (3.3) | 2666 (0.4) | 14,337 (2.8) | 14,291 (6.1) | 10,688 (9.8) | 10,160 (12.8) |
Sex | ||||||
Men | 524,390 (46.3) | 145,396 (43.1) | 187,098 (49.1) | 103,232 (48.6) | 50,658 (46.9) | 38,006 (46.0) |
Women | 707,178 (53.7) | 250,218 (56.9) | 226,902 (50.9) | 121,746 (51.4) | 61,710 (53.1) | 46,602 (54.0) |
Ethnicitya | ||||||
White | 1,075,736 (86.2) | 328,446 (82.4) | 365,821 (87.4) | 203,186 (90.3) | 102,097 (91.3) | 76,186 (91) |
Mixed | 10,480 (1.2) | 4746 (1.5) | 3351 (1.1) | 1382 (0.9) | 592 (0.7) | 409 (0.6) |
Asian | 83,133 (7.1) | 37,553 (9.6) | 24,561 (6.3) | 11,217 (4.7) | 5346 (4.4) | 4456 (4.5) |
Black | 34,454 (2.9) | 14,203 (3.5) | 11,375 (2.8) | 4947 (2.1) | 2305 (1.9) | 1624 (1.7) |
Other | 27,765 (2.6) | 10,666 (3.1) | 8892 (2.5) | 4246 (2.0) | 2028 (1.8) | 1933 (2.3) |
Deprivation | ||||||
5, least deprived | 235,754 (19.4) | 81,389 (19.8) | 83,362 (20.4) | 41,959 (19.4) | 18,217 (17.0) | 10,827 (13.6) |
4 | 250,124 (19.7) | 82,725 (19.9) | 87,364 (20.4) | 45,224 (19.5) | 21,069 (18.6) | 13,742 (16.3) |
3 | 252,981 (20.0) | 81,074 (20.0) | 86,439 (20.2) | 46,676 (20.2) | 22,667 (19.7) | 16,125 (19.2) |
2 | 246,239 (20.5) | 78,306 (20.7) | 80,630 (20.0) | 45,132 (20.2) | 23,514 (21.2) | 18,657 (21.9) |
1, most deprived | 246,470 (20.4) | 72,120 (19.6) | 76,205 (19.2) | 45,987 (20.7) | 26,901 (23.4) | 25,257 (28.9) |
Rurality | ||||||
Urban | 1,031,500 (86.2) | 332,975 (87.1) | 342,929 (85.4) | 187,242 (85.3) | 94,940 (85.7) | 73,414 (87.4) |
Rural | 200,068 (13.8) | 62,639 (12.9) | 71,071 (14.6) | 37,736 (14.7) | 17,428 (14.3) | 11,194 (12.6) |
Employmentb (N = 1,183,578) | ||||||
Not employed | 620,003 (40.4) | 107,342 (20.5) | 207,473 (41.3) | 148,558 (60.8) | 85,795 (76.3) | 70,835 (88.7) |
Employed | 563,575 (59.6) | 279,861 (79.5) | 191,898 (58.7) | 65,565 (39.2) | 19,573 (23.7) | 6678 (11.3) |
Characteristic | All responses, n (weighted %) (N = 15,775) | Number of long term conditions, n (weighted %) | ||||
---|---|---|---|---|---|---|
Zero (N = 6140) | One (N = 5288) | Two (N = 2461) | Three (N = 1057) | Four or more (N = 829) | ||
Age (years) | ||||||
0–39 | 3155 (24.2) | 1765 (35.4) | 1053 (26.2) | 234 (10.5) | 69 (9.0) | 34 (5.1) |
40–49 | 2616 (14.2) | 1278 (18) | 887 (14.9) | 300 (10.8) | 97 (8.6) | 54 (5.0) |
50–59 | 3490 (19.2) | 1440 (20.9) | 1183 (19.6) | 499 (18.1) | 205 (15.6) | 163 (15.4) |
60–69 | 3348 (18.2) | 1017 (14.1) | 1103 (17.8) | 664 (22.6) | 305 (25.1) | 259 (23.8) |
≥ 70 | 3166 (24.2) | 640 (11.6) | 1062 (21.5) | 764 (38.0) | 381 (41.7) | 319 (50.7) |
Sex | ||||||
Male | 6504 (45.3) | 2460 (45.4) | 2261 (48.2) | 997 (40.6) | 472 (48.3) | 314 (38.6) |
Female | 9271 (54.7) | 3680 (54.6) | 3027 (51.8) | 1464 (59.4) | 585 (51.7) | 515 (61.4) |
Ethnicitya | ||||||
White | 13,990 (92.9) | 5387 (92.3) | 4690 (92.1) | 2207 (93.9) | 958 (93.8) | 748 (96.0) |
Asian | 1054 (3.7) | 471 (4.3) | 357 (3.9) | 136 (2.4) | 48 (3.5) | 42 (2.1) |
Black | 398 (1.5) | 129 (1.3) | 144 (1.8) | 67 (1.9) | 32 (1.2) | 26 (1.2) |
Mixed | 247 (1.3) | 114 (1.4) | 74 (1.7) | 39 (1.0) | 12 (0.9) | 8 (0.4) |
Other | 86 (0.6) | 39 (0.6) | 23 (0.6) | 12 (0.7) | 7 (0.7) | 5 (0.3) |
Income | ||||||
5, highest quintile | 4133 (21.2) | 1779 (25.1) | 1418 (22.3) | 601 (19.4) | 225 (13.7) | 110 (8.8) |
4 | 3339 (19.5) | 1289 (21.0) | 1172 (20.4) | 505 (18.3) | 222 (17.7) | 151 (12.8) |
3 | 3029 (20.0) | 1148 (20.3) | 1024 (18.9) | 486 (20.4) | 192 (18.3) | 179 (24.7) |
2 | 2664 (19.1) | 983 (16.7) | 831 (17.8) | 425 (20.9) | 216 (26.2) | 209 (26.0) |
1, lowest quintile | 2610 (20.2) | 941 (17.0) | 843 (20.6) | 444 (21.0) | 202 (24.2) | 180 (27.8) |
Urban/ruralb (N = 15,664) | ||||||
Urban area | 11,570 (74.7) | 4551 (75.1) | 3873 (75.1) | 1773 (72.2) | 756 (73.8) | 617 (77.0) |
Rural area | 4094 (25.3) | 1538 (24.9) | 1375 (24.9) | 680 (27.8) | 295 (26.2) | 206 (23.0) |
Employmentc (N = 15,547) | ||||||
Not employed | 6459 (45.9) | 1746 (30.0) | 2084 (42.6) | 1335 (60.2) | 654 (71.0) | 640 (84.9) |
Employed | 9088 (54.1) | 4355 (70.0) | 3098 (57.4) | 1085 (39.8) | 377 (29.0) | 173 (15.1) |
Shieldingd (N = 15,752) | ||||||
Yes | 1222 (9.7) | 82 (1.3) | 392 (9.2) | 284 (14.0) | 208 (22.3) | 256 (31.6) |
No | 14,530 (90.3) | 6056 (98.7) | 4887 (90.8) | 2171 (86.0) | 846 (77.7) | 570 (68.4) |
Analysis 2: primary care access, April to November 2020 (Understanding Society only)
In our second analysis, we described how access to primary care changed between April and November 2020 (Table 5).
GP/primary care | All responses (n)a (N = 15,775) | Month of 2020, n (weighted %) | |||||
---|---|---|---|---|---|---|---|
April (N = 14,012) | May (N = 13,324) | June (N = 12,369) | July (N = 12,091) | September (N = 11,705) | November (N = 12,006) | ||
Needed to access service (out of all responders) | 52,393 | 9153 (48.8) | 8511 (45.5) | 7782 (46.6) | 7578 (43.7) | 7405 (48.2) | 11,964 (41.7) |
Tried to contact service (out of those who needed to see a GP) | 23,562 | 4374 (80.9) | 3895 (85.2) | 3456 (89.0) | 3181 (92.3) | 3519 (92.2) | 5137 (93.1) |
Able to access service (out of those who tried to contact their GP) | 20,906 | 3499 (91.2) | 3340 (90.9) | 3077 (94.0) | 2896 (93.6) | 3272 (93.2) | 4822 (94.6) |
Face to face (out of all GP contacts) | 19,602 | 3200 (20.3) | 3080 (19.5) | 2907 (25.3) | 2728 (29.2) | 3084 (34.4) | 4603 (41.1) |
The percentage of people who reported a health problem requiring primary care attention in the previous 4 weeks stayed relatively constant across survey waves from April to November 2020, at just < 50%. The percentage in November 2020 was slightly lower than in previous months. However, this reduction is likely to be a methodological artefact, as it is consistent with a change in survey design: for the first time, all survey respondents were asked this question in November, whereas only those with existing conditions or those awaiting treatment were asked previously.
The percentage of people with a problem requiring GP advice who then tried to contact primary care for this problem increased steadily from 80.9% in April 2020 to 93.1% in November 2020.
Among people who did try to contact their GP, the number able to access primary care increased from 91.2% in April 2020 to 94.6% in November 2020, with > 90% of people who tried to see their GP managing to get an appointment in all waves.
Face-to-face appointments constituted only 20.3% of all primary care consultations in April 2020, with almost 80% being online or by telephone; the proportion of face-to-face appointments increased to 41.1% by November 2020.
Analysis 3: the impact of introducing telephone triage for people with multimorbidity (the GP Patient Survey)
In our third analysis, we explored the impact of introducing telephone triage (pre COVID-19) for people with multiple long-term health conditions. Overall, for those with and without multimorbidity, there was a 20.79-percentage-point (p < 0.0001) improvement in the time taken to be seen or spoken to by a GP after a general practice adopted a telephone triage approach (approximately translating to a step change of one better response out of the five response options to this survey question). Considering people with multimorbidity only, there was a 21.16-percentage-point improvement (p < 0.0001; Table 6). Therefore, people with multiple morbidity experienced a very slightly greater average reduction than the overall population in the time taken to be seen or spoken to by a GP when telephone triage was introduced. However, this difference was not statistically significant.
Population | Before; adjusted scorea (95% CI) | After; adjusted scorea (95% CI) | Adjusteda change (95% CI) | p-value | |
---|---|---|---|---|---|
Overall | 55.84 (54.91 to 56.77) | 76.63 (74.55 to 78.72) | 20.79 (18.92 to 22.66) | < 0.0001 | |
Only including people with two or more conditions | 56.44 (55.32 to 57.57) | 77.60 (75.17 to 80.03) | 21.16 (19.01 to 23.31) | < 0.0001 | |
Number of long-term health conditions | Coefficient (95% CI) | p-value | |||
Before | After | Change | Differential change | ||
Zero | Reference | Reference | 20.36 (19.29 to 21.44) | Reference | 0.26 |
One | –2.33 (–2.65 to –2.01) | –2.13 (–2.58 to –1.67) | 20.57 (19.60 to 21.53) | 0.20 (–0.15 to 0.55) | |
Two | –2.45 (–2.84 to –2.05) | –2.04 (–2.78 to –1.30) | 20.77 (19.79 to 21.75) | 0.41 (–0.29 to 1.10) | |
Three | –1.56 (–2.05 to –1.08) | –0.96 (–2.02 to 0.11) | 20.97 (19.86 to 22.09) | 0.61 (–0.44 to 1.66) | |
Four or more | 0.53 (–0.02 to 1.08) | 1.34 (–0.04 to 2.71) | 21.17 (19.85 to 22.50) | 0.81 (–0.59 to 2.21) |
Before introducing telephone triage, there were small differences in the time taken to see or speak to a GP or appropriate health-care professional between people with one, two, three, or four or more long-term health conditions, and people with no long-term health conditions. People with one, two or three conditions had a slightly worse experience/longer wait (a 1–2-percentage-point reduction) and people with four or more conditions had a slightly shorter wait. We found no evidence that the effect of adopting telephone triage differed significantly between people with and without multimorbidity (adjusted change 20.36 percentage points for those with zero long-term health conditions and 21.17 percentage points for those with four or more long-term health conditions; p = 0.26). We did find a slightly less positive impact for people with hearing problems (an 18.81-percentage-point improvement for those with hearing problems compared with a 20.8-percentage-point improvement for people without hearing problems; p = 0.024). The small inequalities experienced by people with multiple long-term health conditions (slightly longer time taken to speak to a GP among people with two or three conditions) did not differ from before to after the introduction of a telephone triage approach (see Table 6).
