Notes
Article history
The research reported in this issue of the journal was funded by the PHR programme as project number 12/211/69. The contractual start date was in June 2014. The final report began editorial review in February 2019 and was accepted for publication in June 2019. The authors have been wholly responsible for all data collection, analysis and interpretation, and for writing up their work. The PHR editors and production house have tried to ensure the accuracy of the authors’ report and would like to thank the reviewers for their constructive comments on the final report document. However, they do not accept liability for damages or losses arising from material published in this report.
Declared competing interests of authors
Christopher G Owen reports that he is an active member of the Public Health Research (PHR) Research Funding Committee (16 June 2015 to present). Steven Cummins was a member of the PHR Research Funding Board (13 May 2009 to 12 May 2015). Diabetes mellitus and obesity prevention research at St George’s, University of London is supported by the National Institute for Health Research (NIHR) Collaboration for Leadership in Applied Health Research and Care (CLAHRC) South London. Bina Ram was supported by a St George’s, University of London, PhD Studentship. Anne Ellaway is funded by the UK Medical Research Council as part of the Neighbourhoods and Communities Programme (MC_UU_12017–10). Billie Giles-Corti is supported by a National Health and Medical Research Council Principal Research Fellowship (#1107672). Ashley R Cooper and Angie S Page are supported by the NIHR Biomedical Research Centre at the University Hospitals Bristol NHS Foundation Trust and the University of Bristol.
Permissions
Copyright statement
© Queen’s Printer and Controller of HMSO 2020. This work was produced by Owen et al. under the terms of a commissioning contract issued by the Secretary of State for Health and Social Care. This issue may be freely reproduced for the purposes of private research and study and extracts (or indeed, the full report) may be included in professional journals provided that suitable acknowledgement is made and the reproduction is not associated with any form of advertising. Applications for commercial reproduction should be addressed to: NIHR Journals Library, National Institute for Health Research, Evaluation, Trials and Studies Coordinating Centre, Alpha House, University of Southampton Science Park, Southampton SO16 7NS, UK.
2020 Queen’s Printer and Controller of HMSO
Chapter 1 Background to the ENABLE London study
Importance of physical inactivity
Physical inactivity is one of the leading causes of premature mortality, responsible for over 5 million deaths per year worldwide. 1 Low levels of physical activity are associated with numerous adverse health outcomes in adulthood, including coronary heart disease, stroke, type 2 diabetes mellitus and cancers. 2 The annual global health-care cost of physical inactivity has been estimated to be near $54B. 3 In the UK, physical activity levels are undesirably low, with over one-third of men and 40% of women not meeting physical activity recommendations of at least 150 minutes of moderate activity per week. 4 The important need to address this has resulted in initiatives to increase population levels of physical activity, which have become enshrined within public health policy. 5,6 Evidence suggests that levels of physical activity are lowest among those who are socioeconomically disadvantaged7 and those who experience greater economic, access and health-related barriers to being physically active,8 which potentially drives social inequalities. Socioeconomic status is also associated with differences in the types of physical activity observed; in particular, higher socioeconomic status is associated with more vigorous leisure-time physical activity. 9 Previous research has found variation in physical activity by day of the week, with studies showing lower levels of activity on Sundays than weekdays in young adults10 and parents and their children. 11
Housing tenure
There is emerging evidence, from UK-based studies12,13 in particular, suggesting that housing tenure (owner vs. private renter vs. public sector renter) may be an important determinant of health. Among particular groups, including those who are economically inactive or unemployed, housing tenure might provide a better indication of socioeconomic status than measures based on occupation or income. 14 Indeed, in several studies housing tenure remained associated with health outcomes following adjustment for conventional measures of socioeconomic status, such as income or education. 12,15 A more nuanced approach is therefore required with respect to measures of socioeconomic status, and they should not be simply regarded as interchangeable. 13,16 Despite this, there has been limited research examining the direct effect of housing tenure on physical activity, and existing evidence is equivocal. Harrison et al. 17 found no association between housing tenure and meeting the recommended levels of physical activity among community-dwelling healthy adults in the north-east of England. 17 Similarly, housing tenure was not associated with self-reported energetic physical activity among older Australians. 18 Ogilvie et al. 19 found overall levels of physical activity to be higher in individuals living in social housing than in owner-occupiers. 19 The authors suggest that social housing tenure may capture occupational physical activity levels that are likely to be higher among those in social housing. 19 By contrast, living in private-rented accommodation was associated with a greater likelihood of taking up exercise over a 9-year period among men aged 18–49 years at baseline than living in local authority accommodation. 20
The residential built environment
Housing tenure may affect health and health behaviours in part through characteristics of the home or the neighbourhood itself,21,22 or through psychological factors such as self-efficacy or self-esteem. 23 Social housing estates, which are common in the UK, may be associated with specific cultures and norms, which in turn shape residents’ behaviours. 12 Subjective characteristics of the neighbourhood environment, such as higher perceived access to recreational facilities and shops in local proximity, have been shown to be associated with higher levels of physical activity. 24,25 Residents who perceive their neighbourhood more positively have been shown to have better mental health and are less likely to relocate. 26 Conversely, real and perceived crime has the potential to constrain residents’ physical activity. 27 However, a recent systematic review suggested a lack of association between physical activity and perceptions of safety from crime; this highlights the need for high-quality evidence, including prospective studies and natural experiments,28 to examine this issue further. In particular, high-quality evidence is needed to understand the potentially multifactorial influence of residential location on health and health behaviours, with effects that are likely to extend beyond simple measures of socioeconomic status. 28
Dose–response meta-analyses suggest that even small increases in physical activity would confer significant health benefits, particularly for cardiovascular and diabetes mellitus-related outcomes. 2 Increasing physical activity levels, particularly among those living in more disadvantaged neighbourhoods, is an important public health priority. However, a wide range of community-wide interventions to increase population levels of physical activity, particularly those associated with change in the residential built environment, have failed to show consistently beneficial effects. 29 Moreover, where modest effects have been shown, there is very little evidence and persistence of effects is not routinely assessed. 29 Despite this, community-wide interventions related to the built environment remain attractive given their population reach.
Physical activity and the built environment
There is growing literature that investigates the influence of the built environment on physical activity levels. 30 It seems highly plausible that improved walkability and improved access to green space, public transport and recreational facilities would encourage increased physical activity. 31 However, evidence is heavily reliant on cross-sectional studies,32 which are prone to selection bias, in which people who are living in less walkable neighbourhoods are often intrinsically different from those who are not. Such studies make it difficult to establish temporality or to infer causal effects. 33 Moreover, evidence has been mixed showing built environment-related associations with adiposity, but not with physical activity, which suggests that other health behaviours might be affected. 34,35 Longitudinal studies have sought to establish causal associations of physical activity and the built environment, but the number of studies are few and the systematic reviews of studies, to date, have found this evidence less convincing. 29,33,36 Specific methodological weaknesses have included (1) comparing movers with non-movers, who may be inherently different and have different reasons for moving or staying that govern their physical activity patterns,33 and (2) often relying on self-reported physical activity, which is prone to measurement error and reporting bias,37 and which could have an appreciable impact on the reporting of health outcomes at a population level. 38 There have been increasing calls for high-quality evidence, particularly natural experiments, to evaluate the effect of the built environment on health behaviours, specifically physical activity levels, in which the population effects of sizeable change in the built environment can be examined. 39–41 However, there have been limited opportunities to carry out such studies.
The ENABLE London study
The Examining Neighbourhood Activities in Built Living Environments in London (ENABLE London) study takes advantage of the natural experiment provided by the rapid change of brown-field land in the London Borough of Newham, UK, to create a novel built environment for public use and occupancy (namely ‘East Village’ E20, formerly the London 2012 Olympic and Paralympic Games Athletes’ Village). The extensive features of East Village, which make this sizeable change in the built environment suitable for evaluation as an intervention, are outlined below.
The East Village ‘intervention’
The planned intervention was the mixed-use development of the East Village residential neighbourhood incorporating commercial, retail, educational and transportation resources.
Specific activity-permissive features designed to encourage physical activity include improved access to open land and parkland, unrivalled transport links, and active travel options (including extensive walking and cycling paths), design features of the local environment (such as street furniture, provision and arrangement of pedestrianised space, public space aesthetics, secure bicycle parking) and the provision of new formal cycling and walking facilities in the Queen Elizabeth Olympic Park, such as the VeloPark, and cycle paths that extend into the Lee Valley and connect to the London Cycle Network. 42,43 A local school, Chobham Academy, is within walking distance and provides schooling for all 3- to 19-year-olds. Retail outlets were planned within easy walking distance for everyday use (creating plazas at ground level within dedicated areas of East Village). 42,43 Moreover, East Village is in close proximity to Westfield Stratford City – Europe’s largest urban shopping centre. Restriction of resident car parking (where fewer than one-sixth of homes have a designated parking space) combined with improved public transport links is designed to encourage local residents to adopt active modes of transport. 42,43
Accommodation and housing tenures in East Village
East Village consists of 2818 units of accommodation, of which 1379 are social and intermediate accommodation (including shared ownership, shared equity and affordable market-rent), and 1439 are for market rent (private rent). The housing development includes a mixture of one- to four-bedroom town houses and apartments designed for single occupancy through to family-sized accommodation. Details of the different housing types are given below:
-
Social housing – approximately 675 units were available to those on the social housing register. The social housing sector is managed by East Thames Group and Triathlon Homes housing associations.
-
Intermediate accommodation – owned by Triathlon Homes (in association with the East Thames Group) with a total of 704 units (a mix of 79 shared ownership units, 269 shared equity units and 356 affordable market-rent units).
-
Market-rent accommodation – 1439 units owned by Get Living London for private rent.
Main research questions
The ENABLE London study sought to address the following primary research question: do those living in social, intermediate and market-rent accommodation in East Village show a sustained change in their physical activity levels, compared with their levels before moving into the Village, and compared with physical activity change among those who did not move into East Village?
Specific objectives of the study were to examine:
-
Whether or not any increase in physical activity observed among those living in East Village is directly attributable to the use of their local built environment and, if so, which facilities (e.g. open spaces, cycle paths, pedestrian walkways, recreational or green space, sporting venues) are important.
-
Whether or not changes in physical activity patterns in those living in East Village are modified by other factors, including age, sex, ethnicity, proximity to facilities, associated with living in East Village, housing tenure (as a marker of socioeconomic status) and employment status.
-
Whether or not adiposity levels among those living in East Village show a change from levels before moving into East Village, compared with any changes observed among those who did not move to East Village over the same period.
-
If changes in adiposity levels were observed, whether or not, and to what extent, changes in adiposity over the study period reflect changes in physical activity.
Secondary research questions included:
-
Does moving to East Village improve levels of mental health (depression and anxiety) and well-being, compared with those who did not move?
-
Does moving to East Village improve measures of the built environment and neighbourhood perceptions before and after moving, compared with those who remained outside East Village throughout?
This report
This report presents an original detailed description and summary of a large body of research, some of which has been published, submitted, or is being prepared for publication in other open-access academic journals. Further details of methods and findings presented in this report can be found in these publications, which are (1) cited in the report, (2) listed in Publications and (3) available on the study website (www.enable.sgul.ac.uk). We will continue to keep the study website updated with further publications emanating from the study.
Chapter 2 ENABLE London study methodology
Introduction
This chapter provides a description of the methods used in the ENABLE London study, providing an overview of the research design, participant recruitment and data collection. Key exposure and outcome variables collected during the study are outlined, along with a summary of the quantitative data analysis methods used. Derived variables and detailed analytic approaches used for different aspects of the study are described in each chapter accordingly.
Overall research design
The main aim of the study was to investigate the effect of a major change in the residential built environment, triggered by moving to East Village, an area purposefully planned on active design principles and on the physical activity levels of residents, in particular the levels of walking and cycling. There was also a broader set of research questions that this study sought to examine; importantly, examining change in the objective measures of the built environment associated with moving to East Village, in addition to neighbourhood perceptions of the area, to confirm that the development had the desired effect. Without being able to demonstrate change in the built environment (i.e. the primary exposure), change in health-related outcomes attributable to the built environment could not be expected. Other outcomes included change in travel mode and other health behaviours, including mental health and well-being. The study was originally conceived as a longitudinal study of adults seeking to move into the three housing tenures in East Village, with assessments taking place prior to and after the move. Within the cohort would be a control population: those who were seeking to move but did not move to East Village. The quantitative methods implemented in this study were supported by qualitative methods on a small sample of the cohort to further understand drivers and perceptions of moving to East Village and the potential effects on travel behaviours.
The East Village development was scheduled for occupancy in the summer of 2013. The original research design envisaged baseline measures to be taken from late 2012 to early 2013, and follow-up to be taken 1 year later from late 2013 to early 2014. However, delays to the opening of East Village led to the postponement of advertising the accommodation for occupancy and staggered release of accommodation by housing type, with the first residents of East Village not moving in until 2014. The research design of the study was therefore adapted to accommodate the delays to the opening of East Village and the staggered release of accommodation. With the delays in finalising the development and staged release of accommodation, coupled with the scientific need for 2-year as opposed to 1-year follow-up,44 recruitment and baseline assessments of participants took place from 2013 to 2015, with a 2-year follow-up from 2015 to 2017.
Ethics considerations
Ethics approval for the study was provided by City Road and Hampstead Review Board (Research Ethics Committee reference number 12LO1031).
Study population
The inclusion criteria of the ENABLE London study were families residing in Greater London (largely from East London and the London Borough of Newham) who were seeking to move into social, intermediate and market-rent accommodation in East Village. Participants who moved to East Village at follow-up were directly exposed to the new social and built environment, and its active design features. Participants who were seeking to move to East Village but remained in their place of origin (largely from East London) or moved elsewhere formed the control group. Applicants registering an interest or applying to move into East Village were invited to take part in the study (including at least one adult and one child aged ≥ 8 years to allow accelerometer assessment); a maximum of four family members per household were included. Although the study was aimed at families (i.e. parents and children), working-aged adult-only households were also invited. Households with disabled family members were invited to the study when appropriate, although the primary focus of the study was able-bodied family members. Non-English-speaking families were also approached, using interpreters when applicable.
Socioeconomic position
The inclusion of occupants seeking social, intermediate and market-rent accommodation in East Village (largely based on level of income) allowed inclusion of people from widely different social and ethnic origins, representative of the diverse East London population. This allowed sociodemographic differences in the use of the local area and potential effects on health outcomes to be gauged,5,45 particularly among individuals and households of lower socioeconomic status, who potentially have the most to gain from improvements in the residential built environment. 46
Recruitment
Participants were recruited through the East Thames Group Housing Association (responsible for social housing tenants), Triathlon Homes (intermediate housing: affordable market-rent/shared ownership/shared equity) and Get Living London (market-rent). Prospective tenants went through a rigorous financial process to determine eligibility for accommodation. Those applying to move into East Village social housing were provided with information about the study and invited to take part by East Thames Group representatives directly, whereas the ENABLE London team (in association with Triathlon Homes and Get Living London) invited those from intermediate and market-rent housing sectors.
Data collection
Baseline and 2-year follow-up of study participants was carried out at the participants’ home (or at a location convenient to the participant). Data items collected in the ENABLE London study at baseline and follow-up are listed in Box 1 and summarised below.
Physical activity and location data:
-
ActiGraph GT3X+ accelerometer (ActiGraph LLC, Pensacola, FL, USA) worn over a consecutive 7-day period.
-
QStarz BT-1000XT GPS travel recorder (QStarz International Co, Ltd, Taipei, Taiwan) worn for 1 week.
-
Ordnance Survey AddressBase® Premium versions 2015 and 2017 (Ordnance Survey, Southampton, UK) mapping of place of residence at baseline and 2-year follow-up to provide measures of land use mix, street connectivity, residential density, walkability and connectivity indices (see www.ordnancesurvey.co.uk/business-government/products/addressbase-premium; accessed 16 September 2019).
Anthropometry:
-
Height measured to the last complete millimetre (Leicester Stadiometer; Seca, Birmingham, UK).
-
Weight measured to the last complete 0.1 kg using an electronic digital scale, and fat mass (kg), fat-free mass (kg) and muscle mass (kg) measured by leg-to-leg bioimpedance (Tanita SC-240 Body Composition Analyser; Tanita Inc, Tokyo, Japan).
-
Body mass index calculated as weight/height squared in kg/m2.
Questionnaire data:
-
Demographics, including date of birth, gender and ethnicity, of the participant.
-
Number of people living in the household, relationships, type of accommodation, household features (including lifts, stairs, garden), type of tenure, duration at current property, vehicles owned and dog ownership.
-
Qualifications, employment status, and job title of adult participants (based on Census 2011 questions). 45
-
Method of travel to work/place of study and daily commuting times.
-
Household income as either weekly or monthly amounts (based on National Evaluation of Sure Start income questions).
-
Perception of general health, self-report of health problems (based on Census 2011 questions)45 and effects on mobility.
-
Health outcomes including assessments of mobility, self-care, usual activities, pain/discomfort, anxiety/depression and overall perception of health on a scale from 0 to 100 [using EuroQol-5 Dimensions (EQ-5D) questions].
-
Satisfaction scores including perception of overall levels of satisfaction, feeling happy and anxious, on a scale from 1 to 10 (based on questions used in the Measuring National Well-being Programme), and further assessment of anxiety and depression based on the Hospital Anxiety and Depression Scale.
-
Current and previous smoking status, and current alcohol consumption (using Health Survey for England questions47).
-
Perceptions of the local area/neighbourhood, including transport, leisure activities, vandalism, litter, traffic, attractiveness and safety, as well as assessment of social participation, support, cohesion and trust.
-
Type of activities carried out and frequency of carrying out vigorous, moderate, walking, sitting activities in the last 7 days (based on the short-form IPAQ).
-
Cost of activities including membership fees, vouchers received and equipment bought to do physical activity.
-
Attitudes to exercise.
-
Television and computers/screen time assessment.
-
Fruit and vegetable consumption, and usual sleeping times.
IPAQ, International Physical Activity Questionnaire.
Physical activity level/pattern and location
Objectively measured physical activity (daily steps) was the primary outcome and was assessed over a consecutive 7-day period using hip-mounted ActiGraph GT3X+ accelerometers, combined with an assessment of physical activity location using Global Positioning System (GPS) travel recorders (BT-1000XT). Accelerometers provided daily measures of steps and different intensities of activity, including moderate to vigorous physical activity (MVPA) (both overall and in 10-minute bouts, in accordance with UK physical activity recommendations, although levels in bouts were too low to be usefully used in analyses). 5 The approaches to analysing ActiGraph data are detailed in Chapter 3. Simultaneous use of ActiGraph accelerometers and GPS travel recorders allowed active travel components of physical activity (i.e. walking), as well as indoor and outdoor activities, to be identified using methods previously described by the investigators48,49 and the methods detailed in Chapter 5. In addition, GPS data allowed the geographical location at which different levels of physical activity occurred to be identified (from sedentary to vigorous, using established cut-off points in accelerometer data), both at baseline and at follow-up. Together, these measures allowed accelerometry data to be interpreted in depth, allowing the nature and location of recorded activities, particularly active forms of transport such as walking and cycling, to be identified. Moreover, it allowed the contribution of active transport local to place of residence to be quantified and compared between those living in East Village and control areas (see Chapter 5).
Environmental exposures
Geographical information systems (GIS) were used to extract objective data on features of the local environment (see Chapter 4). In combination with ActiGraph and GPS data from study participants, this allowed the location of different levels of physical activity (including both high and low levels of activity) to be accurately identified. This method has been used previously by the investigators to establish the important contribution of walking to school and location (including land use type) to MVPA levels in children. 49,50 A number of data sources were used to identify environmental and activity-permissive features within East Village and control areas, including Ordnance Survey (OS) MasterMap Tomography Layer (versions December 2018, May 2014, June 2015) (Ordnance Survey, URL: www.ordnancesurvey.co.uk/business-government/products/mastermap-topography; accessed September 2019), Integrated Transport Network and Transport for London (TfL)51 sources, Olympic Delivery Authority and local authority data, as well as other online resources. In particular, OS data were used to derive indices, such as land use mix, street connectivity, residential density, walkability and connectivity indices, including walking distance to particular features of the built environment, including green space. 52
Anthropometric measurements
Height was measured to the last complete millimetre with a portable stadiometer (Leicester Stadiometer; Seca, Birmingham, UK) at baseline and follow-up. Both weight and leg-to-leg bioimpedance were assessed using an electronic Tanita SC-240 body composition analyser (Tanita Inc., Tokyo, Japan) to provide measures of fat mass (kg) and fat-free mass (kg); body mass index (BMI) was calculated as weight/height2 (kg/m2). In total, eight Leicester Stadiometers and Tanita SC-240 body composition analysers were used to measure the participants. The Tanita devices were operated using factory default settings and were regularly checked in accordance with recommended review procedures.
Questionnaire data
Questionnaires were converted into electronic format using SNAP Surveys software (version 11, SNAP Surveys, London, UK), and completed by study participants using dedicated laptops. The questionnaires used established validated methodologies to collect detailed information on patterns and types of activity that were local to the place of residence. In particular, the ‘Neighbourhood Physical Activity Questionnaire’ provided data to examine walking within the neighbourhood,53 and the ‘Neighbourhood Environment Walking Scale’ provided data on perceptions of the neighbourhood environment. 54,55 Information on self-defined ethnic origin (based on the 2011 Census45) and a range of social markers were recorded (including employment status, income, duration and location of work), together with home address and postcode of residence, allowing GIS-determined distance to local amenities to be measured. Questions about general health/health status,45 well-being, anxiety and depression, including both clinical and subclinical forms of assessment suitable for use in community settings, were also used (see Chapter 6). 22,56–58 Physical activity was assessed using an adaptation of the short-form, self-reported International Physical Activity Questionnaire (IPAQ)59 to provide perceived levels of physical activity in addition to objective measures. Adults were asked about their attitudes to physical activities (including both sedentary, such as screen time, and physically active forms) and factors that influence their physical activity behaviour. Participants were asked about perceived personal, social and environmental influences on physical activity, their use of recreational space (particularly walkways and cycle paths) and facilities in their residential neighbourhood (including costs incurred). Participants were also asked about the availability, accessibility (method of travel and journey times) and usage of local amenities (walkways, cycle paths, parks, swimming pools, etc.); their perceptions of the safety of these amenities and the degree to which they permit their child independent or supervised use were also elicited. The questionnaire also included sections to ascertain levels of social participation, support, cohesion and trust. 60 These items were particularly relevant to gauge how the use and perceptions of the local area by others had an impact on individual use and how this might differ from objectively measured features of their neighbourhood. The main questionnaire is included in Appendix 1. Questions were repeated at follow-up, but further questions were included to capture use and frequency of use of the Olympic Park and shopping facilities in the East Village area. Additionally, given the 2-year follow-up, a further question was included to establish whether or not participants had ever lived in East Village between baseline and follow-up interviews.
Qualitative data
In addition to the rich quantitative data, focus groups among study participants in the social housing sector, who were the first to move into East Village, were carried out to identify issues of importance, particularly about perceptions and use of their local environment, and to confirm whether or not the ENABLE London study questionnaire was capturing a suitable and accurate insight of constructs that would identify environmental factors influencing health behaviour (see Chapter 7). GIS and GPS data were also combined with qualitative spatial narratives among study participants to explore differences in active travel associated with moving to East Village. These narratives used individual participant maps to assess how the built environment influences travel behaviour and physical activity: examining change in travel patterns, reasons for the change, purpose of travel and choice of route (see Chapter 8).
Sample recruited and examined
A total of 1819 households (749 social, 738 intermediate and 332 market-rent) expressed an interest in taking part in the study, of which 1006 (392 social, 421 intermediate and 193 market-rent) were examined at baseline from January 2013 to January 2016 (Figure 1). More than one person per household was invited to take part. In total, 1497 individuals took part: 1278 adults (520 social, 524 intermediate and 234 market-rent) and 219 children (209 social, eight intermediate and two market-rent). Figure 2 shows the geographic home locations of study participants at baseline, which highlights the Newham focus of those in social housing, and Greater London geographic diversity of participants seeking intermediate and market-rent accommodation. The small number of children recruited was not expected given that the accommodation in East Village was largely designed for family occupation. Most of the children recruited (95%) were from the social housing sector, and very few children were recruited from intermediate and market-rent groups, which were largely adult-only households. The overall number of children was too small to provide sufficient power to detect change in physical activity; therefore, only adults were considered from here on. The smaller number of adult participants recruited from the market-rent sector reflected the limitations placed on the extent and duration of access to applicants seeking this type of accommodation in East Village. The ENABLE London cohort was predicated on recruiting 1200 adults from 1200 households and succeeded in recruiting 1278 adults from 1006 households. Given the modest imbalance between movers and non-movers, the compliance and the follow-up rate observed, the study was powered to detect a 750-step change [0.3 standard deviation (SD)] at 90% power and with a probability of 0.01 among those who move to East Village. 61
FIGURE 1.
Flow diagram of households and participants examined at baseline and follow-up in the ENABLE London study. Reproduced from Ram et al. 61 Published by the BMJ Publishing Group Limited. This is an Open Access article distributed in accordance with the terms of the Creative Commons Attribution (CC BY 4.0) license, which permits others to distribute, remix, adapt and build upon this work, for commercial use, provided the original work is properly cited. See: http://creativecommons.org/licenses/by/4.0/.
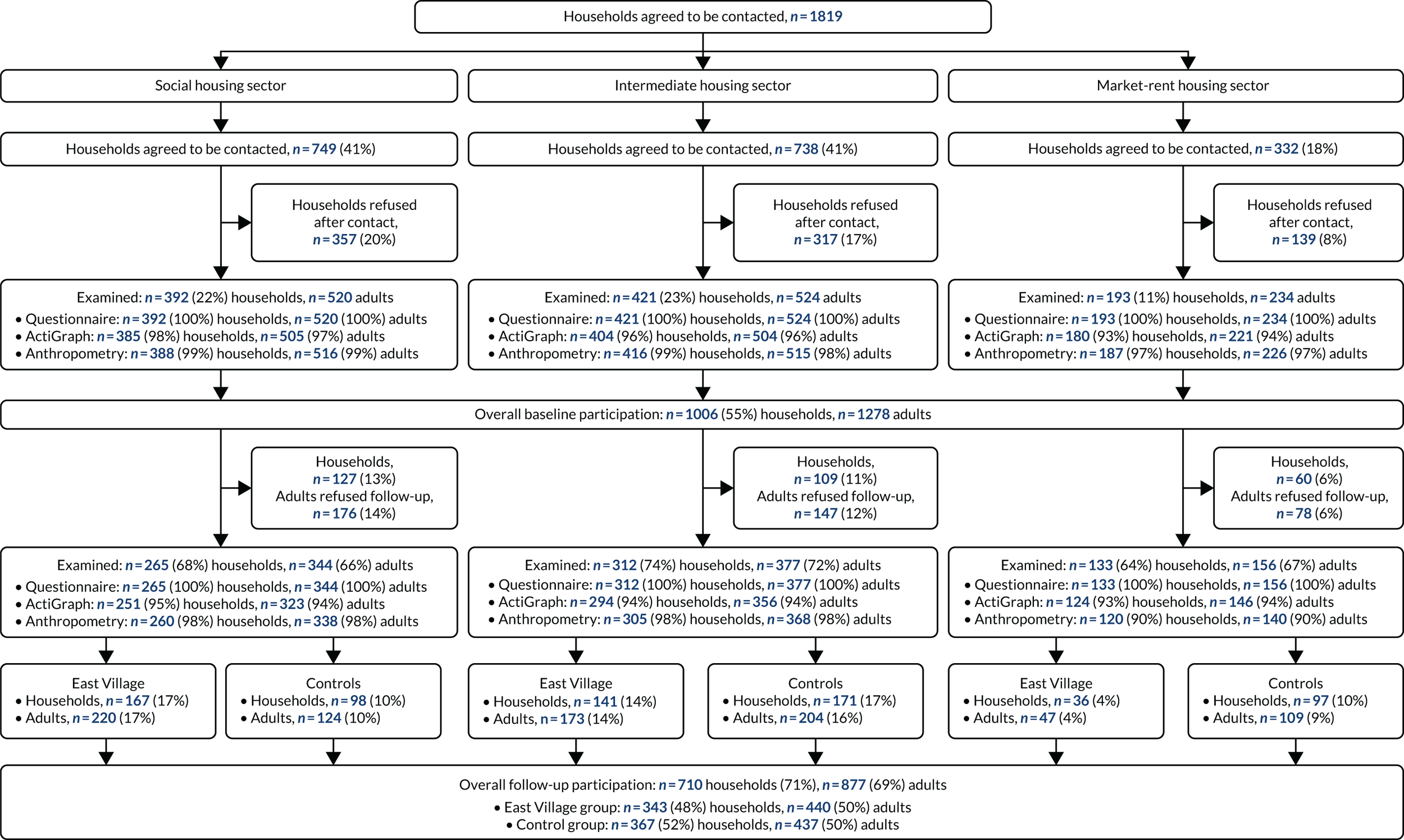
FIGURE 2.
Baseline locations of social, intermediate and market-rent households participating in the ENABLE London study. Reproduced from Ram et al. 61 Published by the BMJ Publishing Group Limited. This is an Open Access article distributed in accordance with the terms of the Creative Commons Attribution (CC BY 4.0) license, which permits others to distribute, remix, adapt and build upon this work, for commercial use, provided the original work is properly cited. See: http://creativecommons.org/licenses/by/4.0/.

The characteristics of the baseline sample by housing sector are summarised in Table 1. Participants seeking social housing in East Village were older, mainly female, and more likely to be from ethnic minority groups than the intermediate and market-rent sectors. The social housing sector was largely residing in households of four or more people (58% vs. intermediate 29% and market-rent 27%), which included children (82% vs. intermediate 18% and market-rent 10%). Only one-quarter (24%) of the social housing sector had a degree qualification compared with 82% of intermediate and 80% of market-rent participants. Half of the social housing sector were employed, compared with 94% of the intermediate and 87% of the market-rent participants. There were also social gradients in the type of employment, with 12% of the social housing sector participants in higher managerial or professional occupations compared with 72% of the intermediate sector participants and 66% of the market-rent sector participants. Fewer participants in the social housing sector reported very good or good overall health (76%) than did intermediate (88%) and market-rent (91%) participants.
Characteristic | All housing sectors, n (%) | Housing sector,a n (%) | ||
---|---|---|---|---|
Social housing | Intermediate | Market-rent | ||
Cohort | ||||
Participants | ||||
Households | 1006 (100) | 392 (52) | 421 (57) | 193 (58) |
Adults | 1278 (100) | 520 (41) | 524 (41) | 234 (18) |
Individual characteristics | ||||
Age, median (IQR) | ||||
Age | 31 (26–40) | 37 (27–44) | 30 (26–35) | 28 (24–33) |
Sex | ||||
Male | 547 (43) | 141 (27) | 275 (52) | 131 (56) |
Female | 731 (57) | 379 (73) | 249 (48) | 103 (44) |
Ethnicity | ||||
White | 617 (48) | 96 (18) | 358 (68) | 163 (70) |
Asian | 214 (17) | 108 (21) | 77 (15) | 29 (12) |
Black | 323 (25) | 251 (48) | 55 (11) | 17 (7) |
Mixed/other | 124 (10) | 65 (13) | 34 (6) | 25 (11) |
Household-level characteristics | ||||
Household composition | ||||
1 person | 97 (8) | 28 (5) | 38 (7) | 31 (13) |
2 people | 385 (30) | 88 (17) | 217 (41) | 80 (34) |
3 people | 278 (22) | 101 (19) | 116 (22) | 61 (26) |
≥ 4 people | 518 (41) | 303 (58) | 153 (29) | 62 (27) |
Living with partner | ||||
Yes | 556 (44) | 202 (39) | 261 (50) | 93 (40) |
No | 615 (48) | 244 (47) | 245 (47) | 126 (54) |
Unknown | 107 (8) | 74 (14) | 18 (3) | 15 (6) |
Living with children | ||||
Yes | 542 (42) | 425 (82) | 93 (18) | 24 (10) |
No | 736 (58) | 95 (18) | 431 (82) | 210 (90) |
Socioeconomic characteristics | ||||
Educationb | ||||
Degree or equivalent/higher | 736 (58) | 122 (24) | 428 (82) | 186 (80) |
Intermediate qualification | 380 (30) | 280 (54) | 70 (13) | 30 (13) |
Other/none | 159 (12) | 117 (23) | 25 (5) | 17 (7) |
Employment statusc | ||||
Employed | 948 (74) | 252 (49) | 492 (94) | 204 (87) |
Unemployed | 91 (7) | 73 (12) | 7 (1) | 11 (5) |
Economically inactive | 236 (18) | 192 (37) | 25 (5) | 19 (8) |
NS-SECd | ||||
Higher managerial/professional | 591 (46) | 61 (12) | 375 (72) | 155 (66) |
Intermediate occupations | 179 (14) | 62 (12) | 79 (15) | 38 (16) |
Routine/manual | 170 (13) | 125 (24) | 34 (6) | 11 (5) |
Health characteristics | ||||
General health status | ||||
Very good | 365 (29) | 140 (27) | 153 (29) | 72 (31) |
Good | 703 (55) | 253 (49) | 310 (59) | 140 (60) |
Fair | 179 (14) | 103 (20) | 58 (11) | 18 (8) |
Bad | 25 (2) | 19 (4) | 2 (0.4) | 4 (2) |
Very bad | 6 (0.5) | 5 (1) | 1 (0.2) | 0 (–) |
Limiting longstanding illness | ||||
Yes | 162 (13) | 107 (21) | 41 (8) | 14 (6) |
Physical activitye | ||||
Daily steps, mean (SD) | 8950 (3190) | 7803 (3303) | 9684 (2924) | 9337 (2990) |
MVPA (minutes/day), mean (SD) | 60 (26) | 50 (26) | 65 (23) | 65 (25) |
MVPA in 10-minute boutsf | 15 (6–30) | 7 (1–15) | 21 (10–34) | 21 (21–36) |
Anthropometry | ||||
Height (m), mean (SD) | 1.69 (0.10) | 1.65 (0.09) | 1.71 (0.10) | 1.72 (0.10) |
Weight (kg)f | 71 (62–82) | 71 (63–84) | 71 (62–81) | 73 (61–80) |
BMI (kg/m2)f | 25 (22–28) | 26.3 (23–31) | 24 (22–27) | 24 (22–26) |
Fat mass (kg)f,g | 18 (13–26) | 23 (16–31) | 15 (11–21) | 15 (11–21) |
Mental health | ||||
Depressionh | 173 (14) | 100 (21) | 52 (10) | 21 (9) |
Anxietyh | 392 (31) | 169 (33) | 146 (28) | 77 (33) |
Well-being | ||||
Low levels of life satisfactioni | 330 (26) | 169 (33) | 117 (22) | 44 (19) |
Low levels of feeling that life is worthwhilei | 265 (21) | 123 (24) | 95 (18) | 47 (20) |
Low levels of feeling happyi | 358 (28) | 157 (30) | 130 (25) | 71 (30) |
Neighbourhood perceptionsj | ||||
Crime-free, mean (SD) | 2.03 (4.40) | 0.69 (4.59) | 2.94 (3.96) | 2.95 (4.18) |
Quality, mean (SD) | 3.52 (4.53) | 2.41 (4.58) | 4.36 (4.41) | 4.13 (4.20) |
Follow-up participation rates
Of the 1278 adult participants from 1006 households examined at baseline, 877 (69%) adults from 710 (71%) households were examined at follow-up (see Figure 1). A total of 401 adult participants seen at baseline were not followed up.
Social housing sector
Social housing sector participants were followed up between February 2015 and September 2016. Of 520 adult participants from 392 households examined at baseline in the social housing sector, 66% (n = 344) of adult participants from 265 (68%) households were examined at follow-up. Overall, the social housing sector represented 39% (n = 344/877) of the adults seen at follow-up and 37% (n = 265/710) of households.
Intermediate housing sector
Follow-up examinations of the intermediate housing sector took place between July 2015 and September 2017. Among the 524 adults from 421 households examined at baseline, 72% (n = 377) from 312 (74%) households were examined at follow-up. The intermediate sector represented 43% (n = 377/877) of adults and 44% (n = 312/710) of households.
Market-rent housing sector
Market-rent participants were followed up between January 2016 and October 2017. Of 234 adults from 193 households examined at baseline, 67% (n = 156/234) of adults from 133 (69%) households were examined at follow-up. The market-rent sector represented 18% (n = 156/877) of the adults, and 19% (n = 133/710) of households seen at follow-up.
Follow-up rates by East Village and control groups
Half of the adult participants (50%, n = 441/877) had moved to East Village at follow-up and half had not; the participants who had not moved formed the control group (see Figure 1). Of the 441 adult participants who moved to East Village at follow-up, 50% were from the social housing sector, 39% were seeking intermediate accommodation and 11% were seeking market-rent accommodation. Within the control group (n = 436 adults), 28% were social housing participants, 47% were intermediate participants and 25% were market-rent participants. Among the social housing sector, participants in the East Village group had a similar sociodemographic profile to those in the control group. No differences were observed in the following characteristics: age, sex, household composition, living with partner, living with children, education, employment status, socioeconomic status [(National Statistics Socioeconomic Classification (NS-SEC)] and limiting longstanding illness (LLI) (Table 2). However, there were marked differences in ethnicity between East Village and control groups (p < 0.001), with a higher proportion of black ethnic participants in the East Village group than in the control group (55% and 32%, respectively), and a lower proportion of Asian participants in the East Village group than in the control groups (14% and 38%, respectively) in the social housing sector. For intermediate participants, the East Village and control groups were similar in household composition, living with partner, qualifications and NS-SEC, but there were differences in age between East Village and control groups (p < 0.001), sex (p = 0.02), ethnicity (p < 0.001), living with children (p = 0.004), employment status (p = 0.02), and LLI (p = 0.004). The intermediate participants in the East Village group were younger, less likely to be female and living with children, and reported a LLI, and were more likely to be of white ethnicity, employed and in intermediate occupations than the control group (see Table 2). Market-rent participants in the East Village and control groups were similar in their baseline sociodemographic profile, with little difference observed. Differences in baseline physical activity levels, anthropometry, neighbourhood perceptions, mental health and well-being outcomes, and the analytic approach used for respective outcomes, are outlined in Chapters 3–6.
Characteristic | All housing sectors (N = 877) | Housing sector | ||||||||||
---|---|---|---|---|---|---|---|---|---|---|---|---|
Social (N = 344) | Intermediate (N = 377) | Market rent (N = 156) | ||||||||||
East Village (N = 441), n (%) | Control (N = 436), n (%) | p-value | East Village (N = 220), n (%) | Control (N = 124), n (%) | p-value | East Village (N = 174), n (%) | Control (N = 203), n (%) | p-value | East Village (N = 47), n (%) | Control (N = 109), n (%) | p-value | |
Individual characteristics | ||||||||||||
Age group (years) | ||||||||||||
16–24 | 104 (24) | 75 (17) | 47 (21) | 18 (15) | 38 (22) | 30 (15) | 19 (40) | 27 (25) | ||||
25–34 | 194 (44) | 185 (42) | 61 (28) | 32 (26) | 113 (65) | 100 (49) | 20 (43) | 53 (49) | ||||
35–49 | 123 (28) | 138 (32) | 95 (43) | 66 (53) | 22 (13) | 61 (30) | 6 (13) | 11 (10) | ||||
≥ 50 | 20 (5) | 38 (9) | 0.01a | 17 (8) | 8 (6) | 0.27a | 1 (1) | 12 (6) | < 0.001a | 2 (4) | 18 (17) | 0.07a |
Sex | ||||||||||||
Female | 247 (56) | 248 (57) | 0.80a | 158 (72) | 91 (73) | 0.76a | 70 (40) | 107 (53) | 0.02a | 19 (40) | 50 (40) | 0.53a |
Ethnicity | ||||||||||||
White | 212 (48) | 225 (51) | 38 (17) | 25 (20) | 139 (80) | 122 (60) | 35 (74) | 78 (72) | ||||
Asian | 56 (13) | 91 (21) | 31 (14) | 47 (38) | 18 (10) | 35 (17) | 7 (15) | 9 (8) | ||||
Black | 131 (30) | 81 (19) | 120 (55) | 40 (32) | 9 (5) | 32 (16) | 2 (4) | 9 (8) | ||||
Mixed/other | 42 (10) | 39 (9) | < 0.001a | 31 (14) | 12 (10) | < 0.001a | 8 (5) | 14 (7) | < 0.001a | 3 (6) | 13 (12) | 0.42b |
Household characteristics | ||||||||||||
Household composition | ||||||||||||
1 person | 30 (7) | 54 (12) | 14 (6) | 25 (4) | 9 (5) | 17 (8) | 7 (15) | 12 (11) | ||||
2 people | 127 (29) | 174 (40) | 33 (15) | 47 (16) | 76 (44) | 82 (40) | 18 (38) | 45 (41) | ||||
3 people | 94 (21) | 121 (28) | 45 (20) | 40 (13) | 38 (22) | 52 (26) | 11 (23) | 29 (27) | ||||
≥ 4 people | 190 (43) | 87 (20) | 0.20a | 128 (58) | 12 (67) | 0.22a | 51 (29) | 52 (26) | 0.45a | 11 (23) | 23 (21) | 0.88a |
Living with partner | ||||||||||||
Yes | 186 (42) | 210 (48) | 84 (38) | 60 (55) | 82 (47) | 101 (50) | 20 (43) | 49 (45) | ||||
No | 215 (49) | 205 (47) | 103 (47) | 50 (46) | 88 (51) | 96 (47) | 24 (51) | 59 (54) | ||||
Unknown | 40 (9) | 21 (5) | 0.02a | 33 (15) | 14 (13) | 0.17a | 4 (2) | 6 (3) | 0.79b | 3 (6) | 1 (1) | 0.17b |
Living with children | ||||||||||||
Yes | 206 (47) | 172 (39) | 0.03a | 177 (20) | 109 (12) | 0.08a | 24 (14) | 52 (26) | 0.004a | 5 (11) | 11 (10) | 0.92a |
Socioeconomic characteristics | ||||||||||||
Educationc | ||||||||||||
Degree or equivalent/higher | 249 (57) | 287 (66) | 55 (25) | 34 (27) | 154 (89) | 166 (82) | 40 (85) | 87 (80) | ||||
Intermediate qualification | 137 (31) | 102 (23) | 118 (54) | 59 (48) | 15 (9) | 26 (13) | 4 (9) | 17 (16) | ||||
Other/none | 55 (13) | 45 (10) | 0.01a | 47 (21) | 30 (24) | 0.60a | 5 (3) | 11 (5) | 0.18a | 3 (6) | 4 (4) | 0.39b |
Employment statusd | ||||||||||||
Employed | 307 (70) | 347 (80) | 98 (45) | 67 (54) | 169 (97) | 183 (90) | 40 (85) | 97 (89) | ||||
Unemployed | 36 (8) | 22 (5) | 33 (15) | 12 (10) | 1 (1) | 4 (2) | 2 (4) | 6 (6) | ||||
Economically inactive | 97 (22) | 67 (15) | 0.004a | 88 (40) | 45 (36) | 0.18a | 4 (2) | 16 (8) | 0.02b | 5 (11) | 6 (6) | 0.51b |
NS-SECe | ||||||||||||
Higher managerial/professional | 179 (41) | 246 (56) | 23 (24) | 24 (36) | 124 (74) | 146 (81) | 32 (80) | 76 (78) | ||||
Intermediate occupations | 69 (16) | 54 (12) | 27 (28) | 16 (24) | 34 (20) | 22 (12) | 8 (20) | 16 (16) | ||||
Routine/manual | 56 (13) | 44 (10) | 0.003a | 46 (48) | 26 (39) | 0.23a | 10 (10) | 13 (7) | 0.12a | 0 (0) | 5 (5) | 0.43b |
Health characteristics | ||||||||||||
General health status | ||||||||||||
Very good | 134 (30) | 112 (26) | 65 (30) | 27 (22) | 52 (30) | 52 (26) | 17 (36) | 33 (30) | ||||
Good | 234 (53) | 253 (58) | 101 (46) | 61 (49) | 107 (61) | 125 (62) | 26 (55) | 67 (61) | ||||
Fair | 63 (14) | 59 (14) | 45 (20) | 28 (23) | 15 (9) | 24 (12) | 3 (6) | 7 (6) | ||||
Bad | 8 (2) | 12 (3) | 7 (3) | 8 (6) | 0 (–) | 2 (1) | 1 (2) | 2 (2) | ||||
Very bad | 2 (1) | 0 (–) | 0.23a | 2 (1) | 0 (–) | 0.27a | 0 (–) | 0 (–) | 0.35 | 0 (–) | 0 (–) | 0.90 |
LLI | ||||||||||||
Yes | 58 (13) | 59 (14) | 0.87a | 49 (22) | 28 (23) | 0.95a | 6 (3) | 23 (11) | 0.004a | 3 (6) | 8 (7) | 1.00b |
Conclusions
The ENABLE London cohort provides a unique opportunity to examine the impact of a major and focused change in the urban built environment, associated with the rapid repurposed East Village development after the London 2012 Olympic and Paralympic Games, on the physical activity patterns and other health-related behaviours of the local population, particularly those from less privileged backgrounds. It provides an important test of principle that the built environment can alter health behaviours and health outcomes. If this is shown to be the case, the results will inform evidence-based urban planning in the future, and the way in which environmental changes have an impact on health inequalities.