Analysis 4: inequalities in access to primary care for people with multiple long-term health conditions between April and November 2020 (Understanding Society)
In our fourth analysis, we explored the relationship between multimorbidity and access to primary care using USoc (Table 7). People with multimorbidity are more likely than people without long-term health conditions to have a problem for which they need to access primary care [one condition: odds ratio (OR) 0.95, 95% confidence interval (CI) 0.82 to 1.09; two conditions: OR 1.65, 95% CI 1.41 to 1.94; three conditions: OR 2.17, 95% CI 1.72 to 2.74; four or more conditions: OR 4.08, 95% CI 3.20 to 5.20; p < 0.0001]. Among people who did have a problem for which they would normally try to contact their GP or primary care, there was no evidence (p = 0.50) of variation in whether or not someone did in fact try to contact their GP for this problem, or whether or not they were able to make an appointment to see their GP (p = 0.11) based on the number of long-term health conditions they had. There was no evidence (p = 0.09) that whether someone was offered a telephone, online or in-person appointment varied based on the number of long-term health conditions that they had, compared with people without long-term health conditions, although the ORs were all < 1.
Number of long-term health conditions | Needed to access service | Tried to contact service | Able to access service | Face to face | ||||
---|---|---|---|---|---|---|---|---|
OR (95% CI) | p-value | OR (95% CI) | p-value | OR (95% CI) | p-value | OR (95% CI) | p-value | |
Zero | Reference | < 0.0001 | Reference | 0.50 | Reference | 0.11 | Reference | 0.09 |
One | 0.95 (0.82 to 1.09) | 1.24 (0.94 to 1.64) | 1.50 (1.02 to 2.20) | 0.99 (0.82 to 1.19) | ||||
Two | 1.65 (1.41 to 1.94) | 1.25 (0.90 to 1.74) | 1.16 (0.74 to 1.83) | 0.81 (0.66 to 0.99) | ||||
Three | 2.17 (1.72 to 2.74) | 1.32 (0.92 to 1.89) | 1.47 (0.90 to 2.39) | 0.76 (0.56 to 1.05) | ||||
Four or more | 4.08 (3.20 to 5.20) | 1.14 (0.72 to 1.79) | 0.91 (0.54 to 1.51) | 0.97 (0.75 to 1.25) |
Analysis 5: exploring whether or not there is any variation in primary care access for certain groups among people with multimorbidity
We explored whether or not certain groups of people living with multiple long-term health conditions waited longer to see or speak to a GP or other health-care professional than others, specifically considering age, sex, ethnicity, deprivation/household, rurality and employment status.
In our analysis using GPPS, we explored whether or not the relationship between time taken to see or speak to a GP among people with multimorbidity by age, sex, ethnicity, deprivation, rurality and employment differed cross-sectionally using data from 2015/16 and 2017 (among general practices with and without a telephone triage approach). We found evidence that there was a differential impact (p < 0.0001 for all except employment, for which p = 0.015). Therefore, we explored the relationship between multimorbidity and the time taken to see or speak to a GP separately, stratified by each included group. These results can be seen in Figures 1–6. In the figures, the presented results show the percentage-point difference for people with one, two, three, or four or more conditions compared with that for someone with zero conditions in each group.
For sex (Figure 1), deprivation (Figure 2), rurality (Figure 3) and employment (Figure 4), although there was evidence that the relationship between multimorbidity and the time taken to see or speak to a GP did vary between groups, the presented results show that the magnitude of any differences between the groups was small. We identified a pattern in which people with one, two or three long-term health conditions had a slightly (1–2-percentage-point) longer time taken, and people with four or more long-term health conditions had a slightly shorter time taken. For ethnicity (Figure 5), a similar relationship was seen among people with white, Asian and black ethnic backgrounds; for people with a mixed or other ethnicity, the relationship was unclear, although the 95% CI was wide.
FIGURE 1.
Inequalities in the time taken to see or speak to a GP among people with multimorbidity, stratified by sex: GPPS. Adjusted and presented on a scale of 0–100, with 100 being the most positive experience.

FIGURE 2.
Inequalities in the time taken to see or speak to a GP among people with multimorbidity, stratified by deprivation: GPPS. Adjusted and presented on a scale of 0–100, with 100 being the most positive experience.

FIGURE 3.
Inequalities in the time taken to see or speak to a GP among people with multimorbidity, stratified by urban/rural location: GPPS. Adjusted and presented on a scale of 0–100, with 100 being the most positive experience.
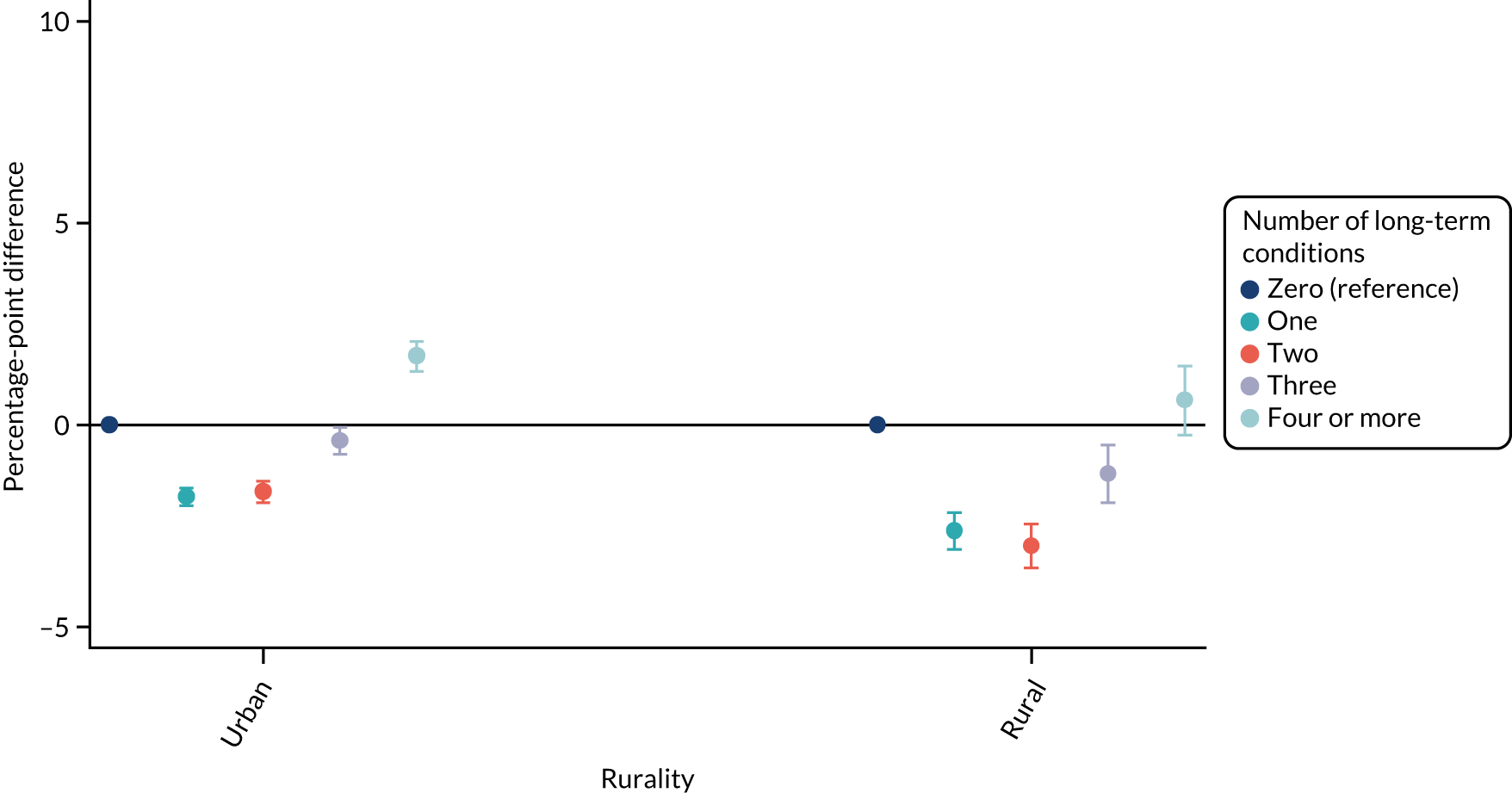
FIGURE 4.
Inequalities in the time taken to see or speak to a GP among people with multimorbidity, stratified by employment status: GPPS. Adjusted and presented on a scale of 0–100, with 100 being the most positive experience.
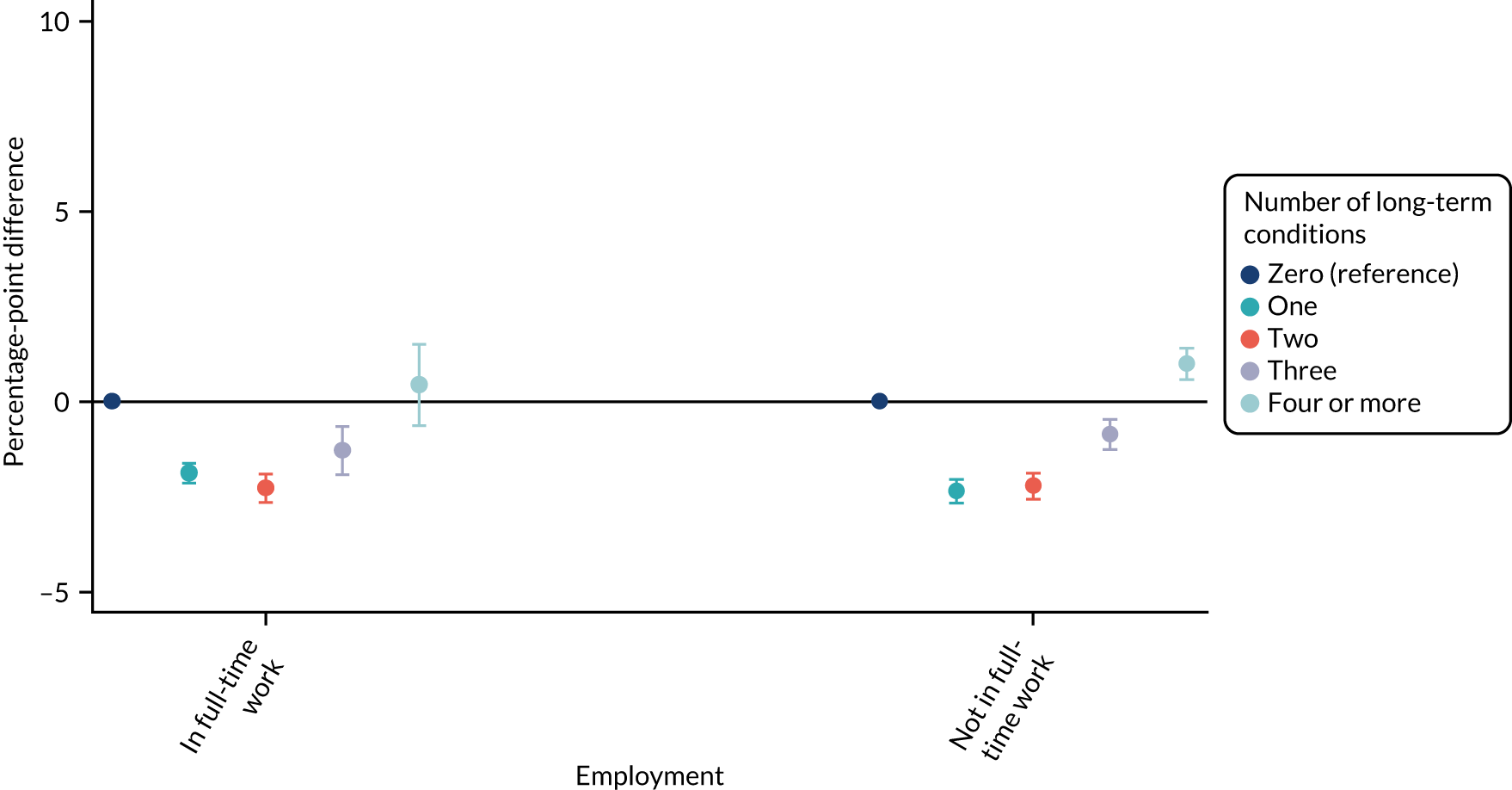
FIGURE 5.
Inequalities in the time taken to see or speak to a GP among people with multimorbidity, stratified by ethnicity: GPPS. Adjusted and presented on a scale of 0–100, with 100 being the most positive experience.
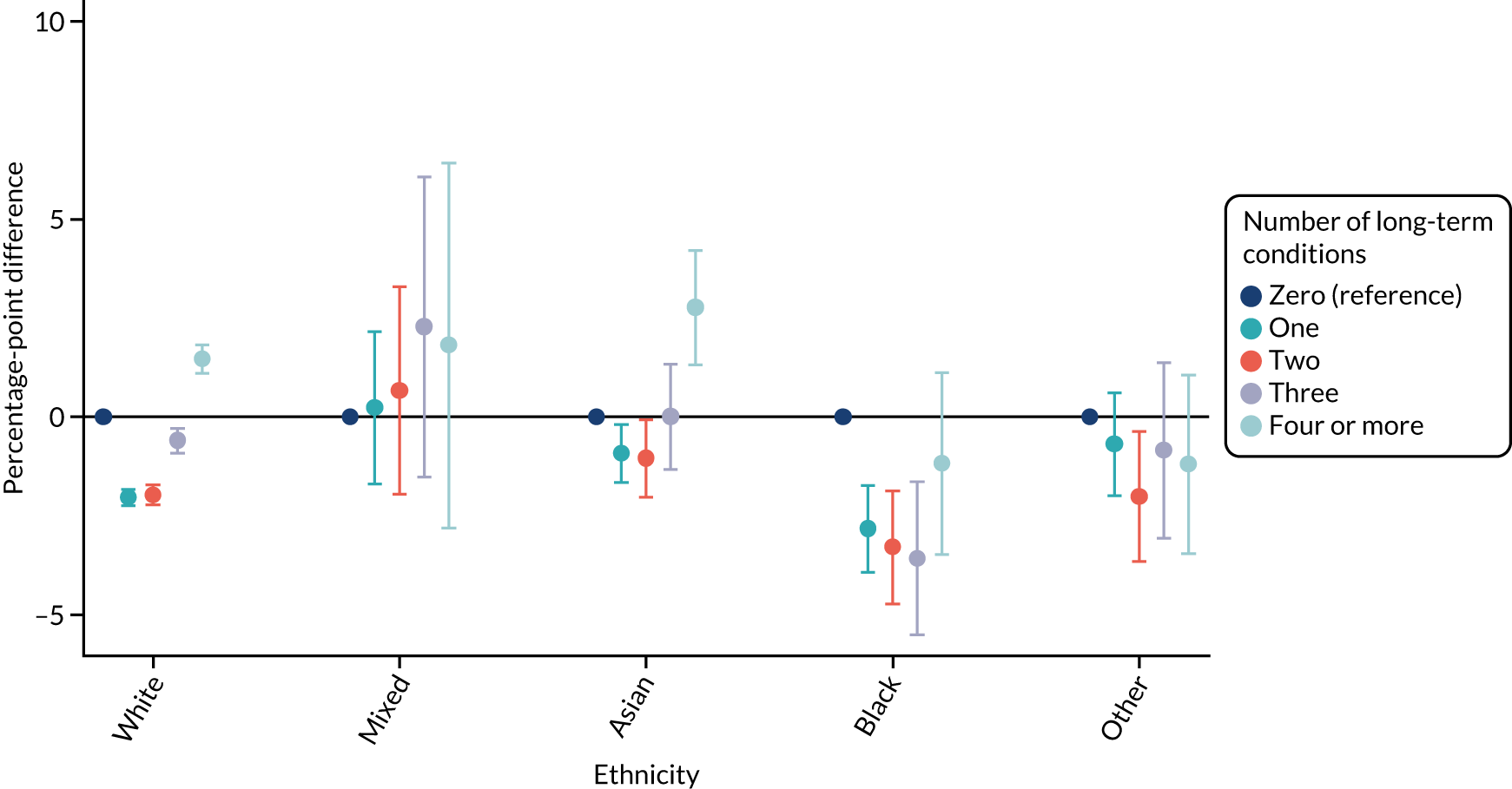
For age (Figure 6), however, this stratified presentation of the relationship between multimorbidity and the time taken to see or speak to a GP showed a different relationship between the age groups. In contrast to the overall relationship, which found that people with zero or four or more long-term health conditions had a slightly more positive experience than people with one, two or three long-term health conditions, these results highlight that in older age groups, the relationship between multimorbidity and the time taken to see or speak to a GP becomes increasingly positive. For older age groups, as the number of long-term health conditions that a person reports increases, the length of time before they see or speak to a GP decreases.
FIGURE 6.
Inequalities in the time taken to see or speak to a GP among people with multimorbidity, stratified by age: GPPS. Adjusted and presented on a scale of 0–100, with 100 being the most positive experience.

The results for caregiving responsibilities and sexual orientation are presented in Report Supplementary Material 8 and 9.
In our analysis using USoc, we explored whether or not the relationship between multimorbidity and whether or not someone needed to access primary care, whether or not they tried to contact a GP for this problem, whether or not they were able to access care, and whether or not the appointment was face to face (analysis 4) varied by age, sex, ethnicity, household income, rurality, employment status and whether or not someone was shielding. There was no evidence (p < 0.05) for any heterogeneity for 22 out of 28 of these hypothesis tests and, after adjusting the significance threshold for multiple testing, there was evidence for age only. For age, when exploring these coefficients in detail, we find that multimorbidity has a greater impact on whether or not someone needs to access a GP for younger people than older people. To explore whether or not the relationship between multimorbidity and primary care access varied based on sociodemographic and personal characteristics, we present the results for the entire sample.
Older people, women, people living in lower income households, people who were unemployed and people who were shielding were more likely than others to have a problem for which they would need to access primary care between April and November 2020. However, among people who did have a problem for which they needed to access a GP, people in employment and people who were shielding were more likely than others to try to access a GP. People living in rural, rather than urban areas, were more likely to be offered a face to face appointment than an online or telephone consultation (Table 8).
Characteristic | Needed to access service | Tried to contact service | Able to access service | Face to face | ||||
---|---|---|---|---|---|---|---|---|
OR (95% CI) | p-value | OR (95% CI) | p-value | OR (95% CI) | p-value | OR (95% CI) | p-value | |
Age (years) | ||||||||
0–39 | Reference | 0.0016 | Reference | 0.07 | Reference | 0.77 | Reference | 0.0003 |
40–49 | 1.03 (0.82 to 1.28) | 0.93 (0.58 to 1.50) | 1.16 (0.72 to 1.87) | 1.04 (0.79 to 1.37) | ||||
50–59 | 1.26 (1.03 to 1.54) | 0.91 (0.60 to 1.40) | 0.94 (0.59 to 1.52) | 1.08 (0.82 to 1.43) | ||||
60–69 | 1.22 (1.00 to 1.48) | 1.28 (0.88 to 1.85) | 1.15 (0.74 to 1.80) | 1.21 (0.93 to 1.58) | ||||
≥ 70 | 1.37 (1.13 to 1.65) | 0.99 (0.67 to 1.45) | 1.20 (0.76 to 1.88) | 1.46 (1.11 to 1.91) | ||||
Sex | ||||||||
Male | Reference | < 0.0001 | Reference | 0.86 | Reference | 0.99 | Reference | 0.056 |
Female | 1.31 (1.17 to 1.46) | 1.02 (0.79 to 1.32) | 1.00 (0.75 to 1.33) | 0.86 (0.74 to 1.00) | ||||
Ethnicity | ||||||||
White | Reference | 0.40 | Reference | 0.95 | Reference | 0.12 | Reference | 0.11 |
Asian | 1.28 (0.8 to 2.05) | 1.12 (0.61 to 2.05) | 0.70 (0.30 to 1.61) | 0.80 (0.50 to 1.27) | ||||
Black | 0.86 (0.58 to 1.28) | 0.74 (0.29 to 1.87) | 0.53 (0.26 to 1.09) | 0.45 (0.24 to 0.85) | ||||
Mixed | 1.11 (0.72 to 1.73) | 1.17 (0.53 to 2.60) | 1.28 (0.35 to 4.66) | 0.99 (0.62 to 1.58) | ||||
Other | 1.9 (0.8 to 4.53) | 0.91 (0.31 to 2.70) | 0.50 (0.25 to 1.01) | 1.62 (0.52 to 5.04) | ||||
Income | ||||||||
Highest | Reference | < 0.0001 | Reference | 0.96 | Reference | 0.54 | Reference | 0.088 |
Second | 1.13 (0.99 to 1.28) | 1.03 (0.80 to 1.33) | 1.34 (0.92 to 1.96) | 1.16 (0.97 to 1.39) | ||||
Third | 1.12 (0.98 to 1.29) | 0.99 (0.77 to 1.27) | 1.00 (0.70 to 1.43) | 0.88 (0.74 to 1.05) | ||||
Fourth | 1.37 (1.19 to 1.59) | 0.94 (0.69 to 1.28) | 1.01 (0.69 to 1.46) | 0.96 (0.79 to 1.16) | ||||
Lowest | 1.39 (1.15 to 1.69) | 0.90 (0.62 to 1.31) | 0.93 (0.60 to 1.43) | 1.04 (0.82 to 1.31) | ||||
Urban/rural | ||||||||
Urban | Reference | 0.25 | Reference | 0.10 | Reference | 0.72 | Reference | 0.0008 |
Rural | 0.93 (0.83 to 1.05) | 0.81 (0.63 to 1.04) | 1.06 (0.76 to 1.50) | 1.29 (1.11 to 1.50) | ||||
Employment | ||||||||
Not employed | Reference | < 0.0001 | Reference | 0.015 | Reference | 0.48 | Reference | 0.24 |
Employed | 0.61 (0.52 to 0.72) | 1.46 (1.07 to 1.99) | 1.14 (0.79 to 1.64) | 0.89 (0.73 to 1.08) | ||||
Shielding status | ||||||||
Shielding | Reference | < 0.0001 | Reference | < 0.0001 | Reference | 0.86 | Reference | 0.30 |
Not shielding | 0.44 (0.36 to 0.53) | 0.45 (0.33 to 0.61) | 1.04 (0.65 to 1.66) | 0.91 (0.76 to 1.09) |
Supplementary analyses
In addition to exploring whether or not the impact of telephone triage on primary care access was different for people with and without multimorbidity, in the main analysis using GPPS, we also considered whether or not there were any differential effects based on age, sex, ethnicity, deprivation, employment status, rurality, and whether or not someone reported a long-term hearing problem or deafness (see Table 10).