Chapter 3 Physical activity and adiposity: baseline and 2-year follow-up findings from the ENABLE London study
Introduction
The ENABLE London is a longitudinal study evaluating how active urban design influences the health and well-being of people moving into the former London 2012 Olympic and Paralympic Games Athletes’ Village, now known as ‘East Village’. 61 East Village is a purpose-built, mixed-use, high-rise residential development, specifically designed to encourage healthy active living by improving walkability and access to public transport, which consists of a mix of social housing, intermediate (including affordable rent, shared ownership and shared equity) housing, and market-rent housing. 61 This chapter draws on baseline data (prior to any potential move to East Village) to:
-
examine predictors of physical activity and adiposity (measured objectively using accelerometry and bioelectrical impedance), including the housing tenure being sought and participants’ perceptions of their neighbourhood
-
examine whether or not physical activity patterns over 7 days vary by housing sector
-
examine whether or not adjustment for perceptions of the neighbourhood environment reduce housing sector differences in physical activity and adiposity.
Given the longitudinal design, the study also provides a unique opportunity to:
-
examine change in objectively measured physical activity and adiposity after 2 years among those who moved to East Village compared with those who did not, which will add robust evidence to the debate about the effect of the built environment on health.
The study was also able to:
-
objectively quantify the quality of the built environment in which people lived among those followed up at both time points using GIS-derived methods
-
gauge the change in neighbourhood perceptions associated with moving to East Village.
Methods
Participants
Examining Neighbourhood Activities in Built Living Environments in London (ENABLE London) study participants were recruited from those seeking or who had applied for new accommodation in East Village. There were three types of housing tenure being sought based on level of income: social (provided by the local authority or housing association at subsidised rates), intermediate (a mixture of shared ownership, shared equity and affordable rent) and market-rent. The inclusion criteria was broad and included anyone interested/applying for single or multiple occupancy accommodation in East Village. There was no explicit exclusion criteria; adults of any age, gender, ethnic group, with or without handicap, were invited to participate. Current housing status was strongly linked to aspirational housing status; those seeking social accommodation were currently in social housing or on social housing waiting lists, and those seeking intermediate and market-rent accommodation were largely in privately rented housing. Aspirational housing tenure is integral to the design of ENABLE London, and we have shown that this provides a clear socioeconomic marker of study participants. For example, those seeking social housing in East Village are more likely to be unemployed, less educated and more likely to represent ethnic minorities (a classic marker of socioeconomic vulnerability), compared with those seeking affordable and market-rent accommodation (see Chapter 2). 61 We have also shown key differences in mental health and well-being between housing sectors; those seeking social housing were more likely to be depressed, anxious and have poorer well-being than other housing sectors (see Chapter 6). 64 Moreover, this is entirely consistent with earlier studies that found both current housing tenure and aspirational housing tenure are associated with a variety of health outcomes, including mental health and measures of general health. 21,65
Assessments of participants were carried out in their place of residence before any potential move to East Village, and 2 years later (February 2015 to October 2017) for those who had moved to East Village, moved elsewhere or not moved. 66
Measures
Physical activity
Participants wore a hip-mounted ActiGraph GT3X+ accelerometer during waking hours over a period of 7 days. Accelerometers provided daily measures of steps (primary outcome), time spent in moderate or vigorous physical activity and time spent sedentary, using established thresholds [sedentary < 100 counts per minute (CPM), moderate physical activity ≥ 1952 CPM]. 67 Periods of non-wear time were defined as ≥ 60 minutes of zero values, allowing for a 2-minute spike tolerance, to provide daily wear time. We excluded days of accelerometer data where the registered wear time was < 540 minutes. Participants with at least 1 day of data were included in the baseline analysis; those with at least 1 day of data at both baseline and follow-up were retained in the longitudinal analysis. We have previously used at least 1 day of 540 minutes (9 hours) of registered time to represent a satisfactory recording of daily activity in a randomised controlled trial of older but overlapping age groups. 68 The at least 1 day of 540 minutes cut-off point was chosen to lessen attrition bias, and was used in this study to maximise inclusion of hard to reach groups, that is those from social housing, who notoriously comply less and recorded less physical activity data.
Adiposity
The protocol for body size and adiposity measurement was identical at baseline and at follow-up. Height was measured to the last complete millimetre using a portable stadiometer. A Tanita SC-240 Body Composition Analyser was used to measure weight to the nearest kilogram and leg-to-leg bioelectrical impedance, from which fat-free mass and fat mass were estimated. BMI was calculated as weight (kg)/height (m)2 and percentage fat mass was calculated as 100 × fat mass (kg)/weight (kg).
Covariates
A team of trained fieldworkers administered self-completion questionnaires using a laptop during home visits (see Table 1). Age, sex, self-defined ethnicity, work status and occupation, and whether or not the participant had a LLI or disability (lasting or expected to last at least 12 months) were collected via a questionnaire. Participants were defined as ‘white’, ‘Asian’, ‘black’, ‘mixed’ or ‘other’; the last two categories were combined in the analysis. Occupation-based socioeconomic status was coded using the NS-SEC to categorise participants into ‘higher managerial or professional occupations’, ‘intermediate occupations’ and ‘routine or manual occupations’. 63 An additional ‘economically inactive’ category included those seeking employment, those unable to work owing to disability or illness, those who were retired, those looking after home and family, and students. We sought information on educational attainment; participants were categorised into ‘degree or equivalent/higher’, ‘intermediate qualifications’ (including A levels and General Certificates of Secondary Education), and ‘other/none’ (including work-based or foreign qualifications).
Built environment variables
Residential built environment characteristics were derived using GIS data at baseline and at follow-up for those households in the Greater London area. These included network distance from home to the closest park (using data from the London Development Database),69 public transport access,51 and measures of neighbourhood walkability, land use mix, residential density and street connectivity computed within a 1-km street network home-centred buffer (see Chapter 4).
Neighbourhood perceptions
Participants completed questionnaires assessing neighbourhood perceptions; the methods used are outlined further in Chapter 6. 64 In brief, five items assessed perceived crime (e.g. ‘There is a lot of crime in my neighbourhood’; Cronbach’s α = 0.87) and six items assessed neighbourhood quality (e.g. ‘This area is a place I enjoy living in’; Cronbach’s α = 0.78). Responses on items were summed and scores ranged from –10 to +10 for perceived crime and –12 to +12 for perceived quality, such that positive scores indicate less perceived crime and better neighbourhood quality whereas negative scores indicate more perceived crime and poorer quality. The scales were derived following an exploratory factor analysis of 14 questions regarding the neighbourhood (see Report Supplementary Material 1). Two neighbourhood perception scores, measuring crime-free neighbourhood (i.e. vandalism, feeling unsafe to walk in neighbourhood, presence of threatening groups) and neighbourhood quality (i.e. accessible features, attractiveness and enjoyment of living in neighbourhood), were derived at baseline using exploratory factor analysis on 14 neighbourhood perception items in the questionnaire,64 and the same items were used to obtain scores at follow-up.
Statistical analysis
All analyses were carried out using Stata® Special Edition version 15 for Windows® (StataCorp LP, College Station, TX, USA).
To obtain average physical activity variables, we fitted a multilevel linear model for each outcome to allow for repeated measurements of daily physical activity, by fitting participant as a random effect and adjusting for day of the week, day order of recording and month as fixed effects. Raw level one residuals were obtained from the model and a within-person average value of each outcome variable was obtained by averaging these raw residuals. The average of these raw residuals for each participant was added to the sample mean for that particular physical variable to derive an unbiased average level of each physical activity variable at baseline and at follow-up for each person.
Baseline analyses
Physical activity and adiposity outcome variables were inspected for normality; BMI was log-transformed because of its skewed distribution. Multilevel linear regression models were fitted, mutually adjusted for housing sector and participant characteristics (sex, age group, ethnic group and LLI) as fixed effects, with a random effect to allow for household clustering. Age groups (16–24 years, 25–34 years, 35–49 years and ≥ 50 years) were chosen to give a wide spread of ages representative of young adults, young professionals, early middle age and later middle-age/older-age people. Residuals did not show departure from linearity, suggesting that the model assumptions were appropriate. Absolute differences or percentage differences for log-transformed outcomes (i.e. BMI) are presented by sex, age group, ethnic group, LLI and housing sector. Sensitivity analyses examined whether or not associations remained when the sample was restricted to 931 participants (84%) with > 4 days of ≥ 540 minutes per day of recording.
To assess differences in physical activity by day of the week as opposed to overall levels of physical activity, we took the following approach. Daily physical activity data were examined using multilevel models with random effects to allow for multiple days of recording within-person and household clustering. An interaction between housing sector and day of the week was fitted and models were adjusted for sex, age group, ethnic group, LLI, day order of recording and month of measurement as fixed effects.
The associations between neighbourhood perception scales and adiposity and physical activity outcomes at baseline were examined. Each of the neighbourhood quality and crime scores were included in the models as quintiles, to examine the differences in outcomes between the top and bottom quintile. The effect of adjustment for neighbourhood perception on differences in the adiposity and physical activity levels between housing sectors was examined. If associations between outcomes and neighbourhood perceptions appeared linear, models examining housing sector differences were additionally adjusted for neighbourhood perceptions as a continuous variable.
Follow-up analyses
Multilevel linear regression models were fitted to examine the effect of moving to East Village on levels of physical activity and adiposity compared with controls who did not live in East Village (in all cases the distributions of residuals from the models were checked for normality). The primary outcome was specified a priori to be daily steps; secondary outcomes included time spent in MVPA (both total and in ≥ 10 minutes bouts per week), the daily sedentary time, BMI and fat mass percentage. 61 Average daily steps at follow-up was regressed on average daily steps at baseline, adjusting for East Village or control group as a fixed effect and baseline household as a random effect to allow for household clustering. Throughout, an alpha of less than 0.05 (p-value < 0.05) was used to determine the statistical significance of effects. Because of baseline differences in sociodemographic factors, further adjustment for participant characteristics, including sex, age group and ethnic group, were examined. Stratified models by housing tenure examined the effect in the different housing sectors (work status, occupation and child status were not adjusted for as these were strongly related to housing status). We also included an interaction term between East Village/control group and housing sector. Sensitivity analyses were carried out for daily steps, the primary outcome, by:
-
restricting the sample to those with at least 4 days of ≥ 540 minutes’ recording at baseline and at follow-up
-
repeating analyses for weekdays only and weekend days only (as this will modify exposure to the residential built environment)
-
comparing East Village participants with controls who remained at their baseline address at follow-up and controls who had moved elsewhere
-
examining the impact of missing accelerometry data at follow-up using imputation methods.
Results
At baseline
Overall participation rates and participation rates by housing sectors were outlined earlier (see Chapter 2). For the main outcomes of interest, complete data on adiposity were available for 1240 participants (97%); of these, a subset of 1107 participants (89%) met the inclusion criteria for analyses of objectively measured physical activity. Participant characteristics (age, sex, ethnicity) and levels of adiposity were similar among those who did and did not provide physical activity data; however, participants from black and Asian ethnic groups were less likely to provide physical activity data. Report Supplementary Material 2 shows characteristics of participants at baseline for the 1240 adults with measurements of adiposity at baseline, which are similar to those with physical activity data.
Adjusted mean levels of physical activity, and adiposity outcomes by housing sector and participant characteristics, are shown in Report Supplementary Material 3. Table 3 shows the association of housing sector and other participant characteristics with objectively measured physical activity (steps, time spent in MVPA, time spent in MVPA in ≥ 10-minute bouts) and BMI and percentage fat mass. Participants seeking social housing had markedly lower levels of steps, MVPA and MVPA in ≥ 10-minute bouts and markedly higher BMI and percentage fat mass than those seeking intermediate housing, although there were no differences between those seeking market-rent and those seeking intermediate accommodation.
n | Daily stepsa | Daily minutes spent in MVPAa | Daily minutes spent in MVPA in ≥ 10-minute boutsa | BMI (kg/m2)b | Fat mass (%) | |||||||||||
---|---|---|---|---|---|---|---|---|---|---|---|---|---|---|---|---|
Differencec | 95% CI | p-value | Differencec | 95% CI | p-value | Differencec | 95% CI | p-value | Differencec | 95% CI | p-value | Differencec | 95% CI | p-value | ||
Sex | ||||||||||||||||
Male (reference) | 522 | – | – | – | – | – | ||||||||||
Female | 718 | –570 | –946 to –194 | 0.003 | –9.3 | –12.2 to –6.4 | < 0.0001 | –4.1 | –6.1 to –2.0 | < 0.001 | –1.2 | –3.2 to 0.9 | 0.26 | 11.1 | 10.3 to 12.0 | < 0.0001 |
Age group (years) | ||||||||||||||||
16–24 (reference) | 269 | – | – | – | – | – | ||||||||||
25–34 | 531 | 502 | 11 to 992 | 0.04 | 4.0 | 0.2 to 7.9 | 0.04 | 1.0 | –1.9 to 3.8 | 0.51 | 6.3 | 3.5 to 9.1 | < 0.0001 | 3.2 | 2.1 to 4.3 | < 0.0001 |
35–49 | 358 | 699 | 173 to 1224 | 0.01 | 3.9 | –0.2 to 8.0 | 0.07 | –1.1 | –4.0 to 1.8 | 0.46 | 13.4 | 10.2 to 16.6 | < 0.0001 | 6.4 | 5.2 to 7.6 | < 0.0001 |
≥ 50 | 82 | –9 | –832 to 813 | 0.98 | –6.0 | –12.4 to 0.5 | 0.07 | –2.0 | –6.8 to 2.7 | 0.40 | 17.6 | 12.6 to 22.9 | < 0.0001 | 9.2 | 7.3 to 11.0 | < 0.0001 |
Ethnic group | ||||||||||||||||
White (reference) | 595 | – | – | – | – | – | ||||||||||
Black | 314 | –1116 | –1657 to –575 | < 0.0001 | –7.4 | –11.7 to –3.2 | < 0.001 | –6.6 | –9.8 to –3.4 | < 0.0001 | 6.2 | 3.3 to 9.3 | < 0.0001 | 3.6 | 2.4 to 4.8 | < 0.0001 |
Asian | 210 | –1409 | –1972 to –845 | < 0.0001 | –11.5 | –15.9 to –7.0 | < 0.0001 | –8.1 | –11.4 to –4.8 | < 0.0001 | –0.3 | –3.1 to 2.7 | 0.85 | 0.02 | –1.2 to 1.3 | 0.97 |
Other/mixed | 121 | –430 | –1100 to 239 | 0.21 | –4.6 | –9.8 to 0.7 | 0.09 | –4.0 | –7.9 to –0.04 | 0.05 | 1.3 | –2.3 to 5.0 | 0.48 | 1.0 | –0.5 to 2.5 | 0.18 |
LLI | ||||||||||||||||
No (reference) | 1087 | – | – | – | – | – | ||||||||||
Yes | 153 | –1081 | –1666 to –496 | < 0.001 | –5.7 | –10.3 to –1.1 | 0.01 | –2.8 | –6.1 to 0.5 | 0.10 | 4.3 | 1.1 to 7.5 | 0.01 | 1.6 | 0.3 to 2.9 | 0.01 |
Housing sector | ||||||||||||||||
Social | 512 | –1125 | –1629 to –620 | < 0.0001 | –7.5 | –11.5 to –3.6 | < 0.001 | –6.5 | –9.5 to –3.5 | < 0.0001 | 5.0 | 2.2 to 7.8 | < 0.001 | 2.7 | 1.5 to 3.8 | < 0.0001 |
Intermediate (reference) | 503 | – | – | – | – | – | ||||||||||
Market-rent | 225 | –104 | –633 to 424 | 0.70 | 2.3 | –1.9 to 6.4 | 0.29 | 2.8 | –0.3 to 6.0 | 0.08 | –0.8 | –3.6 to 2.0 | 0.57 | –0.2 | –1.4 to 1.0 | 0.70 |
All physical activity measures were lower among females. Steps and MVPA were slightly higher in 25- to 34-year-olds and steps were also higher among 35- to 49-year-olds than among 16- to 24-year-olds; however, there were no age group differences for MVPA in ≥ 10-minute bouts. Participants of black and Asian ethnicities had lower levels of steps, MVPA and MVPA in ≥ 10-minute bouts than people of white ethnicity. Participants who reported having a LLI had lower levels of steps and MVPA, but not MVPA in ≥ 10-minute bouts. Percentage fat mass was higher in females than males, although there was no difference in BMI (see Table 3). BMI and percentage fat mass were higher among all older age groups than among 16- to 24-year-olds. Participants of black ethnicity had higher levels of BMI and percentage fat mass than people of white ethnicity; there were no differences in BMI and percentage fat mass between Asian or other/mixed ethnic groups and people of white ethnicity. Those with a LLI had higher levels of both BMI and percentage fat mass. Educational attainment level was not associated with any of the outcomes once housing sector had been adjusted for, and adjustment for educational attainment did not materially alter housing sector differences in physical activity or other outcomes (data not presented).
Sensitivity analyses for physical activity outcomes were carried out in 931 participants who wore an ActiGraph for ≥ 4 days with ≥ 540 minutes of recording per day at baseline (see Report Supplementary Material 4). There were no differences between market-rent and intermediate groups (consistent with the main analysis presented in Table 3). Differences between social and intermediate groups were broadly similar with the results presented in Table 3 for the main analysis.
Baseline differences in physical activity variables between housing sectors were examined by day of the week to explore whether or not differences between sectors were consistent across the week (Figure 3). Levels of physical activity [steps (Figure 3a), MVPA (Figure 3b) and MVPA in ≥ 10-minute bouts (Figure 3c)] were generally consistent across weekdays (Monday to Friday) among all groups. In the intermediate group, steps were higher on Saturdays and lower on Sundays; MVPA and MVPA in ≥ 10-minute bouts were lower on Sundays, but there was no difference on Saturdays compared with weekday activity. In the market-rent sector, steps, MVPA and MVPA in ≥ 10-minute bouts were higher on Saturdays and similar to weekdays on Sundays. In the social group, steps, MVPA and MVPA in ≥ 10-minute bouts were on average lower on Saturdays and lower still on Sundays. Registered time of recorded physical activity (Figure 3d) was lowest on average in the social group during weekdays, decreasing on Saturdays and Sundays. The intermediate and market-rent groups had higher levels of registered time during weekdays than the social group, which decreased on average on Saturdays and Sundays (despite recording more steps and minutes in MVPA, suggesting a higher intensity of activity). Mean levels of steps, MVPA, and MVPA in ≥ 10-minute bouts on weekdays, and differences on Saturday and Sunday compared with weekdays, are shown by housing sector in Report Supplementary Material 5. The marked differences in activity between weekdays and weekend days in the social group were not explained by differences in registered time.
FIGURE 3.
Daily physical activity by day of the week and housing sector: N = 6206 days from 1107 participants. Means and 95% CIs are adjusted for sex, age group, ethnic group, LLI, month of recording, day order of recording, day of week, housing sector, an interaction between housing sector and day of week and random effects to allow for multiple days of measurement and clustering of participants within households. Reproduced from Nightingale et al. 70 © Author(s) (or their employer(s)) 2018. Re-use permitted under CC BY. Published by BMJ. This is an open access article distributed in accordance with the Creative Commons Attribution 4.0 Unported (CC BY 4.0) license, which permits others to copy, redistribute, remix, transform and build upon this work for any purpose, provided the original work is properly cited, a link to the licence is given, and indication of whether changes were made. See: https://creativecommons.org/licenses/by/4.0/.

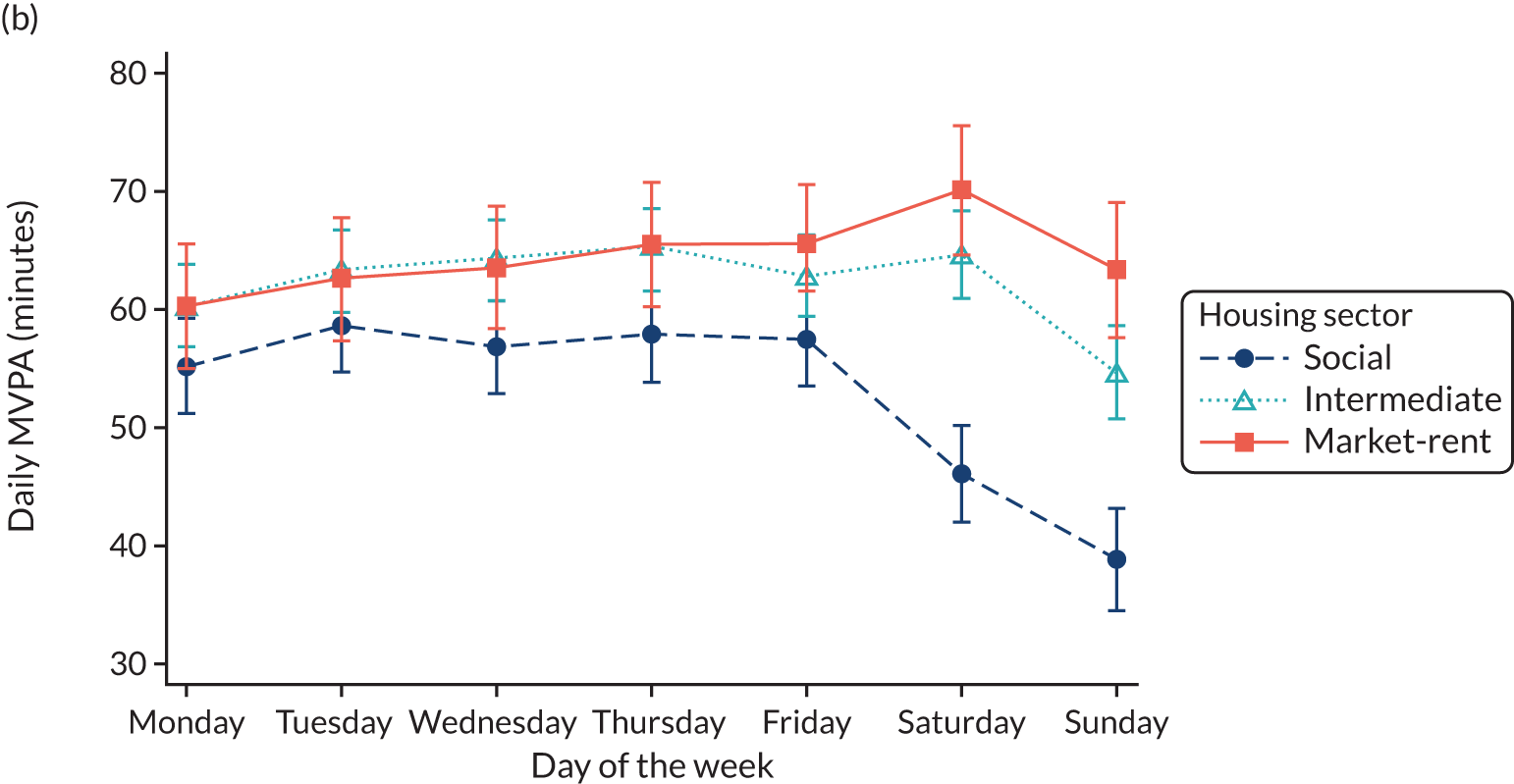

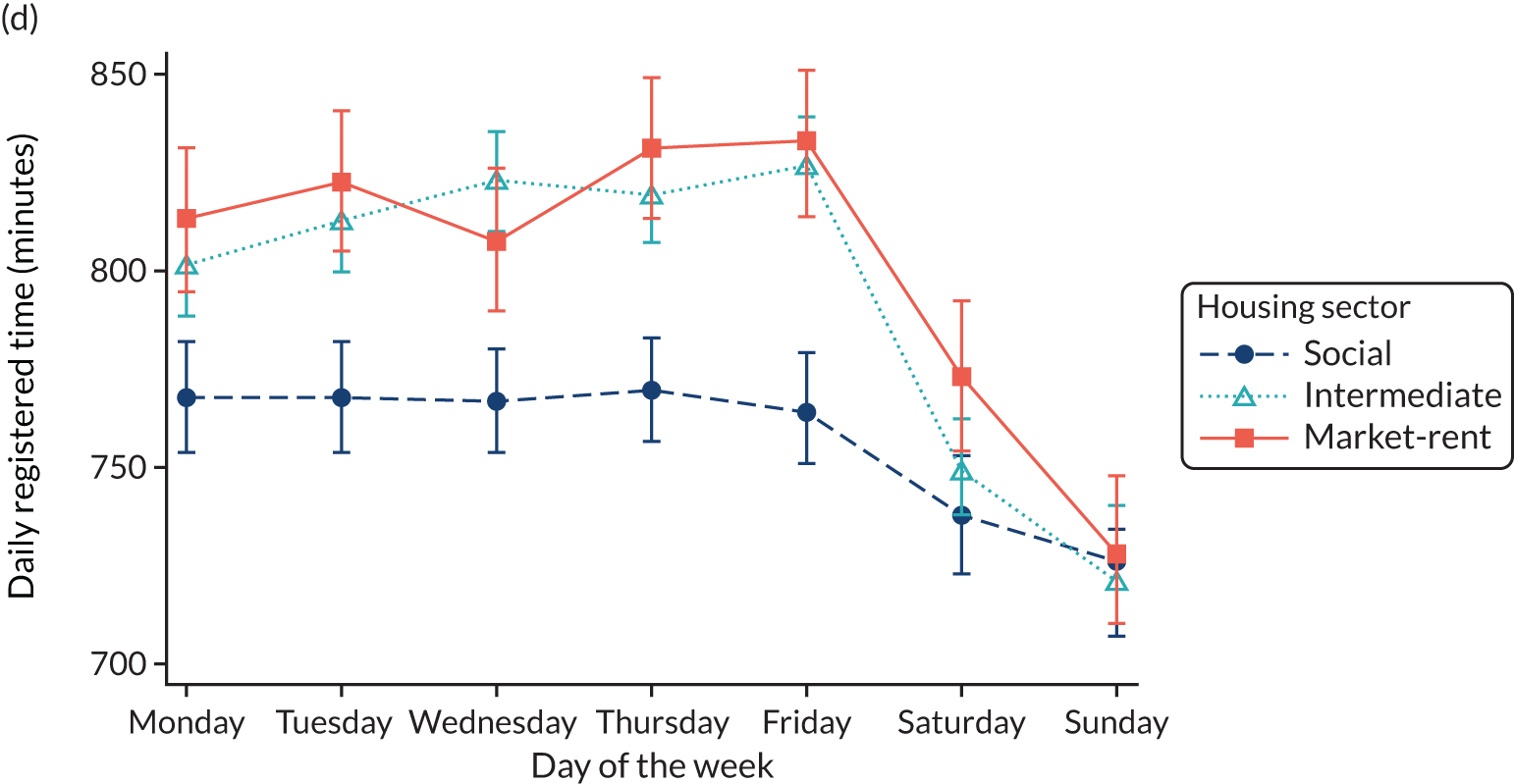
Associations between perceived neighbourhood quality and crime scales, and physical activity and adiposity outcomes are shown in Table 4, adjusted for the participant characteristics (see Table 3). Participants with the most positive perceptions of neighbourhood quality (highest quintile) had higher steps, recorded longer durations of MVPA and had a lower BMI than those who had the most negative perceptions of neighbourhood quality (lowest quintile). There were no significant associations between perceptions of neighbourhood crime and physical activity or adiposity.
Outcome | Perceptions of neighbourhood | |||||
---|---|---|---|---|---|---|
Quality | Crime-free | |||||
Differencea | 95% CI | p-value | Differencea | 95% CI | p-value | |
Physical activity (N = 1107) | ||||||
Daily steps | 677 | 108 to 1247 | 0.02 | –63 | –713 to 587 | 0.85 |
Daily MVPA (minutes) | 4.5 | 0.02 to 9.0 | 0.05 | 1.1 | –4.0 to 6.2 | 0.68 |
Daily MVPA in ≥ 10-minute bouts (minutes) | 2.7 | –0.6 to 6.0 | 0.11 | 2.4 | –1.4 to 6.1 | 0.22 |
Adiposity (N = 1240) | ||||||
Body mass index (kg/m2)b | –3.6 | –6.5 to –0.6 | 0.02 | –2.1 | –5.4 to 1.3 | 0.21 |
Fat mass (%) | –1.2 | –2.5 to 0.06 | 0.06 | –0.8 | –2.2 to 0.7 | 0.30 |
The effect of adjustment for perceived neighbourhood quality on the differences in physical activity and adiposity between housing sector groups is presented in Table 5. All associations between perceived neighbourhood quality and crime-free neighbourhood and outcome variables were approximately linear and were therefore fitted as continuous variables in the model. In addition, associations between perceived neighbourhood quality and crime-free neighbourhood and outcome variables were similar across the three housing sectors (all p > 0.05). Adjustment for perceptions of neighbourhood quality reduced the differences in steps (by 10%), MVPA (by 10%) and MVPA in ≥ 10-minute bouts (by 7%), BMI (by 10%) and percentage fat mass (by 6%) between the social and intermediate groups. Differences between market-rent and intermediate groups in adiposity and physical activity variables were not statistically significant before or after adjustment. Larger proportions of the social–intermediate group differences in steps, MVPA and MVPA in ≥ 10-minute bouts on weekends were explained by adjustment for perceptions of neighbourhood quality (10%, 16% and 16%, respectively) than that for weekdays, which were reduced by 10%, 8% and 3% respectively (data not shown).
Outcome | Housing sector | Model 1a | Model 2b | ||||
---|---|---|---|---|---|---|---|
Differencec | 95% CI | p-value | Differencec | 95% CI | p-value | ||
Physical activity (N = 1107) | |||||||
Daily steps | Social | –1125 | –1629 to –620 | < 0.0001 | –1016 | –1531 to –501 | < 0.001 |
Intermediate | Reference group | ||||||
Market-rent | –104 | –633 to 424 | 0.70 | –96 | –624 to 431 | 0.72 | |
Daily MVPA (minutes) | Social | –7.5 | –11.5 to –3.6 | < 0.001 | –6.8 | –10.8 to –2.7 | 0.001 |
Intermediate | Reference group | ||||||
Market-rent | 2.3 | –1.9 to 6.4 | 0.29 | 2.3 | –1.8 to 6.5 | 0.27 | |
Daily MVPA in ≥ 10-minute bouts (minutes) | Social | –6.5 | –9.5 to –3.5 | < 0.0001 | –6.0 | –9.1 to –3.0 | < 0.001 |
Intermediate | Reference group | ||||||
Market-rent | 2.8 | –0.3 to 6.0 | 0.08 | 2.8 | –0.3 to 6.0 | 0.08 | |
Adiposity (N = 1240) | |||||||
Body mass index (kg/m2)d | Social | 5.0 | 2.2 to 7.8 | < 0.001 | 4.5 | 1.7 to 7.3 | 0.002 |
Intermediate | Reference group | ||||||
Market-rent | –0.8 | –3.6 to 2.0 | 0.57 | –0.9 | –3.6 to 2.0 | 0.55 | |
Fat mass (%) | Social | 2.7 | 1.5 to 3.8 | < 0.0001 | 2.5 | 1.4 to 3.6 | < 0.0001 |
Intermediate | Reference group | ||||||
Market-rent | –0.2 | –1.4 to 1.0 | 0.70 | –0.2 | –1.4 to 0.9 | 0.68 |
At follow-up
In total, 877 adults (69%) from 710 households were examined after 2 years; 441 (50%) had moved and were living in East Village at follow-up. Data were available at baseline and follow-up for 762 participants for physical activity outcomes and 822 participants for adiposity variables. There were no differences in age, sex or ethnic group between those followed up and not followed up (see Report Supplementary Material 6), although those followed up had slightly higher socioeconomic status and recorded more sedentary time at baseline than those not followed up.
Baseline characteristics for the 877 adults who were seen at follow-up are shown in Table 6, by housing sector and overall. In the social housing sector, age, sex, socioeconomic status and children in the household were similar for those living in East Village and for controls, although the East Village group had a higher proportion of participants of black African/Caribbean ethnic origin and a lower proportion of participants of Asian ethnic origin than the control group. In the intermediate sector, the East Village group were younger, less likely to be female, and more likely to be of white ethnicity, be economically active and have no children in the household than controls. In the market-rent sector, age, sex, ethnicity, socioeconomic status and children in the household were similar in the East Village and control groups. For housing sectors combined, the proportion of females in the two groups was similar, but participants in the East Village group were younger, more likely to be of black African–Caribbean ethnicity, less likely to be of Asian ethnicity and less likely to be in higher managerial/professional occupations than the control group. Overall, baseline daily steps and daily levels of MVPA were greater in the control group than in the East Village group, although differences by housing sector were less apparent. Although there was no overall difference in baseline adiposity levels, controls in the intermediate group had higher levels of BMI and fat mass percentage than those living in intermediate East Village accommodation. Those who had moved to East Village reported a sizable increase in scores for the perceptions of crime-free neighbourhood and higher quality neighbourhood at follow-up (Table 7 and Report Supplementary Material 7). Compared with baseline data, participants living in East Village at follow-up lived closer to their nearest park, had better access to public transport and lived in a more walkable area (see Table 7 and Report Supplementary Material 7).
Characteristic | All housing sectors (N = 877) | Housing sector | ||||||||||
---|---|---|---|---|---|---|---|---|---|---|---|---|
Social (N = 344) | Intermediate (N = 377) | Market-rent (N = 156) | ||||||||||
Control (N = 436) | East Village (N = 441) | p-valuea | Control (N = 124) | East Village (N = 220) | p-valuea | Control (N = 203) | East Village (N = 174) | p-valuea | Control (N = 109) | East Village (N = 47) | p-valuea | |
Age (years), n (%) | ||||||||||||
16–24 | 75 (17) | 104 (24) | 0.01 | 18 (15) | 47 (21) | 0.27 | 30 (15) | 38 (22) | < 0.001 | 27 (25) | 19 (40) | 0.07 |
25–34 | 185 (42) | 194 (44) | 32 (26) | 61 (28) | 100 (49) | 113 (65) | 53 (49) | 20 (43) | ||||
35–49 | 138 (32) | 123 (28) | 66 (53) | 95 (43) | 61 (30) | 22 (13) | 11 (10) | 6 (13) | ||||
≥ 50 | 38 (9) | 20 (5) | 8 (6) | 17 (8) | 12 (6) | 1 (1) | 18 (17) | 2 (4) | ||||
Sex, n (%) | ||||||||||||
Female | 248 (57) | 247 (56) | 0.79 | 91 (73) | 158 (72) | 0.75 | 107 (53) | 70 (40) | 0.02 | 50 (46) | 19 (40) | 0.53 |
Ethnic group, n (%) | ||||||||||||
White | 225 (52) | 212 (48) | < 0.001 | 25 (20) | 38 (17) | < 0.001 | 122 (60) | 139 (80) | < 0.001 | 78 (72) | 35 (74) | 0.42 |
Black | 81 (19) | 131 (30) | 40 (32) | 120 (55) | 32 (16) | 9 (5) | 9 (8) | 2 (4) | ||||
Asian | 91 (21) | 56 (13) | 47 (38) | 31 (14) | 35 (17) | 18 (10) | 9 (8) | 7 (15) | ||||
Other | 39 (9) | 42 (10) | 12 (10) | 31 (14) | 14 (7) | 8 (5) | 13 (12) | 3 (6) | ||||
NS-SEC, n (%) | ||||||||||||
Higher managerial/professional | 246 (57) | 179 (41) | < 0.001 | 24 (20) | 23 (11) | 0.12 | 146 (73) | 124 (72) | 0.01 | 76 (70) | 32 (68) | 0.53 |
Intermediate | 54 (12) | 69 (16) | 16 (13) | 27 (12) | 22 (11) | 34 (20) | 16 (15) | 8 (17) | ||||
Routine/manual | 44 (10) | 56 (13) | 26 (21) | 46 (21) | 13 (6) | 10 (6) | 5 (5) | 0 (0) | ||||
Economically inactive | 89 (21) | 133 (30) | 57 (46) | 121 (56) | 20 (10) | 5 (3) | 12 (11) | 7 (15) | ||||
Children in household, n (%) | ||||||||||||
Yes | 172 (39) | 203 (46) | 0.05 | 109 (88) | 178 (81) | 0.09 | 52 (26) | 21 (12) | < 0.001 | 11 (10) | 4 (9) | 1.00 |
Physical activity,b mean (SD) | ||||||||||||
Total, n | 405 | 403 | 112 | 199 | 189 | 164 | 104 | 40 | ||||
Daily steps | 9192 (3284) | 8644 (3104) | 0.01 | 7707 (3069) | 7730 (3345) | 0.95 | 9639 (3224) | 9592 (2584) | 0.88 | 9980 (3128) | 9304 (2468) | 0.22 |
Daily MVPA (minutes) | 61 (26) | 58 (25) | 0.06 | 50 (25) | 50 (25) | 0.97 | 63 (24) | 66 (22) | 0.31 | 70 (26) | 65 (21) | 0.32 |
Daily MVPA in ≥ 10-minute bouts (minutes) | 22 (20) | 19 (18) | 0.02 | 12 (14) | 12 (13) | 0.85 | 23 (19) | 25 (19) | 0.45 | 30 (21) | 28 (21) | 0.70 |
Daily sedentary time (minutes) | 588 (81) | 583 (84) | 0.36 | 544 (84) | 545 (83) | 0.91 | 598 (75) | 617 (68) | 0.01 | 616 (67) | 627 (74) | 0.42 |
Adiposity, mean (SD) | ||||||||||||
Total, n | 428 | 435 | 124 | 218 | 199 | 172 | 105 | 45 | ||||
BMI (kg/m2)c | 26 (5) | 26 (6) | 0.23 | 27 (5) | 28 (7) | 0.10 | 26 (5) | 24 (4) | 0.01 | 24 (4) | 23 (3) | 0.07 |
Fat mass per centd | 27 (10) | 27 (11) | 0.56 | 31 (10) | 32 (11) | 0.28 | 26 (9) | 22 (8) | < 0.001 | 23 (9) | 21 (8) | 0.20 |
Perceptions/characteristics | All housing sectors (N = 436) | Housing sector | ||||||||||
---|---|---|---|---|---|---|---|---|---|---|---|---|
Social (N = 124) | Intermediate (N = 203) | Market-rent (N = 109) | ||||||||||
Mean | 95% CI | p-value | Mean | 95% CI | p-value | Mean | 95% CI | p-value | Mean | 95% CI | p-value | |
Neighbourhood perception scoresa | ||||||||||||
Control group | ||||||||||||
Participants, n | 436 | 124 | 203 | 109 | ||||||||
Crime score | 0.6 | 0.2 to 1.0 | 0.004 | 1.2 | 0.4 to 2.1 | 0.004 | 0.1 | –0.5 to 0.7 | 0.81 | 0.9 | 0.2 to 1.7 | 0.02 |
Quality score | 0.7 | 0.3 to 1.2 | < 0.001 | 1.3 | 0.5 to 2.0 | 0.001 | 0.5 | –0.2 to 1.1 | 0.14 | 0.6 | –0.2 to 1.4 | 0.15 |
East Village group | ||||||||||||
Participants, n | 441 | 220 | 174 | 47 | ||||||||
Crime score | 4.6 | 4.1 to 5.1 | < 0.001 | 5.6 | 4.9 to 6.3 | < 0.001 | 3.8 | 3.2 to 4.4 | < 0.001 | 2.7 | 1.2 to 4.3 | < 0.001 |
Quality score | 6.8 | 6.4 to 7.3 | < 0.001 | 7.1 | 6.4 to 7.8 | < 0.001 | 6.5 | 5.8 to 7.2 | < 0.001 | 6.8 | 5.8 to 7.8 | < 0.001 |
Built environment characteristics | ||||||||||||
Control group | ||||||||||||
Participants, n | 376 | 120 | 178 | 78 | ||||||||
Distance to closest park (m)b | 6 | –37 to 49 | 0.79 | –16 | –72 to 39 | 0.56 | 10 | –58 to 78 | 0.77 | 31 | –83 to 144 | 0.59 |
Access to public transport (PTAL)c | –0.2 | –0.3 to 0.0 | 0.07 | –0.2 | –0.5 to 0.0 | 0.07 | –0.1 | –0.4 to 0.2 | 0.411 | –0.2 | –0.6 to 0.2 | 0.45 |
Walkabilityd | 0.3 | 0.1 to 0.5 | 0.01 | –0.2 | –0.5 to 0.2 | 0.34 | 0.6 | 0.2 to 1.0 | 0.005 | 0.4 | –0.1 to 0.9 | 0.09 |
Land use mixe | 0.02 | 0.00 to 0.04 | 0.05 | –0.03 | –0.05 to 0.00 | 0.04 | 0.04 | 0.01 to 0.07 | 0.005 | 0.03 | –0.01 to 0.06 | 0.13 |
Residential densityf | 1.9 | 1.2 to 2.6 | < 0.001 | 1.4 | 0.7 to 2.1 | < 0.001 | 2.4 | 1.2 to 3.5 | < 0.001 | 1.6 | 0.05 to 3.2 | 0.04 |
Street connectivityg | 0.0 | –0.1 to 0.1 | 0.89 | –0.1 | –0.3 to 0.1 | 0.17 | 0.1 | –0.1 to 0.2 | 0.42 | 0.0 | –0.3 to 0.2 | 0.89 |
East Village group | ||||||||||||
Participants, n | 414 | 216 | 160 | 38 | ||||||||
Distance to closest park (m)b | –525 | –565 to –485 | < 0.001 | –477 | –527 to –427 | < 0.001 | –570 | –633 to –506 | < 0.001 | –614 | –812 to –416 | < 0.001 |
Access to public transport (PTAL)c | 1.6 | 1.4 to 1.9 | < 0.001 | 2.5 | 2.1 to 2.8 | < 0.001 | 0.8 | 0.4 to 1.3 | < 0.001 | 0.2 | –0.7 to 1.0 | 0.66 |
Walkabilityd | 2.5 | 2.2 to 2.7 | < 0.001 | 2.8 | 2.5 to 3.0 | < 0.001 | 2.2 | 1.7 to 2.6 | < 0.001 | 2.1 | 0.6 to 3.7 | 0.01 |
Land use mixe | 0.38 | 0.36 to 0.40 | < 0.001 | 0.39 | 0.37 to 0.41 | < 0.001 | 0.38 | 0.35 to 0.41 | < 0.001 | 0.30 | 0.20 to 0.39 | < 0.001 |
Residential densityf | 13.2 | 12.0 to 14.4 | < 0.001 | 12.9 | 11.4 to 14.4 | < 0.001 | 12.6 | 10.6 to 14.6 | < 0.001 | 17.4 | 12.1 to 22.8 | < 0.001 |
Street connectivityg | –0.9 | –1.1 to –0.8 | < 0.001 | –0.8 | –0.9 to –0.6 | < 0.001 | –1.1 | –1.3 to –0.9 | < 0.001 | –1.1 | –1.7 to –0.5 | < 0.001 |
The effect of moving to East Village on physical activity (daily steps, daily minutes of MVPA, daily sedentary time) and adiposity (BMI and percentage fat mass) is shown in Table 8, both overall and stratified by housing sector. Overall, there was weak evidence that moving to East Village was associated with a small increase in daily steps of 154 [95% confidence interval (CI) –231 to 539] after adjusting for sex, age group, ethnic group and housing sector. This appeared more pronounced in the intermediate sector (433 steps, 95% CI –175 to 1042 steps). There was also weak evidence of an increase in daily minutes of MVPA overall, both in total MVPA and in ≥ 10-minute bouts, in participants in the intermediate and market-rent sectors, and a decrease in daily minutes in participants in the social sector, although none of the effects were statistically significant. There was no evidence of a change in daily sedentary time, BMI or percentage fat mass, both overall and by housing sector (see Table 8). Inclusion of an interaction term between the East Village/control group and housing sector to allow for potential differential effects was not statistically significant (p > 0.1). Restricting analyses to 652 (86%) participants who recorded at least 4 days of ≥ 540 minutes accelerometry wear at baseline and at follow-up gave a larger effect for moving to East Village on daily steps (324 steps, 95% CI –93 to 741 steps; see Report Supplementary Material 8), but CIs still spanned the null value. There was weak evidence that the changes were larger at weekends (428 steps, 95% CI –288 to 1144 steps) than on weekdays (199 steps, 95% CI –223 to 620 steps), but CIs included the null value.
All housing sectors | Housing sector | |||||||||||
---|---|---|---|---|---|---|---|---|---|---|---|---|
Social | Intermediate | Market-rent | ||||||||||
Difference | 95% CI | p-value | Difference | 95% CI | p-value | Difference | 95% CI | p-value | Difference | 95% CI | p-value | |
Physical activity outcomes | (n = 762) | (n = 290) | (n = 335) | (n = 137) | ||||||||
Daily steps | ||||||||||||
Model 1a | 192 | –173 to 557 | 0.30 | –129 | –728 to 469 | 0.67 | 500 | –63 to 1063 | 0.08 | 160 | –784 to 1105 | 0.74 |
Model 2b | 235 | –136 to 605 | 0.21 | –187 | –803 to 429 | 0.55 | 433 | –175 to 1042 | 0.16 | 225 | –730 to 1181 | 0.64 |
Model 3c | 154 | –231 to 539 | 0.43 | |||||||||
Total daily MVPA (minutes) | ||||||||||||
Model 1a | 0.5 | –2.4 to 3.4 | 0.73 | –1.7 | –6.2 to 2.8 | 0.45 | 2.9 | –1.4 to 7.3 | 0.19 | 1.1 | –6.9 to 9.2 | 0.78 |
Model 2b | 0.6 | –2.3 to 3.5 | 0.67 | –2.8 | –7.4 to 1.9 | 0.24 | 1.7 | –3.0 to 6.3 | 0.49 | 1.9 | –6.3 to 10.0 | 0.65 |
Model 3c | 0.2 | –2.9 to 3.2 | 0.91 | |||||||||
Daily MVPA in ≥ 10-minute bouts (minutes) | ||||||||||||
Model 1a | 0.5 | –1.8 to 2.7 | 0.67 | –0.9 | –3.8 to 2.0 | 0.55 | 3.1 | –0.5 to 6.8 | 0.09 | 2.5 | –4.0 to 9.0 | 0.45 |
Model 2b | 0.6 | –1.7 to 2.8 | 0.62 | –1.2 | –4.2 to 1.9 | 0.45 | 1.6 | –2.3 to 5.4 | 0.43 | 3.1 | –3.5 to 9.6 | 0.36 |
Model 3c | 0.8 | –1.5 to 3.1 | 0.48 | |||||||||
Daily sedentary time (minutes) | ||||||||||||
Model 1a | –8 | –18 to 2 | 0.12 | –8 | –28 to 11 | 0.39 | –2 | –17 to 12 | 0.77 | 3 | –19 to 25 | 0.78 |
Model 2b | –8 | –18 to 2 | 0.12 | –13 | –33 to 7 | 0.20 | –4 | –19 to 12 | 0.64 | 7 | –15 to 29 | 0.54 |
Model 3c | –4 | –15 to 7 | 0.45 | |||||||||
Adiposity outcomes | (n = 822) | (n = 327) | (n = 358) | (n = 137) | ||||||||
BMI (kg/m2) | ||||||||||||
Model 1a | 0.3 | 0.0 to 0.5 | 0.06 | 0.4 | –0.2 to 1.0 | 0.16 | 0.1 | –0.2 to 0.5 | 0.54 | 0.2 | –0.4 to 0.8 | 0.52 |
Model 2b | 0.2 | –0.1 to 0.5 | 0.14 | 0.2 | –0.4 to 0.8 | 0.49 | 0.1 | –0.3 to 0.5 | 0.66 | 0.2 | –0.4 to 0.8 | 0.52 |
Model 3c | 0.2 | –0.1 to 0.5 | 0.25 | |||||||||
Percentage fat massd | ||||||||||||
Model 1a | 0.1 | –0.4 to 0.7 | 0.62 | –0.1 | –1.1 to 0.8 | 0.78 | 0.1 | –0.6 to 0.8 | 0.81 | 0.3 | –1.0 to 1.7 | 0.62 |
Model 2b | 0.1 | –0.4 to 0.7 | 0.58 | –0.3 | –1.3 to 0.7 | 0.60 | 0.2 | –0.6 to 0.9 | 0.65 | 0.4 | –1.0 to 1.8 | 0.58 |
Model 3c | 0.1 | –0.5 to 0.6 | 0.80 |
Separate analyses comparing change in daily steps in East Village participants with (1) controls who remained at their baseline address and (2) controls who moved elsewhere, allowed the effect of any move to be examined (see Report Supplementary Material 8). Although none of the differences were statistically significant, there was a suggestion of larger differences in daily steps when those in East Village were compared with those who had moved elsewhere [particularly in the intermediate housing sector where a difference of 677 steps (95% CI 15 to 1339 steps) was observed], with a smaller difference when compared with those who did not move. It is noteworthy that daily steps were marginally lower among those in the social sector who moved to East Village than those who moved elsewhere, but effect sizes were not statistically significant. There was no evidence of a difference after removing 21 women who reported being pregnant at baseline or follow-up. The missing data imputation analyses (adding a further 46 individuals at follow-up) gave similar results to the complete case analysis (see Report Supplementary Material 9).
Discussion
Baseline findings
The baseline findings from this study showed that, in the social housing sector, levels of physical activity were particularly low at weekends compared with weekdays, possibly reflecting higher occupational physical activity and lower leisure-time physical activity; weekday–weekend differences in physical activity were less marked among those seeking intermediate and market-rent housing. However, the lower registered time at weekends but higher MVPA and steps suggests more intense activity at weekends in the intermediate and market-rent housing sectors. Participants seeking social housing in East Village also had lower levels of physical activity and higher levels of BMI and percentage fat mass than those seeking intermediate and market-rent housing, even when adjusted for demographic factors. 70 Positive associations between perceived neighbourhood quality and physical activity, BMI and percentage fat mass at baseline were also shown. Adjustment for differences in perceived neighbourhood quality reduced the differences in physical activity and BMI by approximately 10% between social and intermediate housing sectors, equivalent to a reduction of 111 daily steps, 0.5 minutes of MVPA and 0.5 kg/m2 BMI. However, a larger proportion of the difference in physical activity was apparent at weekends; equivalent to a reduction of 222 daily steps and 2.2 minutes of MVPA. These baseline findings provide further insight into the physical activity patterns of the ENABLE London cohort, and how moving to East Village might affect physical activity and adiposity levels in different socioeconomic groups.
Relation of baseline findings to previous studies
Previous studies have shown that lower socioeconomic status is associated with lower levels of physical activity,71,72 and that those from more socially deprived backgrounds have the most barriers to being physically active. 8 Previous research examining the role of housing tenure is limited. Findings from this study showed marked differences in physical activity and adiposity between those seeking social, intermediate and market-rent housing. In particular, lower physical activity and higher adiposity were observed in participants seeking social housing, a group that comprises a high proportion of people from more socioeconomically disadvantaged backgrounds. 61 The higher levels of BMI and percentage fat mass in those seeking social housing than in those seeking intermediate or market-rent housing is consistent with systematic reviews that have found an association between lower socioeconomic status and higher levels of adiposity, particularly in higher income countries and among women. 73 Although socioeconomic status is a strong determinant of housing status, to our knowledge this is the first study to explicitly examine housing sector differences in objectively measured physical activity and markers of adiposity levels (i.e. BMI and percentage fat mass). However, it is important to appreciate more broadly what these aspirational housing sector differences might represent before considering what change in the residential built environment might do. Related studies have shown that those in social housing are less likely to use active travel than owner occupiers,19 and that those in social housing and home owners with a mortgage are more likely to be obese and have higher levels of illness and disability than outright home owners, even after adjustment for other socioeconomic status markers. 74 These findings suggest that the effect of home ownership may be more complex and cannot be simply explained by socioeconomic status. Neighbourhood quality may offer a partial explanation for these findings. 75 Baseline findings from this study showed that perceptions of better neighbourhood quality were associated with physical activity whereas perceptions of crime were not. In contrast, a large UK-based study found that perceptions of feeling safe in the neighbourhood had the largest effect on levels of physical activity compared with perceptions of leisure facilities, a sense of belonging or access to public transport or amenities. 76 Another study in the USA found that low perceived safety from crime was associated with lower levels of MVPA. 77 However, a recent review concluded that higher-quality evidence is needed, including from prospective studies and natural experiments in areas of wide crime variability, to further understand the effect of crime on physical and mental health. 28 Moreover, previous work has suggested that objective and perceived measures of the built environment correlate differently with physical activity levels, suggesting that these measures are assessing different dimensions of the built environment, which relate differently to health behaviour. 78
The baseline findings showed that physical activity levels were particularly low at the weekend among those seeking social housing, which is consistent with findings from a systematic review which found that leisure-time physical activity (which may be more likely to take place at weekends) was lower among those from lower socioeconomic groups. 9 This suggests that low-cost strategies to increase weekend physical activity may be particularly beneficial to more disadvantaged households. A free community-based programme in Bogotá, Colombia, temporarily closed streets on Sundays to encourage physical activity among more disadvantaged local residents. 79 A similar programme has been trialled in the USA;80 however, the effectiveness, longevity and generalisability of these programmes to other socioeconomically deprived areas is yet to be established.
The baseline findings from the ENABLE London cohort suggest that perceived neighbourhood quality is associated with meaningful differences in physical activity and markers of adiposity. Differences in steps (680 steps) and BMI (3.6 kg/m2) between the lowest and highest quintiles of perceived neighbourhood quality should be considered in the context of an average of 10,000 steps per day, where a 5% increase (500 steps) would be a worthwhile population-level increase and a 5 kg/m2 increase in BMI would be associated with a 31% increase in all-cause mortality. 81 Hence, improvements in neighbourhood quality could be associated with health benefits that are of public health importance. There were also substantial differences in physical activity, BMI and percentage fat mass between the three housing sectors studied. In particular, the very low levels of physical activity in the social housing sector during the weekend could provide a target for intervention to increase levels of physical activity; again, these differences should be considered in relation to 500 steps per day, which can be considered as an increase of population importance. Perceptions of neighbourhood quality reduced baseline differences in physical activity and adiposity between housing sector groups, and the measurement of more objective markers of neighbourhood quality has the potential to explain more. 78
Follow-up findings
Compared with pre-move residential areas, East Village was assessed as a more walkable place with greater access to public transport and closer proximity to parks, and was perceived as having marked improvements in neighbourhood quality. Despite this, at 2-year follow-up there was weak evidence that moving to East Village was associated with an overall increase in daily steps compared with not moving. This increase was the largest in those who moved into intermediate housing, whereas those in social housing showed a small decline in their physical activity. Overall increases in time spent in MVPA daily and decreases in daily sedentary behaviour were also observed, but again effects were small. There were no changes in markers of adiposity associated with moving to East Village, either overall or by housing tenure.
Change in the built environment
A key issue is whether or not the change in the built environment, in this case East Village, ‘the intervention’, is sufficient and rapid enough to observe changes in health behaviour,33,82 and whether or not the correct components of the built environment to maximise physical activity were present. 41,83 East Village includes a multitude of features that could plausibly have a beneficial effect on the physical activity patterns of occupants. 61 These include more equitable access to improved public transport and active travel opportunities. 42,43 East Village is also situated close to leisure-time physical activity-permissive features, including parkland, street furniture and pedestrianised areas, as well as major sporting facilities. It is also situated within walking distance of retail outlets. 42,43 Hence, collectively, East Village has a multitude of activity-permissive features, which could plausibly increase physical activity levels by encouraging time spent outdoors. 84,85 Differences in the built environment of East Village were evident by the substantial changes in objective GIS measures of the built environment (including increased walkability, access to public transport and parks) and the sizeable improvements in neighbourhood perceptions observed among residents, especially those from the social housing sector. This raises the question of why larger improvements in physical activity were not observed.
Relation of follow-up findings to previous studies
Previous research has shown cross-sectional associations between attributes of the built environment and physical activity,32,86,87 whereas prospective studies from fewer housing relocation or neighbourhood change studies have shown more modest effects. 33 To our knowledge, the ENABLE London study is the first longitudinal study to use objective measures of physical activity in a cohort relocating to a new neighbourhood designed for healthy active living, rather than relying solely on less reliable self-report. 88 Steps were chosen a priori as the primary outcome given the focus of examining the effect of changing to active design principles, and hence walkability of the built environment. 61 We are not aware of any other longitudinal studies with a directly comparable outcome. Despite this, some broad comparisons with other longitudinal studies are possible. Although increases in active modes of travel have been observed in these studies (particularly in reported cycling and use of public transport),89–91 change in overall self-reported walking and physical activity levels have been less apparent. 82,92,93 However, change in physical activity may be more nuanced. The RESIDential Environment (RESIDE) study recruited 1800 people who moved into new homes in Perth, Australia, and, although there were no overall differences in self-reported physical activity, increases in recreational walking and opposing decreases in transport-related walking were evident. 94 These differences were attributed to an increase in access to recreational facilities (e.g. parks, sports fields and beaches) and a decrease in access to transport (within a 15-minute walk), respectively. 95
Use of the residential built environment may differ throughout the week. We observed appreciable differences in weekday versus weekend physical activity across housing sectors within this cohort at baseline. 70 Although prospective findings from this study also indicated increased steps at weekends compared with weekdays was associated with moving to East Village (i.e. 428 vs. 199 steps), these differences were both consistent with no effect (i.e. CIs included the null value of zero steps).
Conclusions
Teasing out the causal relationship between the built environment and health behaviours is complex,96 and the reasons for failing to observe clear effects in this longitudinal study when cross-sectional findings are less equivocal remain unclear. 33 Some systematic reviews have avoided this issue by simply excluding longitudinal or experimental studies, which raise concerns that such reviews lead to greater certainty about biased findings,97–100 especially when those living in better neighbourhoods, with built environments more conducive to physical activity, may be fundamentally different from those who do not. However, it is notable that longitudinal studies may also be prone to selection bias, whereby those who choose to move to better neighbourhoods have different health behaviours from those who choose to remain. The ENABLE London study sought to minimise these effects by recruiting a cohort that was seeking to move before any move to East Village, where half of whom moved to East Village and the other half of whom did not. Further consideration of the strengths and limitations of the ENABLE London study and the potential implications of the findings are considered in Chapter 9.
Chapter 4 Residential built environment and physical activity: cross-sectional and longitudinal analyses
Introduction
In this chapter we focus on exploring whether or not there are associations between objectively measured features of the built environment and physical activity at baseline and whether or not changes in the built environment are associated with changes in physical activity.
Physical activity is a protective factor for a wide range of physical and psychological disorders. 1,6,101 The current population levels of physical activity are too low both in the UK and globally,102 and individuals from more disadvantaged socioeconomic groups are less physically active, in terms of both daily steps70 and MVPA. 17,103 In recent years, epidemiological research has increasingly incorporated socioecological models that acknowledge the role of the built environment in determining physical activity behaviours. 104 Researchers have hypothesised that a deprivation amplification effect exists,105 whereby disadvantaged individuals have greater exposure to less health-promoting neighbourhood environments. 106–109 Policies aimed at modifying built environment factors have been promoted as potential levers for reducing socioeconomic inequalities in health behaviours, including physical activity. However, the extent to which differences in residential built environment factors contribute to individual socioeconomic inequalities in physical activity remains uncertain.
In the UK, environmental factors that have been found to be positively associated with higher physical activity levels include greater neighbourhood walkability,110 improved accessibility to green space32,111 and improved accessibility to public transport. 32 However, these findings rely on a limited number of studies. This evidence is also mixed, with, for instance, both positive32,111 and null112 associations found between accessibility to green space and physical activity. More work is needed to establish whether or not specific features of the residential built environment shape physical activity behaviours in the UK, and whether or not differences in the accessibility of these features contribute to socioeconomic differences in physical activity.
Associations between residential physical activity facilities and physical activity have been shown to vary by time of the day and day of the week. 113 Daily variations in the number of steps and minutes of MVPA have been reported, with more physical activity on weekdays than at weekends. 70,114,115 Time use studies show that week and weekend days markedly differ in terms of human activity, with the former focused more on work and the latter more on leisure and recreational activities. 116 Use of the residential built environment may, therefore, be expected to be different at weekdays and weekends. This suggests that we should take into account weekday and weekend variations in physical activity when seeking to better understand the contribution of the residential built environment to physical activity. 117
Many of these studies rely on cross-sectional designs and are therefore prone to biases, such as residential self-selection. 118 As environments can change in response to residents’ preferences and residents may choose to live in locations consistent with their preferred lifestyles, cross-sectional designs limit our ability to make causal inferences. Longitudinal studies that examine associations between time-varying features of the residential built environment and physical activity are needed to strengthen evidence for a causal effect. Natural experiments of changes to the built environment could help generate better ‘causal’ evidence that environmental factors are important for physical activity. 119
Aims
In this chapter we assess whether or not:
-
the residential built environment is associated with the number of daily steps walked and the total daily minutes of MVPA accumulated on weekdays and at the weekend
-
associations between the residential built environment and physical activity levels are modified by socioeconomic status
-
the distribution of residential built environment factors differs by socioeconomic status, and, if so, whether or not this contributes to socioeconomic differences in the number of daily steps and the time in MVPA on weekdays and at the weekend
-
changes in exposure to features of the residential built environment can predict changes in physical activity levels among adult participants and whether or not these effects differ by socioeconomic status.
Methods
In this chapter we use baseline and follow-up measurements of adult physical activity and sociodemographic data before and after relocation into East Village.
Baseline data
Of the 1278 study participants, we excluded those who lived outside Greater London (n = 81) and those without sufficient accelerometer data (at least 9 hours for at least 1 day is sufficient) on either weekdays or weekends (n = 144) (some participants fit multiple exclusion categories). Those who were excluded from the analytical sample had similar characteristics to those included in the sample in terms of housing status (p = 0.17), age (p = 0.27), sex (p = 0.06) and ethnicity (p = 0.18) (see Report Supplementary Material 10). Of the 1064 participants retained for analyses, 442 were seeking relocation into social, 436 into intermediate and 186 into market-rent accommodation; 1053 participants had physical activity data on weekdays, 848 on weekends and 837 on both weekdays and weekends. To maximise power to detect associations and reduce selection bias, analyses were performed with the maximum number of participants available (i.e. 1053 individuals in analyses on physical activity on weekdays and 848 individuals in analyses on physical activity at weekends). Sensitivity analyses were performed on the subset of 837 participants who had data on both weekdays and weekends.
Follow-up data
Of the 877 participants followed up (69%), those who lived outside Greater London at either baseline or follow-up and those who did not have physical activity data at baseline or follow-up (n = 190) were excluded. Those excluded from the analytical sample had similar characteristics to those included with regard to sex (p = 0.36) and ethnicity (p = 0.76), but were younger (p = 0.009) and had more intermediate and less market-rent seekers compared with those included (p = 0.003) (see Report Supplementary Material 11). A total of 687 participants were retained for analyses; 680 participants had physical activity data on weekdays and 517 on weekend days, both at baseline and at follow-up. Of the 687 participants, 283 were seeking relocation into social housing, 301 into intermediate housing, and 103 into market-rent accommodation.
Primary outcomes
Accelerometer-derived physical activity
Mean daily steps and mean daily time (minutes) spent in MVPA were collected from accelerometer data among those who wore the device for at least 9 hours per day for at least 1 day. Daily steps were adjusted for day of the week, day order of recording and month of accelerometer data collection. 70 Mean daily steps and mean daily time spent in MVPA specifically on weekdays and at weekends were derived.
Environmental exposures
Participants were geocoded to the centroid of the footprint of their building of residence, using OS AddressBase Premium (versions 2015 and 2017 for geocoding at baseline and at follow-up, respectively) to match participants’ declared residential addresses with XY coordinates. Residential locations were then used to derive a range of built environment factors that were hypothesised to be associated with physical activity, as follows.
Residential neighbourhood walkability
Walkability scores were calculated by summing the z-scores of the three following GIS-derived variables aggregated within a 1-km street network home-centred buffer: (1) street connectivity, as the number of junctions of three or more road branches per street-kilometre, (2) land use mix, as the evenness of distribution of square footage of residential, commercial, office, entertainment and institutional building footprints, based on existing literature52,110 and (3) net residential density, as the unique number of residential addresses per squared kilometre of building footprint devoted to residential use (i.e. residential building and attached gardens) (see Report Supplementary Material 12). Both baseline and follow-up z-score transformations were based on the mean and standard deviation of the baseline sample, as has been done previously. 89 Rescaling scores at follow-up using the baseline population sample estimates ensured comparability of scores both across participants and across time for the same participant, hence providing a meaningful quantification of the change of walkability over time. Land use mix and net residential density variables were log-transformed to fit a comparable scale. The choice of 1-km street network home-centred buffers was motivated by two considerations. First, destinations that are within 1 km (a 15-minute walk) from home have been defined as reachable by foot in the literature. 120 In the absence of more personalised measures of the residential neighbourhood, 1 km was, thus, judged as a reasonable aggregation unit to encompass the opportunities available in the residential area of each participant. Second, a 1-km buffer has been used in many studies that found significant associations between features of the built environment and physical activity outcomes. 121,122
Proximity from home to parks
Using data from Greenspace Information for Greater London (GiGL),123 proximity to three types of park – ‘metropolitan’, ‘district’ and ‘local’ – was calculated on the basis of street network distance from the home address to the nearest entrance for each park type. Park type is derived from the Greater London Authority London Plan, March 2016,124 and is based on park size and the number and type of facilities a park provides. ‘Metropolitan’ parks are the largest and have the most amenities, and ‘local’ parks are the smallest and are the least well-equipped of these three types (see Report Supplementary Material 13). Where there were missing entrance points to parks in the GiGL database (n = 22, 2.9%), they were manually geocoded based on visual data drawn from Google Maps (Google Inc., Mountain View, CA, USA).
Public transport accessibility
Each ENABLE London participant was assigned a public transport accessibility level (PTAL) score based on the closest location of public transport to their place of residence where a PTAL value was made available by TfL, the local government body responsible for the transport system in Greater London. PTAL is an averaged measure of the densities of the London public transport access points (trains, buses, underground, Docklands Light Railway, trams) and also accounts for the frequency of service. It is available for the centroid of each 100 m × 100 m cell of a grid covering the whole of Greater London and is expressed as a nine-category variable (lower scores reflecting poorer accessibility). The nine PTAL categories were collapsed into three categories (low: PTAL scores 0, 1a, 1b; intermediate: PTAL scores 2, 3, 4; high: PTAL scores 5, 6a, 6b) to increase the number of participants per category, especially for those residing in areas with the lowest accessibility scores.
Data sources and versions used for computing these environmental variables are detailed in Report Supplementary Material 14.
Covariates
Covariates included sex (female, male), age group (16–24, 25–34, 35–49 and ≥ 50 years), ethnicity (white, black, Asian, mixed/other) and aspirational housing tenure being sought (social, intermediate, market-rent). Seekers of intermediate accommodation were used as the reference group, as this group had the largest number of participants.
Household income, occupational status, presence of children in the household and education level were highly correlated to aspirational housing status, hence, these variables were not adjusted for in the final regression models to avoid overadjustment (see Report Supplementary Material 15). Other hypothesised covariates, including car use and attitude towards physical activity, were excluded after initial consideration, as they were not found to be associated with exposures and outcomes in bivariate analyses or their addition to fully adjusted models did not appreciably alter coefficients.
Statistical analyses
All analyses were carried out using Stata Special Edition version 15 for Windows.
Analyses of baseline data
First, aspirational housing tenure differences in sociodemographic characteristics, residential built environment factors (i.e. walkability, distance to parks and accessibility to public transport) and daily steps and MVPA (minutes) were examined. Chi-squared tests were used to assess the differences between housing tenure for sociodemographic characteristics and public transport availability (PTAL score). Analysis of variance was used to assess differences between housing tenure for the residential built environment and physical activity variables. Second, multilevel linear regression models, including a random effect to allow for clustering at baseline household level, were used to assess whether or not environmental variables were associated with the daily steps taken overall, on weekdays and at weekends separately. Environmental variables were examined both separately (i.e. entered in turn in the models) and concomitantly (to examine their independent association). All models were adjusted for clustering at baseline household level, sex, age group, ethnicity and aspirational housing tenures as fixed effects and household as a random effect to allow for clustering at baseline household level. We also examined whether or not effects of environmental variables on physical activity behaviour differed by aspirational housing tenure. MVPA in 10-minute bouts was not used, as most participants had none or low values and no transformation would have permitted easy interpretation of the regression coefficients. Third, where environmental factors showed significant associations with the number of daily steps, multilevel regression models further examined whether or not housing tenure differences in physical activity were attenuated after adjustment for environmental factors.
Analyses of follow-up data
First, changes in neighbourhood walkability, residential density, land use mix, street connectivity, distance to a park and accessibility to public transport were quantified in the whole sample and by housing sector, and differences between housing sectors were assessed using analysis of variance. Second, multilevel linear regression models were fitted to examine the effect of changes in exposure to residential built environment features on changes in total daily steps and total daily MVPA (minute) (one model per residential built environment exposure variable and per physical activity outcome). Average daily steps (daily MVPA) at follow-up were regressed on average daily steps (daily MVPA) at baseline, adjusting for a change in exposure as a fixed effect and baseline household as a random effect to allow for household clustering. Models with further adjustment for sex, age group and ethnic group were also fitted. In addition, the same outcomes were examined separately for both weekdays and weekend days. Finally, an interaction term between each change in environmental exposure (taken in turn) and housing sector was included.
Results
Associations between the built environment and physical activity at baseline
Descriptive analyses
Baseline descriptive statistics are shown in Table 9. Females constituted 58% of the analytical sample, which was largely white (48%). Women, older people and those belonging to ethnic minorities were more prevalent among social housing seekers than among intermediate and market-rent housing seekers (p < 0.001); the sociodemographic characteristics of intermediate and market-rent housing seekers were similar. Participants seeking relocation to social housing were less physically active, with 9.4% (social: 8618 steps, 95% CI 8247 to 8990 steps; intermediate: 9516 steps, 95% CI 9170 to 9862 steps) and 25.3% (social: 6909 steps, 95% CI 6390 to 7428 steps; intermediate: 9385 steps, 95% CI 8925 to 9846 steps) fewer steps on weekdays and at weekends, respectively, than participants seeking intermediate housing. Social housing seekers also resided in less walkable areas, according to walkability score, than intermediate housing seekers (social: –0.51, 95% CI –0.70 to –0.31; intermediate: 0.21, 95% CI –0.05 to 0.46); lived further away (median km) from metropolitan parks [social: 2.53 km, interquartile range (IQR) 1.43–3.71 km; intermediate: 1.90 km, IQR 0.95–2.99 km]; were closer (median km) to their nearest local park than intermediate housing seekers (social: 0.62 km, IQR 0.38–0.98 km; intermediate: 0.88 km, IQR 0.48–1.35 km); and had poorer accessibility to public transport (22.9% had high accessibility compared with 41.3% for intermediate housing seekers).
Characteristic | All housing sectors | Housing sector | p-valuea | ||
---|---|---|---|---|---|
Social | Intermediate | Market-rent | |||
Total participants, n (%) | 1064 (100) | 442 (42) | 463 (44) | 186 (17) | |
Sociodemographics, n (%) | |||||
Sex: female | 621 (58) | 330 (75) | 210 (48) | 81 (44) | < 0.001 |
Age group (years) | |||||
16–24 | 222 (21) | 95 (21) | 77 (18) | 50 (27) | < 0.001 |
25–34 | 464 (44) | 112 (25) | 254 (58) | 98 (53) | |
35–49 | 310 (29) | 198 (45) | 92 (21) | 20 (11) | |
≥ 50 | 68 (6) | 37 (8) | 13 (3) | 18 (10) | |
Ethnicity | |||||
White | 511 (48) | 83 (19) | 301 (69) | 127 (68) | < 0.001 |
Black | 270 (25) | 210 (48) | 46 (11) | 14 (8) | |
Asian | 172 (16) | 90 (20) | 61 (14) | 21 (11) | |
Mixed/other | 111 (10) | 59 (13) | 28 (6) | 24 (13) | |
Physical activity facilities in the residential area | |||||
Walkability, mean (95% CI) | 0.04 (–0.12 to 0.20) | –0.51 (–0.70 to –0.31) | 0.21 (–0.05 to 0.46) | 0.94 (0.48 to 1.40) | < 0.001 |
Street connectivity, mean (95% CI) | 8.7 (8.6 to 8.7) | 8.5 (8.4 to 8.6) | 8.7 (8.6 to 8.8) | 9.0 (8.8 to 9.2) | < 0.001 |
Residential density (1000 habitants/km2), mean (95% CI) | 12.2 (11.9 to 12.6) | 10.3 (9.9 to 10.7) | 13.1 (12.5 to 13.8) | 14.8 (13.8 to 15.9) | < 0.001 |
Land use mix, mean (95% CI) | 0.38 (0.37 to 0.39) | 0.34 (0.33 to 0.36) | 0.39 (0.37 to 0.41) | 0.46 (0.43 to 0.49) | < 0.001 |
Distance to metropolitan parks (km), mean (IQR) | 2.2 (1.2–3.5) | 2.5 (1.4–3.5) | 1.9 (0.9–3.5) | 1.9 (1.0–3.5) | < 0.001 |
Distance to district parks (km), mean (IQR) | 2.2 (1.4–3.1) | 2.4 (1.7–3.1) | 2.0 (1.1–3.1) | 2.0 (1.4–3.1) | < 0.001 |
Distance to local parks (km), mean (IQR) | 0.7 (0.4–1.2) | 0.6 (0.4–1.2) | 0.9 (0.5–1.2) | 0.9 (0.5–1.2) | < 0.001 |
Public transport accessibility (PTAL score), n (%) | |||||
Low | 96 (9) | 47 (11) | 37 (8) | 12 (6) | |
Intermediate | 606 (57) | 294 (67) | 219 (50) | 93 (50) | |
High | 362 (34) | 101 (23) | 180 (41) | 81 (44) | |
Physical activity,b mean (95% CI) | |||||
Daily steps on weekdays | 9126 (8919 to 9333) | 8618 (8247 to 8990) | 9516 (9170 to 9862) | 9409 (8895 to 9923) | 0.005 |
Daily steps on weekend days | 8448 (8170 to 8725) | 6909 (6390 to 7428) | 9385 (8925 to 9846) | 9540 (8874 to 10,206) | < 0.001 |
Daily minutes of MVPA on weekdays | 61 (59 to 63) | 57 (54 to 60) | 63 (61 to 66) | 66 (62 to 70) | 0.001 |
Daily minutes of MVPA on weekend days | 55 (53 to 58) | 46 (41 to 50) | 61 (57 to 65) | 64 (59 to 70) | < 0.001 |
Participants seeking relocation to market-rent housing had a similar level of physical activity to participants seeking intermediate housing in both daily steps and MVPA accumulated both on weekdays and at weekends. Participants seeking market-rent housing also resided in more walkable areas according to walkability score (market-rent: 0.94, 95% CI 0.48 to 1.40; intermediate: 0.21 95% CI –0.05 to 0.46), but both groups lived at a similar distance to their closest metropolitan, district and local parks, and had similar accessibility to public transport.
Associations between built environment and physical activity
The associations between built environment factors and the number of daily steps and amount of MVPA taken on weekdays and at weekends are presented in Table 10, and displayed graphically in Report Supplementary Material 16.
Residential built environment factors | Model 1a β (95% CI) | Model 2b β (95% CI) | Model 3c β (95% CI) | Model 1a β (95% CI) | Model 2b β (95% CI) | Model 3c β (95% CI) |
---|---|---|---|---|---|---|
Daily steps on weekdays (n = 1053) | Daily steps on weekends (n = 848) | |||||
Walkability | 52 (–30 to 134) | –5 (–86 to 76) | 38 (–57 to 132) | 255 (139 to 371)d | 135 (28 to 242)d | 144 (19 to 269)d |
Street connectivity | 48 (–132 to 228) | –39 (–215 to 137) | – | 322 (63 to 582)d | 156 (–79 to 391) | – |
Residential density (1000 habitants/km2) | 41 (5 to 76)d | 5 (–31 to 41) | – | 129 (78 to 179)d | 51 (3 to 99)d | – |
Land use mix | 498 (–644 to 1641) | –291 (–1419 to 837) | – | 3520 (1884 to 5156)d | 1547 (34 to 3061)d | – |
Distance to closest metropolitan park (km) | –294 (–446 to –143)d | –206 (–354 to –58)d | –264 (–422 to –107)d | –363 (–582 to –144)d | –125 (–323 to 74) | –144 (–354 to 66) |
Distance to closest district park (km) | –93 (–265 to 79) | 7 (–160 to 174) | –64 (–240 to 113) | –370 (–617 to –124)d | –180 (–402 to 42) | –138 (–372 to 96) |
Distance to closest local park (km) | 170 (–174 to 514) | –84 (–420 to 253) | –185 (–529 to 158) | 1147 (669 to 1624)d | 597 (161 to 1032)d | 598 (155 to 1040)d |
Accessibility to public transport | ||||||
Low | 442 (–362 to 1247) | 767 (–12 to 1546) | 1186 (296 to 2076)d | –1352 (–2516 to –187)d | –608 (–1657 to 442) | 60 (–1136 to 1256) |
Intermediate | –481 (–948 to –15)d | –152 (–609 to 305) | 8 (–487 to 503) | –1128 (–1803 to –453)d | –364 (–981 to 252) | –8 (–670 to 654) |
High | – | – | – | – | – | – |
Daily MVPA (minutes) on weekdays (n = 1053) | Daily MVPA (minutes) on weekends (n = 848) | |||||
Walkability | 0.7 (–0.0 to 1.3) | 0.1 (–0.6 to 0.6) | 0.4 (–0.3 to 1.2) | 2.2 (1.2 to 3.1)d | 1.2 (0.3 to 2.1)d | 1.4 (0.4 to 2.5)d |
Street connectivity | 0.6 (–0.9 to 2.0) | –0.3 (–1.7 to 1.0) | – | 2.7 (0.6 to 4.8)d | 1.3 (–0.6 to 3.2) | – |
Residential density (1000 habitant/km2) | 0.4 (0.1 to 0.7)d | 0.0 (–0.2 to 0.3) | – | 1.1 (0.7 to 1.5)d | 0.4 (0.0 to 0.8)d | – |
Land use mix | 9.1 (0.0 to 18.2)d | 1.3 (–7.5 to 10.1) | – | 32.1 (18.9 to 45.2)d | 16.8 (4.6 to 29.0)d | – |
Distance to closest metropolitan park (km) | –2.5 (–3.7 to –1.3)d | –1.8 (–2.9 to –0.5)d | –2.1 (–3.4 to –0.9)d | –2.9 (–4.7 to –1.2)d | –1.1 (–2.7 to 0.4) | –1.2 (–2.9 to 0.5) |
Distance to closest district park (km) | –0.6 (–2.0 to 0.7) | 0.3 (–1.0 to 1.6) | –0.2 (–1.5 to 1.2) | –2.4 (–4.4 to –0.4)d | –0.9 (–2.7 to 0.9) | –0.4 (–2.3 to 1.4) |
Distance to closest local park (km) | 2.0 (–0.8 to 4.7) | –0.3 (–3.0 to 2.3) | –1.0 (–3.7 to 1.6) | 9.0 (5.2 to 12.9)d | 4.7 (1.2 to 8.2)d | 4.9 (1.3 to 8.5)d |
Accessibility to public transport | ||||||
Low | 2.7 (–3.7 to 9.1) | 5.8 (–0.3 to 11.9) | 9.7 (2.7 to 16.6)d | –9.5 (–18.9 to –0.1)d | –4.0 (–12.5 to 4.5) | 2.7 (–7.0 to 12.4) |
Intermediate | –4.5 (–8.2 to –0.8)d | –1.7 (–5.3 to 1.8) | –0.2 (–4.1 to 3.6) | –8.2 (–13.7 to –2.7)d | –2.7 (–7.6 to 2.3) | 0.7 (–4.7 to 6.0) |
High | – | – | – | – | – | – |
Weekdays
In models adjusted for sociodemographic characteristics, both daily steps taken and MVPA accumulated were negatively associated with the distance from home to the closest metropolitan park [mean difference in daily steps (–206 steps, 95% CI –354 to –58 steps); mean difference in daily minutes of MVPA (–1.8 minutes, 95% CI –2.9 to –0.5 minutes) per kilometre of distance to the closest metropolitan park], indicating that the more distant the metropolitan park, the smaller the overall number of steps taken and the shorter the time spent in MVPA (Figure 4), particularly among those from intermediate and market-rent tenured groups (see Figure 4). Associations between accessibility to public transport and daily steps and MVPA were borderline statistically significant in models adjusted for sociodemographic characteristics (mean differences in daily steps: 767 steps, 95% CI –12 to 1546 steps; mean difference in daily minutes of MVPA: 5.8 minutes, 95% CI –0.3 to 11.9 minutes for those with low accessibility compared with those with high accessibility), and reached statistical significance after further adjustment for the other residential built environmental factors (mean differences in daily steps: 1186 steps, 95% CI 296 to 2076 steps; mean difference in daily minutes of MVPA: 9.7 minutes, 95% CI 2.7 to 16.6 minutes). Adjustment for other residential built environmental factors, however, led to an inflation of the regression estimate and a widening of the CI compared with models adjusting only for sociodemographic characteristics, raising potential multicollinearity issues.
FIGURE 4.
Daily steps and MVPA (mean and 95% CI) by quintile of selected residential built environmental factors, overall and by housing sector. (a) Daily steps at weekends and walkability; (b) daily steps at weekends and distance to closest local park; (c) daily steps on weekdays and distance to closest metropolitan park; (d) daily MVPA at weekends and walkability; (e) daily MVPA at weekends and distance to closest local park; and (f) daily MVPA on weekdays and distance to closest metropolitan park. Notes: mean daily steps (95% CI) at weekends or on weekdays are plotted against median value of quintile of built environment variable, for all housing sectors combined and by housing sector. Daily steps are adjusted for sex, age group, ethnic group as fixed effects and household as a random effect in a multilevel model. Daily steps for all housing sectors are additionally adjusted for housing sector as a fixed effect. Footnotes: mean daily MVPA (95% CI) at weekends or on weekdays is plotted against median value of quintile of built environment variable, for all housing sectors combined and by housing sector. Daily MVPA is adjusted for sex, age group, ethnic group as fixed effects and household as a random effect in a multilevel model. Daily MVPA for all housing sectors is additionally adjusted for housing sector as a fixed effect.
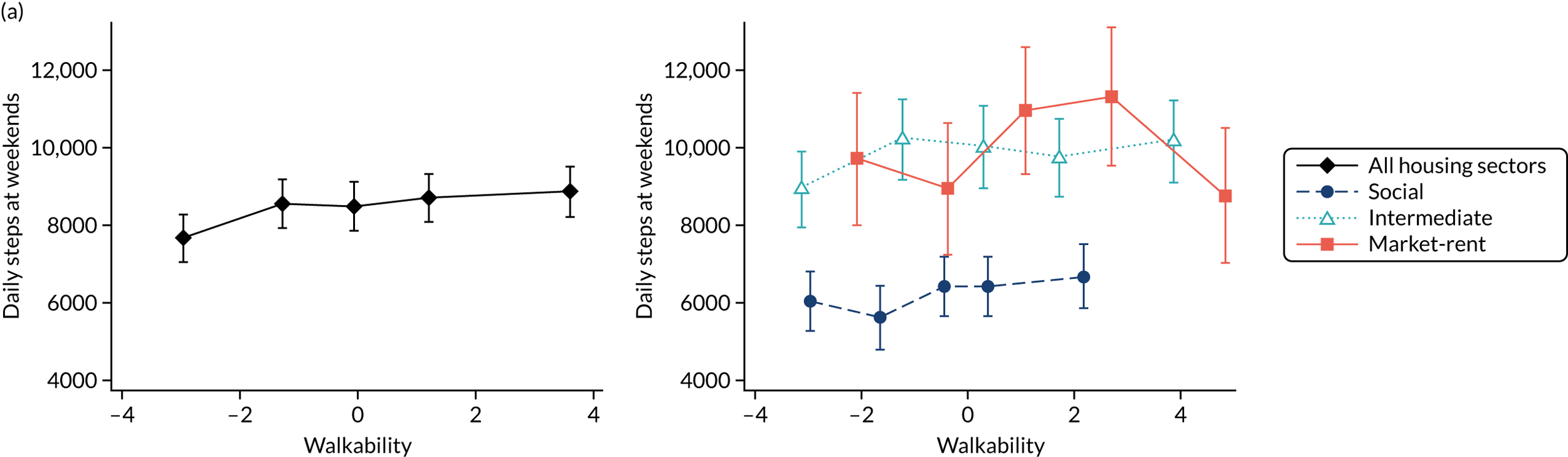
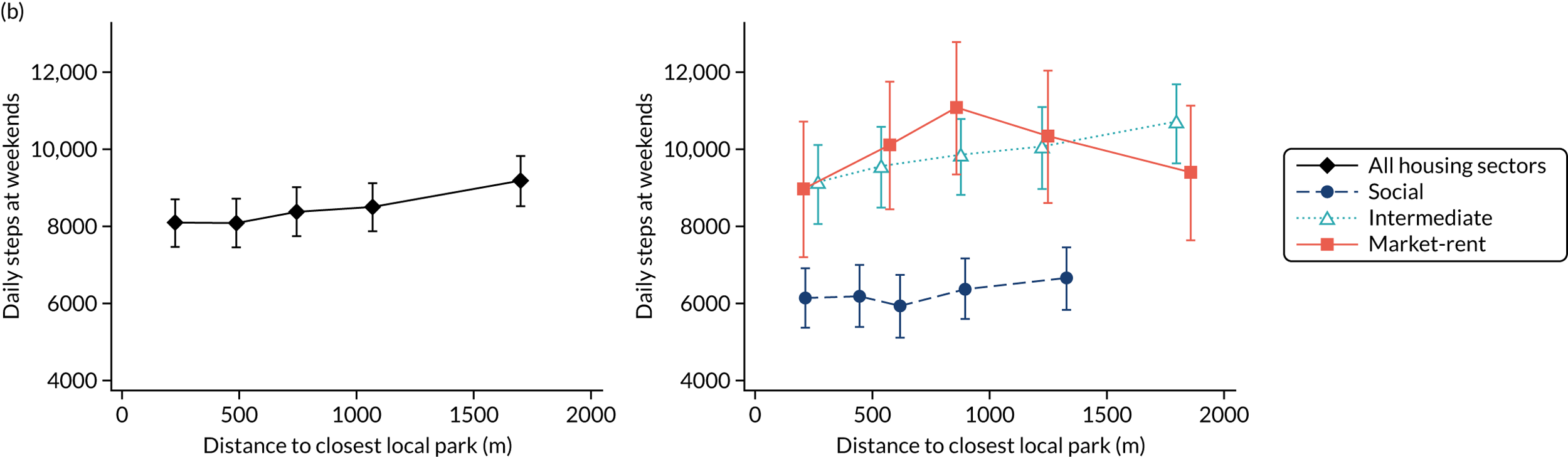
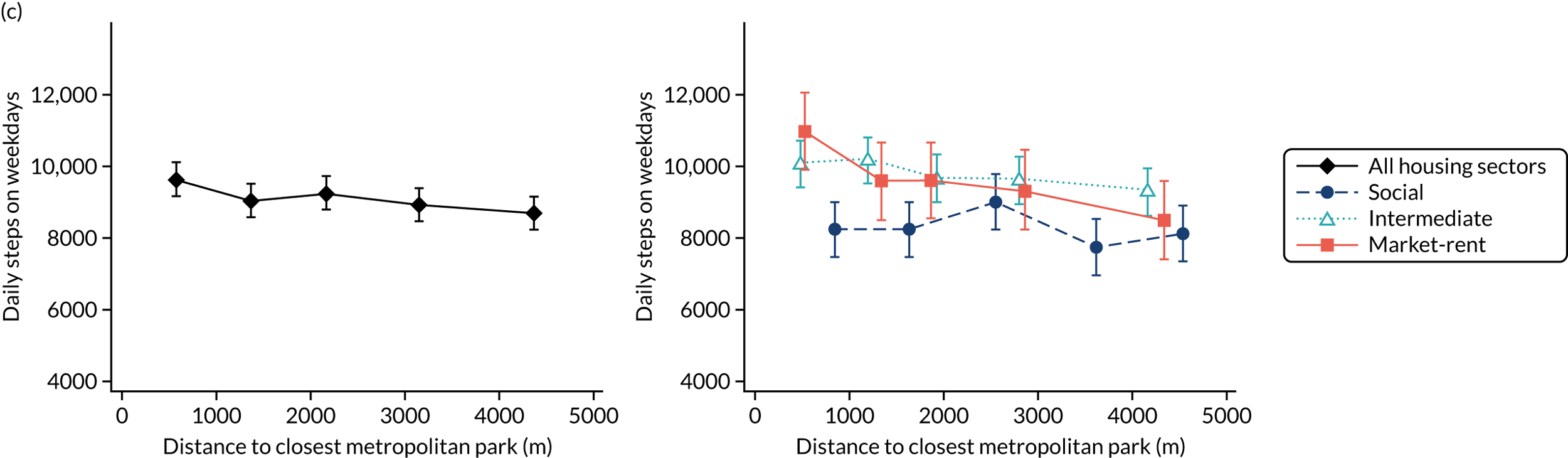

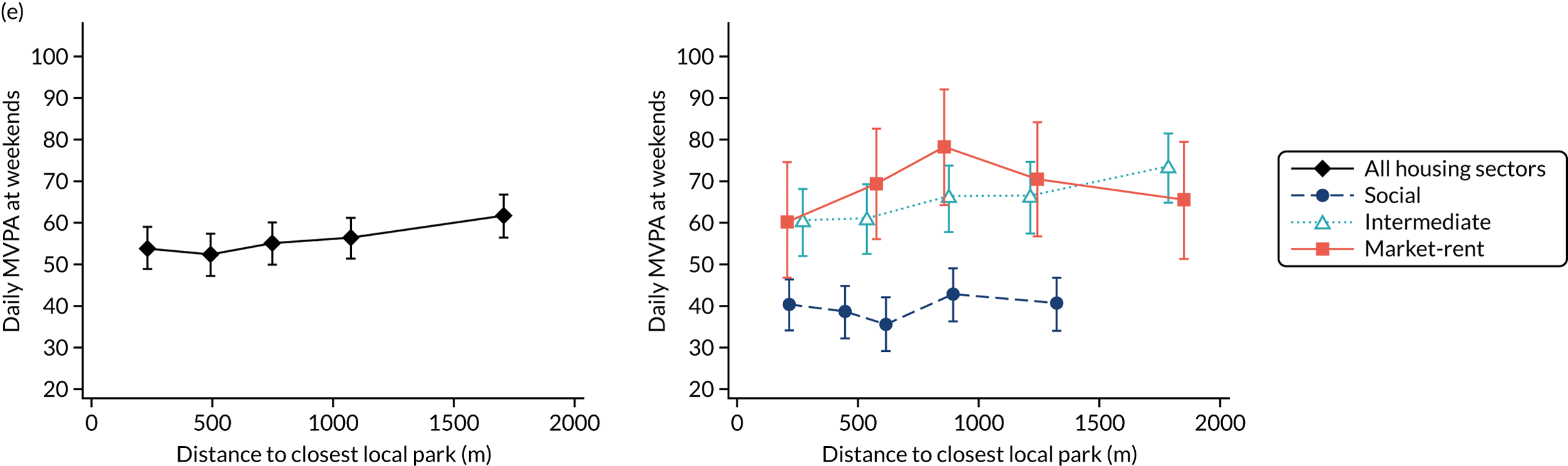
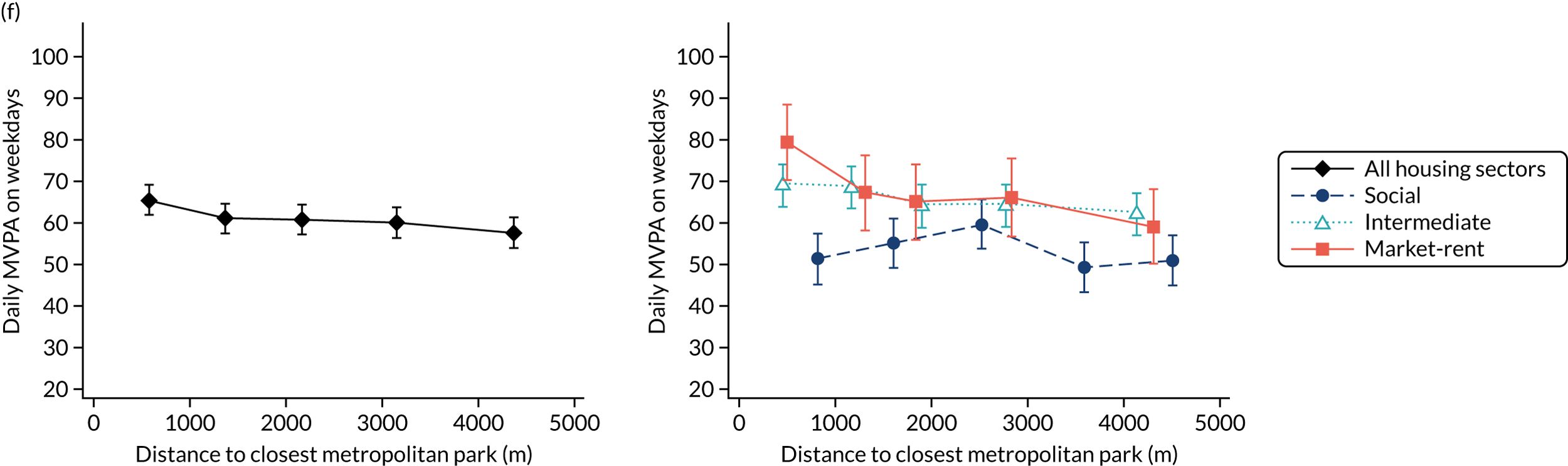
On weekend days
In minimally adjusted models, both daily steps and MVPA accumulated were positively associated with residential walkability (mean difference in daily steps and daily minutes of MVPA per unit of walkability: 255 steps, 95% CI 139 to 371 steps, and 2.2 minutes, 95% CI 1.2 to 3.1 minutes, respectively), and with the distance from home to the closest local park (mean difference in daily steps and daily minutes of MVPA per kilometre of distance to the closest local park: 1147 steps, 95% CI 669 to 1624 steps, and 9.0 minutes, 95% CI 5.2 to 12.9 minutes, respectively). Conversely, steps taken and minutes of MVPA were negatively associated with the distance (km) to the nearest metropolitan park (–363 steps, 95% CI –582 to –144 steps, and –2.9 minutes, 95% CI –4.7 to –1.2 minutes, respectively), the distance (km) to the closest district park (–370 steps, 95% CI –617 to –124 steps, and –2.9 minutes, 95% CI –4.7 to –1.2 minutes, respectively) and accessibility to public transport (–1352 steps, 95% CI –2516, to –187 steps, and –9.5 minutes, 95% CI –18.9 to –0.7 minutes, respectively, for those with low compared with those with high accessibility). After adjustment for sociodemographic characteristics, associations of residential walkability with daily steps taken (135 steps, 95% CI 28 to 242 steps) and with minutes of MVPA (1.2 minutes, 95% CI 0.3 to 2.1 minutes), and of distance from home to the nearest local park with daily steps taken (597 steps, 95% CI 161 to 1032 steps) and with minutes of MVPA (4.7 minutes, 95% CI 1.2 to 8.2 minutes) remained statistically significant. This suggests that the more walkable the residential environment the higher the number of steps taken and the greater the time spent in MVPA, and that overall the further the closest local park, the higher the number of steps taken and the greater the time spent in MVPA on weekend days (Figure 4); this appeared more evident in the social and intermediate housing sectors (Figure 4). Associations remained consistent after further adjustment for the other residential built environmental factors (model 3).