For ethnicity (p = 0.27) and deprivation (p = 0.29), there was no evidence of a differential impact of starting telephone triage. However, for sex (improvement in women 1.03 percentage points more than in men; p = 0.018), rurality (improvement in urban practices 0.65 percentage points more than in rural practices; p = 0.006) and employment (improvement in people who were unemployed 0.92 percentage points lower than in people who were employed; p = 0.037), there was evidence that the impact of telephone triage differed between groups.
For age (p = 0.07), there was a clear age gradient. However, as for multimorbidity, these differences were very small compared with the overall 20-percentage-point difference seen at the start of a telephone triage approach. The impact for people with hearing problems was slightly less positive (an 18.8-percentage-point improvement for people with hearing problems compared with a 20.8-percentage-point improvement for people without hearing problems; p = 0.024). Full results are presented in Table 9. Results for people with caregiving responsibilities (p = 0.16) and an exploration of variation by sexual orientation (p = 0.18) are presented in Report Supplementary Material 10.
Characteristic | Percentage change (95% CI) | p-value | |||
---|---|---|---|---|---|
Before | After | Change | Differential change | ||
Age (years) | |||||
18–24 | 2.54 (0.11 to 4.98) | 1.42 (0.09 to 2.74) | 23.12 (20.3 to 25.94) | –1.13 (–2.35 to 0.09) | 0.07 |
25–34 | 5.07 (3.14 to 7.00) | 4.16 (3.14 to 5.19) | 22.90 (20.31 to 25.49) | –0.90 (–1.88 to 0.07) | |
35–44 | 5.51 (4.05 to 6.98) | 4.84 (4.04 to 5.63) | 22.67 (20.31 to 25.04) | –0.68 (–1.41 to 0.06) | |
45–54 | 0.81 (–0.20 to 1.83) | 0.36 (–0.23 to 0.96) | 22.45 (20.30 to 24.59) | –0.45 (–0.94 to 0.04) | |
55–64 | –0.59 (–1.19 to 0.00) | –0.82 (–1.25 to –0.39) | 22.22 (20.29 to 24.15) | –0.23 (–0.47 to 0.02) | |
65–74 | Reference | Reference | 22.00 (20.27 to 23.72) | Reference | |
75–84 | 2.40 (1.78 to 3.03) | 2.63 (2.16 to 3.10) | 21.77 (20.25 to 23.29) | 0.23 (–0.02 to 0.47) | |
≥ 85 | 6.07 (4.94 to 7.20) | 6.52 (5.74 to 7.30) | 21.55 (20.2 to 22.89) | 0.45 (–0.04 to 0.94) | |
Sex | |||||
Men | Reference | Reference | 20.06 (18.98 to 21.14) | Reference | 0.018 |
Women | 2.70 (2.44 to 2.96) | 3.73 (2.91 to 4.54) | 21.09 (20.07 to 22.1) | 1.03 (0.18 to 1.88) | |
Ethnicity | |||||
White | Reference | Reference | 20.85 (19.88 to 21.81) | Reference | 0.27 |
Mixed | 0.46 (–1.06 to 1.99) | –2.16 (–7.05 to 2.72) | 18.22 (13.06 to 23.38) | –2.63 (–7.74 to 2.49) | |
Asian | 2.65 (2.00 to 3.30) | 0.48 (–1.63 to 2.59) | 18.68 (16.4 to 20.95) | –2.17 (–4.36 to 0.02) | |
Black | 2.80 (1.94 to 3.65) | 1.49 (–1.09 to 4.08) | 19.54 (16.76 to 22.33) | –1.30 (–4.01 to 1.41) | |
Other | 3.06 (2.16 to 3.95) | 2.49 (–0.7 to 5.68) | 20.29 (16.92 to 23.66) | –0.56 (–3.87 to 2.75) | |
Deprivation | |||||
1, least deprived | Reference | Reference | 20.83 (19.62 to 22.04) | Reference | 0.29 |
2 | 0.54 (0.01 to 1.08) | 0.08 (–0.28 to 0.44) | 20.75 (19.73 to 21.78) | –0.08 (–0.44 to 0.28) | |
3 | 0.61 (–0.18 to 1.39) | 0.70 (0.12 to 1.28) | 20.68 (19.72 to 21.63) | –0.16 (–0.88 to 0.57) | |
4 | 1.36 (0.28 to 2.44) | 0.84 (0.21 to 1.47) | 20.60 (19.59 to 21.61) | –0.23 (–1.32 to 0.85) | |
5, most deprived | 1.90 (0.5 to 3.30) | 1.67 (1.00 to 2.34) | 20.52 (19.34 to 21.7) | –0.31 (–1.76 to 1.13) | |
Rurality | |||||
Urban | Reference | Reference | 20.7 (19.72 to 21.69) | Reference | 0.006 |
Rural | 2.12 (0.61 to 3.63) | 1.47 (–1.82 to 4.76) | 20.05 (17.12 to 22.98) | –0.65 (–3.68 to 2.38) | |
Employment | |||||
Employed | Reference | Reference | 21.21 (20.12 to 22.3) | Reference | 0.037 |
Not employed | 1.21 (0.87 to 1.54) | 0.28 (–0.57 to 1.13) | 20.28 (19.24 to 21.32) | –0.92 (–1.79 to –0.06) | |
Hearing problems | |||||
No | Reference | Reference | 20.8 (19.84 to 21.76) | Reference | 0.024 |
Yes | 0.96 (0.41 to 1.51) | –1.03 (–2.68 to 0.62) | 18.81 (16.93 to 20.69) | –1.99 (–3.72 to –0.26) |
A further supplementary cross-sectional analysis explored whether the relationship between multimorbidity and two other measures of primary care access in GPPS (overall experience of access and experience of telephone access) was similar or different from the measures considered in the main analysis. This used cross-sectional data from 2015/16 and 2017. We found that the relationships between multimorbidity and the two other measures of primary care access were slightly different than the relationship between multimorbidity and the time taken to see or speak to a GP or other appropriate health-care professional. Increasing numbers of long-term health conditions were associated with worse overall experiences of access and experiences of telephone access (percentage-point change for one long-term health condition –0.53, for two long-term health conditions –1.36, for three long-term health conditions –2.26 and for four long-term health conditions –2.80, compared with people without long-term health conditions) (Table 10).
Number of long-term health conditions | Measure | |||||
---|---|---|---|---|---|---|
Time until being seen or spoken to | Overall experience of access | Telephone access | ||||
Coefficient (95% CI) | p-value | Coefficient (95% CI) | p-value | Coefficient (95% CI) | p-value | |
Zero | Reference | < 0.0001 | Reference | < 0.0001 | Reference | < 0.0001 |
One | –1.94 (–2.14 to –1.74) | –0.74 (–0.86 to –0.62) | –0.53 (–0.66 to –0.39) | |||
Two | –1.93 (–2.17 to –1.68) | –1.86 (–2.00 to –1.71) | –1.36 (–1.52 to –1.19) | |||
Three | –0.60 (–0.90 to –0.29) | –2.97 (–3.15 to –2.78) | –2.26 (–2.47 to –2.06) | |||
Four or more | 1.44 (1.10 to 1.79) | –4.18 (–4.39 to –3.97) | –2.80 (–3.03 to –2.57) |
In USoc, the main sensitivity analyses explored two further measures of access to health-care services in the community during April to November 2020, namely access to NHS 111 and to prescription medication (Table 11).
Measure of access | Needed to access service | Tried to contact service | Able to access service | |||
---|---|---|---|---|---|---|
OR (95% CI) | p-value | OR (95% CI) | p-value | OR (95% CI) | p-value | |
NHS 111 | ||||||
Number of long-term health conditions | ||||||
Zero | Reference | < 0.0001 | Reference | 0.22 | Reference | 0.19 |
One | 0.85 (0.65 to 1.12) | 1.60 (0.93 to 2.75) | 1.82 (0.97 to 3.40) | |||
Two | 1.48 (1.10 to 1.99) | 1.43 (0.80 to 2.55) | 2.14 (1.07 to 4.30) | |||
Three | 1.96 (1.40 to 2.73) | 0.93 (0.54 to 1.60) | 1.31 (0.66 to 2.60) | |||
Four or more | 3.19 (2.21 to 4.62) | 0.95 (0.49 to 1.84) | 2.08 (0.85 to 5.11) | |||
Prescription medication | ||||||
Number of long-term health conditions | ||||||
Zero | Reference | < 0.0001 | Reference | < 0.0001 | ||
One | 2.43 (2.10 to 2.83) | 1.91 (0.98 to 3.72) | ||||
Two | 6.72 (5.49 to 8.21) | 3.26 (1.92 to 5.54) | ||||
Three | 14.81 (10.12 to 21.68) | 2.33 (1.22 to 4.44) | ||||
Four or more | 15.59 (10.00 to 24.32) | 4.35 (2.13 to 8.90) |
The relationship between multimorbidity and access was similar for these two services compared with the relationship between multimorbidity and primary care access. That is, people living with multiple long-term health conditions were more likely to have a problem appropriate for NHS 111 (one long-term health condition: OR 0.85, 95% CI 0.65 to 1.12; two long-term health conditions: OR 1.48, 95% CI 1.10 to 1.99; three long-term health conditions: OR 1.96, 95% CI 1.40 to 2.73, four or more long-term health conditions: OR 3.19, 95% CI 2.21 to 4.62; p < 0.0001), but with no evidence of variation in whether or not they tried to contact the service compared with people with one long-term health condition (p = 0.22). There was no evidence of variation in ability to access NHS 111 (p = 0.19).
Multimorbidity was associated with an increase in the likelihood of requiring prescription medication (p < 0.0001). People with multiple morbidities were also more likely to be able to access prescription medication when they required it (p < 0.0001).
Testing these findings with the patient and public involvement panel
We held a workshop with the BRACE PPI panel, some members of which live with multiple long-term health conditions, and discussed a preliminary version of these evaluation results. We presented the findings and explained that we thought that our findings suggested that introducing telephone triage was not likely to have increased the inequalities in access to primary care for people living with multiple long-term health conditions, and that the impact of multimorbidity was small compared with the overall impact of the changes associated with the start of a telephone triage approach.
The panel were more cautious in their interpretation, describing challenges in getting through to the GP by telephone and difficulties with telephone triage for people living with multiple long-term health conditions, who have to try to explain complex details on the telephone. Different panel members had had different experiences, both positive and negative. We noted these points and have been cautious in our interpretation of the findings as a result. The panel discussion reflected the fact that the switch to telephone triage at the start of the pandemic clearly had a considerable impact on the panel members, as it had for the whole population.
Chapter 4 Discussion
Summary
-
Our results highlight that, although people with multimorbidity have a greater need for primary care, introducing telephone triage does not have a large differential impact on groups of patients with different numbers of long-term health conditions attending the same general practice.
-
We found no evidence of a differential impact of rolling out telephone triage for patients with different numbers of long-term health conditions.
-
Previous work from an earlier evaluation of telephone triage19 found a large variation in the impact of introducing telephone triage between practices; this variation is much greater than the variation we found between patients within a single practice.
-
Additional funding to support practices struggling with high levels of demand and a lower per capita primary care workforce, for example in areas of high socioeconomic deprivation, is one possible approach to addressing these disparities. National models for the roll-out of general practice-level innovations would be a second approach that may reduce heterogeneity in implementation between practices. These approaches would require further research and evaluation.
-
People living with multiple long-term health conditions experienced similar disparities in health-care need and access to people without multiple long-term health conditions based on sex, ethnicity, deprivation, rurality and employment.
-
We found that people with multimorbidity aged ≥ 85 years were seen more quickly by their GP than those aged ≥ 85 years without multimorbidity, which may represent appropriate triaging by GPs or better familiarity with GP systems among the former group of patients.
-
Rapid quantitative evaluations, such as that reported here, are possible when datasets (and analysis frameworks) are already available, and they are desirable when there are still important unanswered policy questions.
-
At the time of writing (autumn 2021), there is ongoing and contentious policy debate regarding whether or not remote consultation and telephone triage in primary care should continue and, if so, what the most appropriate clinical form should be for this new way of working. Our evaluation brings quantitative evidence of one aspect of the inequalities impact of practices adopting a telephone triage approach to this debate, with particular consideration of patients with multimorbidity.
Key findings
We carried out a rapid quantitative evaluation exploring the impact of introducing telephone triage on the speed of access to a GP, comparing the experience of people living with and without multiple long-term health conditions.
We found that, although there are differences in the time taken before someone can see or speak to an appropriate primary care professional among people with multimorbidity and people without, both before and after introducing telephone triage, these differences are small compared with the overall improvement for all patients that occurs when a general practice switches to a telephone triage approach. In addition, we found no evidence of a difference in the impact of a practice switching to a telephone triage approach for people living with and without multiple long-term health conditions. In supplementary analyses, we found similar results, with small or non-differential impacts from switching to a telephone triage approach based on age, sex, ethnicity, deprivation, rurality, employment, and whether or not someone has hearing problems.
We also explored the impact of the COVID-19 pandemic on primary care access. At the start of the pandemic, we found that people were less likely to try to see a GP if they had a problem for which they would normally try to see a GP than they were pre pandemic. However, by September 2020, > 90% of people with a problem did try to get in touch with a GP. Of people who did try to access primary care, > 90% were able to make an appointment. This was true throughout the period from April to November 2020. In April 2020, only 20% of all GP appointments were face to face; this had increased to about 40% by November 2020.
During the COVID-19 pandemic, people living with multimorbidity were more likely to have a problem that meant that they needed to see a GP than people with no long-term health conditions or a single condition. However, we found no evidence that there was any difference between people with or without multimorbidity regarding whether or not they tried to access a GP if they did have a problem; whether or not they were able to make an appointment; or whether the appointment they obtained was face to face, by telephone or online. These patterns were consistent with supplementary analysis considering access to NHS 111, in which people with multiple long-term health conditions were again more likely to need to access to services than those without, but with little evidence of variation in access to care among people who needed care. The ability to access prescription medication was an exception: people with multiple long-term health conditions were more able to access these services, given a self-reported need for them, than people with just one or no long-term health conditions.
We found little evidence across most measures of access to primary care, both before and during the pandemic, that particular groups of people living with multimorbidity had differentially better or worse experiences of primary care access when considering age, sex, ethnicity, deprivation, rurality, employment or shielding status. This does not mean that disparities do not exist. For example, during 2020, people living in low-income households reported a greater need for health care; however, we found that these disparities impact people living with multiple long-term health conditions in a similar way to people without.
However, we did find variation in the relationship between multimorbidity and time taken to see or speak to a GP or other appropriate primary care professional, and age. In particular, for people aged ≥ 85 years, the larger their number of long-term health conditions was, the quicker they were able to see or speak to a GP/other professional.
Findings in context
In this section, we discuss these findings in the context of previous pre- and post-COVID-19 research on inequalities in primary care access from telephone triage and other remote consultation approaches, with a focus on inequalities experienced by people living with multiple long-term health conditions.
The relationship between health-care need and access in telephone triage or other remote primary care approaches for people living with multiple long-term health conditions
The evaluation reported here builds on and extends previous work30 that considered inequalities in telephone triage or other forms of remote access to primary care for people with multiple long-term health conditions.
Before the COVID-19 pandemic, most studies of telephone triage or other forms of remote consultations focused on general populations, although some have touched on the issues for those living with multimorbidity. A mixed-methods study of alternatives to face-to-face consultations reported that patients with multiple long-term health conditions showed higher rates of accessing alternatives to face-to-face consultations than other patients, although the authors note that this group also had a higher use of any type of consultation. 62 Our analysis builds on and clarifies this existing work. We found that, during the COVID-19 pandemic, people with multimorbidity did need to access a GP more often than people living with no long-term health conditions or a single condition; however, after accounting for the difference in need, there was then no difference in whether someone was offered an in-person or face-to-face appointment.
This idea that the relationship between multimorbidity and need for health care, and the experience of remote access, form two different dimensions is consistent with the findings of an evaluation of another remote model of primary care access in which patients can opt in to a mobile phone application-based approach (Babylon GP at Hand; Babylon, London, UK). 63 That evaluation considered both the characteristics of people who chose to use the remote service and the experiences of people with long-term health conditions. The GP at Hand service was not used by a large number of people with complex health needs, although the majority of people with long-term health conditions who did use the service reported that the service was good.
A mixed picture of inequalities experienced according to age, sex, ethnicity, deprivation, employment and rurality
Considering inequalities more widely than those experienced by people living with multiple long-term health conditions only, a recent systematic review included 13 studies that considered the impact of remote consulting on inequalities: four studies from the USA, two from the UK, two from Denmark, and one each from Italy, Canada, Sweden, Spain and the Netherlands. 30 Of the 13 studies, six compared traditional face-to-face consultations with the use of telephone, e-mail or videocalls. A further four studies focused on specific interventions, including introducing telephone triage. The remaining three studies focused on remote consultations for specific groups: migrants and patients with opioid dependence. Age and sex were the most common measures of inequality in the identified papers, followed by socioeconomic status, ethnicity and immigration status. The authors of the studies found that remote consultations are either more likely to be used by younger, working age people (six studies) or there was no difference across age groups (one study). The authors suggested that this may reflect differences between rural and urban areas, with urban areas typically having younger populations.
In our analysis of data from 2020, during the COVID-19 pandemic, we found no evidence of variation by age, deprivation, ethnicity, employment status or multimorbidity in whether someone was offered a face-to-face or in-person appointment. However, we did find that people in rural areas were more likely to be offered a face-to-face appointment than those in urban areas, and men were more likely to be offered a face-to-face appointment than women. These findings do not substantially clarify the mixed picture described by the systematic review, although they suggest that after accounting for need and ability to access services, the inequalities in whether someone is offered a face-to-face or remote consultation are small.
Robust evaluation of the effect of introducing technologies such as telephone consultations on the nature and extent of health inequalities is challenging, and the pathways through which service innovations reduce or increase inequalities depend on context,64 which may explain some of the variability in evidence. We cautiously suggest that the wide heterogeneity in the models of remote consultation and telephone triage in primary care may further explain the mixed picture in terms of the evidence that exists around possible inequalities in health and health-care outcomes from these models of access. The fact that our supplementary analysis exploring the relationship between multimorbidity and two additional measures of patient experience of primary care access found that inequalities experienced varied between outcome measures further highlights this heterogeneous picture.
The individual- and general practice-level impact of introducing telephone triage on inequalities in the time taken to see or speak to a general practitioner or other appropriate health-care professional
Although our research exploring the inequalities impact of introducing telephone triage is based on data from before the COVID-19 pandemic, rapid qualitative research conducted during the pandemic is consistent with this large main impact of adopting a telephone triage approach and found that patients appreciated the convenience of speaking to a health professional by telephone, and quicker and more efficient access to a health professional. 65 Findings are also consistent with the 2021 GPPS results, which found a reduction in the time before someone would see or speak to a GP. 25 The findings are also consistent with reflections from the BRACE PPI panel, the members of which had all observed large changes (albeit both positive and negative) in primary care access during the COVID-19 pandemic.
We considered the inequalities impact of a general practice starting a telephone triage approach and found that, compared with the large overall improvement in speed of access to a GP, the inequalities experienced by people with multiple long-term health conditions and other sociodemographic characteristics on time taken to see or speak to an appropriate primary care professional are small, and the large improvement occurs across all groups. The impact of introducing telephone triage on existing inequalities is also small.
When a general practice switches to a telephone triage approach, this is a change that has an impact on all patients using that practice, and so it is perhaps to be expected that this is reflected in our findings: telephone triage has a large impact overall, but because it has been implemented in the same way for everyone, the impact on any inequalities between patients within a practice is small. Even for patients with hearing problems, for whom a telephone triage approach might be expected to be more challenging, we still found that, overall, all patients had a large improvement in the time taken for them to see or speak to a GP.
However, the evaluation of telephone triage by Newbould et al. 19,20 did find heterogeneity in the impact of starting a telephone triage approach between practices. The middle 95% of practices showed increases of between 1.4 percentage points and 38.6 percentage points for time taken to see or speak to a GP. We therefore cautiously conclude that heterogeneity in the impact of introducing telephone triage occurs between practices rather than between individuals within practices; between-individual impact is what we considered in this analysis.
The SELFIE (Sustainable intEgrated chronic care modeLs for multimorbidity: delivery, FInancing, and performancE) framework66 is intended to support the development, description, implementation and evaluation of integrated care for multimorbidity and the thematic approach drawn on by the BRACE overarching study of service innovations for people with multiple long-term health conditions. 67 It is a theoretical framework that can be used to understand our findings. The patient and their environment are the core of the SELFIE framework. Concepts of care for people with multimorbidity are described at the micro, meso and macro levels, and are divided based on six World Health Organization (WHO) components: service delivery, leadership and governance, workforce, financing, technologies and medical products, and information and research.
In our evaluation patient (micro-level), general practice (meso-level) and COVID-19 (macro-level) factors are all important, and health services delivery and technology are the two key components relevant to this work. The SELFIE dimension ‘service delivery’ includes ‘treatment interaction’ at the micro level and ‘service availability and access’ at the meso level; telephone triage in primary care is likely to have had an impact on both. Telephone triage in primary care is an intervention delivered at the meso (practice) or macro (national, during COVID-19) level, with a whole practice switching to the telephone triage approach. Therefore, it is perhaps unsurprising that, although the original evaluation of telephone triage19,20 highlighted variation in the impact between practices, our work has found little variation between individuals with different characteristics attending the same practices.
Impact for groups of people living with multiple long-term health conditions
One of our key findings has been that people living with multiple long-term health conditions experienced similar disparities in health-care need and access to people without multiple long-term health conditions based on sex, ethnicity, deprivation, rurality and employment. This finding is consistent with earlier research. For example, our finding that the self-reported need to access care was greater in lower-income households is consistent with previous work. 6
We found that people with multimorbidity aged ≥ 85 were seen more quickly by their GP than people of that age without multimorbidity. Candidacy theory68 describes how people’s eligibility for health care is determined between themselves and health services, and we cautiously suggest that the explanation for the relationship seen with age reflects this combination of GP and patient factors. 68 From the GP’s perspective, an elderly patient with multiple long-term health conditions may be a higher priority and so triaged more urgently. From the patient’s perspective, a patient with multiple conditions may be a more frequent user of primary care and so more familiar with systems for making appointments.
Our finding that people with multimorbidity were more likely to be able to access prescription medications, given self-reported need for medications, during the pandemic is also perhaps consistent with this. Better processes for accessing repeat prescriptions are set up for patients with multiple long-term health conditions managed in the community, and there is no relationship between needs being fully met in patients’ last GP appointment and the number of long-term health conditions. 69
Survey data compared with electronic health-care data for understanding primary care access during the COVID-19 pandemic
Understanding Society has been an important data resource for understanding rapid changes occurring for individuals and households in the UK during the COVID-19 pandemic. 70,71 Survey measures of health-care utilisation are important, particularly for understanding experiences of minority groups for whom demographic data may not be well recorded in electronic health-care records. 72–75 Electronic health-care records provide more nuanced information on health service contacts, however, and previous work comparing health service and survey measures of health service utilisation found reasonable concordance. 76
Our analysis of USoc found that only 20% of appointments in primary care during April 2020 were face to face, rising to about 40% by November 2020. We note that these numbers are not consistent with the estimates presented from analyses of data from NHS Digital, which found that about 50% of patient contacts in primary care were face to face throughout the same period,24 nor with estimates from local primary care databases, which found about 9% of GP and 50% of nurse consultations were face to face during the same period. 77
Regarding differences from local GP research databases, an early evaluation of primary care utilisation during the COVID-19 pandemic suggests that the reduction in GP consultations seen during this period varied between practices. 23 This is consistent with our finding that there would be greater heterogeneity between practices in the impact of switching to a telephone triage approach (described in the earlier evaluation of Newbould et al. 19), rather than between patients within the same practice (described in this analysis). However, this does not explain the differences between estimates of the proportion of consultations occurring face to face in our analysis and in data provided by NHS Digital. Heterogeneity in coding types of consultations in electronic health-care records may explain part of this variation, with some telephone consultations being inaccurately recorded as face to face. Variation in the kind of appointments that general practices offer may also be part of the picture. Neither a telephone call followed up by a face-to-face appointment, nor a triage appointment that turns into a remote consultation would necessarily be straightforward to code in clinical records.