Interaction analyses
An interaction between low accessibility to public transport and social versus intermediate housing seekers in relation to mean daily steps on weekdays was observed (p = 0.042). However, a Wald test for the interaction term between accessibility to public transport and housing suggested that the interaction term could be omitted from the model (p = 0.06).
Effect of adjustment for built environmental factors and sensitivity analyses
The effect of adjustment for built environment factors on the differences between aspirational housing tenures in both daily steps and daily minutes of MVPA is presented in Table 11. Adjustment for walkability decreased differences in daily steps and MVPA on weekend days between social and intermediate housing seekers by 1.5% and 2.6%, respectively (i.e. a decrease of 36 steps and a decrease of 0.4 minutes in the gap between social and intermediate housing seekers). Adjustment for distance to the closest local park decreased differences in daily steps and MVPA on weekend days between social and intermediate housing seekers by 4.7% and 5.9%, respectively (i.e. a decrease of 113 steps and a decrease of 0.9 minutes). Adjustment for distance to the nearest metropolitan park reduced differences in steps taken on weekdays between social and intermediate housing seekers by 9.9% and 11.9%, respectively (i.e. a decrease of 81 steps and a decrease of 0.7 minutes). Sensitivity analyses on the subsample of 837 participants with physical activity data on both weekdays and weekends showed consistency with the main findings presented in the weekday and weekend day sensitivity analyses in the previous sections (see Report Supplementary Materials 17–19). The base model adjusts for sex, age group, ethnic group as fixed effects and household as a random effect to allow for clustering at the household level.
Outcome | Base model, difference (95% CI) | Base model with further adjustments, difference (95% CI) | Land use mix, difference (95% CI) | |||||||
---|---|---|---|---|---|---|---|---|---|---|
Walkability | Distance to local parks | Distance to district parks | Distance to metropolitan parks | Accessibility to public transport | Walkability, distance to local, district and metropolitan parks, accessibility to public transport | Street connectivity | Residential density | |||
Weekday daily steps (N = 1053) (steps) | ||||||||||
Social | –898 (–1448 to –348) | –899 (–1451 to –348) | –914 (–1468 to –360) | –899 (–1452 to –347) | –817 (–1369 to –266) | –884 (–1438 to –330) | –814 (–1373 to –255) | –901 (–1452 to –350) | –888 (–1443 to –333) | –906 (–1458 to –354) |
Intermediate | – | – | – | – | – | – | – | – | – | – |
Market-rent | –107 (–701 to 487) | –103 (–701 to 495) | –107 (–701 to 488) | –107 (–702 to 487) | –95 (–687 to 498) | –86 (–680 to 508) | –87 (–682 to 508) | –95 (–692 to 502) | –115 (–713 to 482) | –88 (–687 to 510) |
Weekday daily MVPA (N =1053) (minutes) | ||||||||||
Social | –6.6 (–10.9 to –2.3) | –6.6 (–10.9 to –2.3) | –6.6 (–11.0 to –2.3) | –6.7 (–11.0 to –2.4) | –5.9 (–10.2 to –1.6) | –6.4 (–10.7 to –2.1) | –5.8 (–10.2 to –1.4) | –6.6 (–10.9 to –2.3) | –6.5 (–10.8 to –2.2) | –6.5 (–10.8 to –2.2) |
Intermediate | – | – | – | – | – | – | – | – | – | – |
Market-rent | 2.3 (–2.4 to 6.9) | 2.2 (–2.4 to 6.9) | 2.3 (–2.4 to 6.9) | 2.3 (–2.4 to 6.9) | 2.4 (–2.2 to 7.0) | 2.4 (–2.2 to 7.1) | 2.3 (–2.3 to 7.0) | 2.4 (–2.3 to 7.0) | 2.2 (–2.5 to 6.9) | 2.2 (–2.5 to 6.9) |
Weekend daily steps (N = 848) (steps) | ||||||||||
Social | –2477 (–3232 to –1721) | –2441 (–3194 to –1689) | –2364 (–3122 to –1607) | –2427 (–3184 to –1671) | –2436 (–3194 to –1678) | –2409 (–3172 to –1646) | –2242 (–3007 to –1477) | –2469 (–3224 to –1714) | –2385 (–3142 to –1627) | –2442 (–3197 to –1688) |
Intermediate | – | – | – | – | – | – | – | – | – | – |
Market-rent | 155 (–624 to 934) | 33 (–748 to 815) | 149 (–628 to 925) | 168 (–609 to 946) | 161 (–617 to 940) | 136 (–644 to 916) | 38 (–742 to 818) | 101 (–682 to 883) | 66 (–715 to 847) | 39 (–747 to 824) |
Weekend daily MVPA (N = 848) (minutes) | ||||||||||
Social | –15.3 (–21.4 to –9.1) | –14.9 (–21.0 to –8.8) | –14.4 (–20.5 to –8.3) | –15.0 (–21.1 to –8.9) | –14.9 (–21.0 to –8.8) | –14.8 (–20.9 to –8.6) | –13.6 (–19.8 to –7.4) | –15.2 (–21.3 to –9.1) | –14.4 (–20.5 to –8.3) | –14.9 (–0.9 to –8.8) |
Intermediate | – | – | – | – | – | – | – | – | – | – |
Market-rent | 3.3 (–3.0 to 9.6) | 2.2 (–4.1 to 8.5) | 3.3 (–3.0 to 9.6) | 3.4 (–2.9 to 9.7) | 3.4 (–2.9 to 9.7) | 3.2 (–3.1 to 9.5) | 2.2 (–4.1 to 8.5) | 2.9 (–3.4 to 9.2) | 2.6 (–3.7 to 8.9) | 2.1 (–4.2 to 8.4) |
Change in built environment and change in physical activity
Descriptive analyses
Baseline descriptive statistics for the followed-up participants are shown in Table 12. Women, those who were middle-aged (35–49 years) and those belonging to ethnic minorities were more prevalent among social than among intermediate housing seekers (p < 0.0001). Compared with participants seeking intermediate accommodation, participants seeking social housing took fewer daily steps and accumulated fewer daily minutes in MVPA overall (p < 0.0001), on weekdays (p < 0.01) and on weekend days (p < 0.0001) at baseline. Sociodemographic characteristics and levels of physical activity at baseline of the intermediate and market-rent housing seekers were largely similar. At baseline, social housing seekers also resided in less walkable areas (walkability score social: –0.4, 95% CI –0.6 to –0.1; intermediate: 0.2, 95% CI –0.1 to 0.5; market-rent 0.4, 95% CI –0.2 to 1.1; p = 0.004), and had poorer accessibility to public transport (PTAL score social: 4.3, 95% CI 4.1 to 4.5; intermediate: 4.8, 95% CI 4.6 to 5.0; market-rent 5.0, 95% CI 4.6 to 5.3; p < 0.001).
Characteristic | All housing sectors | Housing sector | p-valuea | ||
---|---|---|---|---|---|
Social | Intermediate | Market-rent | |||
Total participants, n (%) | 687 (100) | 283 (41) | 301 (44) | 103 (15) | |
Sociodemographics, n (%) | |||||
Sex: female | 401 (58) | 206 (73) | 151 (50) | 45 (44) | < 0.001 |
Age group (years) | |||||
16–24 | 130 (19) | 53 (19) | 50 (17) | 27 (26) | < 0.001 |
25–34 | 289 (42) | 72 (26) | 172 (57) | 45 (44) | |
35–49 | 223 (33) | 137 (48) | 70 (23) | 16 (16) | |
≥ 50 | 45 (7) | 21 (7) | 9 (3) | 15 (15) | |
Ethnicity | |||||
White | 334 (49) | 52 (18) | 209 (69) | 73 (71) | < 0.001 |
Black | 172 (25) | 135 (48) | 30 (10) | 7 (7) | |
Asian | 110 (16) | 59 (21) | 43 (14) | 8 (8) | |
Mixed/other | 71 (10) | 37 (13) | 19 (6) | 15 (15) | |
Physical activity facilities in the residential area | |||||
Walkability, mean (95% CI) | 0.0 (–0.2 to 0.2) | –0.4 (–0.6 to –0.1) | 0.2 (–0.1 to 0.5) | 0.4 (–0.2 to 1.1) | 0.004 |
Street connectivity, mean (95% CI) | 8.6 (8.6 to 8.7) | 8.5 (8.4 to 8.7) | 8.7 (8.6 to 8.9) | 8.8 (8.5 to 9.1) | 0.06 |
Residential density (1000 habitants/km2), mean (95% CI) | 11.9 (11.5 to 12.3) | 10.3 (9.8 to 10.8) | 12.7 (12.0 to 13.4) | 13.7 (12.4 to 15.1) | < 0.001 |
Land use mix, mean (95% CI) | 0.37 (0.36 to 0.39) | 0.35 (0.33 to 0.37) | 0.38 (0.36 to 0.40) | 0.42 (0.38 to 0.46) | 0.01 |
Distance to closest park (m), mean (95% CI) | 663 (633 to 692) | 609 (570 to 648) | 703 (656 to 749) | 694 (602 to 787) | 0.06 |
Public transport accessibility (PTAL score), mean (95% CI) | 4.6 (4.5 to 4.8) | 4.3 (4.1 to 4.5) | 4.8 (4.6 to 5.0) | 5.0 (4.6 to 5.3) | < 0.001 |
Physical activity,b mean (95% CI) | |||||
Daily steps, mean | 8947 (8713 to 9182) | 8162 (7742 to 8582) | 9458 (9077 to 9840) | 9611 (8984 to 10,238) | |
Daily steps on weekdays | 9173 (8915 to 9431) | 8525 (8062 to 8987) | 9581 (9162 to 10,000) | 9754 (9065 to 10,443) | |
Daily steps on weekend days | 9173 (8915 to 9431) | 8525 (8062 to 8987) | 9581 (9162 to 10,000) | 9754 (9065 to 10,443) | |
Daily minutes of MVPA | 60 (58 to 61) | 54 (50 to 57) | 63 (60 to 66) | 67 (62 to 72) | |
Daily minutes of MVPA on weekdays | 61 (59 to 63) | 56 (52 to 59) | 64 (61 to 67) | 69 (63 to 74) | |
Daily minutes of MVPA on weekend days | 55 (53 to 58) | 45 (40 to 50) | 61 (57 to 65) | 64 (57 to 71) |
Longitudinal changes in built environment exposures
Within-person changes in exposure to built environment factors over the 2-year period between baseline and follow-up are shown in Table 13. Followed-up participants experienced a positive change in neighbourhood walkability of 1.4 units (95% CI 1.2 to 1.6 units). Social housing seekers had the greatest improvement in neighbourhood walkability (1.7 units, 95% CI 1.4 to 2.0 units) compared with intermediate (1.3 units, 95% CI 0.9 to 1.6 units) and market-rent (1.0 units, 95% CI 0.3 to 1.7 units) housing participants. Improvement in walkability scores was mostly driven by increases in the residential density (7779 residential units per km2, 95% CI 6910 to 8648 residential units per km2) and land use mix (0.21 units, 95% CI 0.19 to 0.23 units). Participants experienced a mean decrease in distance to the nearest park of 270 m (95% CI 232 to 307 m), with no significant differences across housing sector. They also had a positive change of 0.7 units (95% CI 0.6 to 0.9 units) in accessibility to public transport. Social housing participants experienced the greatest amount of change (1.5 units, 95% CI 1.2 to 1.8 units) compared with intermediate (0.2 units, 95% CI 0.0 to 0.5 units) and market-rent (0.1 units, 95% CI –0.3 to 0.5 units) housing participants.
Change | All housing sectors (N = 687) | Housing sector | p-value | ||
---|---|---|---|---|---|
Social (N = 283) | Intermediate (N = 301) | Market-rent (N = 103) | |||
Physical activity facilities in the residential area, mean (95% CI) | |||||
Walkability (score) | 1.4 (1.2 to 1.6) | 1.7 (1.4 to 2.0) | 1.3 (0.9 to 1.6) | 1.0 (0.3 to 1.7) | 0.03 |
Street connectivity (intersections per km of road) | –0.5 (–0.6 to –0.4) | –0.6 (–0.7 to –0.4) | –0.5 (–0.7 to –0.4) | –0.4 (–0.7 to –0.1) | 0.63 |
Residential density (1000 habitants/km2) | 7.8 (6.9 to 8.7) | 8.9 (7.6 to 10.2) | 7.2 (5.9 to 8.5) | 6.5 (4.0 to 8.9) | 0.09 |
Land use mix (score) | 0.21 (0.19 to 0.23) | 0.25 (0.21 to 0.27) | 0.20 (0.17 to 0.23) | 0.12 (0.07 to 0.17) | < 0.001 |
Distance to the closest park (m) | –270 (–307 to –232) | –303 (–349 to –256) | –262 (–322 to –203) | –201 (–325 to –76) | 0.19 |
Accessibility to public transport (PTAL score) | 0.7 (0.6 to 0.9) | 1.5 (1.2 to 1.8) | 0.2 (0.0 to 0.5) | 0.1 (–0.3 to 0.5) | < 0.001 |
Overall, positive changes in the built environment exposures were mostly observed in those who relocated into East Village (n = 357). On average, they experienced a 2.4-unit (95% CI 2.1 to 2.7 units) increase in neighbourhood walkability, a 531-m (95% CI 488 to 574 m) decrease in the distance to their nearest park, and a 1.6-point (95% CI 1.3 to 1.9 points) increase in accessibility to public transport (see Report Supplementary Material 20). Comparatively small changes in exposures were observed for those who did not move to East Village (n = 330) (see Report Supplementary Material 20).
Longitudinal associations between changes in exposure and changes in physical activity
Associations between changes in the built environment factors and changes in total daily steps and total daily MVPA (overall, weekdays and weekend days) are presented in Table 14. In fully adjusted models, a 1 SD increase in neighbourhood walkability was associated with an overall increase of 302 steps per day (95% CI 110 to 494 steps per day) and 360 steps per weekday (95% CI 149 to 567 steps per weekday). For residential density, a 1 SD increase was associated with an overall increase of 313 steps per day (95% CI 123 to 504 steps per day) and 452 steps per weekday (95% CI 246 to 658 steps per weekday). For land use mix, a 1 SD increase was associated with an overall increase of 201 steps per day (95% CI 5 to 398 steps per day) and 257 steps per weekday (95% CI 43 to 471 steps per weekday). Associations with physical activity on weekend days were all in the same direction as weekdays, but none reached statistical significance.
Change in exposure (baseline to follow-up) | Overall (n = 687) | Weekdays (n = 680) | Weekends (n = 517) | |||
---|---|---|---|---|---|---|
Model 1a | Model 2b | Model 1a | Model 2b | Model 1a | Model 2b | |
Outcome: daily steps, standardised effectsc (95% CI); p-value | ||||||
Walkability | 313 (121 to 504); 0.001 | 302 (110 to 494); 0.002 | 360 (152 to 567); 0.001 | 358 (149 to 567); 0.001 | 273 (–82 to 629); 0.132 | 266 (–86 to 619); 0.139 |
Residential density | 331 (141 to 521); 0.001 | 313 (123 to 504); 0.001 | 460 (255 to 666); < 0.001 | 452 (246 to 658); < 0.001 | 231 (–118 to 580); 0.194 | 199 (–146 to 545); 0.258 |
Land use mix | 217 (25 to 409); 0.027 | 201 (5 to 398); 0.044 | 251 (42 to 560); 0.018 | 257 (43 to 471); 0.019 | 180 (–171 to 531); 0.315 | 162 (–190 to 515); 0.366 |
Street connectivity | 135 (–59 to 324); 0.176 | 133 (–58 to 325); 0.173 | 146 (–63 to 355); 0.170 | 135 (–75 to 344); 0.208 | 104 (–249 to 458); 0.563 | 122 (–230 to 474); 0.497 |
Distance to park | 33 (–159 to 224); 0.739 | 55 (–136 to 247); 0.571 | 3 (–207 to 211); 0.980 | 18 (–192 to 228); 0.863 | 26 (–327 to 380); 0.884 | 55 (–296 to 406); 0.760 |
Accessibility to public transportation | –5 (–198 to 189); 0.963 | –7 (–205 to 191)d; 0.943 | –54 (–264 to 156); 0.612 | –44 (–259 to 172)e; 0.690 | –67 (–424 to 289); 0.711 | 12 (–348 to 373); 0.946 |
Outcome: MVPA (minutes), standardised effectsc (95% CI); p-value | ||||||
Walkability | 1.8 (0.3 to 3.3); 0.018 | 1.7 (0.2 to 3.2); 0.026 | 1.7 (0.0 to 3.4); 0.047 | 1.6 (–0.0 to 3.3); 0.056 | 2.2 (–0.6 to 5.0); 0.123 | 2.2 (–0.6 to 5.0); 0.121 |
Residential density | 1.8 (0.3 to 3.3); 0.017 | 1.7 (0.2 to 3.2); 0.031 | 2.3 (0.6 to 3.9); 0.008 | 2.1 (0.5 to 3.8); 0.012 | 2.2 (–0.5 to 5.0); 0.110 | 2.0 (–0.7 to 4.7); 0.143 |
Land use mix | 0.9 (–0.6 to 2.4); 0.237 | 0.8 (–0.8 to 2.3); 0.336 | 0.6 (–1.1 to 2.3); 0.479 | 0.6 (–1.1 to 2.3); 0.498 | 1.7 (–1.1 to 4.4); 0.238 | 1.5 (–1.3 to 4.3); 0.289 |
Street connectivity | 1.1 (–0.4 to 2.6); 0.134 | 1.1 (–0.4 to 2.6); 0.137 | 1.2 (–0.5 to 2.8); 0.170 | 1.0 (–0.6 to 2.7); 0.221 | 0.6 (–2.2 to 3.4); 0.681 | 0.9 (–1.9 to 3.6); 0.540 |
Distance to park | 0.4 (–1.1 to 1.9); 0.636 | 0.6 (–0.9 to 2.1); 0.440 | 0.3 (–1.3 to 2.0); 0.687 | 0.5 (–1.1 to 2.2); 0.540 | –0.5 (–3.3 to 2.3); 0.718 | –0.2 (–3.0 to 2.6); 0.884 |
Accessibility to public transportation | –0.4 (–1.9 to 1.2); 0.642 | –0.2 (–1.8 to 1.3); 0.755 | –1.0 (–2.7 to 0.7); 0.246 | –0.7 (–2.5 to 1.0)f; 0.400 | –0.6 (–3.4 to 2.2); 0.684 | 0.1 (–2.7 to 2.9); 0.942 |
Interactions
Interactions between accessibility to public transport and housing sector in relation to mean daily steps, mean daily steps on weekdays and mean MVPA on weekdays were observed (results not shown). Effect sizes by housing sector for these associations are shown in the footnotes of Table 14. A 1 SD increase in accessibility to public transport was significantly associated with 395 fewer daily steps (95% CI 70 to 720 daily steps) and 2.9 fewer minutes of MVPA (95% CI 0.3 to 5.5 minutes) on weekdays for social housing seekers, but, conversely, 657 more daily steps (95% CI 4 to 1309 steps) and 5.7 more minutes of MVPA (95% CI 0.4 to 10.9 minutes) on weekdays for market-rent housing participants.
Discussion
It has been suggested that, the more walkable an urban environment is, the more attractive it is for active modes of travel such as walking. 126,127 In this study, we found that, at baseline, participants took more steps and undertook more MVPA at weekends when living in more walkable neighbourhoods. Our findings suggest that individuals may be more influenced by residential built environment factors at weekends than on weekdays, which could be explained by the greater use of their local neighbourhood during the weekend. At follow-up, there were considerable improvements in built environment exposures hypothesised to support physical activity. There were improvements in walkability driven by increases in residential density and land use mix, which were both strongly associated with physical activity. Distance to their nearest park reduced and there was an increased accessibility to public transport. These changes were mostly driven by those who moved to East Village, reflecting its location on the edge of the Queen Elizabeth II Park and the nature of the high-density neighbourhood development built on active design principles. Non-movers to East Village experienced, on average, small changes in exposure. This could be because the environment did not change in any meaningful way for those who remained at the same address, or because the residential locations that individuals moved to were similar to that at baseline. Our multivariable regression findings indicate that positive changes in neighbourhood walkability were associated with increases in daily steps and total amount of MVPA overall and on weekdays, but less so at weekends (although power to examine weekend and weekday associations was reduced).
Baseline findings
Participants took a greater number of steps at the weekend when living further away from a local park. This may arise because physical activity associated with the use of local parks relies on the journey to the park (assuming a physically active commute), rather than on the activities undertaken within them. Conversely, living further away from the closest metropolitan park was associated with fewer steps taken on weekdays. Metropolitan parks are sparser and therefore further away on average than local parks, and this may reflect decreased interest in reaching this type of park with increasing distance. Overall, our findings highlight the importance of distinguishing between different park types. This may help to explain inconsistent findings in the literature on the access to green space and physical activity, which reports both null112,122 and positive32,111,113,128–130 associations. Exploring individuals’ mobility behaviours, for instance through the combined use of GPS and accelerometer data,131 would help unpick the use made of these different parks and refine the mechanisms by which they could be associated with weekday and weekend physical activity.
In line with other studies, we found that with poorer accessibility to pubic transport, fewer steps were taken and time spent in daily MVPA was lowest at the weekends. 32,113 This suggests that on weekends people’s interest in the use of public transport may decrease with decreasing accessibility; however, there was weak evidence for an association after adjustment for sociodemographic factors. Conversely, on weekdays participants with a low accessibility to public transport were taking more daily steps than those with high accessibility. One explanation could be that participants rely on public transport for weekday activities such as commuting to work. As a result, those with poor accessibility to public transport walk more to reach transit points. Overall, differing patterning of associations on weekend days and weekdays suggest that the use of public transport depends on utility and travel function: a commute to work on weekdays and leisure and recreation at the weekends. Further exploration of the driving forces for the use of public transport on weekdays versus at weekends would be valuable to better understand the overall contribution of public transport accessibility to physical activity level.
We explored two plausible pathways through which the built environment may partly contribute to the observed aspirational housing tenure differences in physical activity: differences in the way the three groups interact with the physical activity-supportive features of their residential environment and differences in the availability of these features. We found little evidence for the former explanation, with weak evidence for an interaction. As for the latter explanation, we found that social housing seekers lived in less walkable environments, closer to local parks and further away from metropolitan parks than intermediate and market-rent housing seekers, which, in turn, were all associated with a decreased number of daily steps and daily minutes of MVPA. However, disparities in these three environmental features accounted for little of the variability in daily steps (1.5%, 4.7% and 9.9%, respectively) and in daily minutes of MVPA (2.6%, 5.9% and 11.9%, respectively) between social and intermediate participants. Our findings support previous work that suggests that housing tenure might relate to health behaviours by sorting disadvantaged people into neighbourhoods provided with fewer health-promoting amenities. 132,133
Longitudinal findings
The longitudinal findings strengthen previous cross-sectional evidence that more walkable environments are associated with higher levels of physical activity. 110,121,134–136 The significant associations observed were mostly driven by the two components of walkability, residential density and land use mix, which were both strongly and positively associated with increased physical activity levels, consistent with other longitudinal studies. 89,95,137–140 Greater land use mix is posited to support walking by offering accessibility to a wider range of services and employment. 141 As for higher residential density, it is assumed to provide more of a critical mass of walkers seen by other people, who may, in turn, be encouraged to walk by safety in numbers142 and/or by a desire to comply with the social norm. 143 Traffic congestion associated with higher residential density may also promote active travel. 144 Unlike others,145,146 we did not find that a change in street connectivity was associated with a change in the number of steps taken or the amount of MVPA accumulated. This may be partly because our street connectivity metric relied on street network data and therefore fell short in capturing the permeability of newly built active design environments, like East Village, composed of large pedestrianised areas and informal footpaths. Hence, the limitation of our street connectivity measure to reflect the permeability of the residential neighbourhood as experienced by East Village movers may have underestimated the strength of the effect between walkability and physical activity.
Although the relationships between walkability (and its component variables) and physical activity on weekend days were all in the same direction, none reached statistical significance. This may be because the built environment is more likely to be associated with transportation walking than other types of physical activity, including recreational walking. 147 Alternatively, the limited number of participants who had physical activity data available at weekends compared with weekdays may have resulted in underpowered analyses for models fitting physical activity at weekends as an outcome.
We found evidence that increasing accessibility to public transport is associated with a statistically significant decrease in physical activity on weekdays among social housing participants and, conversely, an increase in weekdays physical activity among market-rent housing participants. Among possible explanations, different patterns in the distance between home and workplace and/or in the availability of individual modes of transport across socioeconomic groups may trigger different responses to increased accessibility to public transport. Overall, socioeconomic differences in the relationship between accessibility to public transport and physical activity may explain the mixed findings outlined by some literature reviews. 147
Unlike others, we did not find evidence that living closer to a park at follow-up than at baseline was associated with a change in physical activity level. 89 Previous studies have highlighted the importance of distinguishing between different types of park, as size and attractiveness are associated with physical activity. 128 However, since half of the ENABLE London participants were relocated to the edge of the Olympic park, a large metropolitan park with many sport facilities, such a classification became meaningless. It was difficult to disentangle the different relationship that individuals may have with different types of parks, which may partly explain the null findings.
Those who have lived in their current home for < 2 years have been found to take longer to orientate themselves within their neighbourhood and have a greater mismatch between objectively measured and perceived neighbourhood features. 148 This may partly explain the absence of findings between changes in exposure to some of the built environment features (i.e. distance to parks) and changes in physical activity behaviours. It also suggests that the associations found may strengthen over time.
Strengths
The strengths of this study include the use of validated objective measures of physical activity,149 considering weekday/weekend variations in physical activity undertaken,113 and exploring the contribution of the residential built environment in explaining socioeconomic differences in physical activity levels, which has been little assessed. Sensitivity analyses further strengthened the findings by showing consistent results in both the inclusive analytical sample and the more restricted sample of participants with physical activity data on both weekdays and weekends. This study is also one of very few longitudinal studies to have enabled examination of how change in GIS-derived residential built environment features is associated with change in accelerometer-based physical activity levels. Its design provided a great variability in changes of exposures to various features of the environment, facilitating the detection of associations. Moreover, a relatively large number of participants were enrolled compared with other longitudinal studies (e.g. Wells and Yang145 and McCormack et al. 150).
It is of note that these analyses looking at physical activity in relation to change in the built environment showed statistically significant increases in physical activity, whereas the increases in physical activity were not statistically significant when comparing the East Village group with those who did not move into East Village. There are a number of possible explanations that we are exploring further. In particular, ‘change in walkability’, for example, is a continuous measure and therefore has more power to detect changes in physical activity than using the dichotomous variable East Village or control.
Limitations
Limitations also warrant consideration. The cross-sectional study design of the baseline data does not rule out the selection of more active people into neighbourhoods supportive of physical activity, and therefore restricts interpretations about the direction of effects. Moreover, not considering physical activity facilities available in routinely visited settings other than place of residence (e.g. workplace) may have led to an overestimation of the association between the residential environment and health behaviours. 151 Because the sample was not randomly selected from the population at large, that is the study was targeted at those seeking to move to East Village, findings may not be more broadly generalisable, which may have implications for external validity.
Conclusions
The findings indicated that the residential built environment is associated with physical activity behaviours differently at weekends and on weekdays and that changes in the residential built environment are associated with changes in physical activity, and both of these differ by weekday and weekend. There was limited evidence that socioeconomic disparities in residential built environment factors contribute to socioeconomic differences in physical activity. These findings provide strong support for considering more walkable urban designs, composed of more mixed land use and higher residential density, as levers to increase physical activity. In addition, this work also suggests that policy-makers should be sensitive to the possibility that environmental interventions might have differential impacts on physical activity at weekdays and weekends.
Chapter 5 The development of an open-source tool to identify different travel modes from hip-worn accelerometer and GPS data, and its application to the ENABLE London study
Introduction
Active travel, predominantly by walking and cycling, is an accessible form of physical activity that is associated with positive health outcomes. 152–154 Quantifying the proportion of time spent in different active travel modes is therefore important to understand how these contribute to overall physical activity and health, and to assess the effectiveness of interventions that aim to increase active travel.
Travel modes have previously been assessed using detailed travel diaries;155 however, self-reported data have limitations, including that they may be subject to social desirability and recall bias and will often relate to only a single day of travel. 156 Activity and movement patterns are now increasingly objectively assessed using accelerometers and GPS receivers;157–159 combining accelerometer and GPS data allows for the identification of both the intensity and the location of physical activity. This combination is potentially valuable in describing travel behaviour, and particularly active travel behaviour. In recent years, supervised machine learning has shown the potential to identify active travel from physical activity data. Supervised machine learning algorithms are trained on an example data set and are then used for prediction to other data sets. Until recently, the most promising algorithms were random forests: ensemble supervised learning algorithms where predictions are taken from a consensus across a large number of decision trees. 160–162 A related algorithm, gradient boosted trees, has recently replaced random forests as a leading algorithm for data science tasks, with many machine learning approaches using the XGBoost (University of Washington, Seattle, WA, USA) implementation of gradient boosting instead of random forests. 163,164
Using data collected in the ENABLE London study, an algorithm was developed to distinguish five travel modes (walk, cycle, motorised vehicle, train and stationary) using accelerometer and GPS data and the supervised machine learning tool XGBoost. We then applied this algorithm to the complete baseline and follow-up ENABLE study data sets to characterise the different modes of travel throughout the day and then examined changes between baseline and follow-up in the different travel modes. All code necessary to replicate our findings and apply our predictive model to other data sets is made available as a package of the open-source statistical software environment R (The R Foundation for Statistical Computing, Vienna, Austria). 165 Full details of the methodology and a full usage example have been published. 165,166
Development of a method to identify travel modes from accelerometry and GPS data
Methods
The method to identify travel modes from accelerometry and GPS data was developed using data collected at baseline in the ENABLE London study. Full details of this are published166 and are summarised below. Parts of this section are adapted or reproduced from Procter et al. 166 This is an Open Access article distributed in accordance with the terms of the Creative Commons Attribution (CC BY 4.0) license, which permits others to distribute, remix, adapt and build upon this work, for commercial use, provided the original work is properly cited. See: http://creativecommons.org/licenses/by/4.0/. The text below includes minor additions and formatting changes to the original text.
Accelerometry has been widely used to measure physical activity, with many different devices deployed. The most common devices in the literature are the ActiGraph, Actiheart (CamNtech, Cambridge, UK), Actical (Philips Respironics, Amsterdam, the Netherlands), activPAL (PAL Technologies Ltd, Glasgow, UK) and GeneActiv (Activinsights Ltd, Huntingdon, UK), with over half of published studies up to 2015 using the ActiGraph. 167 Many of these different devices convert raw acceleration (measured in g) into a form of activity count variable, which has then been used to classify physical activity intensity and energy expenditure. 67,168–170 However, the methods used by instrument manufacturers to convert raw acceleration into counts are often unclear. For this reason, and because the raw acceleration data contain much more information to train an algorithm than derived count variables, we have made use of the raw data in this study.
Previous work on travel mode identification has been developed from the transport perspective rather than that of physical activity, where segmentation into journeys is important to assess travel behaviour. 160,171–173 A focus on journeys often results in short periods of physically active transit behaviour, such as walking between bus stops, being identified as part of a non-active travel mode. However, for physical activity researchers, quantifying the volume and intensity of physical activity when actively travelling is an essential component of the overall purpose of a journey. Consequently, it is important to identify all data points that denote active travel, so that all time in active travel modes can be quantified. As a result, we identify the travel mode of each GPS data point (recorded every 10 seconds) without prior segmentation into journeys.
Participants in the ENABLE London study were asked to wear an accelerometer (ActiGraph GT3X+) and a GPS receiver on an elasticated belt around their waist for 7 consecutive days. Participants also completed a questionnaire to describe their travel patterns to work/place of study. They reported the specific days on which they would be travelling to work or study during the ActiGraph and GPS wear period and whether or not they commuted at the same time on each day. Reported travel modes for these journeys to and from work were underground (tube), train (over ground), bus/minibus/coach, taxi, motorcycle/moped, driving a car or a van, passenger in a car or a van, bicycle, walk, jog and other. These travel modes were recategorised into walk, cycle, vehicle (taxi, motorcycle, car/van driver, car/van passenger and bus/minibus/coach) and train (underground and overground rail). Insufficient participants consistently jogged to work for us to be able to separate ‘jog’ as an additional mode. In addition, time of leaving and arriving for each journey to and from work were collected.
Data preparation and cleaning
The raw accelerometer data were extracted as .csv files using ActiLife 6 software (ActiGraph, Florida, USA) summarised in 10-second epochs and merged by time stamp to GPS data. For the GPS data, measures of satellite signal quality were derived using the sum of the signal to noise ratio from each satellite the GPS device was connected to at each epoch. This takes into account possible obstructions to the GPS signal caused by the participant being indoors and gives a single measure of signal quality. Variations in speed and accelerometer data across a 10-second epoch were smoothed out using a 4-minute moving window calculating mean, SD, 10th and 90th percentiles of data from each accelerometer axis and speed from the GPS device. Distance from GPS time points to train lines was calculated using a combination of Meridian 2 (Ordnance Survey, Southampton, UK) rail network data for the UK and OS OpenMAP (Ordnance Survey, Southampton, UK) data for central London. 174 Distance travelled over the previous and next minute were also calculated. All variables were chosen as they were likely to differ between travel modes: vehicles and trains have higher speed than walking and cycling; walking shows greater accelerometer activity than other modes; both vehicles and trains have metal structures that may obstruct GPS signal; distance over the previous or next minute would be low if the participant is stationary.
Development of the algorithm
A training data set was created using a subset of participants (n = 326) identified from the baseline data who commuted to and from work using the same mode of transport every day and specified the time that they usually travelled for both journeys. All combined accelerometer and GPS data were extracted and exported to a Geographical Information System [ArcGIS 10.4 (ESRI, CA, USA)], and all points during the commute of each participant were manually identified to confirm the stated mode of commute. Vehicle and train journeys were confirmed by location of appropriate features (e.g. roads or rail tracks). Points in the commute clustered around a single location were classified as ‘stationary’. It should be noted that ‘stationary’ does not imply inactive, as walking within a building will appear stationary in terms of GPS signal and be classified as such using our method. Any sequences of points for which a mode of travel could not be clearly identified were excluded, for example repeated GPS signal loss. If the participant appeared to be travelling by a method other than that stated, for example they usually commute by train but on one day they do not move along a train line, then these points were not assigned a travel mode and were excluded from the training data set.
The training data set was then used to develop a model. We tested different moving window sizes (i.e. 1-, 2-, 3-, 4-, and 5-minute moving windows) and assessed how accurate each was for predicting travel mode on a subset of the training data. To test the generalisability of our fitted model to other data sets, we compared manually identified data from 10 participants from the Sedentary Time and Metabolic Health in People with type-2 diabetes mellitus study (STAMP-2) (Professor Ashley R Cooper, University of Bristol, 2018, personal communication) data set with those predicted using our model. The STAMP-2 study was a cross-sectional observational study of sedentary behaviour in adults with newly diagnosed type 2 diabetes mellitus, conducted in two English NHS foundation trusts in south-west England. Participants in one centre wore an accelerometer and GPS monitor concurrently for 7 days, providing similar data to the ENABLE London study. The advantages of testing our model on the STAMP-2 participants are that they were independent of the ENABLE London study and recruited from a city with different travel options from that of central London. They were also recently diagnosed with type 2 diabetes mellitus, were generally older and had a higher prevalence of obesity than the ENABLE London participants. A good predictive performance on this data set would demonstrate the generalisability of our algorithm to other populations.
Results
Full results are available elsewhere;166 a summary is provided here. Parts of this section are adapted or reproduced from Procter et al. 166 This is an Open Access article distributed in accordance with the terms of the Creative Commons Attribution (CC BY 4.0) license, which permits others to distribute, remix, adapt and build upon this work, for commercial use, provided the original work is properly cited. See: http://creativecommons.org/licenses/by/4.0/. The text below includes minor additions and formatting changes to the original text.
Training data
The training data set consisted of 326 participants providing 131,573 data points (365.5 hours): 66 (20%) who walked to work (12,791 points, 35.5 hours), 34 (10%) cyclists (11,607 points, 32.2 hours), 94 (29%) vehicle users (29,407 points, 81.7 hours), 132 (40%) train users (18,269 points, 50.7 hours) plus an additional 59,499 ‘stationary’ data points (165.3 hours). Approximately one-quarter of the training data (33,529 points) were used to test moving window sizes of 1, 2, 3, 4 and 5 minutes and the 4-minute moving window resulted in the highest predictive accuracy for active travel modes. The remaining 98,387 points were used to build a cross-validated model.
Model prediction
In the model cross-validation, we correctly predicted 97.3% of points and all five travel modes were predicted with high accuracy. In comparison with manually identified data (n = 21), overall accuracy was still high, with 96% of predictions correct, although this was driven by the fact that most people spent most of their time stationary (83.7% of time stationary). Cycling was least well predicted. When our model was tested on the STAMP-2 data set, we correctly predicted 96.5% of points compared with manual identification of travel modes, demonstrating that our predictions performed well considering the different participants and context. However, this was again driven by our high accuracy on stationary points, which was the dominant mode (86.8% of time stationary), and the poorest-performing predictive mode was, again, cycling.
Applying the algorithm to the ENABLE London study: baseline and 2-year follow-up findings
Methods
The algorithm developed on a subset of the ENABLE London baseline data set was applied to the full baseline and 2-year follow-up accelerometry and GPS data.
Outcomes
The primary outcome was minutes of ‘active’ travel, that is, daily minutes of walking and cycling. Secondary outcomes were vehicle, train, stationary and total minutes.
Statistical methods
The data collection and processing procedures were identical at baseline and at follow-up. Daily GPS data were processed to classify each 10-second epoch into one of five travel modes: walking, cycling, vehicle, train and stationary. Owing to the loss of GPS signal when travelling underground by tube, thus leading to gaps in the data, further work was carried out to classify some of these gaps as ‘underground’, when all of the following conditions were true:
-
GPS signal was lost within 200 m of an underground station or had the predicted travel mode ‘train’.
-
GPS signal was regained within 200 m of an underground station or had the predicted travel mode ‘train’.
-
The time gap between loss and reacquisition of the signal was between 2 minutes and 2 hours.
-
The loss and regaining of GPS signal did not take place within 200 m of the same underground station.
It should be noted that underground trains in the London transport system also run above ground, so there is potential for overlap between ‘underground’ and ‘train’ as methods of travel in our data.
The daily 10-second epoch data were summed to provide daily minutes for each travel mode and total daily GPS minutes. Walking and cycling minutes were also combined to provide a measure of ‘active travel’. This provided a data set of up to 7 days of data for each participant, with daily time spent in each travel mode. Daily data were processed to provide an average summary daily figure for each participant, using identical methods to those for the ENABLE London accelerometer data, as described in Chapter 3. Participants were asked to wear the accelerometer at the same time as the GPS monitor, and in line with accelerometry analyses, days where accelerometer wear time was less than 540 minutes (9 hours) were dropped from further analyses. For each mode of travel, average daily minutes at baseline were derived using multilevel linear regression models (level 1 was day within individual and level 2 was individual), regressing daily time on day order of wear, day of week and month of wear. This was repeated for the follow-up data. The change in average daily minutes from baseline to follow-up for each travel mode was then examined using multilevel linear regression models, where level 1 was individual and level 2 was household. For each travel mode, average daily minutes at follow-up was regressed on average daily minutes at baseline, East Village/control group, sex, age group and ethnic group as fixed effects and household as a random effect. The model coefficient for East Village/control group is thus the additional change in the East Village group adjusted for any change in the control group. This method of analysis measures change while controlling for regression to the mean, and has been shown to both minimise bias and maintain power. Checks were carried out to confirm that the distribution of residuals from the models were normally distributed. Sensitivity analyses included limiting to those who were working or studying at baseline. Imputation analyses for the main outcome of interest, ‘active travel’ (walking and cycling minutes), were carried out using multiple imputation methods using Stata Special Edition version 15 for Windows.