Regarding the total number of primary care appointments dropping at the start of the pandemic and then recovering,24,78 our analysis adds to this, suggesting that this drop was at least in part because a substantial proportion of patients (about 20%) with problems that they would normally see or speak to a GP about did not contact a GP at that time.
Implications for policy and practice
At the time of writing (autumn 2021), there is ongoing and contentious policy debate around whether or not remote consultation and telephone triage in primary care should continue and, if so, what the most appropriate clinical form should be for this new way of working. Primary care, the pandemic and the current policy and political context are all evolving. The final picture is still unclear, alongside the ongoing evolution of health-care associated with COVID-19. 27,28,79–81 Government policy in the UK in recent years has encouraged general practices to move to digital approaches, including the requirement (in England) that all patients have the right to online consultations by April 2021. 17 The nature of a primary care consultation continues to change rapidly and telephone triage is part of this evolving picture; future rapid evaluation (both quantitative and qualitative) of these ongoing changes will be important.
People with multimorbidity are more likely to need to access primary care, and our analysis suggests that this is being equitably met for both people with and without multiple long-term health conditions. The fact that more heterogeneity in the impact of introducing telephone triage occurs between practices than for groups of patients within practices remains important and reflects previous work exploring inequalities in access to primary care. This previous work found that, although there were no changes within practices in inequalities in access to primary care for patients living in more deprived areas compared with less deprived areas, overall national inequalities are widening, as access to primary care gets worse more quickly in practices in more deprived areas. 15 Longer-term evaluation of the impact of telephone triage on inequalities in access to primary care could usefully focus on understanding the differential impact between practices of new models of care. Evaluation of the impact of digital first primary care for people with multimorbidity is ongoing. 82
Strengths and limitations of the evaluation
One of the strengths of survey data is the rich detail regarding participant characteristics and experiences that are not routinely available in electronic health-care data. Further strengths are the large sample size and the large overall impact of introducing telephone triage on the outcome studied, which make this rigorous evaluation of the impact on inequalities possible.
Survey non-response for both GPPS83 and USoc61,84 has been evaluated previously, and non-response weights were used in our analysis to mitigate the possible impact for both data sets. For USoc in particular, response rates are known to be higher among people living with disabilities,61,84,85 although overall response rates are lower for younger people than for older people, and for people reporting very good or very poor health than for those reporting another health status. People living in more deprived areas and from ethnic minority groups are also less likely to respond to surveys than those in less deprived areas and non-ethnic minority groups. Variation in survey response rates between organisations is a further concern. 86 In part, concerns about these imbalances in responses are mitigated in this work by the use of adjusted analysis, including the use of mixed models. However, we acknowledge that it is important that the voices of people who do not respond to surveys are heard in research and that the use of data from survey responders only is a limitation here. In addition, for the USoc analyses, we included both the telephone and online responses as a further approach to minimising bias and improving representativeness. 50
We also acknowledge that there will be additional groups of people who experience challenges with primary care access that we have not been able to include in this work.
A limitation of the GPPS analysis in this work is that we considered people who have accessed primary care in the previous 6 or 12 months only, and that the experiences of people who did not access care are not captured. This is mitigated in part by our analysis of USoc, which asked about primary care access among people who did and did not access their GP during the study period.
A further limitation to acknowledge is that for the evaluation of the inequalities impact of the introduction of telephone triage between 2011–2017, we have only considered one measure of primary care access, namely the time taken to see or speak to a GP or other primary care professional. This is by design because this is the outcome for which the main effect of the intervention was large enough for an inequalities impact analysis to be adequately powered. The exploration of other measures of primary care access using USoc, plus supplementary analyses, allowed a more rounded picture to be assessed.
A final limitation is that we were not able to fully explore the quality of remote consultations. A survey of patients that The Patients Association (London, UK) carried out at the start of the COVID-19 pandemic found that many respondents still had a preference for face-to-face consultations. 87 This is an area in which further work is needed.
Lessons for the conduct of rapid evaluations
This evaluation built on previous research,19,20 and so one piece of learning for future rapid evaluations is that rapid quantitative evaluations are possible when data sets (and analysis frameworks) are already available and there are still important unanswered policy questions. Existing data sets available from the UK Data Archive are a second valuable resource to consider. These are designed to be used for policy research. Access for researchers from UK higher education and research organisations is straightforward and often the data resources (including USoc) are responsive to policy and data needs, such as the COVID-19 waves included in longitudinal studies.
Understanding the quantitative inequalities impacts of innovations is a second area of this work from which other rapid evaluations could learn. This evaluation was able to consider an innovation with a large overall impact, the data available had a large sample size, and multimorbidity and other sociodemographic characteristics were measured. Therefore, it was possible to explore quantitative inequalities in detail. Understanding the inequalities impact of innovations is important and future evaluations should always consider whether or not this would be possible.
A strength of this work was the role of PPI in the design and interpretation of this work; having an existing PPI panel within BRACE made this feasible in the context of rapid evaluation.
Recommendations for future research
Throughout this report we have made several recommendations for future research:
-
Future rapid evaluation (both quantitative and qualitative) of the ongoing changes in primary care within the context of the COVID-19 pandemic remains important. Understanding the health impacts of the lower rates of consultation in the early months of the COVID-19 pandemic should be a focus for future research.
-
More generally, understanding the inequalities impact of the implementation of service innovations (both related to COVID-19 and otherwise) is important, and future evaluations should always consider whether or not quantitative inequalities impact analyses would be possible.
-
Future research projects should aim to maximise the value of existing social and health data resources such as USoc.
-
Longer-term evaluation of the impact of telephone triage or other service innovations could usefully focus on understanding and evaluating approaches to addressing the differential impact between practices.
Conclusions
In this evaluation, we explored the impact of telephone triage on inequalities in primary care access for people living with multiple long-term health conditions compared with those living without, with analysis of two nationally representative surveys. We found that, although people with multimorbidity have a greater need for health services, there was little evidence during 2020 of variation in whether or not someone with multiple long-term health conditions was able to access primary care or the type of appointment that they were offered. Introducing telephone triage to a general practice (whether as a tool for managing demand pre COVID-19 or as a health protection measure introduced since March 2020) has a large impact for all patients at the practice on access overall. Between-practice inequalities in the impact of telephone triage and remote consultation are more important than patient characteristics in driving heterogeneity in outcomes from this intervention. Policy and practice could usefully focus on reducing disparities between practices, rather than between patients within a practice.
Within a general practice, our evaluation found that patients with different levels of health-care needs and numbers of long-term health conditions have equitable access, with no evidence of a differential impact of the roll-out of telephone triage. Variation is greater between practices. Additional funding to support practices struggling with high levels of demand and a lower per capita primary care workforce, for example in areas of high socioeconomic deprivation, is one possible approach to addressing these disparities. National models for the roll-out of general practice-level innovations (e.g. telephone triage or online consultations) could be a second approach that may reduce heterogeneity in implementation between practices. These approaches would require further research and evaluation.
Acknowledgements
We are grateful to the data owners NHS England (Leeds, UK) and the University of Essex (Colchester, UK) via the UK Data Archive for facilitating access to data for this evaluation. We are also very grateful to Dr Jenny Newbould, Dr Gary Abel and Professor Martin Roland for their earlier work on the evaluation of telephone triage in primary care, on which this report builds.
We would also like to thank members of the BRACE Centre Executive, Health and Care Panel, PPI Group, Steering Group and Core Team for their input and constructive comments throughout the study. Specifically, we would like to thank:
-
Jon Sussex (RAND Europe, Cambridge, UK) for providing project advice, his ongoing support and sharing learning from his own evaluations and research throughout
-
Dr Jenny Newbould for co-ordinating all of the PPI work
-
the members of the PPI panel who contributed to workshops and reviews of the project lay summaries
-
Dr Charlotte Davies (RAND Europe) and Dr Efthalia Massou (University of Cambridge, Cambridge, UK) for support with data cleaning
-
Dr Jon Ford for ongoing advice and sharing learning throughout the project
-
Professor Russell Mannion (University of Birmingham, Birmingham, UK) and Dr Kate Morley (RAND Europe), who reviewed our study protocol from a policy and quantitative perspective
-
Dr Kate Morley (RAND Europe), Professor Tom Marshall (University of Birmingham), Professor Martin Roland (University of Cambridge), and Dr Jo Ellins and Professor Judith Smith (University of Birmingham) for providing critical reviews of the draft report
-
participants at the Health Economists’ Study Group Summer 2021 Meeting for critical feedback on an earlier draft
-
Cathy Dakin and Ann Evans (BRACE administrators, University of Birmingham) who provided excellent support throughout.
Contributions of authors
Catherine L Saunders (https://orcid.org/0000-0002-3127-3218) (Senior Research Associate) led the study. She contributed to the conception and design of the study, overall data analysis and interpretation, and is corresponding author of the final report. She led the writing. Catherine L Saunders takes responsibility for the overall editing of the final report and is the guarantor.
Evangelos Gkousis (https://orcid.org/0000-0002-8560-8650) (Analyst) contributed to data analysis, project management, writing and interpretation, and is co-author of the final report.
Catherine L Saunders and Evangelos Gkousis contributed to integrating the findings of the study, made critical revisions to the report for important intellectual content and approved the final manuscript. All authors agree to be accountable for all aspects of the work in ensuring that questions related to the accuracy or integrity of any part of the article are appropriately investigated and resolved.
Publications
Saunders K, Gkousis E. The Impact of the Introduction of Telephone Triage in Primary Care (Pre- and During COVID-19) on Inequalities Experienced by People with Multiple Morbidities. Oral presentation at the Health Studies User Conference, [online] 8 July 2021. URL: https://dam.ukdataservice.ac.uk/media/622965/covidsaunders.pdf (accessed 22 May 2022).
Gkousis E, Saunders K, Sussex J. Inequalities in Access to Primary Care Experienced by People with Multiple Morbidities During the Covid-19 Pandemic. Paper presented at the Health Economists’ Study Group Summer 2021 Meeting, [online] 30 June–2 July 2021.
Ethics statement
This evaluation involves the secondary analysis of data from GPPS. Full details of the ethics and data governance guidance for GPPS are given on the study website. 88
The GPPS data are held by the University of Cambridge under a data-sharing agreement with NHS England, and permission was obtained from the data controller (NHS England) for this analysis.
The USoc data were obtained from the UK Data Archive under a standard end-user agreement [SN8644].
For the analysis of linked USoc data presented in Report Supplementary Material 1, ethics approval for linkage consent at wave 1 was from the National Research Ethics Service (NRES) Oxfordshire REC A (08/H0604/124), at British Household Panel Survey (BHPS) wave 18 it was from the NRES Royal Free Hospital and Medical School (08/H0720/60) and at wave 4 it was from NRES Southampton REC A (11/SC/0274).
Data-sharing statement
The GPPS data were shared for the specific purpose of this work and will not be made publicly available. The USoc COVID-19 data set is available to researchers via the UK Data Archive. For any queries, please contact the corresponding author.
Patient data
This work uses data provided by patients and collected by the NHS as part of their care and support. Using patient data are vital to improve health and care for everyone. There is huge potential to make better use of information from people’s patient records, to understand more about disease, develop new treatments, monitor safety, and plan NHS services. Patient data should be kept safe and secure, to protect everyone’s privacy, and it’s important that there are safeguards to make sure that it is stored and used responsibly. Everyone should be able to find out about how patient data are used. #datasaveslives You can find out more about the background to this citation here: https://understandingpatientdata.org.uk/data-citation
Disclaimers
This report presents independent research funded by the National Institute for Health and Care Research (NIHR). The views and opinions expressed by authors in this publication are those of the authors and do not necessarily reflect those of the NHS, the NIHR, the HSDR programme or the Department of Health and Social Care. If there are verbatim quotations included in this publication the views and opinions expressed by the interviewees are those of the interviewees and do not necessarily reflect those of the authors, those of the NHS, the NIHR, the HSDR programme or the Department of Health and Social Care.
References
- Hobbs FDR, Bankhead C, Mukhtar T, Stevens S, Perera-Salazar R, Holt T, et al. Clinical workload in UK primary care: a retrospective analysis of 100 million consultations in England, 2007–14. Lancet 2016;387:2323-30. https://doi.org/10.1016/S0140-6736(16)00620-6.
- Office for National Statistics . National Life Tables, UK: 2015 to 2017 2018. www.ons.gov.uk/peoplepopulationandcommunity/birthsdeathsandmarriages/lifeexpectancies/bulletins/nationallifetablesunitedkingdom/2015to2017 (accessed January 2022).
- Salisbury C, Lay-Flurrie S, Bankhead CR, Fuller A, Murphy M, Caddick B, et al. Measuring the complexity of general practice consultations: a Delphi and cross-sectional study in English primary care. Br J Gen Pract 2021;71:e423-e431. https://doi.org/10.3399/BJGP.2020.0486.
- Asaria M, Cookson R, Fleetcroft R, Ali S. Unequal socioeconomic distribution of the primary care workforce: whole-population small area longitudinal study. BMJ Open 2016;6. https://doi.org/10.1136/bmjopen-2015-008783.
- Ford J, Sowden S, Olivera J, Bambra C, Gimson A, Aldridge R, et al. Transforming health systems to reduce health inequalities. Future Healthc J 2021;8:e204-e209. https://doi.org/10.7861/fhj.2021-0018.
- Hart JT. The inverse care law. Lancet 1971;1:405-12. https://doi.org/10.1016/S0140-6736(71)92410-X.
- Cowling TE, Harris MJ, Majeed A. Evidence and rhetoric about access to UK primary care. BMJ 2015;350. https://doi.org/10.1136/bmj.h1513.
- Cowling TE, Harris MJ, Watt HC, Gibbons DC, Majeed A. Access to general practice and visits to accident and emergency departments in England: cross-sectional analysis of a national patient survey. Br J Gen Pract 2014;64:e434-9. https://doi.org/10.3399/bjgp14X680533.
- Cowling TE, Gunning EJ. Access to general practice in England: political, theoretical, and empirical considerations. Br J Gen Pract 2016;66:e680-2. https://doi.org/10.3399/bjgp16X686977.
- Cowling TE, Cecil EV, Soljak MA, Lee JT, Millett C, Majeed A, et al. Access to primary care and visits to emergency departments in England: a cross-sectional, population-based study. PLOS ONE 2013;8. https://doi.org/10.1371/journal.pone.0066699.
- NHS England . The NHS Long Term Plan 2019. www.longtermplan.nhs.uk/wp-content/uploads/2019/08/nhs-long-term-plan-version-1.2.pdf (accessed January 2022).
- NHS England . GP Access Fund 2017. www.england.nhs.uk/gp/gpfv/redesign/improving-access/gp-access-fund/ (accessed January 2022).
- NHS England . Improving Access for All: Reducing Inequalities in Access to General Practice Services 2017. www.england.nhs.uk/publication/improving-access-for-all-reducing-inequalities-in-access-to-general-practice-services/ (accessed January 2022).
- NHS England . A Five-Year Framework for GP Contract Reform to Implement The NHS Long Term Plan 2019. www.england.nhs.uk/publication/gp-contract-five-year-framework/ (accessed January 2022).
- Saunders CL, Flynn S, Massou E, Lyratzopoulos G, Abel G, Burt J. Sociodemographic inequalities in patients’ experiences of primary care: an analysis of the General Practice Patient Survey in England between 2011 and 2017. J Health Serv Res Policy 2021;26:198-207. https://doi.org/10.1177/1355819620986814.
- Bienkowska-Gibbs T, King S, Saunders CL, Henham M-L. New Organisational Models of Primary Care to Meet the Future Needs of the NHS: A Brief Overview of Recent Reports 2015.
- NHS England . General Practice Forward View 2016. www.england.nhs.uk/gp/gpfv/ (accessed January 2022).
- Ball SL, Newbould J, Corbett J, Exley J, Pitchforth E, Roland M. Qualitative study of patient views on a ‘telephone-first’ approach in general practice in England: speaking to the GP by telephone before making face-to-face appointments. BMJ Open 2018;8. https://doi.org/10.1136/bmjopen-2018-026197.
- Newbould J, Abel G, Ball S, Corbett J, Elliott M, Exley J, et al. Evaluation of telephone first approach to demand management in English general practice: observational study. BMJ 2017;358. https://doi.org/10.1136/bmj.j4197.
- Newbould J, Ball S, Abel G, Barclay M, Brown T, Corbett J, et al. A ‘telephone first’ approach to demand management in English general practice: a multimethod evaluation. Health Serv Deliv Res 2019;7. https://doi.org/10.3310/hsdr07170.
- Newbould J, Exley J, Ball S, Corbett J, Pitchforth E, Roland M. GPs’ and practice staff’s views of a telephone first approach to demand management: a qualitative study in primary care. Br J Gen Pract 2019;69:e321-e8. https://doi.org/10.3399/bjgp19X702401.
- Campbell JL, Fletcher E, Britten N, Green C, Holt TA, Lattimer V, et al. Telephone triage for management of same-day consultation requests in general practice (the ESTEEM trial): a cluster-randomised controlled trial and cost-consequence analysis. Lancet 2014;384:1859-68. https://doi.org/10.1016/S0140-6736(14)61058-8.
- Clarke G, Pariza P, Wolters A. How Has COVID-19 Affected Service Delivery in GP Practices That Offered Remote Consultations Before the Pandemic? 2020. www.health.org.uk/news-and-comment/charts-and-infographics/how-has-covid-19-affected-service-delivery-in-gp-practices (accessed January 2022).
- Watt T, Firth Z, Fisher R, Thorlby R, Kelly E. Use of Primary Care During the COVID-19 Pandemic: Patient-Level Data Analysis of the Impact of COVID-19 on Primary Care Activity in England. London: The Health Foundation; 2020.
- NHS England . GP Patient Survey National Report 2021 Publication 2021.
- Dunn P, Allen L, Cameron G, Malhotra AM, Alderwick H. A Timeline of National Policy and Health System Responses to COVID-19 in 2020 2020. www.health.org.uk/news-and-comment/charts-and-infographics/covid-19-policy-tracker (accessed January 2022).
- Royal College of General Practitioners . The Future Role of Remote Consultations &Amp; Patient ‘Triage’ 2021. www.rcgp.org.uk/policy/general-practice-covid-19-recovery-consultations-patient-triage.aspx (accessed January 2022).
- British Medical Association . Reducing COVID-19 Transmission and PPE 2021. www.bma.org.uk/advice-and-support/covid-19/gp-practices/covid-19-toolkit-for-gps-and-gp-practices/reducing-covid-19-transmission-and-ppe (accessed January 2022).
- eConsult Health Limited . EConsult n.d. https://econsult.net (accessed 22 May 2022).
- Parker RF, Figures EL, Paddison CA, Matheson JI, Blane DN, Ford JA. Inequalities in general practice remote consultations: a systematic review. BJGP Open 2021;5. https://doi.org/10.3399/BJGPO.2021.0040.
- Whitty CJM, MacEwen C, Goddard A, Alderson D, Marshall M, Calderwood C, et al. Rising to the challenge of multimorbidity. BMJ 2020;368. https://doi.org/10.1136/bmj.l6964.
- Roland M, Paddison C. Better management of patients with multimorbidity. BMJ 2013;346. https://doi.org/10.1136/bmj.f2510.
- Cummings MJ, Baldwin MR, Abrams D, Jacobson SD, Meyer BJ, Balough EM, et al. Epidemiology, clinical course, and outcomes of critically ill adults with COVID-19 in New York City: a prospective cohort study. Lancet 2020;395:1763-70. https://doi.org/10.1016/S0140-6736(20)31189-2.
- Salisbury C, Man MS, Bower P, Guthrie B, Chaplin K, Gaunt DM, et al. Management of multimorbidity using a patient-centred care model: a pragmatic cluster-randomised trial of the 3D approach. Lancet 2018;392:41-50. https://doi.org/10.1016/S0140-6736(18)31308-4.
- Mujica-Mota RE, Roberts M, Abel G, Elliott M, Lyratzopoulos G, Roland M, et al. Common patterns of morbidity and multi-morbidity and their impact on health-related quality of life: evidence from a national survey. Qual Life Res 2015;24:909-18. https://doi.org/10.1007/s11136-014-0820-7.
- Paddison CA, Saunders CL, Abel GA, Payne RA, Campbell JL, Roland M. Why do patients with multimorbidity in England report worse experiences in primary care? Evidence from the General Practice Patient Survey. BMJ Open 2015;5. https://doi.org/10.1136/bmjopen-2014-006172.
- Paddison CA, Saunders CL, Abel GA, Payne RA, Adler AI, Graffy JP, et al. How do people with diabetes describe their experiences in primary care? Evidence from 85,760 patients with self-reported diabetes from the English General Practice Patient Survey. Diabetes Care 2015;38:469-75. https://doi.org/10.2337/dc14-1095.
- Stafford M, Steventon A, Thorlby R, Fisher R, Turton C, Deeny S. Understanding the Health Care Needs of People with Multiple Health Conditions. London: The Health Foundation; 2018.
- Gopfert A, Deeny SR, Fisher R, Stafford M. Primary care consultation length by deprivation and multimorbidity in England: an observational study using electronic patient records. Br J Gen Pract 2021;71:e185-e192. https://doi.org/10.3399/bjgp20X714029.
- Smith J, Sussex J, Ntouva A, Leach B. Protocol: Overarching Study of Service Innovations for People With Multiple Long-Term Conditions: What Works for Whom, How and Why? 2021. https://fundingawards.nihr.ac.uk/award/NIHR134284 (accessed January 2022).
- Powell GA, Bonnett LJ, Tudur-Smith C, Hughes DA, Williamson PR, Marson AG. Using routinely recorded data in the UK to assess outcomes in a randomised controlled trial: the trials of access. Trials 2017;18. https://doi.org/10.1186/s13063-017-2135-9.
- Khoury MJ, Flanders WD. Nontraditional epidemiologic approaches in the analysis of gene-environment interaction: case-control studies with no controls!. Am J Epidemiol 1996;144:207-13. https://doi.org/10.1093/oxfordjournals.aje.a008915.
- Bardsley M, Steventon A, Smith J, Dixon J. Evaluating Integrated and Community-Based Care: How Do We Know What Works?. London: Nuffield Trust; 2013.
- Ioannidis JP. Why most published research findings are false. PLOS Med 2005;2. https://doi.org/10.1371/journal.pmed.0020124.
- NHS . GP Patient Survey n.d. www.gp-patient.co.uk (accessed 22 May 2022).
- Understanding Society . The UK Household Longitudinal Study n.d. www.understandingsociety.ac.uk (accessed 22 May 2022).