Results
The ENABLE London baseline cohort
At baseline, 1063 out of 1278 (83%) participants provided GPS data, of whom 991 provided ≥ 1 corresponding day with ≥ 540 minutes accelerometer wear time. Baseline characteristics for these 991 participants are shown in Table 15, overall and by housing sector. Across all the housing sectors, two-thirds were aged < 35 years, 56% were female and 52% were of white ethnic origin. Participants from the social housing sector were more likely to be female (74%), older and of black or Asian ethnic origin. Intermediate and market-rent participants were younger, equally likely to be male or female and more likely to be of white ethnic origin. These patterns are similar to those seen in the full ENABLE London cohort (see Chapter 2). Overall, the majority of the cohort (85%) were either working or studying at baseline, although this fell to two-thirds in the social housing sector and was higher (95%) in the intermediate and market-rent sectors. This is reflected in the proportions that were classified as economically inactive: 47% in the social sector and 7–9% in the intermediate and market-rent sectors. Three-quarters of the cohort used public transport to travel to or from their work or study and 50% reported some walking, cycling or jogging, which can be classified as ‘active travel’. The proportions of ‘active travel’ varied between the housing sectors: the social sector participants were less likely to walk, cycle or jog and more likely to use private transport. Table 15 also shows the time spent in different travel modes at baseline from the GPS monitors. Participants in the intermediate and market-rent sectors were broadly similar in their average daily time spent in each travel mode. In contrast, social sector participants recorded fewer walking, train and underground minutes, and higher vehicle and stationary minutes. Differences between housing sectors for time spent in different travel modes were statistically significant for all travel modes.
Characteristic | All housing sectors (N = 991) | Housing sector | p-valuea | ||
---|---|---|---|---|---|
Social (N = 351; 35%) | Intermediate (N = 446; 45%) | Market-rent (N = 194; 20%) | |||
Age (years), n (%) | |||||
16–24 | 209 (21) | 68 (19) | 82 (18) | 59 (30) | < 0.001 |
25–34 | 441 (45) | 91 (26) | 253 (57) | 97 (50) | |
35–49 | 275 (28) | 162 (46) | 93 (21) | 20 (10) | |
≥ 50 | 66 (7) | 30 (9) | 18 (4) | 18 (9) | |
Sex, n (%) | |||||
Female | 559 (56) | 260 (74) | 211 (47) | 88 (45) | < 0.001 |
Ethnic group, n (%) | |||||
White | 516 (52) | 70 (20) | 312 (70) | 134 (69) | < 0.001 |
Black | 232 (23) | 174 (50) | 44 (10) | 14 (7) | |
Asian | 151 (15) | 69 (20) | 60 (13) | 22 (11) | |
Other | 92 (9) | 38 (11) | 30 (7) | 24 (12) | |
NS-SEC, n (%) | |||||
Higher managerial/professional | 498 (51) | 47 (14) | 318 (72) | 133 (69) | < 0.001 |
Intermediate occupations | 154 (16) | 49 (14) | 70 (16) | 35 (18) | |
Routine/manual occupations | 120 (12) | 87 (25) | 25 (6) | 8 (4) | |
Economically inactive | 211 (21) | 163 (47) | 30 (7) | 18 (9) | |
Car or van in household | 357 (46) | 132 (50) | 159 (44) | 66 (41) | 0.14 |
Working or studying | 839 (85) | 232 (66) | 423 (95) | 184 (95) | < 0.001 |
Work or study away from home | 773 (92) | 213 (92) | 397 (94) | 163 (89) | 0.08 |
Mode of travel between home and work (modes not mutually exclusive), n (%) | |||||
Public transport | 590 (73) | 155 (69) | 312 (76) | 123 (69) | 0.09 |
Private car/motorbike/taxi | 113 (14) | 49 (22) | 48 (12) | 16 (9) | < 0.001 |
Walk/cycle/jog | 439 (54) | 95 (42) | 246 (60) | 98 (55) | < 0.001 |
GPS variables (minutes), mean (SD) | p-valueb | ||||
Walking | 38 (25) | 32 (26) | 41 (22) | 43 (28) | < 0.001 |
Cycling | 5 (10) | 3 (6) | 6 (11) | 6 (14) | < 0.001 |
Walking and cycling | 43 (28) | 35 (27) | 47 (25) | 49 (31) | < 0.001 |
Vehicle | 37 (38) | 46 (47) | 32 (29) | 30 (33) | < 0.001 |
Train | 14 (20) | 8 (13) | 18 (22) | 15 (24) | < 0.001 |
Underground | 15 (18) | 8 (15) | 19 (18) | 17 (17) | < 0.001 |
Stationary | 446 (194) | 500 (179) | 422 (192) | 403 (205) | < 0.001 |
Total GPS minutes | 554 (206) | 597 (185) | 538 (206) | 515 (228) | < 0.001 |
Changes in mode of travel baseline to follow-up
At follow-up, 714 out of 877 (81%) participants provided GPS data, 681 with ≥ 1 corresponding day with ≥ 540 minutes of accelerometry wear time. Longitudinal analyses were restricted to 578 participants who had valid GPS data at both baseline and follow-up.
Baseline characteristics for the 578 adults with GPS data at baseline and follow-up are shown in Table 16 by East Village/control group and housing sector. Reassuringly, age, sex, ethnicity and NS-SEC patterns were similar to those for the full 877 who were followed up (see Chapter 2). In the social housing sector, the East Village and control group were similar in age, sex and socioeconomic distributions, but the East Village group were more likely to be of black ethnic origin. In the intermediate sector, the East Village group were more likely to be younger, male, of white ethnic origin and economically active. In the market-rent sector, age, sex, ethnic group and socioeconomic status were similar in the East Village and control groups. There were no differences between the East Village group and the control group in the proportions of households who owned a car at baseline. Slightly fewer of the East Village group were working at baseline and 24% were classified as economically inactive compared with 18% of the control group. At baseline, there was greater use of public transport for travel to work or study among those who moved into East Village (p = 0.004 for all housing sectors). Use of private transport and walk/cycle/jog were similar in the East Village and control groups, although social housing sector participants were more likely to use private transport and less likely to walk/cycle/jog than intermediate and market-rent participants.
Characteristic | All housing sectors (N = 578) | p-value | Housing sector | ||||||
---|---|---|---|---|---|---|---|---|---|
Social (N = 201) | Intermediate (N = 283) | Market-rent (N = 94) | |||||||
Control (n = 285; 49%) | East Village (n = 293; 51%) | Control (n = 74; 37%) | East Village (n = 127; 63%) | Control (n = 141; 50%) | East Village (n = 142; 50%) | Control (n = 70; 74%) | East Village (n = 24; 26%) | ||
Age (years), n (%) | |||||||||
16–24 | 44 (15) | 68 (23) | 0.005 | 7 (9) | 26 (20) | 20 (14) | 29 (20) | 17 (24) | 13 (54) |
25–34 | 116 (41) | 133 (45) | 18 (24) | 34 (27) | 65 (46) | 93 (65) | 33 (47) | 6 (25) | |
35–49 | 100 (35) | 80 (27) | 44 (59) | 57 (45) | 46 (33) | 19 (13) | 10 (14) | 4 (17) | |
≥ 50 | 25 (9) | 12 (4) | 5 (7) | 10 (8) | 10 (7) | 1 (1) | 10 (14) | 1 (4) | |
Sex, n (%) | |||||||||
Female | 173 (61) | 159 (54) | 0.12 | 58 (78) | 91 (72) | 82 (58) | 57 (40) | 33 (47) | 11 (46) |
Ethnic group, n (%) | |||||||||
White | 152 (53) | 161 (55) | 0.001 | 17 (23) | 24 (19) | 87 (62) | 117 (82) | 48 (69) | 20 (83) |
Black | 48 (17) | 78 (27) | 22 (30) | 75 (59) | 21 (15) | 3 (2) | 5 (7) | 0 (0) | |
Asian | 57 (20) | 30 (10) | 29 (39) | 13 (10) | 23 (16) | 15 (11) | 5 (7) | 2 (8) | |
Other | 28 (10) | 24 (8) | 6 (8) | 15 (12) | 10 (7) | 7 (5) | 12 (17) | 2 (8) | |
NS-SEC, n (%) | |||||||||
Higher managerial/professional | 163 (57) | 130 (45) | 0.03 | 17 (23) | 16 (13) | 97 (69) | 99 (70) | 163 (70) | 15 (63) |
Intermediate occupations | 43 (15) | 56 (19) | 12 (16) | 21 (17) | 18 (13) | 29 (21) | 13 (19) | 6 (25) | |
Routine/manual occupations | 29 (10) | 33 (11) | 16 (22) | 24 (19) | 11 (8) | 9 (6) | 2 (3) | 0 (0) | |
Economically inactive | 50 (18) | 71 (24) | 29 (39) | 64 (51) | 15 (11) | 4 (3) | 6 (9) | 3 (13) | |
Car or van in household | 111 (46) | 104 (46) | 0.96 | 31 (53) | 47 (51) | 55 (46) | 49 (43) | 25 (41) | 8 (40) |
Working or studying | 245 (86) | 240 (82) | 51 (69) | 80 (63) | 128 (91) | 138 (97) | 66 (94) | 22 (92) | |
Work or study away from home | 217 (89) | 226 (94) | 0.04 | 44 (86) | 74 (92) | 118 (92) | 132 (96) | 55 (83) | 20 (89) |
Mode of travel to or from work or study (not mutually exclusive), n (%) | |||||||||
Public transport | 156 (67) | 182 (79) | 0.004 | 31 (66) | 56 (74) | 83 (67) | 110 (82) | 42 (67) | 16 (76) |
Private car/motorbike/taxi | 35 (15) | 28 (12) | 0.36 | 10 (21) | 16 (21) | 19 (15) | 10 (7) | 6 (10) | 2 (10) |
Walk/cycle/jog | 141 (61) | 128 (55) | 0.27 | 19 (40) | 40 (53) | 82 (67) | 77 (57) | 40 (63) | 11 (52) |
GPS variables (minutes), mean (SD) | |||||||||
Walking | 40 (26) | 38 (24) | 0.003 | 31 (21) | 31 (25) | 41 (24) | 43 (22) | 47 (31) | 38 (28) |
Cycle | 6 (13) | 4 (7) | 0.06 | 2 (3) | 2 (4) | 7 (14) | 5 (9) | 8 (18) | 2 (4) |
Walking and cycling | 46 (30) | 41 (26) | 0.84 | 33 (22) | 34 (26) | 48 (28) | 48 (25) | 55 (36) | 40 (28) |
Vehicle | 37 (38) | 38 (39) | 0.98 | 43 (47) | 49 (48) | 36 (32) | 29 (28) | 34 (38) | 32 (30) |
Train | 15 (21) | 15 (19) | 0.55 | 8 (12) | 9 (14) | 16 (23) | 20 (22) | 17 (25) | 12 (12) |
Underground | 14 (17) | 15 (18) | 0.58 | 12 (20) | 8 (15) | 15 (17) | 21 (18) | 16 (16) | 19 (15) |
Stationary | 440 (193) | 449 (188) | 0.73 | 507 (192) | 496 (184) | 421 (190) | 418 (179) | 407 (184) | 380 (207) |
Total GPS | 552 (207) | 558 (195) | 0.02 | 602 (201) | 596 (190) | 537 (206) | 537 (188) | 529 (210) | 482 (225) |
Table 17 shows the change in the neighbourhood perceptions and built environment variables for the East Village and control groups. Compared with the baseline data, those participants who had moved to East Village lived closer to their nearest park, had better access to public transport and lived in a more walkable area. They also reported more positive perceptions of their local area. These differences were most marked for social housing sector participants.
Measure | All housing sectors, mean (95% CI); p-value | Housing sector, mean (95% CI); p-value | ||
---|---|---|---|---|
Social | Intermediate | Market-rent | ||
Built environment characteristics | ||||
Control group | ||||
Total participants (n) | 256 | 73 | 127 | 56 |
Distance to closest park (m)a | 28 (–23 to 78);0.28 | 27 (–44 to 97);0.45 | 31 (–39 to 101);0.39 | 21 (–123 to 166);0.77 |
Access to public transport (PTAL)b | –0.1 (–0.3 to 0.1);0.35 | –0.2 (–0.5 to 0.1);0.20 | –0.1 (–0.4 to 0.3);0.63 | 0.0 (–0.5 to 0.4);0.94 |
Walkabilityc | 0.3 (0.0 to 0.6);0.09 | –0.2 (–0.7 to 0.3);0.38 | 0.5 (0.0 to 1.0);0.04 | 0.3 (–0.2 to 0.9);0.25 |
Land use mixd | 0.02 (0.00 to 0.04);0.08 | –0.02 (–0.05 to 0.01);0.22 | 0.04 (0.01 to 0.08);0.03 | 0.02 (–0.02 to 0.06);0.25 |
Residential densitye | 1.8 (1.0 to 2.7);< 0.001 | 1.2 (0.2 to 2.3);0.02 | 2.1 (0.7 to 3.5);0.003 | 2.0 (0.2 to 3.8);0.03 |
Street connectivityf | –0.1 (–0.2 to 0.1);0.38 | –0.2 (–0.4 to 0.0);0.13 | 0.0 (–0.2 to 0.2);0.93 | –0.1 (–0.4 to 0.2);0.63 |
East Village group | ||||
Total participants (n) | 277 | 124 | 130 | 23 |
Distance to closest park (m)a | –547 (–597 to –498);< 0.001 | –463 (–529 to –397);< 0.001 | –602 (–673 to –530);< 0.001 | –693 (–952 to –435);< 0.001 |
Access to public transport (PTAL)b | 1.2 (0.9 to 1.5);< 0.001 | 2.0 (1.5 to 2.5);< 0.001 | 0.7 (0.2 to 1.1);0.007 | 0.3 (–0.9 to 1.5);0.61 |
Walkabilityc | 2.4 (2.1 to 2.7);< 0.001 | 2.7 (2.3 to 3.1);< 0.001 | 2.0 (1.5 to 2.5);< 0.001 | 2.4 (0.4 to 4.3);0.02 |
Land use mixd | 0.38 (0.36 to 0.41);< 0.001 | 0.40 (0.37 to 0.43);< 0.001 | 0.37 (0.34 to 0.41);< 0.001 | 0.35 (0.23 to 0.47);< 0.001 |
Residential densitye | 14.4 (12.8 to 16.0);< 0.001 | 14.7 (12.3 to 17.0);< 0.001 | 13.3 (11.0 to 15.6);< 0.001 | 19.1 (12.4 to 25.9);< 0.001 |
Street connectivityf | –1.1 (–1.2 to –0.9);< 0.001 | –0.9 (–1.1 to –0.7);< 0.001 | –1.2 (–1.4 to –1.0);< 0.001 | –1.2 (–2.0 to –0.4);0.006 |
Neighbourhood perceptionsg | ||||
Control group | ||||
Total participants (n) | 285 | 74 | 141 | 70 |
Crime score | 0.6 (0.1 to 1.0);0.02 | 1.3 (0.4 to 2.2);0.005 | 0.0 (–0.7 to 0.7);1.00 | 1.0 (0.0 to 1.9);0.04 |
Quality score | 0.7 (0.2 to 1.2);0.01 | 1.5 (0.6 to 2.4);0.001 | 0.1 (–0.6 to 0.9);0.73 | 0.9 (0.0 to 1.9);0.06 |
East Village group | ||||
Total participants (n) | 293 | 127 | 142 | 24 |
Crime score | 4.7 (4.1 to 5.2);< 0.001 | 6.1 (5.2 to 7.1);< 0.001 | 3.5 (2.8 to 4.2);< 0.001 | 3.8 (1.7 to 5.9);0.001 |
Quality score | 7.0 (6.4 to 7.5);< 0.001 | 7.9 (7.0 to 8.7);< 0.001 | 6.3 (5.5 to 7.1);< 0.001 | 6.5 (5.1 to 7.9);< 0.001 |
The effect of moving to East Village on the time spent in different travel modes is shown in Table 18. Overall, there was little change in participants’ walking or cycling minutes. However, vehicle minutes decreased overall by 7–8 minutes per day, with greater effects in the intermediate housing sector (10 minutes’ decrease, 95% CI –17 to –2 minutes’ decrease; p = 0.01), and time spent travelling by underground increased by 3–4 minutes, particularly in the market-rent sector (12 minutes, 95% CI 4 to 19 minutes; p = 0.001). There were large decreases in both stationary and total minutes; however, this was as a result of issues with the GPS signal being blocked by East Village housing, discovered after follow-up had been completed.
Change | All housing sectors (N = 578), effect (95% CI); p-value | Housing sector, effect (95% CI); p-value | ||
---|---|---|---|---|
Social (N = 201) | Intermediate (N = 283) | Market-rent (N = 94) | ||
Daily walking minutes | ||||
Model 1a | –1.3 (–4.9 to 2.3); 0.49 | –0.3 (–6.4 to 5.8); 0.92 | 0.1 (–5.1 to 5.3); 0.97 | –4.9 (–15.5 to 5.7); 0.36 |
Model 2b | –1.7 (–5.4 to 2.0); 0.36 | –1.8 (–8.4 to 4.8); 0.59 | –1.9 (–7.5 to 3.8); 0.52 | –4.2 (–15.4 to 7.0); 0.46 |
Model 3c | –1.4 (–5.3 to 2.5); 0.48 | |||
Daily cycling minutes | ||||
Model 1a | 0.7 (–0.8 to 2.2); 0.34 | 0.5 (–0.5 to 1.5); 0.37 | 1.6 (–0.9 to 4.1); 0.21 | 1.9 (–3.3 to 7.0); 0.48 |
Model 2b | 0.7 (–0.8 to 2.2); 0.36 | 0.3 (–0.8 to 1.4); 0.58 | 1.0 (–1.6 to 3.7); 0.44 | 1.9 (–3.6 to 7.4); 0.50 |
Model 3c | 1.1 (–0.5 to 2.7); 0.17 | |||
Daily walk plus cycle minutes | ||||
Model 1a | –0.7 (–4.7 to 3.3); 0.75 | 0.1 (–6.2 to 6.4); 0.98 | 1.6 (–4.2 to 7.3); 0.59 | –2.2 (–15.4 to 11.0); 0.74 |
Model 2b | –1.1 (–5.2 to 2.9); 0.59 | –1.5 (–8.4 to 5.4); 0.68 | –0.9 (–7.1 to 5.4); 0.78 | –2.4 (–16.0 to 11.3); 0.74 |
Model 3c | –0.4 (–4.7 to 3.8); 0.85 | |||
Vehicle minutes | ||||
Model 1a | –7.6 (–13.0 to –2.2); 0.01 | –7.2 (–18.9 to 4.5); 0.23 | –11.4 (–18.2 to –4.7); < 0.001 | –5.9 (–18.2 to 6.4); 0.35 |
Model 2b | –7.0 (–12.5 to –1.5); 0.01 | –6.2 (–18.9 to 6.6); 0.34 | –9.6 (–16.9 to –2.2); 0.01 | –6.9 (–19.9 to 6.1); 0.30 |
Model 3c | –8.3 (–14.0 to –2.5); 0.01 | |||
Train minutes | ||||
Model 1a | –1.4 (–4.2 to 1.4); 0.32 | –0.1 (–3.9 to 3.7); 0.95 | –1.3 (–5.4 to 2.8); 0.53 | 2.1 (–7.5 to 11.7); 0.67 |
Model 2b | –1.7 (–4.6 to 1.1); 0.23 | –1.7 (–5.8 to 2.4); 0.41 | –1.5 (–6.0 to 3.0); 0.51 | 0.2 (–10.1 to 10.6); 0.97 |
Model 3c | –0.8 (–3.7 to 2.2); 0.62 | |||
Underground minutes | ||||
Model 1a | 2.6 (0.0 to 5.1); 0.05 | 2.9 (–0.9 to 6.6); 0.14 | 2.2 (–1.8 to 6.2); 0.27 | 10.9 (4.1 to 17.6); 0.002 |
Model 2b | 2.6 (0.1 to 5.2); 0.04 | 4.0 (–0.1 to 8.1); 0.06 | 1.6 (–2.7 to 6.0); 0.46 | 11.5 (4.4 to 18.6); 0.001 |
Model 3c | 3.9 (1.2 to 6.5); 0.005 | |||
Stationary minutes | ||||
Model 1a | –248 (–278 to –218); < 0.001 | –346 (–393 to –299); < 0.001 | –199 (–243 to –155); < 0.001 | –143 (–229 to –56); 0.001 |
Model 2b | –248 (–279 to –217); < 0.001 | –354 (–405 to –304); < 0.001 | –205 (–252 to –157); < 0.001 | –127 (–217 to –38); 0.01 |
Model 3c | –247 (–279 to –214); < 0.001 | |||
Total minutes | ||||
Model 1a | –256 (–289 to –223); < 0.001 | –347 (–400 to –295); < 0.001 | –211 (–259 to –162); < 0.001 | –139 (–234 to –44); 0.004 |
Model 2b | –255 (–289 to –222); < 0.001 | –357 (–412 to –302); < 0.001 | –217 (–269 to –164); < 0.001 | –126 (–223 to –29); 0.01 |
Model 3c | –253 (–288 to –217); < 0.001 |
Discussion
Using the ENABLE London study accelerometry and GPS data, we have developed an accurate predictive algorithm that identifies five travel modes, including the active travel modes walking and cycling, and identifies each travel mode correctly > 90% of the time. Our levels of accuracy in cross-validation outperform recent similar studies. 160,162 The models developed here are freely available to apply to similar data in the statistical software environment R. 165 We have applied this algorithm to the complete baseline and 2-year follow-up ENABLE London data sets to characterise the travel patterns of a large group of adults in London, identifying time spent in different modes of travel. Furthermore, we have examined changes in time spent in different travel modes following a move to East Village. Despite the built environment variables showing that East Village is a more walkable area, we found no change in the time spent walking or cycling compared with those living elsewhere. However, there was some suggestion that vehicle travel had decreased, particularly in the intermediate housing sector, and that underground travel had increased, more so in the market-rent sector.
Strengths of the algorithm developed
Our study has a number of important strengths. Applying the algorithm to the STAMP-2 data set resulted in similar levels of accuracy of travel mode prediction within a very different group of participants living in a different environmental setting. This finding suggests that our method may be generalisable to other data sets and could be used by other researchers without the time-consuming steps of creating new training data. However, until more robust tests have been completed, we would recommend a manual identification checking stage similar to our methods to verify the generalisability of the method.
We exhibit similar levels of accuracy to the personal activity location measurement system (PALMS),173 which is a freely available method to process physical activity data. The purpose of the output is somewhat different though, with PALMS identifying journeys and our method identifying each data point. The preferred method will depend on the research question. One advantage of our R package that the server-based system in PALMS does not have is that it can be run on a researcher’s own machine. Data used for the present (and other similar) analyses are subject to strict data privacy and ethical conditions. Running the analyses on a researcher’s own machine, rather than uploading to a server for remote processing, can help avoid problems related to data privacy. Furthermore, our method is open source, which means that all code is freely available online. 165 As a result, other researchers can suggest edits and improvements and contribute to the development of our method, and its utility to the research community.
The prediction of active travel modes should complement existing analyses of physical activity using accelerometers. Accelerometers have been used with great effect to objectively quantify activity, but they are not without limitations. A well-known problem of traditional physical activity analyses using accelerometers is that cycling is not recognised as a form of MVPA because cycling generates relatively low readings on a waist-worn accelerometer compared with other active modes. Identification of cycling from combined accelerometer and GPS data will allow better quantification of cycling as a form of physical activity. Traditional physical activity analyses will still miss cycling as a form of activity whereas our method will quantify it, albeit with moderate precision. Furthermore, assessment of active travel using our method will help to understand how people are active. For example, activity at a single location, such as at home or at the gym, will not be classed as active travel using our method but as a stationary travel mode; therefore, a participant may show high levels of overall physical activity but low levels of active travel. Incorporation of this extra information will help to understand participants’ overall physical activity patterns. For example, if a participant shows increased physical activity but not active travel, it is likely that they have increased their activity at locations such as at home or at the gym. If we see no change in physical activity but an increase in active travel, then participants have replaced some of their physical activity at a location with active travel.
Limitations of the algorithm developed
It is unsurprising that our accuracy scores are lower when compared with manual identification of both participants within this study and from elsewhere than in cross-validation of the training data set. Identification is most likely to be accurate during journeys of a consistent mode, which is how the training data were defined. In full days of data there are likely to be other more ambiguous forms of movement that may be short in duration and, therefore, difficult to identify, or which do not fully represent one of the forms of travel we have included. Our model performs least well for the detection of cycling and, therefore, is probably overfitted to our training data. As a result, we would recommend manual checking of cycling data to improve accuracy. In this study, cycling represents a relatively small amount of total time; therefore, manual checking of this subset represents a much smaller time investment than in the full analysis.
Visual inspection of the data in the GIS revealed that much of the disagreement between prediction and manual classification was found at the start and the end of journeys. This highlights the challenge of identifying modal shift, that is when to switch from one mode to another. This is a limitation of the current method and all other indirect methods. However, the strength of identifying individual data points rather than full journeys is that the imprecision in identifying modal shift leads to small numbers of misclassified points, rather than entire misclassified journeys. Small numbers of misclassified points will only have a small effect on total time in each travel mode. Our inaccuracy at the start and end of trips means that any prediction to a data set where many short-duration trips are expected would be predicted to yield lower accuracy. Conversely, within a data set containing longer journeys, travel mode should be able to be identified with greater precision.
It is also worth highlighting that some disagreement within our test data sets may not be true errors. For example, if a participant is stationary but on a train it is questionable whether they should be classified as using a train or stationary; this may not matter as long as the rule is applied consistently. However, when comparing the predicted and manually derived methods this causes disagreement because during manual classification stationary points on train lines were termed stationary (e.g. standing at a train station), yet the algorithm identifies them as ‘train’. A number of the misclassifications between our manual identification and predicted data sets may, therefore, be open to interpretation and may not be true misclassifications.
Using the model
First, we were only able to identify the active modes of walking and cycling: there is no consideration of running or any other activity. This limitation is based on our study sample, where no commuters consistently used these modes so we could not include them in our training data. However, walking and cycling represent the most frequently used active transport modes and other modes were rarely reported by our participants. Any form of running will most likely be identified as walking using our method because of the high acceleration that running causes on an accelerometer, and so will still be identified as active travel. We therefore feel that this limitation will have little impact on our study, although we would recommend caution in applying our method to a data set where a large quantity of running is expected. Second, we have demonstrated our capacity to identify major travel modes but were not able to discriminate car travel from public transport. Consequently, if a study is attempting to quantify the use of public transport then the current method is inadequate. To address this, we have developed a second model that discriminates bus travel. However, this leads to reduced overall accuracy, because the pattern of speed/activity can be confused between buses and other vehicles. Further work in this area would need to assess the generalisability of our methods. Third, although we assess different geographical contexts, both are in the UK. We do not know how well the method would perform elsewhere in the world, where other transport options may be available. Furthermore, we have only tested the current model on adults, and further research could assess how well our model performs on older adults or children, to potentially be of use in a wider group of studies.
Applying the model to the ENABLE London baseline and follow-up data
Using the algorithms developed on a subset of the baseline ENABLE London GPS and accelerometry data sets, we have been able to characterise the travel use of a large group of adults in London and identify the time spent in different modes of travel. Furthermore, we have examined changes in the time spent in different travel modes following a move to East Village. However, despite the built environment variables showing that East Village is a more walkable area, we found no change in the time spent walking or cycling compared with those living elsewhere. However, there was some suggestion that vehicle travel had decreased, particularly in the intermediate housing sector, and that underground travel had increased, more so in the market-rent sector.
Strengths of applying the model to the ENABLE London data
The East Village neighbourhood was designed to encourage active living. Restrictions are placed on parking facilities in East Village but it has good public transport links, being close to Stratford station with both overground links and underground links. Half of the cohort were living in East Village at 2-year follow-up and half had either stayed at their baseline address or moved elsewhere. Following up the same individuals 2 years later enabled us to examine change in behaviour at an individual level with participants acting as their own controls. The GPS monitors were worn at the same time as the accelerometers, which provided direct and time-stamped comparisons of physical activity and location. GPS monitors rely on having good signal links with satellite receivers and the signals can be blocked by some buildings and when travelling underground, but the concurrent use of accelerometry data helped to identify different modes of travel and daily wear time. The eligibility criteria for moving into East Village, set externally by the housing association and companies, meant that we could examine differences between three housing sectors: social, intermediate and market-rent. Eligibility for these different housing sectors was based on income, which provided a measure of socioeconomic status. Finally, our method of analysis, regressing follow-up-values on baseline values, allows direct comparison of change in outcomes between the East Village and the control groups and minimised the issues of regression to the mean.
Limitations
Lack of adequate wear time led to a reduced sample of 548 participants with GPS data at both baseline and follow-up. However, baseline demographic characteristics were similar to the full cohort. After data collection had been completed and the GPS data were being processed, it was discovered that the East Village housing blocked the transmission of the GPS signal leading to low GPS-recorded time in the East Village group. However, as the accelerometers were worn at the same time as the GPS monitors and provided an estimate of wear time, we were able to use this as a proxy for wear time for the GPS monitor. Additionally, the decrease in total GPS minutes was almost identical to the decrease in stationary time in the East Village group, confirming that the GPS time lost was most likely to be spent stationary by participants in their East Village homes.
Conclusions
In summary, we have developed a method to identify travel modes from accelerometer, GPS and GIS data for the ENABLE London study, which successfully predicts > 90% of points tested in a range of contexts. This method can be of use to complement existing analyses of physical activity data and assess active travel alongside physical activity. All code necessary to replicate the analysis, apply the method to other data sets or predict from our models to other data sets is provided to facilitate usage by other researchers.
Chapter 6 Mental health, well-being and neighbourhood perceptions
Background
In this chapter, the effect of change in the built environment on the mental health (depression and anxiety) and well-being of the ENABLE London study cohort is examined, overall and by housing sector. This chapter also examines whether or not change in neighbourhood perceptions is associated with change in mental health and well-being.
Introduction
Depression and anxiety are recognised as global causes of disease. 176 Depressive disorders are one of the leading causes of years lived with disability and a leading cause of disability-adjusted life-years. 176 Globally, > 30 million people, 4.4% of the world population,176 are estimated to be affected by depression. This number is increasing, especially in low-income countries where common mental disorders (CMDs) such as depression and anxiety are more likely to occur as a result of population increases and people living longer. 176,177 These figures highlight the importance of non-fatal health outcomes in the measurement of disease burden. 178 In the UK, CMDs are the most prevalent health condition. 179 Around one in six people in England report depression and/or anxiety at any one time. 180 Subjective well-being (how people feel about their lives) is recognised to be a critical measure of the overall mental health status of the population and an important marker of quality of life,181 a key indicator of inequalities in health. 182
There is a growing recognition of the importance of the local built environment to mental health. Increasing evidence from both cross-sectional and longitudinal studies suggests that both structural and social attributes of a neighbourhood can affect the mental health of residents. 183–185 Negative perceptions of the neighbourhood (e.g. perceived level of accessibility to green space, more crime, feeling unsafe, less walkability) have been found to be associated with depression, anxiety,186–188 and both physiological and self-reported measures of stress. 189 Positive perceptions are associated with higher rates of physical activity, which, in turn, may reduce depression and cardiovascular risk with potential benefits for both physical and mental health. 190
The World Health Organization reports that through healthier environments premature death and disease can be prevented, and estimates that 23% of global mortality is caused by modifiable environmental factors. 191 Environments that are healthy and sustainable (i.e. well-designed, accessible features such as walkability, transport and green space) provide residents with positive perceptions of where they live, which can lead to better mental health and enhanced well-being, and improve both physiological and self-reported measures of stress. 189 Positive perceptions are associated with higher rates of physical activity, which, in turn, may reduce depression and cardiovascular risk and, therefore, have potential benefits for both physical and mental health. 190 The influence of the built environment on mental health may be stronger among those who are more socioeconomically disadvantaged188 and who often reside in poor-quality environments192–194 characterised by lower perceived safety, access to fewer shops and leisure facilities, and higher levels of crime;195,196 however, the evidence is weak.
The ENABLE London study provided a unique opportunity to evaluate the effect of moving into accommodation based on active design principles on mental health and well-being outcomes.
Methods
Outcomes
Depression, anxiety and well-being
Depression and anxiety at baseline and follow-up were measured using the Hospital Anxiety and Depression Scale; seven items assessed depression and seven items assessed anxiety. 57 Each item was scored between 0 and 3, which provided a maximum score of 21 for each subscale. Higher scores indicated the presence of depression and anxiety. One missing response on any of the subscales was imputed using the mean from the sum of scores in each subscale. 197 Three questions examined well-being: ‘Overall, how satisfied are you with your life nowadays?’, ‘Overall, to what extent do you feel that the things you do in life are worthwhile?’ and ‘Overall, how happy did you feel yesterday?’. Each item was rated on a scale from 1 (not at all) to 10 (extremely), with higher scores indicating positive well-being. 198
Neighbourhood perceptions
Two neighbourhood perception scales were developed at baseline, crime-free and quality, using exploratory factor analysis on 14 neighbourhood perception items included in the questionnaire64 (see Chapter 3 for further details). Scores for each participant were derived for each scale; higher scores indicated positive neighbourhood perceptions, that is that neighbourhoods were crime-free and were of better neighbourhood quality. The same items were used to derive scores at follow-up.
Statistical methods
Baseline
Univariate analyses examined baseline differences in the proportion of participants who reported depression, anxiety and low levels of well-being, and baseline differences in mean neighbourhood perception scores by housing sector and between East Village and control groups. Multilevel logistic regression models were used to examine the associations between the housing sector and the binary outcomes of depression, anxiety and well-being, which allowed for household clustering as a random effect (model A), with adjustment for covariates including age, sex and ethnicity (model B), marital status (model C), educational level (model D) and health status (i.e. ‘LLI’, model E). Analyses were restricted to 1213 (95%) participants with complete data on all outcomes and covariates at baseline.
Follow-up
A total of 92% (n = 808/877) of participants had complete data on all outcomes of interest at follow-up. Multilevel linear regression models examined change in the levels of depression, anxiety, well-being and neighbourhood perceptions, comparing those who moved to East Village with those who did not, for the cohort as a whole and in stratified analyses by housing sector. Analyses were adjusted for baseline household clustering, as well as age group, sex and ethnic group (model 1). Associations between change in mental health/well-being outcomes and change in neighbourhood perception scores were also examined.
Results
At baseline, 14% of the cohort were classified as depression cases, 31% reported anxiety and one-quarter reported lower levels of well-being (see Table 1). A gradient was observed in the depression levels across housing sectors, where a higher proportion of social housing participants reported depression (21%), than intermediate (10%) and market-rent groups (9%). Similar proportions of participants in social housing and market-rent groups reported anxiety (33%), with a lower proportion in the intermediate group (28%). A gradient was also observed in low levels of life satisfaction across the housing sectors: 33% of social housing participants reported low levels of life satisfaction, compared with 22% of intermediate and 19% of market-rent participants. Low levels of feeling that life is worthwhile and happiness were reported similarly across the housing sectors.
Baseline mental health and well-being
Logistic regression analyses showed appreciably higher levels of depression among participants seeking social housing than in participants from the intermediate group [odds ratio (OR) 2.5, 95% CI 1.6 to 3.9] (Table 19, model A). The ORs were similar after adjustment for age, sex, ethnicity (model B) and marital status (model C), but marginally attenuated after adjustment for educational level (model D) and health status (model E). Levels of depression were marginally lower in the market-rent group than in the intermediate group for model A but almost identical in models B to E (see Table 19). The social housing sector were more likely to be anxious than those seeking intermediate housing (OR 1.3, 95% CI 0.9 to 1.8; model A) and ORs were slightly stronger after adjustment for age, sex, ethnicity (model B) and other covariates (models C to E). Levels of anxiety were also higher in the market-rent group than in the intermediate group, with similar ORs (OR 1.3, 95% CI 0.9 to 2.0; model A), which remained after adjustment for covariates (models B to E). Those in the social housing sector were more likely to report lower levels of life satisfaction than those participants seeking intermediate housing (OR 1.7, 95% CI 1.2 to 2.3), although this was progressively weakened by adjustment (models B to E) and was no longer statistically significant. Similarly, there were no significant differences in low levels of satisfaction between market-rent and intermediate groups. Low feelings such as ‘things you do in life are worthwhile’ were marginally higher in social housing and market-rent groups than in those seeking intermediate housing, but differences were not statistically significant and not materially affected by adjustment (models A to E). Low levels of happiness were higher in the social group than in the intermediate housing sector (model A, OR 1.3, 95% CI 1.0 to 1.8), and in the market-rent group compared with the intermediate housing sector (model A, OR 1.5, 95% CI 1.0 to 2.2), which remained similar after adjustment for sociodemographic factors (models B to D) and health status (model E).
Outcome by housing sector | Model, OR (95% CI); p-value | ||||
---|---|---|---|---|---|
A | B | C | D | E | |
Depression case | |||||
Intermediate | 1.00 | 1.00 | 1.00 | 1.00 | 1.00 |
Social housing | 2.50 (1.61 to 3.87); < 0.001 | 2.32 (1.37 to 3.94); 0.002 | 2.34 (1.37 to 3.99); 0.002 | 1.95 (1.07 to 3.54); 0.03 | 1.89 (1.02 to 3.48); 0.04 |
Market-rent | 0.85 (0.47 to 1.54); 0.59 | 0.99 (0.53 to 1.83); 0.96 | 0.98 (0.53 to 1.82); 0.95 | 0.99 (0.53 to 1.85); 0.97 | 1.04 (0.55 to 1.97); 0.90 |
Anxiety case | |||||
Intermediate | 1.00 | 1.00 | 1.00 | 1.00 | 1.00 |
Social housing | 1.27 (0.92 to 1.75); 0.15 | 1.45 (0.99 to 2.13); 0.06 | 1.47 (1.00 to 2.16); 0.05 | 1.45 (0.94 to 2.25); 0.09 | 1.38 (0.89 to 2.13); 0.15 |
Market-rent | 1.34 (0.90 to 2.00); 0.15 | 1.30 (0.88 to 1.94); 0.19 | 1.28 (0.86 to 1.90); 0.23 | 1.29 (0.87 to 1.92); 0.21 | 1.33 (0.89 to 1.98); 0.16 |
Low levels of life satisfaction | |||||
Intermediate | 1.00 | 1.00 | 1.00 | 1.00 | 1.00 |
Social housing | 1.66 (1.20 to 2.30); 0.002 | 1.44 (0.98 to 2.12); 0.06 | 1.41 (0.96 to 2.09); 0.08 | 1.32 (0.85 to 2.04); 0.22 | 1.25 (0.81 to 1.94); 0.31 |
Market-rent | 0.80 (0.53 to 1.23); 0.32 | 0.82 (0.53 to 1.26); 0.36 | 0.78 (0.50 to 1.20); 0.26 | 0.78 (0.50 to 1.20); 0.26 | 0.80 (0.52 to 1.24); 0.31 |
Low levels of feeling that life is worthwhile | |||||
Intermediate | 1.00 | 1.00 | 1.00 | 1.00 | 1.00 |
Social housing | 1.33 (0.97 to 1.81); 0.08 | 1.35 (0.93 to 1.97); 0.11 | 1.36 (0.93 to 2.00); 0.12 | 1.20 (0.78 to 1.85); 0.41 | 1.15 (0.74 to 1.78); 0.54 |
Market-rent | 1.15 (0.78 to 1.71); 0.48 | 1.13 (0.76 to 1.69); 0.54 | 1.09 (0.73 to 1.64); 0.67 | 1.09 (0.73 to 1.64); 0.66 | 1.12 (0.75 to 1.68); 0.58 |
Low levels of happiness | |||||
Intermediate | 1.00 | 1.00 | 1.00 | 1.00 | 1.00 |
Social housing | 1.33 (0.96 to 1.84); 0.09 | 1.41 (0.96 to 2.08); 0.08 | 1.38 (0.94 to 2.04); 0.10 | 1.33 (0.86 to 2.06); 0.20 | 1.29 (0.83 to 2.01); 0.25 |
Market-rent | 1.48 (0.99 to 2.20); 0.05 | 1.51 (1.01 to 2.24); 0.04 | 1.46 (0.98 to 2.18); 0.06 | 1.48 (0.99 to 2.21); 0.05 | 1.50 (1.01 to 2.24); 0.05 |
Neighbourhood perceptions
There were consistent inverse associations between neighbourhood perceptions and all mental health and well-being outcomes, where better perceptions were associated with poorer mental health and well-being scores (see Report Supplementary Material 21). Hence, we examined the impact of further adjustment of baseline housing sector differences in depression, anxiety and well-being for neighbourhood perception scores (Table 20). Additional adjustment for neighbourhood perceptions of crime (see model 2, Table 20) and quality (see model 3, Table 20) weakened the higher levels of depression and other marginally higher adverse mental health/well-being outcomes among social housing participants compared with intermediate participants, suggesting that neighbourhood perceptions are a partial explanation for these housing sector differences. However, adjustment for neighbourhood perceptions had no effect on the mental health/well-being associations between market-rent and intermediate participants (see Table 20).
Outcome by housing sector | Model, OR (95% CI); p-value | ||
---|---|---|---|
1 | 2 | 3 | |
Depression case | |||
Intermediate | 1.00 | 1.00 | 1.00 |
Social housing | 1.89 (1.02 to 3.48); 0.04 | 1.73 (0.93 to 3.21); 0.08 | 1.57 (0.85 to 2.91); 0.15 |
Market-rent | 1.04 (0.55 to 1.97); 0.90 | 1.05 (0.55 to 1.98); 0.89 | 1.05 (0.55 to 1.98); 0.89 |
Anxiety case | |||
Intermediate | 1.00 | 1.00 | 1.00 |
Social housing | 1.38 (0.89 to 2.13); 0.15 | 1.20 (0.78 to 1.87); 0.41 | 1.23 (0.79 to 1.90); 0.363 |
Market-rent | 1.33 (0.89 to 1.98); 0.16 | 1.32 (0.89 to 1.96); 0.16 | 1.32 (0.89 to 1.96); 0.16 |
Low levels of life satisfaction | |||
Intermediate | 1.00 | 1.00 | 1.00 |
Social housing | 1.25 (0.81 to 1.94); 0.311 | 1.13 (0.72 to 1.77); 0.60 | 1.02 (0.65 to 1.61); 0.92 |
Market-rent | 0.80 (0.52 to 1.24); 0.31 | 0.80 (0.51 to 1.24); 0.31 | 0.79 (0.51 to 1.24); 0.30 |
Low levels of worthwhile | |||
Intermediate | 1.00 | 1.00 | 1.00 |
Social housing | 1.15 (0.74 to 1.78); 0.54 | 1.06 (0.68 to 1.65); 0.80 | 0.97 (0.62 to 1.51); 0.88 |
Market-rent | 1.12 (0.75 to 1.68); 0.58 | 1.12 (0.74 to 1.68); 0.59 | 1.11 (0.74 to 1.67); 0.61 |
Low levels of happiness | |||
Intermediate | 1.00 | 1.00 | 1.00 |
Social housing | 1.29 (0.83 to 2.01); 0.25 | 1.17 (0.75 to 1.83); 0.50 | 1.07 (0.69 to 1.66); 0.77 |
Market-rent | 1.50 (1.01 to 2.24); 0.045 | 1.51 (1.00 to 2.25); 0.047 | 1.50 (1.01 to 2.22); 0.047 |
A comparison of the baseline characteristics of those who subsequently moved to East Village with those of people who did not (Table 21) showed little difference in mental health and well-being outcomes, overall and by housing sector. The exception was that there were higher median anxiety scores in the control group than in the East Village group among social housing participants and conversely higher levels in the East Village group than in the control group among the intermediate group. There were also baseline differences in neighbourhood perceptions by subsequent control and intervention groups. The overall baseline crime-free and quality scores were markedly higher in the control group than in those who subsequently moved to East Village; for quality, these differences were also evident by housing sector.
Outcomes/perceptions | All housing sectors, median (IQR) | Housing sector, median (IQR) | ||||||||||
---|---|---|---|---|---|---|---|---|---|---|---|---|
Social | Intermediate | Market-rent | ||||||||||
Control (N = 402) | East Village (N = 406) | p-valuea | Control (N = 109) | East Village (N = 192) | p-valuea | Control (N = 189) | East Village (N = 171) | p-valuea | Control (N = 104) | East Village (N = 43) | p-valuea | |
Mental health and well-being | ||||||||||||
Depressionb | 3.5 (1.2–5.8) | 3.5 (1.2–5.8) | 0.71 | 4.7 (2.3–7.0) | 4.7 (2.3–7.0) | 0.74 | 3.5 (1.2–5.8) | 3.5 (1.2–4.7) | 0.34 | 2.3 (1.2–4.7) | 2.3 (1.2–3.5) | 0.46 |
Anxietyb | 6.0 (4.0–8.0) | 5.9 (3.0–8.0) | 0.71 | 6.0 (4.0–9.0) | 5.0 (3.0–8.0) | 0.03 | 5.0 (3.0–7.0) | 6.0 (4.0–9.0) | 0.02 | 7.0 (4.0–9.0) | 5.0 (3.0–8.0) | 0.29 |
Life satisfactionc | 7.0 (7.0–8.0) | 7.0 (6.0–8.0) | 0.94 | 7.0 (6.0–9.0) | 7.0 (6.0–9.0) | 0.64 | 7.0 (7.0–8.0) | 7.0 (6.0–8.0) | 0.40 | 7.0 (7.0–8.0) | 7.0 (7.0–8.0) | 0.72 |
Worthwhilec | 8.0 (7.0–9.0) | 8.0 (7.0–9.0) | 0.50 | 8.0 (7.0–9.0) | 8.0 (7.0–10.0) | 0.21 | 8.0 (7.0–9.0) | 8.0 (7.0–9.0) | 0.37 | 8.0 (7.0–8.0) | 7.0 (7.0–8.0) | 0.60 |
Happyc | 8.0 (6.0–9.0) | 8.0 (6.0–9.0) | 0.40 | 8.0 (6.0–9.0) | 8.0 (6.0–9.0) | 0.54 | 8.0 (7.0–9.0) | 8.0 (6.0–8.0) | 0.08 | 7.0 (6.0–8.0) | 7.0 (6.0–9.0) | 0.72 |
Neighbourhood perceptions | ||||||||||||
Crimed | 3.0 (0.0–5.0) | 2.0 (–2.0–5.0) | 0.001 | 1.0 (–2.0–4.0) | 0.0 (–3.0–3.0) | 0.08 | 3.0 (1.0–6.0) | 3.0 (0.0–6.0) | 0.45 | 4.0 (1.0–5.5) | 4.0 (2.0–7.0) | 0.38 |
Qualitye | 5.0 (2.0–8.0) | 3.0 (–1.0–6.0) | < 0.001 | 4.0 (1.0–7.0) | 2.0 (–2.0–5.0) | <0.001 | 5.0 (2.0–8.0) | 4.0 (0.0–7.0) | 0.02 | 5.0 (3.0–9.0) | 4.0 (1.0–6.0) | 0.05 |
Follow-up
Moving to East Village was associated with small improvements in depression and anxiety scores (except for the social housing sector who showed a slight increase in anxiety), although none of these effects was statistically significant (Table 22). Although moving to East Village was also associated with modest improvements in well-being scores, none of the effects was statistically significant, except for life satisfaction among the intermediate sector (0.34, 95% CI 0.04 to 0.65). However, moving to East Village was strongly associated with improved neighbourhood perceptions of a crime-free and better quality neighbourhood, both overall and by housing sector. Notably, the largest effect size for improved perceptions of a crime-free neighbourhood associated with moving to East Village was observed among the social housing group (see Table 22).