- Office for National Statistics . Population Estimates for the UK, England and Wales, Scotland and Northern Ireland: Mid-2020 2021. www.ons.gov.uk/peoplepopulationandcommunity/populationandmigration/populationestimates/bulletins/annualmidyearpopulationestimates/mid2020#population-change-for-uk-countries (accessed January 2022).
- Ipsos MORI. GP Patient Survey Technical Annex 2015–2016 2016. www.gp-patient.co.uk/downloads/archive/2016/July/July%202016%20National%20Technical%20Report.pdf (accessed January 2022).
- Ipsos MORI. GP Patient Survey Technical Annex 2017 Annual Report 2017. www.gp-patient.co.uk/downloads/archive/2017/GPPS%202017%20Technical%20Annex%20PUBLIC.pdf (accessed January 2022).
- Benzeval M, Burton J, Crossley TF, Fisher P, Gardiner C, Jäckle A, et al. Understanding Society Working Paper Series 2021-03: High Frequency Online Data Collection in an Annual Household Panel Study: Some Evidence on Bias Prevention and Bias Adjustment. Colchester: Institute for Social and Economic Research, University of Essex; 2021.
- Payne RA, Mendonca SC, Elliott MN, Saunders CL, Edwards DA, Marshall M, et al. Development and validation of the Cambridge Multimorbidity Score. CMAJ 2020;192:E107-E114. https://doi.org/10.1503/cmaj.190757.
- University of Essex . In House Analysis of Understanding Society Linked Scottish Morbidity Records 2019.
- Payne RA, Avery AJ, Duerden M, Saunders CL, Simpson CR, Abel GA. Prevalence of polypharmacy in a Scottish primary care population. Eur J Clin Pharmacol 2014;70:575-81. https://doi.org/10.1007/s00228-013-1639-9.
- Payne RA, Abel GA, Guthrie B, Mercer SW. The effect of physical multimorbidity, mental health conditions and socioeconomic deprivation on unplanned admissions to hospital: a retrospective cohort study. CMAJ 2013;185:E221-8. https://doi.org/10.1503/cmaj.121349.
- Clegg A, Bates C, Young J, Ryan R, Nichols L, Ann Teale E, et al. Development and validation of an electronic frailty index using routine primary care electronic health record data. Age Ageing 2016;45:353-60. https://doi.org/10.1093/ageing/afw039.
- Fortin M, Stewart M, Poitras ME, Almirall J, Maddocks H. A systematic review of prevalence studies on multimorbidity: toward a more uniform methodology. Ann Fam Med 2012;10:142-51. https://doi.org/10.1370/afm.1337.
- Ho IS, Azcoaga-Lorenzo A, Akbari A, Black C, Davies J, Hodgins P, et al. Examining variation in the measurement of multimorbidity in research: a systematic review of 566 studies. Lancet Public Health 2021;6:e587-e597. https://doi.org/10.1016/S2468-2667(21)00107-9.
- University of Essex, Institute for Social and Economic Research . Understanding Society: COVID-19 Study, 2020–2021 2021.
- Lynn P, Kaminska O. Understanding Society Working Paper Series: Weighting Strategy for Understanding Society. Colchester: Understanding Society; 2010.
- Saunders CL, Sussex J. Study Protocol: The Impact of Telephone Triage in Primary Care on Inequalities Experienced by People With Multiple Morbidities: A Quantitative Evaluation 2021. https://fundingawards.nihr.ac.uk/award/NIHR134285 (accessed January 2022).
- Lynn P, Burton J, Kaminska O, Kneis G, Nandi A. Understanding Society Working Paper Series: An Initial Look at Non-Response and Attrition in Understanding Society. Colchester: Understanding Society; 2012.
- Atherton H, Brant H, Ziebland S, Bikker A, Campbell J, Gibson A, et al. The potential of alternatives to face-to-face consultation in general practice, and the impact on different patient groups: a mixed-methods case study. Health Serv Deliv Res 2018;6. https://doi.org/10.3310/hsdr06200.
- Ipsos MORI, York Health Economics Consortium, Salisbury C . Evaluation of Babylon GP At Hand: Final Evaluation Report 2019. allcatsrgrey.org.uk/wp/download/informatics/Evaluation-of-Babylon-GP-at-Hand-Final-Report.pdf (accessed January 2022).
- Weiss D, Rydland HT, Øversveen E, Jensen MR, Solhaug S, Krokstad S. Innovative technologies and social inequalities in health: a scoping review of the literature. PLOS ONE 2018;13. https://doi.org/10.1371/journal.pone.0195447.
- Healthwatch England, National Voices, Traverse, Private Public Limited . The Doctor Will Zoom You Now: Getting the Most Out of the Virtual Health and Care Experience – Insight Report: Key Findings from Research June to July 2020 2020. www.healthwatch.co.uk/report/2020-08-18/doctor-will-zoom-you-now-getting-most-out-virtual-health-and-care-experience (accessed January 2022).
- Leijten FRM, Struckmann V, van Ginneken E, Czypionka T, Kraus M, Reiss M, et al. The SELFIE framework for integrated care for multi-morbidity: development and description. Health Policy 2018;122:12-2. https://doi.org/10.1016/j.healthpol.2017.06.002.
- Smith J, Sussex J, Ntouva A, Leach B. Protocol: Overarching Study of Service Innovations for People With Multiple Long-Term Conditions: What Works for Whom, How and Why? 2021. https://fundingawards.nihr.ac.uk/award/NIHR134284 (accessed January 2022).
- Dixon-Woods M, Cavers D, Agarwal S, Annandale E, Arthur A, Harvey J, et al. Conducting a critical interpretive synthesis of the literature on access to healthcare by vulnerable groups. BMC Med Res Methodol 2006;6. https://doi.org/10.1186/1471-2288-6-35.
- Rolewicz L, Keeble E, Paddison C, Scobie S. Are the needs of people with multiple long-term conditions being met? Evidence from the 2018 General Practice Patient Survey. BMJ Open 2020;10. https://doi.org/10.1136/bmjopen-2020-041569.
- Pierce M, Hope H, Ford T, Hatch S, Hotopf M, John A, et al. Mental health before and during the COVID-19 pandemic: a longitudinal probability sample survey of the UK population. Lancet Psychiatry 2020;7:883-92. https://doi.org/10.1016/S2215-0366(20)30308-4.
- Pierce M, McManus S, Hope H, Hotopf M, Ford T, Hatch SL, et al. Mental health responses to the COVID-19 pandemic: a latent class trajectory analysis using longitudinal UK data. Lancet Psychiatry 2021;8:610-19. https://doi.org/10.1016/S2215-0366(21)00151-6.
- Hull SA, Mathur R, Badrick E, Robson J, Boomla K. Recording ethnicity in primary care: assessing the methods and impact. Br J Gen Pract 2011;61:e290-4. https://doi.org/10.3399/bjgp11X572544.
- Jain A, van Hoek AJ, Walker JL, Mathur R, Smeeth L, Thomas SL. Identifying social factors amongst older individuals in linked electronic health records: an assessment in a population based study. PLOS ONE 2017;12. https://doi.org/10.1371/journal.pone.0189038.
- Mathur R, Bhaskaran K, Chaturvedi N, Leon DA, vanStaa T, Grundy E, et al. Completeness and usability of ethnicity data in UK-based primary care and hospital databases. J Public Health 2014;36:684-92. https://doi.org/10.1093/pubmed/fdt116.
- Saunders CL, Abel GA, El Turabi A, Ahmed F, Lyratzopoulos G. Accuracy of routinely recorded ethnic group information compared with self-reported ethnicity: evidence from the English Cancer Patient Experience survey. BMJ Open 2013;3. https://doi.org/10.1136/bmjopen-2013-002882.
- Saunders CL, Steventon A, Janta B, Stafford M, Sinnott C, Allen L, et al. Healthcare utilization among migrants to the UK: cross-sectional analysis of two national surveys. J Health Serv Res Policy 2021;26:54-61. https://doi.org/10.1177/1355819620911392.
- Murphy M, Scott LJ, Salisbury C, Turner A, Scott A, Denholm R, et al. Implementation of remote consulting in UK primary care following the COVID-19 pandemic: a mixed-methods longitudinal study. Br J Gen Pract 2021;71:e166-e177. https://doi.org/10.3399/BJGP.2020.0948.
- Fraser C, Fisher R. How Has the COVID-19 Pandemic Impacted Primary Care? 2021. www.health.org.uk/news-and-comment/charts-and-infographics/how-has-the-covid-19-pandemic-impacted-primary-care (accessed January 2022).
- Greenhalgh T, Rosen R. Remote by default general practice: must we, should we, dare we? Editorial. Br J Gen Pract 2021;71:149-50. https://doi.org/10.3399/bjgp21X715313.
- Salman B, Hillman S, Atherton H, Dale J. Remote by default general practice: must we, should we, dare we?. Br J Gen Pract 2021;71. https://doi.org/10.3399/bjgp21X715925.
- British Medical Association . GPs Let Down by ‘Tone Deaf’ NHS England Letter on Face-to-Face Appointments, Says BMA 2021. www.bma.org.uk/bma-media-centre/gps-let-down-by-tone-deaf-nhs-england-letter-on-face-to-face-appointments-says-bma (accessed January 2022).
- Newbould J, Sidhu M, Hocking L. Protocol: Digital First Primary Care for Those With Multiple Long-Term Conditions: The Views of Patients, Carers and Health Professionals 2021. https://fundingawards.nihr.ac.uk/award/NIHR134286 (accessed January 2022).
- Campbell SM, Reeves D, Kontopantelis E, Sibbald B, Roland M. Effects of pay for performance on the quality of primary care in England. N Engl J Med 2009;361:368-78. https://doi.org/10.1056/NEJMsa0807651.
- Lynn P, Borkowska M. Understanding Society Working Paper Series: Some Indicators of Sample Representativeness and Attrition Bias for BHPS and Understanding Society. Colchester: Understanding Society; 2018.
- Jackle A, Pudney S. Understanding Society Working Paper Series: Survey Response Behaviour and the Dynamics of Self-Reported Health and Disability: An Experimental Analysis 2015.
- Saunders CL, Elliott MN, Lyratzopoulos G, Abel GA. Do differential response rates to patient surveys between organizations lead to unfair performance comparisons? Evidence from the English Cancer Patient Experience Survey. Med Care 2016;54:45-54. https://doi.org/10.1097/MLR.0000000000000457.
- The Patients Association . Pandemic Patient Experience: UK Patient Experience of Health, Care and Other Support During the COVID-19 Pandemic 2020.
- GP Patient Survey . GP Patient Survey: Confidentiality and Data Protection n.d. www.gp-patient.co.uk/confidentiality (accessed 22 May 2022).
List of abbreviations
- BRACE
- Birmingham, RAND and Cambridge Rapid Evaluation Centre
- CI
- confidence interval
- EQ
- evaluation question
- GP
- general practitioner
- GPPS
- The GP Patient Survey
- OR
- odds ratio
- PPI
- patient and public involvement
- SELFIE
- Sustainable intEgrated chronic care modeLs for multimorbidity: delivery, FInancing, and performancE
- USoc
- Understanding Society
Notes
-
Survey questions: long-term health conditions in GPPS and USoc
-
Inequalities in time taken to see or speak to a GP (adjusted, presented on a 0–100 scale, with 100 being the most positive experience) among people with multimorbidity, stratified by caregiving responsibilities, GPPS
-
Inequalities in time taken to see or speak to a GP (adjusted, presented on a 0–100 scale, with 100 being the most positive experience) among people with multimorbidity, stratified by sexual orientation, GPPS
-
The impact of introducing telephone triage among people with multimorbidity, GPPS: caregivers and sexual orientation
Supplementary material can be found on the NIHR Journals Library report page (https://doi.org/10.3310/UCCE5549).
Supplementary material has been provided by the authors to support the report and any files provided at submission will have been seen by peer reviewers, but not extensively reviewed. Any supplementary material provided at a later stage in the process may not have been peer reviewed.