Outcome | All housing sectors (N=808), difference (95% CI); p-value | Housing sector, difference (95% CI); p-value | ||
---|---|---|---|---|
Social (N = 301) | Intermediate (N = 360) | Market-rent (N = 147) | ||
Depression | ||||
Model 1a | –0.26 (–0.65 to 0.14); 0.20 | –0.35 (–1.10 to 0.40); 0.36 | –0.26 (–0.80 to 0.29); 0.35 | 0.00 (–0.83 to 0.82); 0.99 |
Model 2b | –0.18 (–0.59 to 0.22); 0.38 | –0.12 (–0.87 to 0.63); 0.76 | –0.16 (–0.74 to 0.42); 0.59 | 0.07 (–0.79 to 0.93); 0.87 |
Anxiety | ||||
Model 1a | –0.15 (–0.61 to 0.32); 0.54 | 0.45 (–0.44 to 1.34); 0.32 | –0.49 (–1.10 to 0.13); 0.12 | –0.45 (–1.49 to 0.59); 0.39 |
Model 2b | –0.11 (–0.59 to 0.37); 0.65 | 0.46 (–0.47 to 1.39); 0.33 | –0.45 (–1.10 to 0.21); 0.18 | –0.44 (–1.45 to 0.57); 0.39 |
Life satisfaction | ||||
Model 1a | 0.19 (–0.04 to 0.41); 0.10 | 0.06 (–0.38 to 0.50); 0.78 | 0.31 (0.03 to 0.60); 0.03 | 0.11 (–0.36 to 0.58); 0.64 |
Model 2b | 0.17 (–0.06 to 0.40); 0.15 | 0.10 (–0.36 to 0.56); 0.67 | 0.34 (0.04 to 0.65); 0.03 | 0.11 (–0.37 to 0.58); 0.66 |
Feeling life is worthwhile | ||||
Model 1a | 0.07 (–0.15 to 0.28); 0.54 | 0.15 (–0.28 to 0.58); 0.50 | 0.00 (–0.28 to 0.28); 0.99 | 0.12 (–0.33 to 0.57); 0.59 |
Model 2b | 0.06 (–0.16 to 0.29); 0.57 | 0.18 (–0.27 to 0.63); 0.44 | 0.08 (–0.21 to 0.38); 0.58 | 0.14 (–0.31 to 0.60); 0.53 |
Feeling happy yesterday | ||||
Model 1a | 0.21 (–0.06 to 0.48); 0.12 | 0.16 (–0.34 to 0.66); 0.52 | 0.33 (–0.05 to 0.71); 0.09 | 0.12 (–0.47 to 0.71); 0.69 |
Model 2b | 0.19 (–0.09 to 0.46); 0.19 | 0.19 (–0.35 to 0.72); 0.49 | 0.36 (–0.05 to 0.76); 0.08 | 0.14 (–0.47 to 0.75); 0.65 |
Crime | ||||
Model 1a | 3.37 (2.85 to 3.90); < 0.001 | 4.14 (3.21 to 5.07); < 0.001 | 3.29 (2.55 to 4.02); < 0.001 | 1.85 (0.62 to 3.08); 0.003 |
Model 2b | 3.36 (2.83 to 3.90); < 0.001 | 3.95 (2.97 to 4.94); < 0.001 | 3.54 (2.76 to 4.32); < 0.001 | 1.88 (0.64 to 3.12); 0.003 |
Quality | ||||
Model 1a | 4.91 (4.41 to 5.40); < 0.001 | 4.52 (3.68 to 5.37); < 0.001 | 5.11 (4.43 to 5.80); < 0.001 | 5.08 (3.81 to 6.35); <0.001 |
Model 2b | 4.98 (4.48 to 5.48); < 0.001 | 4.40 (3.51 to 5.29); < 0.001 | 5.30 (4.58 to 6.03); < 0.001 | 5.19 (3.92 to 6.47); <0.001 |
Relating 2-year change in neighbourhood perceptions to mental health and well-being scores (see Report Supplementary Material 22) showed that improved crime-free and quality scores were associated with decreased levels of depression and anxiety (all p-values ≤ 0.01). Although there was less of an effect of neighbourhood perceptions on well-being scores, there was some evidence that improved perceptions of quality were related to reduced reporting of low levels of life satisfaction (p = 0.05) and feeling worthwhile (p = 0.01).
Discussion
Baseline findings
At baseline, those seeking social housing were more likely to be depressed, anxious and less satisfied with life than those from intermediate and market-rent housing sectors. These housing sector differences in mental health and well-being outcomes appeared to be partially or wholly explained by demographic factors, including age, sex, ethnicity and partner/marital status, which are all well-recognised factors associated with psychological distress and well-being. 199,200 However, increased levels of depression among the social group persisted after adjustment for these potential confounders. This finding is consistent with a body of literature showing that those in social housing are more socioeconomically disadvantaged and at greater risk of poorer mental health outcomes, which is associated with increased stress exposures related to their surroundings. 22,201–203 Poorer well-being and neighbourhood characteristics, such as perceived levels of increased crime and decreased safety and access to green space, have all been shown to have adverse effects on depression, anxiety and well-being;46,188,194,204 it has been suggested that prolonged exposure to poor-quality neighbourhoods may have an amplifying effect. 205 Our results show that positive perceptions of neighbourhoods being crime-free and quality appeared to reduce levels of depression (as well as moderating non-significant associations with anxiety and poorer well-being) among those in the social sector compared with those seeking intermediate housing, suggesting that the negative neighbourhood perceptions observed among those seeking social housing may partly contribute to the higher levels of depression within this group. It was noteworthy that the sociodemographic characteristics of those seeking market-rent and intermediate accommodation were similar, and there were little differences in mental health and well-being except in having a marginally lower prevalence of feeling happy the previous day among the market-rent group. Allowing for neighbourhood perceptions attenuated these modest differences in levels of happiness and, unsurprisingly, did not alter the similarities in other mental health and well-being-related outcomes.
The differences observed with the social sector demonstrate the potential importance of the built environment on mental health among the more disadvantaged, which is entirely consistent with a body of literature showing the effect of the built environment on depression, anxiety and well-being. 153,187,204 Hence, it remains entirely plausible that the mental health outcomes of those affected by poorer surroundings might be improved by moving people into environments that encourage positive well-being,206 which longitudinal follow-up of the ENABLE London cohort was able to examine.
Follow-up findings
Despite observing sizeable improvements in objective measures of the built environment (see Chapter 3) and neighbourhood perceptions associated with moving to East Village, particularly in the social sector where there were marked improvements in perceptions of a crime-free neighbourhood, there was little evidence of improved mental health and well-being outcomes after 2 years (although there was a modest improvement in life satisfaction among those living in intermediate East Village accommodation).
Unfortunately, there is very limited literature to put these study findings in context, as few longitudinal studies have examined the long-term effects of urban regeneration schemes on health, and even fewer have examined effects specifically on mental health and well-being-related outcomes. 207 One notable exception is a large-scale experimental study carried out in five US cities, the Moving to Opportunity (MTO) study, which examined the long-term effect of being randomised to receive or not receive housing vouchers to encourage moving from high- to low-poverty neighbourhoods on the physical and mental health of 4606 minority low-income families. 208–210 Although improvements in living conditions among the intervention group were demonstrated over a 10- to 15-year period, there was little difference in adult mental health-related outcomes and economic self-sufficiency between groups, although a small improvement in subjective well-being associated with moving to less deprived neighbourhoods was observed. 206,208 However, there were mixed effects among the participants’ offspring. There was evidence of increased rates of depression, stress and conduct disorders at follow-up among teenage boys who moved to less deprived areas but, conversely, reduced rates of conduct disorders among girls. 210 Other more direct evaluations of urban regeneration schemes in Spain211 and the Netherlands212 have shown little effect on mental health outcomes from after 3211 to 5 years. 212 From a UK perspective, a large long-term housing improvement programme in Glasgow, Scotland, showed a small positive effect on mental health scores after 2 years213 and found that mental health scores in the most deprived areas that received higher levels of investment improved more after 5 years than lower investment areas. 214 However, effects were small and less favourable findings showed that the scheme may have actually increased fear of crime, as relocation may have disturbed established social networks. 215 Other UK studies that have examined the effect of urban regeneration programmes, including change in the built environment, have also shown little effect on mental health and well being outcomes, although change in the built environment has not always been well defined. 216 The closest study to ours geographically also used a natural experiment to examine the effect of London Olympic regeneration among 2254 children attending secondary schools across the London Borough of Newham, compared with pupils attending schools in other east London boroughs. They found no effect of urban regeneration on self-reported physical activity, mental health and well-being outcomes after 18 months, and repeated cross-sectional surveys among 995 parents suggested that levels of anxiety and depressive symptoms might have increased as opposed to decreased in Newham, compared with control areas. 217
In summary, the effects of urban regeneration on mental health-related outcomes appears modest at best and mixed, not applying equally across population groups. Our finding that moving to East Village did not have any sizeable effect on mental health/well-being-related outcomes is entirely consistent with these studies, suggesting that more needs to be done to demonstrate the effect of change in the local environment on mental health and well-being, and to understand why greater differences are not observed. 96
The ENABLE London study was carried out to provide robust evidence of the effect of the built environment on health (particularly in a UK context). However, a key limitation is that the mental health and well-being outcomes were secondary to the main hypothesis of the study (where the primary outcome was physical activity) and lacked statistical power. Moreover, the staged recruitment, whereby those in social housing were moved in before those in other housing types, before the East Village development was fully complete, may have dampened exposure effects and not allowed sufficient time for social networks to become established. Although no appreciable changes in physical activity and adiposity outcomes were observed (see Chapter 3), which could have plausibly affected mental health and well-being, it remains possible that longer-term follow-up may demonstrate significant effects. However, this seems unlikely given the modest effects on mental health and well-being outcomes observed after 2 years and that continued development of the area, including high-rise accommodation blocks and reductions in green space,218 could potentially lead to adverse effects (see Chapter 9).
Despite the growing need for more housing, particularly in major cities, opportunities to examine the potential health impact of such developments are limited. It is widely accepted that area regeneration programmes should be designed to have positive impacts on its residents, as well as reducing health inequalities. 219,220 However, it is challenging to create high-density urban environments with appropriate local facilities to promote positive health behaviours while also protecting residents from the potentially adverse effects of high-density housing. 221 There is little understanding of what is considered to be the optimum density to encourage social contact while moderating other exposures, especially among the more disadvantaged. 153 Further research is needed to establish how people from different socioeconomic backgrounds living in the same neighbourhood interact with each other and their local built environment to identify specific aspects that may be contributing to poorer health. Such studies will inform the planning and design of housing developments for improved mental health and well-being.
Chapter 7 Qualitative data from the ENABLE London study
Previous work has indicated that there were limited positive effects of the London 2012 Olympic and Paralympic Games on the most disadvantaged residents of the Olympic boroughs. An important part of the legacy of the London Games was the conversion of the Athletes’ Village into East Village, which was designed on active living principles and aimed to provide social, intermediate (affordable) and market-rent housing. The ENABLE London study investigated the effect of moving to this new environment on levels of physical activity among individuals from all housing sectors. The present analysis is based on focus group discussion with the social housing residents of East Village. Participants were asked about personal the social and environmental influences on their levels of physical activity and their use of local recreational space and facilities. Participants were positive about their new homes and the safer neighbourhood; however, they also complained about the high cost of living, restrictions on children’s play areas and limited facilities for older children/teenagers. The high costs, in particular, had a strong impact on participants’ plans to continue living in the development. These concerns need to be addressed to ensure a sustainable community.
Introduction
Defining and understanding the legacy of large events such as the Olympics is complex and can encompass elements of economics, built environment and sustainability. 222 ‘Soft’ or non-infrastructural elements of a legacy include individual-level changes to mental and physical health and skills development, as well as community-level changes such as increased social cohesion. 223 The bid for the London 2012 Olympics emphasised legacy. 224 The Olympic Games were positioned as an opportunity to tackle the problems of London’s East End, which included deprivation, unemployment, poor health outcomes and a shortage of housing, by driving regeneration and through increased investment. 225
High-quality evidence supporting the role of major sporting events in delivering a tangible (infrastructural) or non-infrastructural legacy is limited. 223,226 Evaluations of the London 2012 Olympics also suggest that there was little positive impact on the most disadvantaged residents of these boroughs. Previous research has noted that the housing legacy of the London Olympic Games has fallen short of its ambitions; this appears particularly true in the case of social housing provision. 227 Indeed, development and gentrification has led to the decanting of existing communities to make way for Olympic facilities,228 the growth of developments that are perceived by the local population as ‘not for them’229 and the displacement of residents as a result of the increases in rent and house prices. 227 In terms of a health legacy, studies have failed to find sustained health improvements in the area. 217,230,231
Although several studies have examined the effect of the Games on residents of the Olympics boroughs, there has been relatively little research examining the views of residents of the new East Village. The Speaking Out of Place project232 interviewed tenants from the social, intermediate and market-rent groups in East Village, in addition to individuals with a community role in East Village and residents from neighbouring areas. Their analysis found a growing sense of community among residents, although there were tensions around the high levels of securitisation and control. Both social housing and intermediate housing tenants reported concerns about the high costs of living in the neighbourhood and insecure tenancies. 232 In contrast, Wilson’s233 work found relatively little interaction between residents, which was largely confined to individuals living in the same block. The work also suggested that certain features of the built environment in East Village may serve to limit interactions between different housing tenures. 233
This chapter presents an analysis on the experiences of social housing tenants who moved into East Village.
Methods
The focus group was conducted in 2015 among social housing tenants approximately 1 year after they had moved to East Village, and aimed to identify factors relevant to the built environment and health that had not been covered or were inadequately covered by the baseline questionnaire used in the study. It was expected that findings from this focus group would provide additional context to the findings, as well as inform the spatial narratives work that would be carried out (see Chapter 8). Although the initial proposal included carrying out focus groups with members of the social and the intermediate housing sectors, time constraints meant that only social housing sector participants were contacted as they were the first group to move into East Village.
The focus groups were conducted using established procedures,234 audio-recorded and transcribed. The focus group started with a free discussion by participants regarding their experiences of living in East Village. As the discussion progressed, additional topics such as community cohesion, transport, traffic, noise, parking, safety, shopping, and the levels of exercise and use of local parks, gyms and swimming pools (including use of the Olympic Park) were introduced by the facilitator. All transcripts were anonymised. Following this, a thematic analysis235 was used to identify the main themes from the content of the focus group transcripts. This involved reading and rereading the transcripts and grouping data extracts together according to their main themes. A coding framework was developed, based on emergent themes, which identified the major topics and issues.
Eighty ENABLE London study participants who took part in the follow-up assessments between January 2015 and June 2015 were asked if they would be interested in taking part in the focus group. A total of 75 individuals from 65 households expressed an interest and were sent a formal invitation letter in July 2015. Thirteen households agreed to take part and were sent a further invitation letter with three suggested dates. The most popular date of participant availability was determined. Eleven individuals from separate households agreed to attend the focus group. On the day, seven attended; two confirmed that they were not able to attend and there were two no-shows. The participants were three black females (aged 32 years, 45 years and 39 years), two Asian females (aged 43 years and 45 years), one mixed ethnic origin female (47 years) and one white male (45 years). Five participants lived in Newham prior to moving into East Village; of the remaining two participants, one lived in Hackney and the other lived in Tower Hamlets. The focus group was conducted at a local school (Chobham Academy, E20) in August 2015 and lasted 100 minutes. East Village residents had been living in their new homes for 12–18 months. Five participants were in employment, one was a student and one was unemployed because of ill health. Shopping voucher incentives were given to those who participated.
Results
Five themes emerged from the analysis of the focus group transcript: (1) ‘cost of living’, (2) perception of new home, (3) perceptions of the neighbourhood, (4) sense of community and (5) healthy living. Quotations are identified using unique initials for each participant and their gender.
Cost of living
Participants commented on issues around the expense of living in an area of London that is undergoing rapid redevelopment and gentrification. They were concerned about the higher costs associated with living in the new development, including higher costs of basic utilities and council tax, which meant they were unable to save:
My first thing is the expense. I can’t save. Whereas in my other house, I could save. I can’t save at all. Obviously, it’s a band D area, I wasn’t in a band D, so council tax has gone up, the rent has gone up. I’m paying for water. I’m paying to heat my water. I’m paying the electricity. I’m paying to clean my communal area. It’s too much. I can’t save. I have to pick and choose the brands I buy in the supermarket to try and keep my costs down. I’m more financially strained now.
A, female
And to compare with what we were paying before and here . . . my water bill has gone by, I’d say about 70%, so that’s a lot . . . We were told it was going to be a decrease. One of the reasons I chose the house is that it would be 25% less living cost compared to where we were living. So I thought, ‘Oh! 25%. That’s quite a bit.’ But actually it’s about 50% increase.
B, female
The high costs were cited by residents as a major reason why they would not consider living in East Village long term:
I don’t see myself living here. With my children. It’s a lot of expensive. You can’t save money.
C, female
. . . more and more expensive, I just think, oh right, because they say it’s the East Village, I don’t know if you’re paying because of all the re-development they’ve done, we’re now paying for it, umm, but it wouldn’t be feasible to live here long term, if it carries on.
G, female
Amenities such as shopping facilities were limited for the social housing sector who were the first to move to East Village. The residents did not really use the Westfield Shopping Centre, which forms a gateway to the Olympic Park, citing expense as the main barrier. Many reported going to shops near their former residence:
And it’s funny to say, I don’t shop at Westfield, I do go around, just to browse around. I’ll probably go to some of my familiar shops to do you know like clothes shopping and stuff, but I don’t buy my groceries . . .
F, female
Waitrose did until umm Sainsbury’s opened. It did get used but I used to find– I’ve got grown-up adults children, who are eating for the world, I would go in there and get like two bags and it would be like £20/£30 and that was nearly enough every day . . . Oh my God, I thought, I can’t live here.
B, female
Perceptions of new home
East Village residents were generally happy and reported a sense of pride in their new homes. Many noted that there was a greater sense of calm in their new homes associated with better family space and family members having more of their own space. Better ventilation, no damp and brighter homes were all noted as positives:
The positive is because my children are older they’ve got their own rooms now. We’ve got a bigger kitchen . . . and now the whole family can sit down around the table. We have a family meal every day, whereas before it was once every so often. So it’s brought us closer . . . This place is cleaner. The area is cleaner compared to where I lived before. . . . there are a lot of positives. Everyone’s calmer. It makes the family a little bit more calmer. Also, they’re very proud: it’s a nice place to live. They feel happy.
B, female
I’m quite happy here. It’s bigger and the children are a lot more happier here . . . I feel secure in here because the security is 24 hours . . . We have a bigger kitchen now and we have family meals every day now, we eat together. They’ve all got their own rooms. So we [are] calm.
D, female
Anyway, I live in a townhouse and I found the place very good. As you said the ventilation is excellent in the house. The rooms are big and everything. About the house I’ve got no issues with the house, it’s just excellent.
D, female
Despite this, the families also had some complaints about the new homes. Individuals in the flats complained that there were limited storage options and strict rules regarding where they were allowed to store items. A further complaint centred around rules for the social housing tenants, which stated that they were not allowed to personalise their flats, including adding curtains to the windows, which had an impact on privacy:
Most of the proportions are– once you put a wardrobe and a chest of drawers in it’s really tight.
E, male
I must say that what we’ve said about storage, the storage was better in my old house because I did– I’m not necessarily a hoarder, but I have things that I know that, for example, we will use in the summer, whereas now I had to buy storage, I had to buy a big storage to put on my balcony. I’ve had to buy storage containers to put in my hallway. I’ve had to buy another big shoe storage to put– I’ve had to spend loads of money, hundreds of pounds, just to– because I’m a believer that umm everything should have a home and I don’t like clutter, so if it’s out of order, I throw it in the bin, and obviously– so as I said storage is a big problem. Can’t store my buggies anywhere whereas in my old house we had like a downstairs sort of like cupboard where we could store stuff.
A, female
Perceptions of the neighbourhood
Overall, participants were very positive about their new neighbourhood, in particular commenting on the cleanliness, order and greater security compared with where they lived before:
That’s really nice actually. I think the greenery is lovely. The greenery is lovely. The way that like we’re close to transport, close to Westfield, all of that’s nice as well. The streets are clean. I like the way that they have tried to upkeep the cleanliness of the area as well, you don’t hardly see any garbage on the streets. Even down to– well I haven’t experienced it, like unruly kids on the street, like– you know you get normally like people hanging around, because that was my fear here, was coming here and having like little kids hanging around, gangs hanging around, or whatever, because of the way that the community is laid out, but I haven’t experienced any of that, I haven’t seen any of that yet.
A, female
However, participants also reported certain frustrations with the development. In particular, many of the social housing tenants had young children and they were concerned about the number of restrictions on children’s play areas. Despite having access to the playing areas in the Olympic Park, many parents felt that those areas were too far away for their young children to go to alone or to play in unsupervised:
I feel that there is a lot of restriction, so children cannot, for example, play ball games, ride bikes in the terrace. If they have to they have to go to different parts, even though the parks are not so far, but it requires leaving everything.
F, female
The issue of limited facilities to keep teenagers engaged was also noted by the residents. Participants were concerned that as children grow up, there would be little for them to do, which could lead to antisocial behaviour. This was a major source of worry for these parents, and would be very likely to have an impact on their decision to stay on in the development:
I think also like for my daughter, who is a teenager, what we were saying before, there’s not much in the area for them to do, apart from going to Drapers Fields and I think there should be like a little community thing where they can do things like table tennis and– under supervision, you know, like after school, because my daughter does get bored. She just– in my area, in my block, in the community, there’s a lot of young children, not much children her age, and I’ve noticed that children who come to the school that do live in the area umm I don’t know, it’s like they are forced to argue. Because they don’t really have much to do, a lot of the time my daughter’s on her tablet, with people in the area, umm, abusing each other, and I think if they– yes, no, but seriously, there’s no sense of cohesion with the teenagers in the community. There’s a sense of cohesion with the small ones, but me personally– and a lot of the time I see them sitting on the steps and stuff like that, as I was saying about finding the condom in the sand pit area, they are doing things like that, whereas if there was something positive that they can go to . . .
A, female
People hanging around on the staircases, it’s going to get worse and worse and especially if they don’t build a communal area for the teenagers, it’s going to get worse and worse. They’ve only built it up for young children. So that’s one thing that I’m dreading in the future and that’s one reason, another reason, why I’d want to leave as well is . . .
A, female
Sense of community
Participants’ relationships with their neighbours were generally positive. The management was also active in organising a range of activities for residents to meet each other and managers. However, it appeared that only a few were aware of or had made use of facilities organised by the management:
Well my neighbours are really really nice. My neighbours on my floor are really really nice because we send each other Christmas cards, even when I had my daughter, they sent me gifts, etc. That’s really good. I must say that I don’t– I know there’s a lot of community things going on and they display it on the boards in the flats, but I don’t make use of them personally, so I can’t really.
A, female
Encouraging healthy living
East Village was designed to encourage healthy living and has access to gym and sports facilities in the Village and nearby Olympic Park, as well as the swimming facilities in the Aquatics Centre. Parents with young children felt that facilities for children to play should be made available closer to the houses. A few concerns were also raised regarding security in the available play areas:
And we’ve got so much open space, I think for children, they should do something– like a proper park, with swings, a slide, things like that, because they don’t want you to use the communal gardens, so they should provide a little local park for them.
G, female
In comparison to my old house we had like, as we said, a little park, where my child could go to. Here you’ve got little bits of parks, here and there, which is a bit of a bummer and I don’t think some of the parks are controlled very well around here, whereas in my old house, they were very very controlled . . .
A, female
Overall, experiences with increasing physical activity were mixed. Participants were often unaware of the facilities available to them and many reported not using them. In certain cases the lack of local shops made it necessary for people to travel further away to buy things, which meant they were more likely to drive or use public transport rather than walk, therefore reducing their physical activity:
Umm I would say mine is the opposite. Umm– because where I used to live before, umm, you could walk to like the local shops, etc. I know I’ve got Sainsbury’s now but sometimes if you want something elsewhere, I used to like umm– because of my culture we buy certain foods that we can’t buy in Sainsbury’s, but I used to have shops close to me that I could just walk to, but now I have to drive to work to get those things, so I won’t walk, I will just take the car, so it’s restricting me, umm, I don’t walk as much in that sense now . . . The only difference I would say is walking from my house to the car park, that’s probably the only thing that’s changed, because in my other house, I could just walk outside my house and my car would be there, so in terms of physical activity, no.
A, female
I think I’ve gained some weight since I have moved from my old address to this new address and that’s partly because, what you just said, I drive around as well, I don’t– because I find things a bit far, I mean, where I was in my old home before, I could go to my work, because I work in the school as well, that’s part time. I used to take a walk to the children’s centre but now I can’t take a walk, it’s quite far, so if I have to get to my children’s centre, I have to drive.
F, female
Umm I’m less active. Because where I lived before I used to walk to work, walk home, now I have to get a bus.
G, female
Others reported using the facilities to a greater extent and encouraging their children to do the same:
I do make use of the– because I go to the gyms as well there and the swimming. I get the kids involved. I’ve got a 12-year-old who has put on a bit of weight since we moved in here. He actually put on weight when we moved here, so I said, right, swimming, basketball, table tennis, these are all free for him, so I said right, I’m registering you in to everything and then what we do we go there, weather’s good, soon as I’m finished from here that’s what I’m getting ready to go, we will sit and spend the whole day there.
B, female
Well for me, my activity is because I have to walk more. Before I was not. Because the parking (. . .) to leave your car at work because when you move your car, when you come back, there won’t be parking, so why don’t you leave it here, walk, just across the other side of Stratford and go to work, so health-wise I’ve seen a lot concerning my life and the children and then, umm, the rest I don’t know the rest but for me it works for me.
C, female
As East Village was designed to encourage active living, there are restrictions on parking and limited availability of parking spaces. Although residents were made aware of this, some participants, particularly those with large families or with health problems, found this restriction limiting:
The only problem is the parking because I can leave my car and go to work, but when it comes to shopping, as a family of five children, there’s no way you can do shopping by bus or train, so you need that car, but sometimes I have to wait to go shopping, you can’t have a place to park, even though you have a free parking along there, outside, or– so you have to pay the inside one, which is £60 a month, which is very, very expensive for a mother of five, so that’s the only problem, apart from that I’m really enjoying the place.
C, female
I needed my car, but coming here, because I never had two children under the age of 10, I wasn’t allocated free parking, which was horrible, I mean I’ve had parking tickets, I mean the parking guidelines are not clear here, as well . . . I wasn’t allocated underground parking, which was obviously a thing, and now I’ve got another daughter as well, so it’s a big problem, so I have to pay £65 Stratford International to park my car every month.
A, female
I gave my car up just as we moved in, umm, because I wasn’t allowed– I applied for the parking, I didn’t get it, and you know, the one that you get with the underground, and at that time, I actually didn’t know about the council parking, because I did ask about that, and all they told me about was the parking that you know the Triathlon– the East Village parking so I wasn’t aware of [whether] we was going to get a council parking, afterwards, because the first car is free, so I got rid of my car, so I do find shopping is a little bit hard, because I’ve got umm early-onset arthritis and to carry shopping from anywhere it’s a bit difficult, but I mean, I suppose we do online shopping and get them in somehow.
B, female
Discussion
Residents of the new East Village had largely positive views about their new homes and enjoyed the security and cleanliness of the neighbourhood. Although participants in the focus group were largely unaware of, or did not choose to participate in, many community events, they reported good relationships with their neighbours. Most participants were, however, quite concerned about the high costs of living in East Village. Quantitative analyses of the ENABLE London study data suggest that there were no significant improvements in physical activity levels (see Chapter 3) or well-being (see Chapter 6) of participants associated with moving to East Village. 66 Furthermore, the quantitative analysis showed that the residents perceived the new neighbourhood to be of a better quality and as having less crime than the areas where they previously lived. 66 These findings are broadly supported by the focus group data. Findings from the focus group analysis also support the results of the Speaking Out of Place project, which noted that organised community activities as well as tenants’ groups were contributing to the building of social networks and a growing sense of community solidarity. 232
The social housing sector was the first to move into the new development; however, many facilities such as nearby shops were not yet open. These amenities are now available in East Village (see Chapter 9). Analysis of the focus group data indicates that many of the participants found costs associated with living in the new housing development to be prohibitive and in some cases individuals felt that they were getting less for their money than with their previous accommodation. Many facilities that were available were also unaffordable. This had clear implications for the participants’ shopping in the local area, which discourages a sense of community. Many commercial developments in East Village appear to target wealthier residents. 233 Exclusions because of affordability were also noted in the Speaking Out of Place project. 232 The findings highlight existing uncertainties around mixed-tenure developments, in which many facilities or nearby developments may not be affordable to residents on lower incomes. 236
Another difficulty cited by participants related to the provision of facilities for children. Many felt that parks were too far away for young children to access and the areas near their homes had strict play times and restrictions imposed on children’s play areas. The social housing residents were also concerned about the availability of activities for older children, particularly teenagers, and were worried that as their children grew up the lack of facilities would mean boredom and possible antisocial behaviour. Previous work has noted the ‘sterile’ quality of the East Village neighbourhood brought about by the strict policies regarding cleanliness, playing areas and noise enforced by the management, which do appear to find some support from the private renters who complained about noisy children. 232,237
The lack of nearby play areas for children and limited local shops led to a decrease in physical activity for some participants. Many were also unaware of discounts at the Olympic Park facilities that were available to them. Findings from the quantitative analysis in the ENABLE London study suggest that participants in social housing were closer to a park in East Village than they were in their previous houses, with walkability and access to public transport also higher in East Village. 66 However, when the social housing tenants first moved into the development many amenities such as the parks were still being developed, and access to certain areas might have been limited. Later work examining the use of shared spaces in East Village found that the presence of high-end stores around one of the largest parks led some social housing tenants to believe that the park was solely for the use of the market-rent tenants,233 suggesting that certain design features may inadvertently stop individuals from using these amenities. Although the limited parking led to increases in walking for some members, others found this to be very inconvenient and an added cost. Planned developments, including building on existing green space, may lead to a further decrease in activity levels. 218
Our analyses are limited by the fact that we carried out only a single focus group with social housing tenants from East Village; additional focus groups would have been able to provide us with a fuller picture of life in East Village for this housing sector. Additionally, carrying out focus groups with participants from the intermediate and market-rent sectors would have allowed us to better understand how the different housing sectors experience their environment.
Conclusions
The Olympics represented an opportunity to have an impact on the social and structural determinants of health in a deprived part of London;224 however, the housing legacy of the London Olympic Games has fallen short of its ambitions. 227 We and others have identified many positives associated with the social housing in East Village; however, concerns regarding affordability and facilities for children need to be addressed to ensure a sustainable community with health benefits for all.
Chapter 8 Qualitative data: using spatial narratives to explore how relocating to East Village influenced perceptions of the environment and active travel decision-making
Introduction
Physical activity is not represented by a single behaviour; it is an outcome underpinned by a series of behaviours, which may have different determinants. In adults, these behaviours or domains commonly include occupational (work-related), domestic (household, active child care, chores), work transportation (walking or cycling for commuting), non-work transportation (non-work walking or cycling trips) and leisure time (discretionary or recreational time, active hobbies, sports and exercise). These domains are also not exclusive; for example, walking or cycling to shops to buy food combines two domains (non-work transport and domestic). For a move to a new neighbourhood to influence physical activity, it must increase activity in these domains by influencing their domain-specific determinants either directly or indirectly. 238 New opportunities to take up active travel or change from passive to active travel can particularly increase daily levels of physical activity. For example, Sahlqvist et al. 239 reported that, after controlling for differences in demographic characteristics, adults who used active travel (i.e. walking or cycling) for either commuting purposes or non-commuting purposes engaged in an additional 321 and 279 minutes of self-reported physical activity per week, respectively, compared with individuals who travelled solely by motorised transportation. The combined use of active and motorised travel was also associated with greater physical activity than exclusively motorised travel. Prospectively, a positive change in active travel was related to an increase in total physical activity, in part because it was not related to a decrease in recreational physical activity. 240 Similar studies based on objective measures of physical activity highlight the importance of active travel for adult physical activity, which contributes on average nearly half of total weekday MVPA (47.3%). This represents a 60% difference in physical activity between those who do and those who do not use active forms of transport. 241 Furthermore, 11.25 metabolic equivalent hours per week of walking or cycling (akin to meeting the current recommendation of 150 minutes of moderate intensity activity per week) is associated with a reduced risk of all-cause mortality of one-tenth. This relationship held after adjusting for leisure-time physical activity, emphasising the value of promoting transport-related and leisure-time physical activity for health.
However, there are significant barriers to sustainably increasing physical activity in adults for both leisure-related and transport-related activity. For leisure-time or recreational activity, these include lack of facilities and time, feeling too tired or weak and having no one to exercise with. 242 Environmental barriers are particularly influential for transport-related activity: high traffic volume and speeds, distance to amenities, lack of pavements or cycle infrastructure, and unpleasant surroundings all dissuade individuals from walking and cycling. 238
Aspects of environmental design can help to increase levels of active commuting. Grid-based street layouts lend themselves more to walkability243 and cycle lanes segregated from motorised traffic can encourage more people to use bicycles. 244 Long-term policies to develop cycling-friendly infrastructure in European countries have resulted in increased cycling journeys and improved health and productivity. 245 Positive policy determinants include a mixture of hard and soft measures, ranging from laws relating to speed limits and urban design favouring bicycle transport to education and car-sharing initiatives. In addition, policy decisions involve consulting the population on their wishes and concerns about cycling before implementation. 246
In more recent policy recommendations, UK policy now includes environmental approaches to increase physical activity such as recommendations to promote urban design that encourage active travel at all levels, including transport and regeneration planning, in both urban and rural areas. 247 Research from the RESIDE study in Perth, Western Australia, supports evidence for considering the wider environmental levels of influence on active travel. 94 After relocating to a new area, both transport and recreational walking changed in response to local transport provision, local facilities and attractiveness of the area. Over the first 12 months after relocating, transport walking declined overall but those with a greater number of destinations such as places of work, schools and green spaces increased their transport walking on average by 6 minutes per week with an additional 3 minutes per week for each additional local facility.
In addition to using objective measures to assess the use of the environment and the environmental elements most related to physical activity, qualitative data can add important complementary information. Qualitative enquiry can highlight the most salient perceptions for individuals, provide a temporal frame beyond the short-term objective measures of behaviour and provide additional contextual information that can help explain behaviour as well as the complex interactions between determinants, which is not possible with quantitative data. Qualitative semistructured interviews were carried out with a group of the ENABLE London study participants living in East Village61 to investigate their perceptions of the new environment compared with their previous neighbourhood, and how these environmental factors influenced subsequent transport-related and leisure-related decisions and behaviours. These analyses, therefore, sought to investigate whether or not moving to the East Village resulted in improved perceptions of the environment and whether or not this influenced levels of activity in different domains, particularly transportation and leisure-time domains.
The East Village development has housing in blocks of apartments, with green areas and pathways among the buildings. It is situated next to the Queen Elizabeth Park, also built for the 2012 Olympic Games, which includes various leisure facilities that are open to the public and a large area of parkland known as the Waterglades (Figure 5). The design of the development included deliberate restriction of car parking, extensive bicycle lanes and storage in the form of outdoor stands, as well as various exercise classes and similar activities available to residents free of charge. There are numerous shops and eateries in close proximity to the residential accommodation, as well as an underground station at Stratford and a station for the Docklands Light Railway. There is a very large nearby shopping centre known as Westfield, which is situated between the apartment block complex and the Stratford underground station; security guards patrol the development regularly. The area also includes world-class sports venues [main stadium, aquatics centre, velodrome, BMX (bicycle motorcross) and mountain-bike tracks, road and cycle routes]. In comparison, the type of neighbourhoods from which these participants moved can be seen in Figure 6.
FIGURE 5.
The East Village development.

FIGURE 6.
Street of terraced housing in Newham. ©2020 Google.
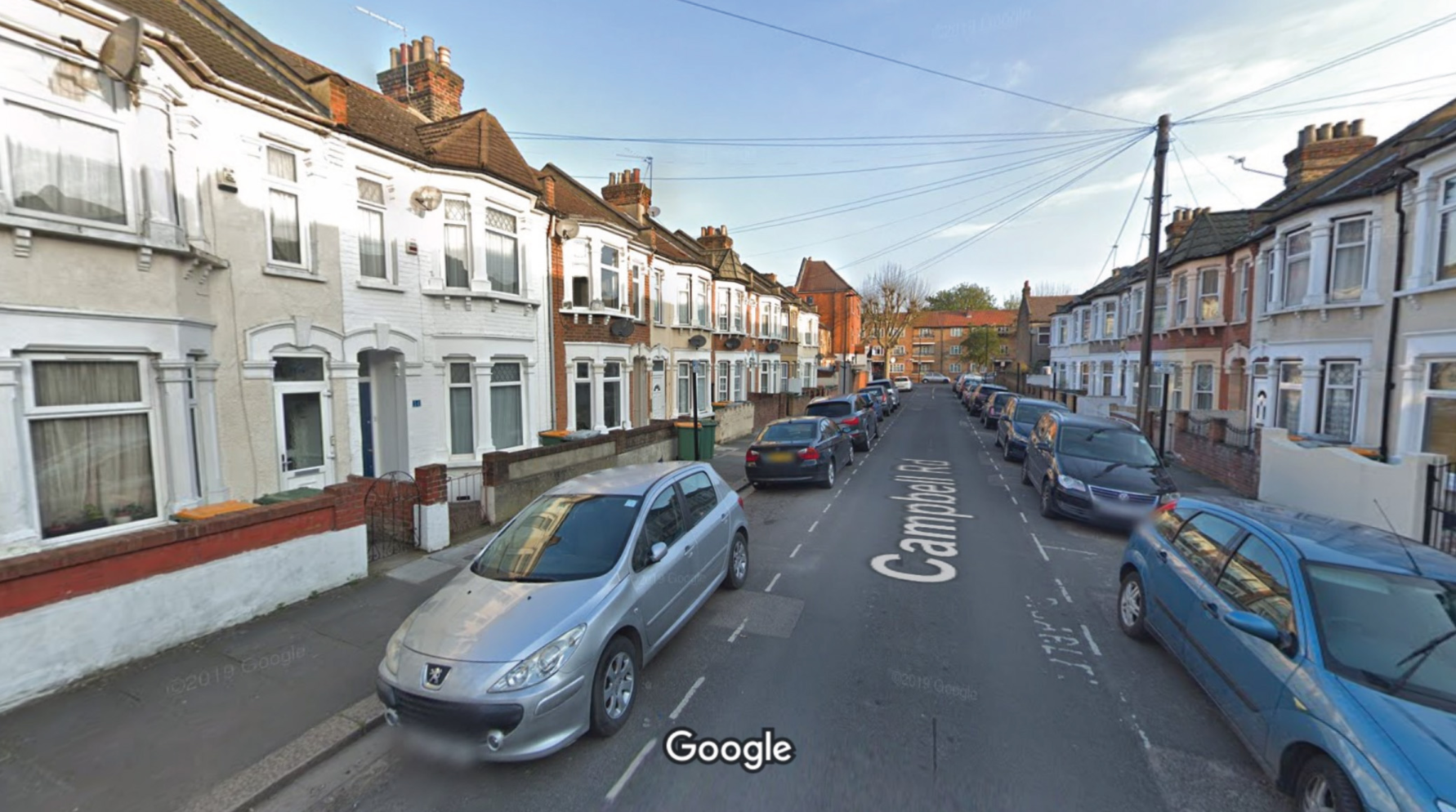
Methods
Design
This was a qualitative study based on an analysis of semistructured interview data generated with individuals living in East Village.
Participants and sampling
Purposeful recruitment was used to select participants with regard to change in travel behaviour over a 2-year period based on measures derived via an algorithm developed for the ENABLE London study, which used GPS, accelerometry and GIS measures (see Chapter 5). 166 Participants eligible for recruitment were selected on the following basis:
-
They all were living in East Village at the follow-up interview.
-
They met the ActiGraph compliance threshold at both phases (including ≥ 1 day at both time points).
-
Participants from all housing sectors were included (social, n = 40; intermediate, n = 92; and market-rent, n = 14 adults).
-
Information on age, sex and ethnicity was also provided.
From this sampling frame, three groups were targeted based on change in travel behaviour:
-
Those who were in the lowest quartile for the proportion of time spent in active travel at baseline and who had increased the proportion of time spent in active travel (walking and/or cycling) by ≥ 5%.
-
Those who were in the lowest quartile for active travel but did not change the proportion of their time spent in active travel over the 2-year period.
-
Those who decreased the time spent in active travel by ≥ 5% over the 2-year period.
Participants from the control group were not invited, as comparing perceptions of new and old neighbourhoods and the change in travel behaviour in response to the move was a key element of data generation. Exclusion criteria were limited to anyone who was non-English-speaking and all participants were offered a voucher incentive. All participants were given information about the study and gave their written consent before interviews took place, including for the recording of the interview. Focusing on those in the lowest activity levels is important because they potentially have the most to gain; for example, Kelly et al. 248 found in their systematic review that reduced risk of all-cause mortality was greatest in individuals with the lowest levels of activity (i.e. ≤ 120 minutes of walking or ≤ 101 minutes of cycling per week).
Qualitative data generation
Semistructured interviews were conducted in participants’ homes between November 2017 and January 2018 by two experienced qualitative researchers (Rachel Hahn and Bina Ram). The responses were recorded on a digital recorder, transcribed verbatim, anonymised and stored on a University of Bristol secure server before being imported into NVivo10 (QSR International, Warrington, UK) for data organisation. The interview schedule was based around comparative perceptions of individuals’ old and new neighbourhoods, aspects of everyday travel and leisure and how moving to the East Village had influenced this (see Appendix 2 for the questionnaire used). This study provided a unique opportunity to use individual travel patterns, which were generated by a programme using GPS, accelerometry and GIS data as part of the ENABLE London study166 to provide the framework for a spatial narrative whereby residents could describe their routines, influences and decision-making within a spatial framework. Individual maps were generated for the interviews, which described patterns and places before and after moving to East Village. The interview schedule was developed in discussion between a researcher from the ENABLE London study (AP), a PhD student who was analysing interviews as part of her PhD (ER) and the researcher who conducted the majority of the interviews (RH). Interviews were structured to take no longer than 45 minutes (see Appendix 2 for the interview schedule). One-to-one interviews were selected as the method of inquiry because of the highly variable activity patterns of individuals, which meant that the interviews could be tailored more effectively.
Results
Table 23 outlines characteristics of the 26 participants who agreed to be interviewed. Overall for the increase in active travel and no change in active travel groups, there was good representation from the social and intermediate housing sectors and by gender and ethnicity. The profile was different for the decrease in active travel group but was representative of the smaller pool eligible for invite, whereby the majority of participants who were active at baseline but decreased their activity by at least 5% were from the intermediate housing sector, male, white and relatively young. Across all three groups the age of participants represented a relatively narrow range, which skewed towards younger participants. The market-rent sector was under-represented in the sample largely owing to the small number who met the eligibility criteria.
Characteristic | Active travel | ||
---|---|---|---|
Increased (n = 10) | No change (n = 9) | Decreased (n = 6) | |
Housing (n) | |||
Intermediate | 5 | 4 | 4 |
Social | 5 | 5 | 2 |
Gender (n) | |||
Female | 6 | 4 | 2 |
Male | 4 | 5 | 4 |
Age (years) | |||
Range | 24–54 | 26–49 | 19–32 |
Mean | 38.6 | 32.4 | 29.1 |
Ethnicity (n) | |||
White | 6 | 5 | 5 |
Black/African/Caribbean/British | 4 | 1 | 1 |
Mixed/multi | 0 | 1 | 0 |
Asian | 0 | 2 | 0 |
Perceptions of the environment and relationships with physical activity
The majority of participants generally expressed more positive perceptions of their new built environment in the East Village irrespective of gender, type of housing or whether or not their travel had changed since moving to East Village. The initial preliminary themes generated from the interviews are extracted and summarised below in relation to change in four key features of the built environment: Functional features, Feeling safer, Aesthetics and Destination features. This structure allows interpretation in relation to existing frameworks249 and the most contemporary systematic review on qualitative studies on the perceptions of the built environment and physical activity in adults. 250 Examples of functional features include direct routes, street and path design and maintenance, traffic control and vehicle parking. Safety features include surveillance, crossing aids and lighting. Aesthetic features include cleanliness, interesting sights, maintenance, greenery, architecture, noise and pollution. Destination features include proximity, accesses and availability of local facilities, green spaces, shops, health care, sports facilities, parking facilities, public transport and other destinations.
Functional features
The main functional feature that emerged from the interviews was parking and its impact on regular access to a car. Perceptions on the feasibility of owning a car varied. Negative perceptions included:
Now if you have a car, it’s a problem, the biggest problem is having a car here.
F (female), SH (social housing), NC (no change activity group)
It’s too expensive to drive.
F, SH, D (decreased activity)
Some residents had a more positive view, and indeed owned a car, mainly because of the option to purchase a dedicated car parking space in or close to the building in which they lived. Although a significant cost, this was seen as attractive, even if it was not used that often, and affordable relative to other costs and income, largely for those in the intermediate housing sector. Car ownership was reported less by those in the social housing sector, who tended to rely on friends and taxis for access. Other transport options influenced the decision whether to own a car or not, which was expressed as a weighing-up of options, for example the cost of car parking versus the cost of train travel at weekends:
From this side of London you’ve got access to quite a few different sort of bigger places and the train fare [sic] sometimes are just ridiculously high for a spur-of-the-moment kind of thing that you want to do.
F, IH (intermediate housing), D
Cars were rarely used during the week if a participant was commuting for work into London, as public transport was a more viable option. Good public transport infrastructure was frequently cited as a key reason for not needing a car:
There’s no point having a car.
F, SH, D
Furthermore, the view was expressed that it would be different if public transport infrastructure was not available:
If I lived somewhere with minimal transport . . . I’d get a car.
F, SH, D
Other than differences between housing sectors (e.g. less likely to own a car in the social housing sector), car ownership did not vary particularly between the activity change groups (increase, no change, decrease).
Feeling safer
A consistently reported change across all groups since moving was an improved perception of safety to be out at night. This was largely a result of improved lighting and the presence of other people, and led to more reported walking at night both for leisure and to get to destinations than was reported in their old neighbourhood:
It’s going to be 9:30 or 10, so there’s still activity and there’s lighting, whereas where I lived before, that whole side I had to walk it was a very quiet road, there’s no lighting there, so that makes a difference for me . . . you can even walk at 11 p.m. There’s always activity . . .
F, SH, I (increased activity)
This was most frequently reported by women in relation to personal safety and removed what was a recognised barrier to activity:
. . . the perception is it’s safer and then when you actually see like the crime stats and things it is safer, so that kind of makes you think, right, I’ll go out for a run, I’ll be fine.
F, IH, D
This was also reported in relation to the general design, which had wider open spaces to increase visibility:
I’ve never felt unsafe . . . feel more unsafe in areas where there are more cars because there’s more potential for me not to see something coming up on me, whereas here . . . you can tell someone’s intention when they’re on foot, so in that respect, I feel safer here.
F, IH, D
Therefore, the combination of good lighting, other people being around and space to observe those around were key to the improved perceptions of safety at night.
Aesthetics
Participants reported the advantages of wider pavements and diverse buildings, with the combination of more green spaces amid the built spaces seen as a positive compared with previous neighbourhoods. Noise, although not traditionally included as an aesthetic feature, is included here as it was mentioned by many participants in several contexts both positive and negative.
A reduction in traffic-related noise was consistently reported as a positive aspect of the residents’ new environment; however, noise within East Village was also reported as a potential negative. This was dependent to some extent on familial status, with residents without children commenting on children’s noise from playing outside whereas those with children commented on loud parties/groups sometimes in the communal areas, such as the barbecue areas. This affected the use of space and the choice of spaces to go to (e.g. to avoid identified ‘play spaces’ or those that attract crowds, such as barbecue spaces). This led to clear patterns in use of space with residents matching their needs and led, to some extent, to spatial segregation. However, the large amount of space and diversity of options meant that this did not necessarily restrict the use of outdoor space although to some extent it limited social interaction between some groups:
I don’t use the central courtyard at all. I just find that really awkward. It’s a nice space to have but . . . it doesn’t feel very adult to me, so there’s lots of different sized families and people that live here and the kids use that space quite a lot, but it doesn’t really feel very grown-up to me, so I just don’t bother with it.
M (male), IH, D
Destination features
Use of sports facilities in East village
Despite awareness and positive views about living in a neighbourhood with sporting facilities and the status of being connected to the Olympics, very few participants reported using the facilities. The most consistent reason was price:
The gym is too expensive . . . the swimming pool . . . no I haven’t used that . . . due to expenses again.
F, SH, I
There was awareness of potential subsidies and possible use in the future when eligible:
I feel that when I become a pensioner I will take off whatever they do there . . .
F, IH, I
Others reported limited interest, as some participants did not feel that the sports facilities were appropriate for them as they had little experience or interest in sport.
There were a small number of examples of participants taking up new sports activities in the village, mainly those who had previous experience of running or playing sport. Social media was a key way to communicate for group-based activities:
By Facebook [Facebook, Inc., Menlo Park, CA, USA] so there’s lots of Facebook groups for East Village, different things, all sorts of things, umm, football being one of them, so yes, I play with my neighbour, B, and he’s a teacher at the school so he was like the initial contact and then I met other people through the football . . .
M, IH, NC (no change)
Flexible public transport options
Public transport was a dominant component of most conversations owing to the multiple options available to residents of East Village. This affected their active travel decisions and behaviour but did not necessarily have an impact on increased active travel compared with where they used to live. The active component was largely unchanged:
. . . same distance, 10-minute walk . . . but it’s just different routes.
F, SH, D
In some instances, public transport options actually reduced travel-related physical activity since moving to East Village, either through direct factors (e.g. participant moved there to be nearer work) or because transport connections were improved compared with their previous residence. Participants generally felt that wayfinding was relatively easy within East Village and took advantage of the variable transport options when planning their journey. They invested considerably in minimising journey times by having highly planned use of public transport routes, which included minimising the amount of walking as part of the journey. This was related more to time saving than to avoid walking, but had probably affected the length of the walking component of regular journeys (e.g. to work) since moving to East Village. Participants also selected travel options to avoid certain places, particularly Westfield Shopping Centre, which was seen as a busy and uninviting through-route unless participants were actually shopping. Options included using the Docklands Light Railway or taking the bus:
But I use the bus a bit more now because it’s so convenient because it’s literally a 5- or 10-minute walk to the bus stop and it drops me off pretty much right outside work . . . but also it’s a short walk through Stratford, through Westfield, to get to the Central Line and then it’s two stops on the Central Line. I don’t like going that way because it feels like I’m walking ages and I hate going through shopping centres in the mornings, but yes, I can get the bus, so it’s more tempting if I don’t want to cycle.
F, IH, NC
Participants also valued through-routes where changes were not required or that were less busy, which was often the case for bus routes:
I know, because before, when I was travelling to . . . there was no direct route, really. You’d have to change and . . . the one thing about public transport [here] is I love it, I love a smooth journey, or I just sit there and I go A to B, which is why the bus journey is so attractive because it just/it’s just there, and it just takes you right to the station. You don’t have to get off and change for a second one or anything like that. So I think that’s probably why I use it a bit more than I did.
F, IH, NC
Indeed, public transport was often a factor in the decision to move and stay in the East Village:
. . . the transport links are better from here, so that’s kind of the appeal for staying here, I kind of think, you know, why would I want to move somewhere that’s going to be harder to get to work.
M, IH, NC
Green space
Many residents appeared to consider walking or cycling in the local area in a more positive light than they did in their previous area, but more in relation to leisure activity. Some residents found walking or cycling an enjoyable activity regardless of their location, but the addition of a more pleasant green environment influenced both their journey decisions and their decision to move to East Village:
. . . you know, we’re not near any main roads, tick, that’s really nice. We’ve got the park, tick . . .
M, IH, I
That park out there, as well, it’s quite small now . . . half of it has now been built on, but the other half is kind of a little/I call it a glen-style with trees wrapping around a kind of a very large grassy area and there’s lots of just trees and convenient seating and that on the walk up, so those are very heavily used.
F, IH, NC
Better access to resources: shopping, health care and destinations
Many participants commented on the resources being more easily accessible than in their previous neighbourhood, that is they were close by with minimal travel required. It was perceived to be easy to get to local resources such as general practice surgeries and dental practices as well as local shops and cafés:
Yes. If you wanted to go for a walk you could/2 minutes out there and you’re walking around the Olympic Park. If you want to go to shop, 5 minutes and you’re in Westfield. It’s/you can have whatever you want here. As much . . . as you want, so it’s quite/it’s well placed.
F, IH, NC
However, some residents articulated that they felt that East Village was less diverse in relation to destinations and places to go and was less centrally located to link to other neighbourhoods, which might prompt less planned or structured physical activity:
Not small shops . . . No. There’s just/around here there’s no sweet shops sort of thing.
F, SH, D
But we don’t walk into town as much as we used to, only because it’s not quite a central location as [before] . . . we might have walked into other neighbourhoods . . . We tend to go to the park for a stroll, umm, but otherwise not really I’ll just be on the couch for a couple of hours, umm, whereas before we might have gone for a stroll a bit more I think.
M, IH, D
Positive perceptions not enough
Although, as highlighted above, the residents’ perceptions of environmental features in the East Village were generally very positive, these positive perceptions were not in themselves enough to promote increased physical activity overall. Other factors mediated the relationship between the environment and the participants’ behaviour. Some of these were individual factors related to motivation to be active for health reasons and a sense of being active to take responsibility for your own health:
. . . if I have to lose weight, let me discipline myself and walk up and down [I don’t need a gym].
F, SH, I
The environment was a prompt for participants with a lot of previous activity experience to reintroduce old practised behaviours, such as re-joining a gym or taking up running again. A change in circumstances also superseded environmental influences, for example new work patterns (e.g. working long hours), change in capacity (e.g. because of surgery) or age, which was a reported barrier to taking up new activities owing to high levels of perceived risk, even within a supportive environment:
No, not going to ride a bicycle, not at my age now. Even if I did it would be in a very safe place. I’m not Lara Croft!
F, IH, I
Early stages of neighbourhood development
It was also acknowledged by participants that East Village was still developing and did not feel as vibrant as more established areas:
I think it’s because we . . . could kind of walk down . . . which was kind of cool, a bit snazzy and then make our way back through the back streets or something, whereas here it’s not quite the same, like you haven’t quite got the same vibes.
F, IH, I
However, it was also noted that the East Village was changing and evolving into a more interesting space as new facilities were introduced, albeit they were relatively limited:
. . . it feels like its grown into itself so there’s much more going on, like you said, the café’s downstairs, there’s some restaurants . . .
F, SH, I
Part of this evolution that was particularly slow was the social development between residents within the village. Many residents reported having positive experiences with visitors to East Village and maintaining contact with their old neighbourhood by visiting or by using social media, but there were few examples of social interactions between residents apart from among children within families and for structured activities, for example playing football. Residents were aware of group activities but did not report participation in activity. There was, however, participation in more static social events, such as when football was screened outside or in the barbecue area.
The level of social connection was to some extent influenced by a sense of connectedness to East Village. Some were less connected as a result of strong ties to their previous neighbourhood; this was particularly true for younger adults for whom the move was more of a family decision than their own decision. In most cases this was the result not necessarily of a negative view of the new neighbourhood but of a specific connection to their old neighbourhood. Others had little connection to their old neighbourhood and were keen to explore their new neighbourhood, extending their range and sense of place as time went on. They were keen to invest in their new neighbourhood environment marked by, for example, walking and/or testing new neighbourhoods, appreciating new activities and shops, and having a clear sense of pride in where they lived.
Discussion
The spatial narrative interviews show that the majority of residents felt that East Village had many positive elements and, in many cases, was an improvement on their previous neighbourhood. These perceptions generally reflected a positive view of their move and some were directly related to their travel patterns and activity (e.g. availability of facilities, green space, perceived safety owing to lighting of walkways), whereas others potentially had an indirect effect (e.g. nice buildings to view, perceptions of space).
Consistent with the findings from the quantitative data reported in Ram et al. ,64 those living in social housing particularly emphasised the positive environment of their new neighbourhood relative to their old neighbourhood. This was not necessarily because they found the new environment any more positive than did other groups but rather because they had more negative perceptions of the built environment in their old neighbourhood than the other housing sectors. Safety was a particularly consistent positive change, particularly feeling safe to be out at night, and is consistent with the shift in crime perceptions reported in the survey data (see Chapter 6).
The improvement in perceived personal safety is an important finding as it is a consistent correlate of physical activity, particularly for women,250 and demonstrates that effective design such as good-quality lighting can extend the time period available for activity (i.e. to include dark hours). Improved lighting may also prompt more people to be out, which in turn was cited as a reason for increased confidence to walk at night.
One of the factors normally associated with increased opportunities to be active is a good public transport system. However, the relatively sophisticated options in East Village may in fact have diluted this effect, as participants were able to effectively minimise walking time by using a range of options. Although cycle lanes were acknowledged, there was little evidence in these interviews of their regular use for commuting; they were used more for leisure than for routine physical activity. The high level of accessibility of destinations is similarly a recognised determinant of increased activity,94,250 and this was viewed as an advantage by residents. Although distances to destinations were short, for example there were facilities such as general practice surgeries, dental care and shops on site, this may have limited the need for sustained walking. These observations may help to explain the very limited change in active travel based on the GPS and accelerometry data highlighted in Chapter 5. This in turn may have affected the potential change in overall activity, as transport-related activity is such a strong component of activity profiles. 241 These qualitative findings are also consistent with objective indicators of the environment, as reported in Chapter 4, with the East Village demonstrating high levels of walkability, green space and access to destinations.
The limited potential for change in the transport-related activity highlighted above emphasises the important role that leisure activity plays in physical activity levels. Interviews supported the view that many elements in East Village stimulated physical activity, but these examples were often infrequent and ‘one-off’ events rather than routine activities that would have an impact on daily physical activity levels. However, these were highly valued by residents, and it is possible that with time these examples would grow into more habitual activity. On the other hand, it should be acknowledged that for many these were new or different behaviours compared with their previous experience (e.g. going for a bike ride or longer walks, taking part in exercise groups outside), so environmental stimulus alone will have only limited impact. Social–ecological frameworks acknowledge that change at individual, social and environmental levels is required to promote and sustain significant change. This also explains to some extent why significantly improved perceptions of the environment do not necessarily have an impact on physical activity behaviour. Other factors (e.g. personal capacity, motivation) as well as social and familial factors mediate the relationship between the environment and physical activity. There is less evidence that these have changed since moving to the East Village, particularly social relations between residents within East Village. Many social activities are still dependent on social activities outside the village. There is evidence that this ‘social infrastructure’ is developing as the built environment develops, but the effect is subtle and takes time. It relies on places to meet, share and engage beyond the provision of green space or large shopping centres. The provision of smaller shops and cafes is an example of how the environment is becoming more diversified and one successful social space does attract residents (the barbecue area and screening of events). As acknowledged in other similar studies,94 changes can take some time to develop, particularly if they are not a result of shifts to routine behaviour via transport activity. These qualitative data emphasise how a newly built environment takes time to evolve; this is reflected in residents’ reported early interactions with the environment. This is described both practically in terms of knowing where to go to fulfil daily life activities, but also emotionally in terms of where to feel comfortable in terms of a sense of neighbourhood. This process can be accelerated by environmental shifts; as facilities and businesses become established, clusters to represent known and comfortable destinations for residents emerge. Although the sporting facilities are generally seen as an asset to the neighbourhood, price remains a barrier to participation.
The strengths of these findings include the use of participants’ own spatial data both to select a group of participants who have changed differently from baseline to follow up in the ENABLE London study and to provide a basis for participants to describe their use of the environment and their perceptions of it. Although reports were retrospective in relation to participants’ previous environments, having examples of traces both before and after from the GPS data provided an important memory prompt, as well as a dynamic basis for discussion of change. These qualitative one-to-one interviews were also able to capture temporal shifts and how these may oscillate over time. This provides an important complement to the objective measures that, although longitudinal, can provide only a snapshot at baseline and follow-up. This is particularly important because participants’ spatial patterns are highly variable, which makes it difficult to capture them with short-term measures. Participants were also able to focus on the factors most salient to them and explain how they interact to influence behaviour, which is challenging with quantitative data even with sophisticated analyses.
Limitations include the relatively unusual transport structure within London, which provides residents with transport options and a decision-making structure that may not relate to other, less urban or smaller, areas. Although the use of participant data and information at baseline allowed sampling to be targeted to reflect a range of participants, those who were interviewed were relatively young compared with both the wider sample and the population, so these findings may not necessarily translate to the perceptions of older adults. Participants were also sampled based on travel patterns, which may not necessarily reflect overall activity for some individuals.
Conclusions
East Village is seen as a very attractive place to live, with the majority of participants expressing more positive perceptions of their built environment than of their previous neighbourhoods. It was also seen as a place that included many environmental elements, to encourage increased activity. However, these positive perceptions in themselves were not enough to prompt use of the environment to the extent that it increased physical activity overall. It did stimulate use of the space for leisure, but any change was offset to some extent by the limited options available to increase transport-related activity. This was in part explained by the sophisticated use of an extensive public transport network close to East Village. It is clear that the built environmental structure of East Village is still evolving, with smaller facilities, shops and cafes opening that will continue to diversify and build the neighbourhood. This alongside a more developed social infrastructure may have an impact on physical activity in the long term, encouraging residents to make use of their environment more frequently for activity and leisure.
Chapter 9 Discussion
Introduction
Low physical activity is widespread and poses a serious public health challenge both in the UK and worldwide. 251 The need to increase population levels of physical activity is recognised in current health policy recommendations. 5,252 However, interventions to increase physical activity levels, particularly community-wide interventions, have shown limited effects that are poorly maintained in the longer term. 29 There has been increasing interest in whether or not the built environment, especially in urban settings, might be a key constraint that limits opportunities for physical activity. 31 However, there is very limited high-quality experimental evidence examining the influence of change in the built environment on physical activity. 30,31 The ENABLE London project was established to address this issue, by providing evidence from the investigation of a natural experiment examining whether or not a sizeable change in the built environment can increase physical activity levels, as well as indicators of both physical and mental health, in the general population. This question has important public health relevance as small shifts in population levels of physical activity, in addition to other markers of health, can have an appreciable impact on health-related outcomes. 253
Principal findings
The East Village development, a neighbourhood repurposed in accordance with active design principles, resulted in sizeable improvements in objective measures of the built environment and neighbourhood perceptions of the local built environment among those who moved there compared with those who did not. However, after 2 years, moving to East Village did not have any consistent beneficial effects on objectively measured physical activity, adiposity or other health-related outcomes of public health importance. Although modest reductions in car travel and increased use of public transport were observed among those who moved to East Village, these did not materialise in demonstrable change in active modes of travel (i.e. time spent walking or cycling). These findings suggest that the built environment is insufficient on its own to change physical activity behaviour. More evidence from similar longitudinal studies is needed to confirm these findings. However, given the difficulties and costs of this type of study, which relies on urban regeneration, we may need to think of innovative approaches to evaluate the effect of housing and urban change developments on health, to inform future evidence-based housing policy.
Principal contributions and implications of the study
Explaining the effects of the intervention
Understanding the mechanism through which the built environment might influence health behaviour is not straightforward and may well be multifaceted. 96 Reasons for the lack of clear effects of the East Village development, the ‘intervention’, on the physical activity-related behaviour of residents remain to be fully understood,33 especially when findings from cross-sectional studies are less equivocal. 93 One potential explanation is that those seeking to move are inextricably different from those who do not. The ENABLE London study sought to minimise this bias by recruiting a cohort of individuals seeking to move to East Village, which we believe, in the absence of randomisation, offers the best possible study design. It is also possible that the magnitude of change in the built environment was insufficient to induce a major change in physical activity. However, the East Village development showed marked changes in objective GIS-derived markers of the built environment and sizeable improvements in neighbourhood perceptions (particularly in perceptions of crime and quality) among residents moving to the area compared with those who did not move or moved elsewhere. So why did the change in the built environment not result in a bigger change? This report ventures a number of explanations, including whether exposure to the environment was long enough or sufficient enough to induce the change expected; the environment was incomplete when the first social housing residents moved in and was compromised by further high-rise development and loss of green space more latterly. 218 In the absence of more discernible effects on health and behaviour, it is difficult to establish clearer explanations for the lack of more definitive findings. However, it appears that the built environment alone is insufficient to induce the change in health behaviour expected by passive means and that more interactive strategies, perhaps in conducive environments such as East Village, are needed (i.e. perhaps higher-agency programmes combined with low-agency environments are needed to induce change). However, although high-agency population interventions are often used, particularly by governments to improve diet and reduce obesity, they are often ineffective254 with time constraints and low levels of motivation often being cited as reasons for poor uptake. 255 Moreover, high-agency interventions that require more effort from the individual may reinforce or even worsen socioeconomic inequalities in health. 254 Hence, low-agency interventions that require less investment from the individual may be more effective, having a small effect in greater numbers and hence may be more equitable by having greater reach. 254 In the context of East Village, greater agency could be achieved through greater awareness among the residents of the activity-inducing features and facilities that exist (such as walkways, cycleways and sporting venues) and fostering the belief, particularly among social tenants, that these are available to all. However, the premise of this study was to examine the effect of the built environment per se, without these additional ‘nudges’ to promote use. 256
Generalising the effects of the intervention
In terms of the representativeness of the ENABLE London cohort, we compared our physical activity data at baseline to a nationally representative study, the Health Survey for England,257 that used a similar methodology: the same waist-worn accelerometer (ActiGraph) worn for an equivalent wear time (1 week). Adults aged 16–34 years from this study recorded 40 minutes per day in MVPA, of which, on average, 15 minutes was in 10-minute bouts. Our baseline data suggest comparable levels of activity among those of a similar age in the social sector with 47 minutes of daily MVPA and 7 minutes in bouts (with an IQR between 1 and 15 minutes), but higher levels among those in the intermediate and market-rent sectors with 65 minutes of MVPA and > 20 minutes recorded in bouts. Although this suggests differences in baseline physical activity levels across the housing sectors in the ENABLE London cohort, there was no evidence of a trend across other social markers (i.e. income groups) in the Health Survey for England study. Moreover, the greater change in physical activity associated with moving to East Village in the intermediate and market-rent tenured groups compared with those in social housing, does not suggest that these higher levels (albeit not significantly higher) limited scope for change (i.e. participants from these non-social tenured groups had reached the capacity for further increases). In terms of geographic patterns in physical activity, reanalysis of the Health Survey for England7 data did not suggest that self-reported higher levels of physical activity in London were unduly higher or lower than other government office regions. 258 Hence, we believe that null findings from the ENABLE London study do not reflect bias within our cohort and are transferable beyond our study population. However, it is acknowledged that a cohort of ‘movers’ seeking to move to East Village, an active permissible space being built on active design principles, were targeted for the study and their activity patterns may differ from the population at large. Hence, this may have some implications for the generalisability of the findings.
In terms of specific features of the East Village development that could have influenced health and well-being (such as the use of pathways, cycle paths, links to public transport, open spaces and leisure facilities) are (or could be) features of many built environment developments. Hence, we believe that findings from this study have substantial potential for wider generalisability to other urban areas. An ultimate goal of the project was to identify evidence-based design features of the built environment that encourage physical activity and improve health behaviours. It was hoped that the identification of these environmental features would provide architects, urban designers and planners with evidence-based urban design elements, which are required for future developments.
We hope that findings from the ENABLE London study will be generalisable to other urban residential housing developments, especially given the urgent need for higher-density housing, and will help inform evidence-based urban planning in future.
Methodological investigations and development
We believe that the ENABLE London study design that we have developed offers a robust test of the effect of the built environment on physical activity and health behaviour, in the absence of randomisation. We did explore carrying out randomisation of accommodation with Triathlon Homes, a partner organisation of housing associations, which manages social and intermediate homes in East Village, but unfortunately this was not feasible. Hence, the approach used offers the next-best alternative. The richness of the data recorded, although making participation and data collection more onerous, provided an excellent opportunity for novel exploration. The development of an automated method of recording travel mode journeys from combined accelerometry and GPS data allowed change in active forms of travel to be examined in an objective automated way, which would have been prohibitively lengthy using previous manual approaches. 48,49 The tool developed (outlined in Chapter 5) has been validated in the cohort (as well as in a separate study) and although it showed little change in active forms of travel (i.e. walking and cycling), consistent with no overall change in physical activity levels (see Chapter 3), it showed modest reductions in car travel and an increased use of public transport (i.e. underground), which was associated with moving to East Village. These methods have been published and made available for use by the wider scientific community, offering an ongoing legacy from the work.
Engagement with patient and public involvement/stakeholders
The ENABLE London study was developed in partnership with a network of both local and regional stakeholders identified through our collaborator links to agencies, which were involved with the design, planning and management of large-scale accommodation developments. Locally, these included local authorities (particularly Newham) and a number of housing associations, in particular the East Thames Group and Triathlon Homes (which continue to be responsible for social and intermediate tenured housing in East Village). Open meetings held by the East Thames Group and Triathlon Homes were attended by the investigators at an early stage of the project, to directly interface with those contemplating moving to East Village, to establish the drivers and reasons for considering moving to East Village, and to ensure that these were fully captured in the forms of assessment. Moreover, qualitative work was also convened early on in the project to gauge experiences among those who were the very first to move to East Village, so that follow-up examinations could be adapted to ensure that all relevant areas were covered, and that assessments and participation remained engaging and enjoyable, and to ensure the continued significance and potential generalisability of the work. Another key aspect of engagement/stakeholder involvement was attendance and presentation at national and international conferences and special stakeholder workshops, particularly those convened by the Town and Country Planning Association at an early stage of the project, to encourage further stakeholder participation and to share experiences with other researchers carrying out similar work; this provided the opportunity for ENABLE London study methods to be refined and optimised. Similarly, publications to date, particularly those describing study methods and baseline data, also provided opportunities for further dissemination and potential input from those working within the field. The investigators continue to be in contact with representatives of the Town and Country Planning Association, Public Health England and the London Borough of Newham not only to disseminate findings from the ENABLE London study but, more importantly, to inform the future direction of research in this area, with the goal of providing stakeholders, architects and planners with the evidence base needed to inform future housing developments.
Challenges, strengths and limitations of the study
Challenges of the study
The ENABLE London study provided a unique opportunity to observe a ‘natural experiment’ to assess the impact of a rapidly changing built environment, specifically designed to encourage walking and cycling, on the physical activity patterns of residents. Natural experiments are associated with a number of challenges that are often out of the control of the researchers. Our original plan, as specified in our bid for funding, was to carry out the baseline recruitment between January 2013 and mid-2014 and then to follow up participants after 1 year, between January 2014 and mid-2015. However, there were marked delays in the release of East Village accommodation, which resulted in a prolonged period of recruitment from January 2013 to January 2016. In addition, the delayed opening of East Village facilities (a crucial element of the East Village environment and the natural experiment under evaluation) led to a postponement of the follow-up assessment from 1 year to 2 years. The scientific case for delayed follow-up was strong in that (1) East Village was not fully open for use in 2014 when first follow-up was initially envisaged and (2) previous studies have suggested that it takes 2 years as opposed to 1 year for habitual levels of physical activity to change in response to a changed built environment. 44 The scientific rationale for 2-year follow-up was approved by our Trial Steering Group. As a result, the timing of the follow-up examination (originally envisaged to take place between January 2014 and mid-2015) began in February 2015 and was completed in October 2017. We remained adaptive to these challenges throughout the study by modifying the working hours of field workers in response to demand (particularly making use of part-time as opposed to full-time appointments) to ensure that the research funding that was awarded covered the extended period of fieldwork. However, the prolonged period of fieldwork inevitably resulted in difficulties in consistency of the exposure to the built environment, which, as outlined above, was underdeveloped at first follow-up, becoming fully open and then more latterly overdeveloped from what was initially envisaged (see Limitations of the study). Moreover, the high levels of rental tenure in East Village resulted in a more mobile population, and many of the intermediate and market-rent occupants who took part in the study have since moved away from the area.
Strengths of the study
The building of East Village provided an important novel opportunity to evaluate a ‘natural experiment’ based on the major and focused change of an inner-city urban built environment that has been specifically designed to encourage walking, cycling and healthy living. It is also unique because the study included residents from widely differing socioeconomic backgrounds. Although many developments of this scale are under way or are being planned in cities globally, few have been (or are being) evaluated and most are less easily evaluated given that the timescale of their development is much longer than in the case presented by East Village. The rapid occupancy of this development was a major strength, providing the opportunity for pre and post assessment and to compare 2-year change in health outcomes among those who did and did not move to East Village. The different housing tenures within East Village also allowed for the evaluation of socioeconomic position as an effect modifier, as the impact of the built environment may vary by socioeconomic position. The focus on increasing levels of accessible and low-cost forms of physical activity remains particularly relevant to individuals and households of lower socioeconomic status and has the potential to inform efforts to reduce health inequalities. 259 This allowed us to examine whether or not the built environment favourably influenced higher levels of physical activity (particularly walking and cycling), as well as reducing time in sedentary activities, particularly among low-income groups with fewer opportunities for recreational activities. 259 Moreover, the co-location of this diverse population of differing housing tenures to one community also constituted a social experiment, providing opportunities for residents to observe and learn from the behaviour of others. 260
Another key strength is that the study sought to recruit a cohort who were seeking to move, half of whom succeeded and half of whom did not, which avoided potential biases in health behaviour. In the absence of randomisation, we believe that this study design offers the next-best alternative, providing less biased and more robust evidence about the potential effect of the built environment on health-related behaviours and outcomes. Following the same individuals before and after any move to East Village also offers statistical efficiencies in that individuals act as their own controls, which eliminates confounding factors that do not change within subject. 261,262 Moreover, the consistency of effects using 1 or 4 days of objectively measured physical activity allays fears about potential selection effects associated with using fewer days of recording, which were needed to maximise the number of study participants, particularly among the harder-to-reach social housing sector. The use of accurate objective assessment of physical activity is another strength, including assessments of MVPA that underpin current physical activity recommendations;4 this allows the potential public health importance of the findings to be gauged. However, effects on MVPA were small, suggesting that the change in the built environment had limited impact in achieving current physical activity recommendations, which focus on higher levels of activity. 4 Objective assessment of physical activity assessment reduces measurement error and potential biases arising from self-reported levels of physical activity,88 which could be artefactually associated with moving to a new environment being advocated for healthy active living. Furthermore, we have recently published a paper comparing the effect of a successful pedometer-based walking intervention on change in physical activity observed from accelerometry and IPAQ forms of assessment, and have shown that the change in physical activity was estimated with far less precision compared with the hip-worn accelerometry form of assessment. 263 Hence, use of the IPAQ data would lead to greater uncertainty about the findings, which already show no clear evidence of effect. Similarly, objective measures of adiposity using validated measures of bioimpedance minimise measurement error and limit any potential biases in ascertainment, particularly among a multiethnic population. 264,265 The ability to examine different socioeconomic groups allows social inequalities in effects and potential determinants to be examined. 266 Although there was the suggestion that change in physical activity was greater in the intermediate sector than in the social housing and market-rent groups, there were no clear differences observed by housing sector, emphasising that the study was underpowered to formally examine impacts on health inequalities.
Limitations of the study
A key limitation was that the study was powered to detect a 750-step difference. 61 Hence, the study was underpowered to show that the change in steps observed associated with moving to East Village compared with the control group was statistically significant overall (i.e. 154 steps, 95% CI –231 to 539), let alone in housing tenure subgroups with fewer participants. Effects on MVPA associated with moving to East Village (particularly in ≥ 10 bouts, commensurate with current UK physical activity guidelines)4 were small and uncertain (i.e. effect estimates were associated with wide CIs). Limitations in sample size were a result of the modest participation rates (50–60%) and unforeseen restrictions on recruitment, particularly among the market-rent sector. 61 Moreover, the staged recruitment in which those in the social sector were moved in first when East Village was not fully completed, may have resulted in partial exposure to the physical activity promoting environment and before residential density had reached capacity, which may have limited the full impact of the exposure. 61 This was partly a reason for follow-up after 2 years rather than 1 year, but also because previous work has suggested that longer durations are needed for habitual health behaviours to evolve and to avoid early ‘honeymoon’ effects44,267–269 or, conversely, for residents to become fully familiar and make optimal use of their residential area. 148 The RESIDE study found stronger differences over time, as suburbs evolved and new facilities were built and habits became more established. 95 Further follow-up could plausibly demonstrate beneficial effects but given the mobility of the cohort and the fact that the study is underpowered these are unlikely to yield statistically significant findings. Moreover, East Village is evolving and the building of high-rise accommodation (> 30 storeys) among the existing 10- to 12-storey accommodation, and subsequent loss of green space, may dampen exposure effects even further. 218
Information on social environmental factors, including qualitative research exploring the lived experience of residents, may help to explain why residents of this new area did not respond to an environment designed to be health promoting. It is plausible that the facilities provided did not meet the needs of the new residents and/or they did not feel welcome to use those facilities, especially social housing participants. 233 This requires further exploration. However, another reason why effects were not more evident might be that London is largely a ‘green city’, especially in relation to other capital cities,270 limiting the opportunities to detect change if controls moved to an equally good space. However, the effect was in fact marginally strengthened when comparing East Village with controls who moved elsewhere and the difference was reduced when comparing East Village with controls who remained at their baseline address, suggesting that this is not the case. There was also no effect of moving to East Village on adiposity levels, and no effect on mental health and well-being outcomes of public health importance.
Another limitation was the adult focus of the study, as East Village did not attract as many families as anticipated, leading to a limited number of children being recruited to participate in the study. This was also evidenced by the Chobham Academy, originally designated to educate children moving to East Village, having to increase the catchment area to fill its pupil capacity. This was an unforeseen circumstance, out of the control of the researchers, which resulted in the study being underpowered to determine any change in physical activity and health behaviours associated with moving to East Village among this age group. Hence, this limits the generalisability of the findings, particularly to younger age groups.
Implications for future research
Although it remains plausible that the built environment alters physical activity patterns and other health behaviours, this study suggests that the effects may be small, particularly, as in this case, when people relocate into new high-density neighbourhoods. There are many discussions globally about the health impacts of high-density urban living, particularly high rise, and there is an urgent need to mitigate potentially adverse consequences. 26 However, this does not mean that a small effect across a whole population is unimportant. In contrast to other community-wide interventions, which have shown limited effects on physical activity,29 change in the built environment potentially has greater reach. Even though there were modest effects on MVPA, potentially a 400 step per day change (observed in the intermediate group) across the whole population could have appreciable effects on health outcomes at a population level, potentially reducing all-cause mortality by 2% (95% CI 1% to 4%). 271 However, further evidence is required, particularly about the factors that resulted in social housing residents who relocated actually decreasing their level of physical activity. Such studies would have to be large in scale, larger than the ENABLE London study, to demonstrate change. As availability and affordability of housing is a pressing concern, particularly in major conurbations such as London, and the environments in which we live and the accompanying social context have been shown to be related to physical activity levels (including higher levels of physical activity),104,272 more studies evaluating the effect of large-scale housing redevelopment and/or new development programmes are needed to inform the debate about future housing policy. These studies should be diverse in nature to encompass high-density as well as low-density housing. Moreover, suitability and attractiveness of these housing types to different populations (young and old, different tenures and income groups) should be considered to improve the generalisability of potential findings, whether these be favourable or otherwise.
Although there is a strong scientific case for such large-scale studies, challenges of funding, especially in the current economic climate, will limit opportunities. Hence, novel approaches using simulation studies or alternative low-cost forms of population surveillance may be needed to make such large-scale studies viable.
Conclusions
There is a need to shift from the paradigm of cross-sectional studies to stronger research longitudinal designs that make use of large-scale residential relocation opportunities. Natural experiments that make use of circumstances in which people relocate to a new built environment are ideal. 41,83 However, despite the growing need for housing, especially in major conurbations such as London,273 opportunities for such studies are limited. Challenges include the need to identify and engage with proposed developments from an early stage, the need to obtain access to those planning to move well before any move occurs, the need to recruit sufficient participants, the unpredictability of creating environmental interventions as planned, and the need for flexible funding to adapt to unforeseen delays given the researchers lack of control over any potential build. Only by assimilating evidence from such studies conducted in different types of neighbourhood, which employ common methodologies that can be directly combined, can the effect of the built environment on physical activity be fully elucidated and understood to inform the planning and provision of housing for optimal health.
Acknowledgements
The authors thank the East Thames Group, Triathlon Homes and Get Living London, which assisted in recruiting participants into the ENABLE London study. The ENABLE London study was advised by a Steering Committee chaired by Professor Hazel Inskip (University of Southampton) with Dr David Ogilvie (University of Cambridge) and Professor Andy Jones (University of East Anglia) as academic advisors and Mrs Kate Worley (formerly East Thames Group Assistant Director for Strategic Housing) as the lay/stakeholder member. The authors are grateful to the members of the ENABLE London study team (in particular Aine Hogan, Katrin Peuker, Cathy McKay) and to participating households, without whom this study would not be possible. We are also grateful to Emma Ranger and Rachel Hahn (University of Bristol), who carried out spatial narratives with Bina Ram, and Lee Berney (Queen Mary, University of London), who carried out the focus groups. Emma Ranger also carried out the analysis for Chapter 8, as part of her PhD studies. Our thanks to Charlotte Wahlich (St George’s, University of London), who assisted the authors in the preparation of this report.
Contributions of authors
Christopher G Owen (https://orcid.org/0000-0003-1135-5977) (Professor of Epidemiology, St George’s, University of London) was the principal investigator leading on the design and execution of the study, oversaw all study analyses and publications and led the preparation of the final report.
Elizabeth S Limb (https://orcid.org/0000-0003-0830-7376) and Claire M Nightingale (https://orcid.org/0000-0002-4803-7617) (Senior Research Fellow and Lecturer in Medical Statistics, St George’s, University of London) were the primary statisticians for the ENABLE London study, responsible for data management, curation and analyses, leading on some and contributing to other publications emanating from the study, as well as the final report.
Alicja R Rudnicka (https://orcid.org/0000-0003-0369-8574) (Professor of Statistical Epidemiology, St George’s, University of London) co-designed and raised funding for the ENABLE London study, and oversaw analyses emanating from the study. Alicja R Rudnicka contributed to all publications emanating from the study and the final report.
Bina Ram (https://orcid.org/0000-0003-0023-1573) (Programme Manager and St George’s, University of London, PhD student) was responsible for delivery of the ENABLE London study and data collection and management. She was also responsible for the mental health and well-being assessment in the ENABLE London cohort (with support from Aparna Shankar), leading on analyses and publications, and has contributed to publications emanating from the study and the final report.
Aparna Shankar (https://orcid.org/0000-0002-7948-8189) (Senior Lecturer in Behavioural Medicine, St George’s, University of London) was responsible for the qualitative analysis chapter and has contributed to publications emanating from the study and the final report.
Steven Cummins (https://orcid.org/0000-0002-3957-4357) [Professor of Population Health, London School of Hygiene & Tropical Medicine (LSHTM)] co-designed and raised funding for the ENABLE London study, and was the lead researcher on the built environment indices used in the study, with Daniel Lewis (https://orcid.org/0000-0002-2111-4256) (Medical Research Council Skills Development Fellow, LSHTM) and Christelle Clary (https://orcid.org/0000-0001-5959-5043) (Research Fellow, LSHTM). Together they led multiple study analyses and publications and contributed to others, as well as having responsibility for built environment sections of the final report.
Ashley R Cooper (https://orcid.org/0000-0001-8644-3870) (Professor of Physical Activity and Public Health, University of Bristol) and Angie S Page (https://orcid.org/0000-0001-6427-9336) (Professor of Physical Activity and Public Health, University of Bristol) co-designed and raised funding for the ENABLE London study, and were the lead researchers on the Geographical Positioning Systems-Accelerometry assessment and spatial narratives carried out in the ENABLE London study. In association with Duncan Procter (https://orcid.org/0000-0003-1874-1205) (Research Fellow, University of Bristol), they led multiple study analyses and publications and contributed to others, and with Elizabeth S Limb (Senior Research Fellow, University of London), were responsible for travel mode sections of the final report.
Anne Ellaway (https://orcid.org/0000-0002-2117-4451) (Professor and Programme Leader of the Neighbourhoods and Communities Programme, University of Glasgow), Billie Giles-Corti (https://orcid.org/0000-0003-0102-0225) (Distinguished Professor, Director of Urban Futures Enabling Capability Platform, Royal Melbourne Institute of Technology) and Peter H Whincup (https://orcid.org/0000-0002-5589-4107) (Professor of Cardiovascular Epidemiology, University of London) co-designed and raised funding for the ENABLE London study, and contributed to the design, execution and oversight of the study in general, and contributed to study analyses and publications.
Derek G Cook (https://orcid.org/0000-0002-9723-5759) (Professor of Epidemiology, St George’s, University of London) co-designed and raised funding for the ENABLE London study, and oversaw analyses emanating from the study. Derek G Cook contributed to all publications emanating from the study and the final report.
All authors contributed to the final report and approved the final version.
Publications
Ram B, Nightingale CM, Hudda MT, Kapetanakis VV, Ellaway A, Cooper AR, et al. Cohort profile: Examining Neighbourhood Activities in Built Living Environments in London: the ENABLE London-Olympic Park cohort. BMJ Open 2016;6:e012643.
Ram B, Shankar A, Nightingale CM, Giles-Corti B, Ellaway A, Cooper AR, et al. Comparisons of depression, anxiety, well-being, and perceptions of the built environment among adults seeking social, intermediate and market-rent accommodation in the former London Olympic Athletes’ Village. Health Place 2017;48:31–9.
Nightingale CM, Rudnicka AR, Ram B, Shankar A, Limb ES, Procter D, et al. Housing, neighbourhood and sociodemographic associations with adult levels of physical activity and adiposity: baseline findings from the ENABLE London study. BMJ Open 2018;8:e021257.
Procter DS, Page AS, Cooper AR, Nightingale CM, Ram B, Rudnicka AR, et al. An open-source tool to identify active travel from hip-worn accelerometer, GPS and GIS data. Int J Behav Nutr Phys Act 2018;15:91.
Nightingale CM, Limb ES, Ram B, Shankar A, Clary C, Lewis D, et al. The effect of moving to East Village, the former London 2012 Olympic and Paralympic Games Athletes’ Village, on physical activity and adiposity (ENABLE London): a cohort study. Lancet Public Health 2019;4:e421–e430.
Limb ES, Procter DS, Cooper AR, Page AS, Nightingale CM, Ram B, et al. The effect of moving to East Village, the former London 2012 Olympic and Paralympic Games Athletes' Village, on mode of travel (ENABLE London study, a natural experiment). Int J Behav Nutr Phys Act 2020;17:15.
Clary C, Lewis D, Limb E, Nightingale CM, Ram B, Page AS, et al. Longitudinal impact of changes in the residential built environment on physical activity: findings from the ENABLE London cohort study. Int J Behav Nutr Phys Act 2020;17:96.
Clary C, Lewis D, Limb ES, Nightingale CM, Ram B, Rudnicka AR, et al. Weekend and weekday associations between the residential built environment and physical activity: Findings from the ENABLE London study. PLOS ONE 2020;15:e0237323.
Ram B, Limb ES, Shankar A, Nightingale CM, Rudnicka AR, Cummins S, et al. Evaluating the effect of change in the built environment on mental health and subjective well-being: a natural experiment. J Epidemiol Community Health 2020;74:631–8.
Data-sharing statement
We welcome proposals for collaborative projects. For general data-sharing enquiries please contact the corresponding author.
Disclaimers
This report presents independent research funded by the National Institute for Health Research (NIHR). The views and opinions expressed by authors in this publication are those of the authors and do not necessarily reflect those of the NHS, the NIHR, NETSCC, the PHR programme or the Department of Health and Social Care. If there are verbatim quotations included in this publication the views and opinions expressed by the interviewees are those of the interviewees and do not necessarily reflect those of the authors, those of the NHS, the NIHR, NETSCC, the PHR programme or the Department of Health and Social Care.
References
- Lee IM, Shiroma EJ, Lobelo F, Puska P, Blair SN, Katzmarzyk PT. Lancet Physical Activity Series Working Group . Effect of physical inactivity on major non-communicable diseases worldwide: an analysis of burden of disease and life expectancy. Lancet 2012;380:219-29. https://doi.org/10.1016/S0140-6736(12)61031-9.
- Kyu HH, Bachman VF, Alexander LT, Mumford JE, Afshin A, Estep K, et al. Physical activity and risk of breast cancer, colon cancer, diabetes mellitus , ischemic heart disease, and ischemic stroke events: systematic review and dose-response meta-analysis for the Global Burden of Disease Study 2013. BMJ 2016;354. https://doi.org/10.1136/bmj.i3857.
- Ding D, Lawson KD, Kolbe-Alexander TL, Finkelstein EA, Katzmarzyk PT, van Mechelen W, et al. Lancet Physical Activity Series 2 Executive Committee . The economic burden of physical inactivity: a global analysis of major non-communicable diseases. Lancet 2016;388:1311-24. https://doi.org/10.1016/S0140-6736(16)30383-X.
- NHS Digital . Statistics on Obesity, Physical Activity and Diet, England 2017 2017. www gov uk/government/statistics/statistics-on-obesity-physical-activity-and-diet-england-2017 (accessed July 2018).
- Department of Health and Social Care (DHSC) . Start Active, Stay Active: A Report on Physical Activity from the Four Home Countries’ Chief Medical Officers 2011.
- World Health Organization (WHO) . Global Recommendations on Physical Activity for Health 2010. www.who.int/dietphysicalactivity/publications/9789241599979/en/ (accessed July 2018).
- Craig R, Mindell J. Health Survey for England 2012: Health, Social Care and Lifestyles. Leeds: NHS Digital; 2013.
- Chinn DJ, White M, Harland J, Drinkwater C, Raybould S. Barriers to physical activity and socioeconomic position: implications for health promotion. J Epidemiol Community Health 1999;53:191-2. https://doi.org/10.1136/jech.53.3.191.
- Beenackers MA, Kamphuis CB, Giskes K, Brug J, Kunst AE, Burdorf A, et al. Socioeconomic inequalities in occupational, leisure-time, and transport related physical activity among European adults: a systematic review. Int J Behav Nutr Phys Act 2012;9. https://doi.org/10.1186/1479-5868-9-116.
- McVeigh JA, Winkler EA, Howie EK, Tremblay MS, Smith A, Abbott RA, et al. Objectively measured patterns of sedentary time and physical activity in young adults of the Raine study cohort. Int J Behav Nutr Phys Act 2016;13. https://doi.org/10.1186/s12966-016-0363-0.
- Sigmundová D, Sigmund E, Vokáčová J, Kopčáková J. Parent–child associations in pedometer-determined physical activity and sedentary behaviour on weekdays and weekends in random samples of families in the Czech Republic. Int J Environ Res Public Health 2014;11:7163-81. https://doi.org/10.3390/ijerph110707163.
- Ellaway A, Macdonald L, Kearns A. Are housing tenure and car access still associated with health? A repeat cross-sectional study of UK adults over a 13-year period. BMJ Open 2016;6. https://doi.org/10.1136/bmjopen-2016-012268.
- Macintyre S, Hiscock R, Kearns A, Ellaway A. Housing tenure and car access: further exploration of the nature of their relations with health in a UK setting. J Epidemiol Community Health 2001;55:330-1. https://doi.org/10.1136/jech.55.5.330.
- Davey-Smith GD, Egger M. Socioeconomic differences in mortality in Britain and the United States. Am J Public Health 1992;82:1079-81. https://doi.org/10.2105/AJPH.82.8.1079.
- Dalstra JA, Kunst AE, Mackenbach JP. EU Working Group on Socioeconomic Inequalities in Health . A comparative appraisal of the relationship of education, income and housing tenure with less than good health among the elderly in Europe. Soc Sci Med 2006;62:2046-60. https://doi.org/10.1016/j.socscimed.2005.09.001.
- Macintyre S, McKay L, Der G, Hiscock R. Socioeconomic position and health: what you observe depends on how you measure it. J Public Health Med 2003;25:288-94. https://doi.org/10.1093/pubmed/fdg089.
- Harrison RA, McElduff P, Edwards R. Planning to win: health and lifestyles associated with physical activity amongst 15,423 adults. Public Health 2006;120:206-12. https://doi.org/10.1016/j.puhe.2005.08.021.
- Kendig H, Browning C, Teshuva K. Health actions and social class among older Australians. Aust N Z J Public Health 1998;22:808-13. https://doi.org/10.1111/j.1467-842X.1998.tb01498.x.
- Ogilvie D, Mitchell R, Mutrie N, Petticrew M, Platt S. Personal and environmental correlates of active travel and physical activity in a deprived urban population. Int J Behav Nutr Phys Act 2008;5. https://doi.org/10.1186/1479-5868-5-43.
- Boniface DR, Cottee MJ, Neal D, Skinner A. Social and demographic factors predictive of change over seven years in CHD-related behaviours in men aged 18–49 years. Public Health 2001;115:246-52. https://doi.org/10.1038/sj/ph/1900762.
- Macintyre S, Ellaway A, Hiscock R, Kearns A, Der G, McKay L. What features of the home and the area might help to explain observed relationships between housing tenure and health? Evidence from the west of Scotland. Health Place 2003;9:207-18. https://doi.org/10.1016/S1353-8292(02)00040-0.
- Ellaway A, Macintyre S. Does housing tenure predict health in the UK because it exposes people to different levels of housing related hazards in the home or its surroundings?. Health Place 1998;4:141-50. https://doi.org/10.1016/S1353-8292(98)00006-9.
- Macintyre S, Ellaway A, Der G, Ford G, Hunt K. Do housing tenure and car access predict health because they are simply markers of income or self esteem? A Scottish study. J Epidemiol Community Health 1998;52:657-64. https://doi.org/10.1136/jech.52.10.657.
- Hoehner CM, Brennan Ramirez LK, Elliott MB, Handy SL, Brownson RC. Perceived and objective environmental measures and physical activity among urban adults. Am J Prev Med 2005;28:105-16. https://doi.org/10.1016/j.amepre.2004.10.023.
- Sallis JF, Bowles HR, Bauman A, Ainsworth BE, Bull FC, Craig CL, et al. Neighborhood environments and physical activity among adults in 11 countries. Am J Prev Med 2009;36:484-90. https://doi.org/10.1016/j.amepre.2009.01.031.
- Giles-Corti B, Ryan K, Foster S. Increasing Density in Australia: Maximising the Health Benefits and Minimising Harm – Report to the National Heart Foundation of Australia, Melbourne 2012. www.heartfoundation.org.au/images/uploads/publications/Increasing-density-in-Australia-Evidence-Review-2012-trevor.pdf.
- Foster S, Giles-Corti B. The built environment, neighborhood crime and constrained physical activity: an exploration of inconsistent findings. Prev Med 2008;47:241-51. https://doi.org/10.1016/j.ypmed.2008.03.017.
- da Silva IC, Payne VL, Hino AA, Varela AR, Reis RS, Ekelund U, et al. Physical activity and safety from crime among adults: a systematic review. J Phys Act Health 2016;13:663-70. https://doi.org/10.1123/jpah.2015-0156.
- Baker PR, Francis DP, Soares J, Weightman AL, Foster C. Community wide interventions for increasing physical activity. Cochrane Database Syst Rev 2015;1. https://doi.org/10.1002/14651858.CD008366.pub3.
- Brownson RC, Hoehner CM, Day K, Forsyth A, Sallis JF. Measuring the built environment for physical activity: state of the science. Am J Prev Med 2009;36:99-123. https://doi.org/10.1016/j.amepre.2009.01.005.
- National Institute for Health and Care Excellence (NICE) . Physical Activity and the Environment [Guideline NG90] 2018. www.nice.org.uk/guidance/ng90 (accessed July 2018).
- Sallis JF, Cerin E, Conway TL, Adams MA, Frank LD, Pratt M, et al. Physical activity in relation to urban environments in 14 cities worldwide: a cross-sectional study. Lancet 2016;387:2207-17. https://doi.org/10.1016/S0140-6736(15)01284-2.
- Ding D, Nguyen B, Learnihan V, Bauman AE, Davey R, Jalaludin B, et al. Moving to an active lifestyle? A systematic review of the effects of residential relocation on walking, physical activity and travel behaviour. Br J Sports Med 2018;52:789-99. https://doi.org/10.1136/bjsports-2017-098833.
- Ellaway A, Lamb KE, Ferguson NS, Ogilvie D. Associations between access to recreational physical activity facilities and body mass index in Scottish adults. BMC Public Health 2016;16. https://doi.org/10.1186/s12889-016-3444-8.
- Mason KE, Pearce N, Cummins S. Associations between fast food and physical activity environments and adiposity in mid-life: cross-sectional, observational evidence from UK Biobank. Lancet Public Health 2018;3:e24-e33. https://doi.org/10.1016/S2468-2667(17)30212-8.
- Kärmeniemi M, Lankila T, Ikäheimo T, Koivumaa-Honkanen H, Korpelainen R. The built environment as a determinant of physical activity: a systematic review of longitudinal studies and natural experiments. Ann Behav Med 2018;52:239-51. https://doi.org/10.1093/abm/kax043.
- van Poppel MN, Chinapaw MJ, Mokkink LB, van Mechelen W, Terwee CB. Physical activity questionnaires for adults: a systematic review of measurement properties. Sports Med 2010;40:565-600. https://doi.org/10.2165/11531930-000000000-00000.
- Rose G. The Strategy of Preventive Medicine. Oxford: Oxford University Press; 1992.
- Gebel K, Ding D, Foster C, Bauman AE, Sallis JF. Improving current practice in reviews of the built environment and physical activity. Sports Med 2015;45:297-302. https://doi.org/10.1007/s40279-014-0273-8.
- Bauman A. The physical environment and physical activity: moving from ecological associations to intervention evidence. J Epidemiol Community Health 2005;59:535-6. https://doi.org/10.1136/jech.2004.032342.
- Craig P, Cooper C, Gunnell D, Haw S, Lawson K, Macintyre S, et al. Using natural experiments to evaluate population health interventions: new Medical Research Council guidance. J Epidemiol Community Health 2012;66:1182-6. https://doi.org/10.1136/jech-2011-200375.
- East Village London E20 . About East Village London 2016. www.eastvillagelondon.co.uk/about-us (accessed July 2016).
- London Legacy Development Corporation . Your Sustainability Guide to Queen Elizabeth Olympic Park 2030 2012. www.queenelizabetholympicparkcouk/our-story/transforming-east-london/sustainability (accessed April 2016).
- Goodman A, Sahlqvist S, Ogilvie D. iConnect Consortium . New walking and cycling routes and increased physical activity: one- and 2-year findings from the UK iConnect Study. Am J Public Health 2014;104:e38-46. https://doi.org/10.2105/AJPH.2014.302059.
- Office for National Statistics . 2011 Census 2011. www.ons.gov.uk/census/2011census (accessed September 2019).
- Marmot M, Bell R. Fair society, healthy lives. Public Health 2012;126:4-10. https://doi.org/10.1016/j.puhe.2012.05.014.
- NHS Digital . Health Survey for England n.d. https://digital.nhs.uk/data-and-information/publications/statistical/health-survey-for-england (accessed September 2019).
- Cooper AR, Page AS, Wheeler BW, Griew P, Davis L, Hillsdon M, et al. Mapping the walk to school using accelerometry combined with a global positioning system. Am J Prev Med 2010;38:178-83. https://doi.org/10.1016/j.amepre.2009.10.036.
- Southward EF, Page AS, Wheeler BW, Cooper AR. Contribution of the school journey to daily physical activity in children aged 11–12 years. Am J Prev Med 2012;43:201-4. https://doi.org/10.1016/j.amepre.2012.04.015.
- Lachowycz K, Jones AP, Page AS, Wheeler BW, Cooper AR. What can global positioning systems tell us about the contribution of different types of urban greenspace to children’s physical activity?. Health Place 2012;18:586-94. https://doi.org/10.1016/j.healthplace.2012.01.006.
- Transport for London . Accessibility and Connectivity 2018. www.tfl.gov.uk/info-for/urban-planning-and-construction/transport-assessment-guide/transport-assessment-inputs/accessibility-analysis (accessed November 2018).
- Frank LD, Sallis JF, Saelens BE, Leary L, Cain K, Conway TL, et al. The development of a walkability index: application to the Neighborhood Quality of Life Study. Br J Sports Med 2010;44:924-33. https://doi.org/10.1136/bjsm.2009.058701.
- Giles-Corti B, Timperio A, Cutt H, Pikora TJ, Bull FC, Knuiman M, et al. Development of a reliable measure of walking within and outside the local neighborhood: RESIDE’s Neighborhood Physical Activity Questionnaire. Prev Med 2006;42:455-9. https://doi.org/10.1016/j.ypmed.2006.01.019.
- Saelens BE, Sallis JF, Black JB, Chen D. Neighborhood-based differences in physical activity: an environment scale evaluation. Am J Public Health 2003;93:1552-8. https://doi.org/10.2105/AJPH.93.9.1552.
- Rosenberg D, Ding D, Sallis JF, Kerr J, Norman GJ, Durant N, et al. Neighborhood Environment Walkability Scale for Youth (NEWS-Y): reliability and relationship with physical activity. Prev Med 2009;49:213-18. https://doi.org/10.1016/j.ypmed.2009.07.011.
- Office for National Statistics (ONS) . Measuring What Matters: National Statistician’s Reflections on the National Debate on Measuring National Well-Being 2011. http://webarchive.nationalarchives.gov.uk/20160105160709/http://www.ons.gov.uk/ons/guide-method/user-guidance/well-being/about-the-programme/index.html (accessed April 2016).
- Zigmond AS, Snaith RP. The hospital anxiety and depression scale. Acta Psychiatr Scand 1983;67:361-70. https://doi.org/10.1111/j.1600-0447.1983.tb09716.x.
- Brooks R. EuroQol: the current state of play. Health Policy 1996;37:53-72. https://doi.org/10.1016/0168-8510(96)00822-6.
- Craig CL, Marshall AL, Sjöström M, Bauman AE, Booth ML, Ainsworth BE, et al. International physical activity questionnaire: 12-country reliability and validity. Med Sci Sports Exerc 2003;35:1381-95. https://doi.org/10.1249/01.MSS.0000078924.61453.FB.
- Araya R, Dunstan F, Playle R, Thomas H, Palmer S, Lewis G. Perceptions of social capital and the built environment and mental health. Soc Sci Med 2006;62:3072-83. https://doi.org/10.1016/j.socscimed.2005.11.037.
- Ram B, Nightingale CM, Hudda MT, Kapetanakis VV, Ellaway A, Cooper AR, et al. Cohort profile: Examining Neighbourhood Activities in Built Living Environments in London – the ENABLE London-Olympic Park cohort. BMJ Open 2016;6. https://doi.org/10.1136/bmjopen-2016-012643.
- International Labour Organization . Resolution Concerning Statistics of the Economically Active Population, Employment, Unemployment and Underemployment n.d. www.ilo.org/global/statistics-and-databases/standards-and-guidelines/resolutions-adopted-by-international-conferences-of-labour-statisticians/WCMS_087481/lang--en/index.htm (accessed September 2019).
- Office for National Statistics (ONS) . The National Statistics Socioeconomic Classification: User Manual 2005.
- Ram B, Shankar A, Nightingale CM, Giles-Corti B, Ellaway A, Cooper AR, et al. Comparisons of depression, anxiety, well-being, and perceptions of the built environment amongst adults seeking social, intermediate and market-rent accommodation in the former London Olympic Athletes’ Village. Health Place 2017;48:31-9. https://doi.org/10.1016/j.healthplace.2017.09.001.
- Mason KE, Baker E, Blakely T, Bentley RJ. Housing affordability and mental health: does the relationship differ for renters and home purchasers?. Soc Sci Med 2013;94:91-7. https://doi.org/10.1016/j.socscimed.2013.06.023.
- Nightingale CM, Limb E, Ram B, Shankar A, Clary C, Lewis D, et al. The effect of moving to East Village, the former London 2012 Olympic and Paralympic Games Athletes’ Village, on physical activity and adiposity (ENABLE London): a cohort study. Lancet Public Health 2019;4:e421-30. https://doi.org/10.1136/jech-2018-SSMabstracts.78.
- Freedson PS, Melanson E, Sirard J. Calibration of the computer science and applications, inc. accelerometer. Med Sci Sports Exerc 1998;30:777-81. https://doi.org/10.1097/00005768-199805000-00021.
- Harris T, Kerry SM, Limb ES, Victor CR, Iliffe S, Ussher M, et al. Effect of a primary care walking intervention with and without nurse support on physical activity levels in 45- to 75-year-olds: the Pedometer And Consultation Evaluation (PACE-UP) cluster randomised clinical trial. PLOS Med 2017;14. https://doi.org/10.1371/journal.pmed.1002210.
- Mayor of London . London Development Database 2018. www.london.gov.uk/what-we-do/planning/london-plan/london-development-database (accessed November 2018).
- Nightingale CM, Rudnicka AR, Ram B, Shankar A, Limb ES, Procter D, et al. Housing, neighbourhood and sociodemographic associations with adult levels of physical activity and adiposity: baseline findings from the ENABLE London study. BMJ Open 2018;8. https://doi.org/10.1136/bmjopen-2017-021257.
- Allen MS, Vella SA. Longitudinal determinants of walking, moderate, and vigorous physical activity in Australian adults. Prev Med 2015;78:101-4. https://doi.org/10.1016/j.ypmed.2015.07.014.
- Juneau CE, Sullivan A, Dodgeon B, Côté S, Ploubidis GB, Potvin L. Social class across the life course and physical activity at age 34 years in the 1970 British birth cohort. Ann Epidemiol 2014;24. https://doi.org/10.1016/j.annepidem.2014.06.096.
- McLaren L. Socioeconomic status and obesity. Epidemiol Rev 2007;29:29-48. https://doi.org/10.1093/epirev/mxm001.
- Tranter BD, Donoghue J. Housing tenure, body mass index and health in Australia. Int J Housing Policy 2017;17:469-88. https://doi.org/10.1080/14616718.2016.1241937.
- Turrell G, Haynes M, Wilson LA, Giles-Corti B. Can the built environment reduce health inequalities? A study of neighbourhood socioeconomic disadvantage and walking for transport. Health Place 2013;19:89-98. https://doi.org/10.1016/j.healthplace.2012.10.008.
- Harrison RA, Gemmell I, Heller RF. The population effect of crime and neighbourhood on physical activity: an analysis of 15,461 adults. J Epidemiol Community Health 2007;61:34-9. https://doi.org/10.1136/jech.2006.048389.
- Brown BB, Werner CM, Smith KR, Tribby CP, Miller HJ. Physical activity mediates the relationship between perceived crime safety and obesity. Prev Med 2014;66:140-4. https://doi.org/10.1016/j.ypmed.2014.06.021.
- McGinn AP, Evenson KR, Herring AH, Huston SL, Rodriguez DA. Exploring associations between physical activity and perceived and objective measures of the built environment. J Urban Health 2007;84:162-84. https://doi.org/10.1007/s11524-006-9136-4.
- Sarmiento O, Torres A, Jacoby E, Pratt M, Schmid TL, Stierling G. The Ciclovía-Recreativa: a mass-recreational program with public health potential. J Phys Act Health 2010;7:163-80. https://doi.org/10.1123/jpah.7.s2.s163.
- Hipp JA, Eyler AA, Zieff SG, Samuelson MA. Taking physical activity to the streets: the popularity of Ciclovía and Open Streets initiatives in the United States. Am J Health Promot 2014;28:114-15. https://doi.org/10.4278/ajhp.28.3s.S114.
- Di Angelantonio E, Bhupathiraju SN, Wormser D, Gao P, Kaptoge S, . The Global BMI Mortality Collaboration . Body-mass index and all-cause mortality: individual-participant-data meta-analysis of 239 prospective studies in four continents. Lancet 2016;388:776-86. https://doi.org/10.1016/S0140-6736(16)30175-1.
- Braun LM, Rodriguez DA, Song Y, Meyer KA, Lewis CE, Reis JP, et al. Changes in walking, body mass index, and cardiometabolic risk factors following residential relocation: longitudinal results from the CARDIA study. J Transp Health 2016;3:426-39. https://doi.org/10.1016/j.jth.2016.08.006.
- Craig P, Katikireddi SV, Leyland A, Popham F. Natural experiments: an overview of methods, approaches, and contributions to public health intervention research. Annu Rev Public Health 2017;38:39-56. https://doi.org/10.1146/annurev-publhealth-031816-044327.
- Thompson Coon J, Boddy K, Stein K, Whear R, Barton J, Depledge MH. Does participating in physical activity in outdoor natural environments have a greater effect on physical and mental well-being than physical activity indoors? A systematic review. Environ Sci Technol 2011;45:1761-72. https://doi.org/10.1021/es102947t.
- Puett R, Teas J, España-Romero V, Artero EG, Lee DC, Baruth M, et al. Physical activity: does environment make a difference for tension, stress, emotional outlook, and perceptions of health status?. J Phys Act Health 2014;11:1503-11. https://doi.org/10.1123/jpah.2012-0375.
- Barnett DW, Barnett A, Nathan A, Van Cauwenberg J, Cerin E. Council on Environment and Physical Activity (CEPA) – Older Adults working group . Built environmental correlates of older adults’ total physical activity and walking: a systematic review and meta-analysis. Int J Behav Nutr Phys Act 2017;14. https://doi.org/10.1186/s12966-017-0558-z.
- Cerin E, Nathan A, van Cauwenberg J, Barnett DW, Barnett A. Council on Environment and Physical Activity (CEPA) – Older Adults working group . The neighbourhood physical environment and active travel in older adults: a systematic review and meta-analysis. Int J Behav Nutr Phys Act 2017;14. https://doi.org/10.1186/s12966-017-0471-5.
- Prince SA, Adamo KB, Hamel ME, Hardt J, Connor Gorber S, Tremblay M. A comparison of direct versus self-report measures for assessing physical activity in adults: a systematic review. Int J Behav Nutr Phys Act 2008;5. https://doi.org/10.1186/1479-5868-5-56.
- Beenackers MA, Foster S, Kamphuis CB, Titze S, Divitini M, Knuiman M, et al. Taking up cycling after residential relocation: built environment factors. Am J Prev Med 2012;42:610-15. https://doi.org/10.1016/j.amepre.2012.02.021.
- Panter J, Heinen E, Mackett R, Ogilvie D. Impact of new transport infrastructure on walking, cycling, and physical activity. Am J Prev Med 2016;50:e45-53. https://doi.org/10.1016/j.amepre.2015.09.021.
- Clark B, Chatterjee K, Melia S. Changes to commute mode: the role of life events, spatial context and environmental attitude. Transport Res A POL 2016;89:89-105. https://doi.org/10.1016/j.tra.2016.05.005.
- Christian H, Knuiman M, Bull F, Timperio A, Foster S, Divitini M, et al. A new urban planning code’s impact on walking: the residential environments project. Am J Public Health 2013;103:1219-28. https://doi.org/10.2105/AJPH.2013.301230.
- Lee IM, Ewing R, Sesso HD. The built environment and physical activity levels: the Harvard Alumni Health Study. Am J Prev Med 2009;37:293-8. https://doi.org/10.1016/j.amepre.2009.06.007.
- Giles-Corti B, Bull F, Knuiman M, McCormack G, Van Niel K, Timperio A, et al. The influence of urban design on neighbourhood walking following residential relocation: longitudinal results from the RESIDE study. Soc Sci Med 2013;77:20-3. https://doi.org/10.1016/j.socscimed.2012.10.016.
- Knuiman MW, Christian HE, Divitini ML, Foster SA, Bull FC, Badland HM, et al. A longitudinal analysis of the influence of the neighborhood built environment on walking for transportation: the RESIDE study. Am J Epidemiol 2014;180:453-61. https://doi.org/10.1093/aje/kwu171.
- Humphreys DK, Panter J, Sahlqvist S, Goodman A, Ogilvie D. Changing the environment to improve population health: a framework for considering exposure in natural experimental studies. J Epidemiol Community Health 2016;70:941-6. https://doi.org/10.1136/jech-2015-206381.
- Van Holle V, Deforche B, Van Cauwenberg J, Goubert L, Maes L, Van de Weghe N, et al. Relationship between the physical environment and different domains of physical activity in European adults: a systematic review. BMC Public Health 2012;12. https://doi.org/10.1186/1471-2458-12-807.
- Cunningham GO, Michael YL. Concepts guiding the study of the impact of the built environment on physical activity for older adults: a review of the literature. Am J Health Promot 2004;18:435-43. https://doi.org/10.4278/0890-1171-18.6.435.
- Casagrande SS, Whitt-Glover MC, Lancaster KJ, Odoms-Young AM, Gary TL. Built environment and health behaviors among African Americans: a systematic review. Am J Prev Med 2009;36:174-81. https://doi.org/10.1016/j.amepre.2008.09.037.
- Van Cauwenberg J, De Bourdeaudhuij I, De Meester F, Van Dyck D, Salmon J, Clarys P, et al. Relationship between the physical environment and physical activity in older adults: a systematic review. Health Place 2011;17:458-69. https://doi.org/10.1016/j.healthplace.2010.11.010.
- Allender S, Foster C, Scarborough P, Rayner M. The burden of physical activity-related ill health in the UK. J Epidemiol Community Health 2007;61:344-8. https://doi.org/10.1136/jech.2006.050807.
- Hallal PC, Andersen LB, Bull FC, Guthold R, Haskell W, Ekelund U. Lancet Physical Activity Series Working Group . Global physical activity levels: surveillance progress, pitfalls, and prospects. Lancet 2012;380:247-57. https://doi.org/10.1016/S0140-6736(12)60646-1.
- Roberts D, Townsend N, Foster C. Use of new guidance to profile ‘equivalent minutes’ of aerobic physical activity for adults in England reveals gender, geographical, and socioeconomic inequalities in meeting public health guidance: a cross-sectional study. Prev Med Rep 2016;4:50-6. https://doi.org/10.1016/j.pmedr.2016.05.009.
- Sallis JF, Cervero RB, Ascher W, Henderson KA, Kraft MK, Kerr J. An ecological approach to creating active living communities. Annu Rev Public Health 2006;27:297-322. https://doi.org/10.1146/annurev.publhealth.27.021405.102100.
- Macintyre S, Ellaway A, Cummins S. Place effects on health: how can we conceptualise, operationalise and measure them?. Soc Sci Med 2002;55:125-39. https://doi.org/10.1016/S0277-9536(01)00214-3.
- Dai D. Racial/ethnic and socioeconomic disparities in urban green space accessibility: where to intervene?. Landscape Urban Plan 2011;102:234-44. https://doi.org/10.1016/j.landurbplan.2011.05.002.
- Gordon-Larsen P, Nelson MC, Page P, Popkin BM. Inequality in the built environment underlies key health disparities in physical activity and obesity. Pediatrics 2006;117:417-24. https://doi.org/10.1542/peds.2005-0058.
- Powell LM, Slater S, Chaloupka FJ. The relationship between community physical activity settings and race, ethnicity and socioeconomic status. Evid Based Prevent Med 2004;1:135-44.
- Estabrooks PA, Lee RE, Gyurcsik NC. Resources for physical activity participation: does availability and accessibility differ by neighborhood socioeconomic status?. Ann Behav Med 2003;25:100-4. https://doi.org/10.1207/S15324796ABM2502_05.
- Stockton JC, Duke-Williams O, Stamatakis E, Mindell JS, Brunner EJ, Shelton NJ. Development of a novel walkability index for London, United Kingdom: cross-sectional application to the Whitehall II Study. BMC Public Health 2016;16. https://doi.org/10.1186/s12889-016-3012-2.
- Coombes E, Jones AP, Hillsdon M. The relationship of physical activity and overweight to objectively measured green space accessibility and use. Soc Sci Med 2010;70:816-22. https://doi.org/10.1016/j.socscimed.2009.11.020.
- Foster C, Hillsdon M, Jones A, Grundy C, Wilkinson P, White M, et al. Objective measures of the environment and physical activity: results of the environment and physical activity study in English adults. J Phys Act Health 2009;6:70-8. https://doi.org/10.1123/jpah.6.s1.s70.
- Cerin E, Mitáš J, Cain KL, Conway TL, Adams MA, Schofield G, et al. Do associations between objectively-assessed physical activity and neighbourhood environment attributes vary by time of the day and day of the week? IPEN adult study. Int J Behav Nutr Phys Act 2017;14. https://doi.org/10.1186/s12966-017-0493-z.
- Hirvensalo M, Telama R, Schmidt MD, Tammelin TH, Xiaolin Y, Magnussen CG, et al. Daily steps among Finnish adults: variation by age, sex, and socioeconomic position. Scand J Public Health 2011;39:669-77. https://doi.org/10.1177/1403494811420324.
- Davis MG, Fox KR, Hillsdon M, Sharp DJ, Coulson JC, Thompson JL. Objectively measured physical activity in a diverse sample of older urban UK adults. Med Sci Sports Exerc 2011;43:647-54. https://doi.org/10.1249/MSS.0b013e3181f36196.
- Hill MS, Juster Thomas, Stafford Frank P. Time, Goods, and Well-being. Ann Arbor, MI: University of Michigan; 1985.
- Kwan M-P. The uncertain geographic context problem. Ann Assoc Am Geogr 2012;102:958-68. https://doi.org/10.1080/00045608.2012.687349.
- Boone-Heinonen J, Gordon-Larsen P, Guilkey DK, Jacobs DR, Popkin BM. Environment and physical activity dynamics: the role of residential self-selection. Psychol Sport Exerc 2011;12:54-60. https://doi.org/10.1016/j.psychsport.2009.09.003.
- Petticrew M, Cummins S, Ferrell C, Findlay A, Higgins C, Hoy C, et al. Natural experiments: an underused tool for public health?. Public Health 2005;119:751-7. https://doi.org/10.1016/j.puhe.2004.11.008.
- O’Sullivan S, Morrall J. Walking distances to and from light-rail transit stations. Transport Res Rec 1996;1538:19-26. https://doi.org/10.1177/0361198196153800103.
- Frank LD, Schmid TL, Sallis JF, Chapman J, Saelens BE. Linking objectively measured physical activity with objectively measured urban form: findings from SMARTRAQ. Am J Prev Med 2005;28:117-25. https://doi.org/10.1016/j.amepre.2004.11.001.
- Perchoux C, Kestens Y, Brondeel R, Chaix B. Accounting for the daily locations visited in the study of the built environment correlates of recreational walking (the RECORD Cohort Study). Prev Med 2015;81:142-9. https://doi.org/10.1016/j.ypmed.2015.08.010.
- Greenspace Information for Greater London . Greenspace Information for Greater London CIC n.d. www.gigl.org.uk/ (accessed September 2019).
- Mayor of London . The London Plan: The Spatial Development Strategy for London Consolidated With Alterations Since 2011 2016.
- Clary C, Lewis D, Limb ES, Nightingale CM, Ram B, Rudnicka AR, et al. Weekend and weekday associations between the residential built environment and physical activity: Findings from the ENABLE London study. PLOS ONE 2020;15. https://doi.org/10.1371/journal.pone.0237323.
- Leslie E, Coffee N, Frank L, Owen N, Bauman A, Hugo G. Walkability of local communities: using geographic information systems to objectively assess relevant environmental attributes. Health Place 2007;13:111-22. https://doi.org/10.1016/j.healthplace.2005.11.001.
- Frank LD, Kerr J, Sallis JF, Miles R, Chapman J. A hierarchy of sociodemographic and environmental correlates of walking and obesity. Prev Med 2008;47:172-8. https://doi.org/10.1016/j.ypmed.2008.04.004.
- Giles-Corti B, Broomhall MH, Knuiman M, Collins C, Douglas K, Ng K, et al. Increasing walking: how important is distance to, attractiveness, and size of public open space?. Am J Prev Med 2005;28:169-76. https://doi.org/10.1016/j.amepre.2004.10.018.
- Chaix B, Simon C, Charreire H, Thomas F, Kestens Y, Karusisi N, et al. The environmental correlates of overall and neighborhood based recreational walking (a cross-sectional analysis of the RECORD Study). Int J Behav Nutr Phys Act 2014;11. https://doi.org/10.1186/1479-5868-11-20.
- Charreire H, Weber C, Chaix B, Salze P, Casey R, Banos A, et al. Identifying built environmental patterns using cluster analysis and GIS: relationships with walking, cycling and body mass index in French adults. Int J Behav Nutr Phys Act 2012;9. https://doi.org/10.1186/1479-5868-9-59.
- Troped PJ, Wilson JS, Matthews CE, Cromley EK, Melly SJ. The built environment and location-based physical activity. Am J Prev Med 2010;38:429-38. https://doi.org/10.1016/j.amepre.2009.12.032.
- Mackenbach JP, Howden-Chapman P. Houses, neighbourhoods and health. Eur J Public Health 2002;12:161-2. https://doi.org/10.1093/eurpub/12.3.161.
- Shaw M. Housing and public health. Annu Rev Public Health 2004;25:397-418. https://doi.org/10.1146/annurev.publhealth.25.101802.123036.
- Frank LD, Sallis JF, Conway TL, Chapman JE, Saelens BE, Bachman W. Many pathways from land use to health: associations between neighborhood walkability and active transportation, body mass index, and air quality. JAMA 2006;72:75-87. https://doi.org/10.1080/01944360608976725.
- Owen N, Cerin E, Leslie E, duToit L, Coffee N, Frank LD, et al. Neighborhood walkability and the walking behavior of Australian adults. Am J Prev Med 2007;33:387-95. https://doi.org/10.1016/j.amepre.2007.07.025.
- Sallis JF, Saelens BE, Frank LD, Conway TL, Slymen DJ, Cain KL, et al. Neighborhood built environment and income: examining multiple health outcomes. Soc Sci Med 2009;68:1285-93. https://doi.org/10.1016/j.socscimed.2009.01.017.
- Cao X, Mokhtarian PL, Handy SL. Do changes in neighborhood characteristics lead to changes in travel behavior? A structural equations modeling approach. Transportation 2007;34:535-56. https://doi.org/10.1007/s11116-007-9132-x.
- Handy S, Cao X, Mokhtarian PL. Self-selection in the relationship between the built environment and walking: empirical evidence from Northern California. J Am Plan Assoc 2006;72:55-74. https://doi.org/10.1080/01944360608976724.
- Boone-Heinonen J, Guilkey DK, Evenson KR, Gordon-Larsen P. Residential self-selection bias in the estimation of built environment effects on physical activity between adolescence and young adulthood. Int J Behav Nutr Phys Act 2010;7. https://doi.org/10.1186/1479-5868-7-70.
- Coogan PF, White LF, Adler TJ, Hathaway KM, Palmer JR, Rosenberg L. Prospective study of urban form and physical activity in the Black Women’s Health Study. Am J Epidemiol 2009;170:1105-17. https://doi.org/10.1093/aje/kwp264.
- Handy SL, Boarnet MG, Ewing R, Killingsworth RE. How the built environment affects physical activity: views from urban planning. Am J Prev Med 2002;23:64-73. https://doi.org/10.1016/S0749-3797(02)00475-0.
- Robinson DL. Safety in numbers in Australia: more walkers and bicyclists, safer walking and bicycling. Health Promot J Austr 2005;16:47-51. https://doi.org/10.1071/HE05047.
- Ball K, Jeffery RW, Abbott G, McNaughton SA, Crawford D. Is healthy behavior contagious: associations of social norms with physical activity and healthy eating. Int J Behav Nutr Phys Act 2010;7. https://doi.org/10.1186/1479-5868-7-86.
- Downs A. Still Stuck in Traffic: Coping with Peak-hour Traffic Congestion. Washington, DC: Brookings Institution Press; 2005.
- Wells NM, Yang Y. Neighborhood design and walking: a quasi-experimental longitudinal study. Am J Prev Med 2008;34:313-19. https://doi.org/10.1016/j.amepre.2008.01.019.
- Hirsch JA, Moore KA, Clarke PJ, Rodriguez DA, Evenson KR, Brines SJ, et al. Changes in the built environment and changes in the amount of walking over time: longitudinal results from the multi-ethnic study of atherosclerosis. Am J Epidemiol 2014;180:799-80. https://doi.org/10.1093/aje/kwu218.
- McCormack GR, Shiell A. In search of causality: a systematic review of the relationship between the built environment and physical activity among adults. Int J Behav Nutr Phys Act 2011;8. https://doi.org/10.1186/1479-5868-8-125.
- Ball K, Jeffery RW, Crawford DA, Roberts RJ, Salmon J, Timperio AF. Mismatch between perceived and objective measures of physical activity environments. Prev Med 2008;47:294-8. https://doi.org/10.1016/j.ypmed.2008.05.001.
- Santos-Lozano A, Santín-Medeiros F, Cardon G, Torres-Luque G, Bailón R, Bergmeir C, et al. Actigraph GT3X: validation and determination of physical activity intensity cut points. Int J Sports Med 2013;34:975-82. https://doi.org/10.1055/s-0033-1337945.
- McCormack GR, McLaren L, Salvo G, Blackstaffe A. Changes in objectively-determined walkability and physical activity in adults: a quasi-longitudinal residential relocation study. Int J Environ Res Public Health 2017;14. https://doi.org/10.3390/ijerph14050551.
- Chaix B, Duncan D, Vallée J, Vernez-Moudon A, Benmarhnia T, Kestens Y. The ‘residential’ effect fallacy in neighborhood and health studies: formal definition, empirical identification, and correction. Epidemiology 2017;28:789-97. https://doi.org/10.1097/EDE.0000000000000726.
- Flint E, Cummins S, Sacker A. Associations between active commuting, body fat, and body mass index: population based, cross-sectional study in the United Kingdom. BMJ 2014;349. https://doi.org/10.1136/bmj.g4887.
- Giles-Corti B, Vernez-Moudon A, Reis R, Turrell G, Dannenberg AL, Badland H, et al. City planning and population health: a global challenge. Lancet 2016;388:2912-24. https://doi.org/10.1016/S0140-6736(16)30066-6.
- Saunders LE, Green JM, Petticrew MP, Steinbach R, Roberts H. What are the health benefits of active travel? A systematic review of trials and cohort studies. PLOS ONE 2013;8. https://doi.org/10.1371/journal.pone.0069912.
- Stopher P, FitzGerald C, Xu M. Assessing the accuracy of the Sydney Household Travel Survey with GPS. Transportation 2007;34:723-41. https://doi.org/10.1007/s11116-007-9126-8.
- Adams SA, Matthews CE, Ebbeling CB, Moore CG, Cunningham JE, Fulton J, et al. The effect of social desirability and social approval on self-reports of physical activity. Am J Epidemiol 2005;161:389-98. https://doi.org/10.1093/aje/kwi054.
- Jankowska MM, Schipperijn J, Kerr J. A framework for using GPS data in physical activity and sedentary behavior studies. Exerc Sport Sci Rev 2015;43:48-56. https://doi.org/10.1249/JES.0000000000000035.
- McCrorie PR, Fenton C, Ellaway A. Combining GPS, GIS, and accelerometry to explore the physical activity and environment relationship in children and young people: a review. Int J Behav Nutr Phys Act 2014;11. https://doi.org/10.1186/s12966-014-0093-0.
- Troiano RP, McClain JJ, Brychta RJ, Chen KY. Evolution of accelerometer methods for physical activity research. Br J Sports Med 2014;48:1019-23. https://doi.org/10.1136/bjsports-2014-093546.
- Brondeel R, Pannier B, Chaix B. Using GPS, GIS, and accelerometer data to predict transportation modes. Med Sci Sports Exerc 2015;47:2669-75. https://doi.org/10.1249/MSS.0000000000000704.
- Breiman L. Random forests. Mach Learn 2001;45:5-32. https://doi.org/10.1023/A:1010933404324.
- Ellis K, Godbole S, Marshall S, Lanckriet G, Staudenmayer J, Kerr J. Identifying active travel behaviors in challenging environments using GPS, accelerometers, and machine learning algorithms. Front Public Health 2014;2. https://doi.org/10.3389/fpubh.2014.00036.
- Chen T, Guestrin C. XGBoost: A Scalable Tree Boosting System n.d.:785-94. https://doi.org/10.1145/2939672.2939785.
- Chen T. Awesome XGBoost. 2018 n.d. www.github.com/dmlc/xgboost/tree/master/demo#machine-learning-challenge-winning-solutions (accessed June 2018).
- Procter D. Modeid: A Package to Process Accelerometer and GPS Data and Identify Travel Modes 2018. www.github.com/dprocter/modeid (accessed 4 June 2018).
- Procter DS, Page AS, Cooper AR, Nightingale CM, Ram B, Rudnicka AR, et al. An open-source tool to identify active travel from hip-worn accelerometer, GPS and GIS data. Int J Behav Nutr Phys Act 2018;15. https://doi.org/10.1186/s12966-018-0724-y.
- Wijndaele K, Westgate K, Stephens SK, Blair SN, Bull FC, Chastin SF, et al. Utilization and harmonization of adult accelerometry data: review and expert consensus. Med Sci Sports Exerc 2015;47:2129-39. https://doi.org/10.1249/MSS.0000000000000661.
- Evenson KR, Catellier DJ, Gill K, Ondrak KS, McMurray RG. Calibration of two objective measures of physical activity for children. J Sports Sci 2008;26:1557-65. https://doi.org/10.1080/02640410802334196.
- Butte NF, Wong WW, Lee JS, Adolph AL, Puyau MR, Zakeri IF. Prediction of energy expenditure and physical activity in preschoolers. Med Sci Sports Exerc 2014;46:1216-26. https://doi.org/10.1249/MSS.0000000000000209.
- Zakeri IF, Adolph AL, Puyau MR, Vohra FA, Butte NF. Cross-sectional time series and multivariate adaptive regression splines models using accelerometry and heart rate predict energy expenditure of preschoolers. J Nutr 2013;143:114-22. https://doi.org/10.3945/jn.112.168542.
- Kerr J, Duncan S, Schipperijn J, Schipperjin J. Using global positioning systems in health research: a practical approach to data collection and processing. Am J Prev Med 2011;41:532-40. https://doi.org/10.1016/j.amepre.2011.07.017.
- Siła-Nowicka K, Vandrol J, Oshan T, Long JA, Demšar U, Fotheringham AS. Analysis of human mobility patterns from GPS trajectories and contextual information. Int J Geogr Inf Sci 2016;30:881-906. https://doi.org/10.1080/13658816.2015.1100731.
- Carlson JA, Jankowska MM, Meseck K, Godbole S, Natarajan L, Raab F, et al. Validity of PALMS GPS scoring of active and passive travel compared with SenseCam. Med Sci Sports Exerc 2015;47:662-7. https://doi.org/10.1249/MSS.0000000000000446.
- Baddeley A, Turner R. Spatstat: an R package for analyzing spatial point patterns. J Stat Softw 2005;12:1-42. https://doi.org/10.18637/jss.v012.i06.
- Limb ES, Procter DS, Cooper AR, Page AS, Nightingale CM, Ram B, et al. The effect of moving to East Village, the former London 2012 Olympic and Paralympic Games Athletes' Village, on mode of travel (ENABLE London study, a natural experiment). Int J Behav Nutr Phys Act 2020;17.
- World Health Organization (WHO) . Depression and Other Common Mental Health Disorders: Global Health Estimates 2017.
- GBD 2015 Disease and Injury Incidence and Prevalence Collaborators . Global, regional, and national incidence, prevalence, and years lived with disability for 310 diseases and injuries, 1990–2015: a systematic analysis for the Global Burden of Disease Study 2015. Lancet 2016;388:1545-602. https://doi.org/10.1016/S0140-6736(16)31678-6.
- Ferrari AJ, Charlson FJ, Norman RE, Patten SB, Freedman G, Murray CJ, et al. Burden of depressive disorders by country, sex, age, and year: findings from the global burden of disease study 2010. PLOS Med 2013;10. https://doi.org/10.1371/journal.pmed.1001547.
- World Health Organization (WHO), Calouste Gulbenkian Foundation . Social Determinants of Mental Health 2014.
- McManus S, Bebbington P, Jenkins R, Brugha T. Mental Health and Well-being in England: Adult Psychiatric Morbidity Survey 2014. Leeds: NHS Digital; 2016.
- Department of Health and Social Care (DHSC) . Healthy Lives, Healthy People: Our Strategy for Public Health in England 2010.
- Department of Health and Social Care (DHSC) . No Health Without Mental Health: A Cross-Government Mental Health Outcomes Strategy for People of All Ages 2011.
- Diez Roux AV, Mair C. Neighborhoods and health. Ann NY Acad Sci 2010;1186:125-45. https://doi.org/10.1111/j.1749-6632.2009.05333.x.
- Kling JR, Liebman JB, Katz LF. Experimental analysis of neighborhood effects. Econometrica 2007;75:83-119. https://doi.org/10.1111/j.1468-0262.2007.00733.x.
- Astell-Burt T, Feng X, Kolt GS, Jalaludin B. Does rising crime lead to increasing distress? Longitudinal analysis of a natural experiment with dynamic objective neighbourhood measures. Soc Sci Med 2015;138:68-73. https://doi.org/10.1016/j.socscimed.2015.05.014.
- Ellaway A, Morris G, Curtice J, Robertson C, Allardice G, Robertson R. Associations between health and different types of environmental incivility: a Scotland-wide study. Public Health 2009;123:708-13. https://doi.org/10.1016/j.puhe.2009.09.019.
- Lorenc T, Clayton S, Neary D, Whitehead M, Petticrew M, Thomson H, et al. Crime, fear of crime, environment, and mental health and well-being: mapping review of theories and causal pathways. Health Place 2012;18:757-65. https://doi.org/10.1016/j.healthplace.2012.04.001.
- James P, Hart JE, Banay RF, Laden F, Signorello LB. Built environment and depression in low-income African Americans and Whites. Am J Prev Med 2017;52:74-8. https://doi.org/10.1016/j.amepre.2016.08.022.
- Abraham A, Sommerhalder K, Abel T. Landscape and well-being: a scoping study on the health-promoting impact of outdoor environments. Int J Public Health 2010;55:59-6. https://doi.org/10.1007/s00038-009-0069-z.
- Shanahan DF, Bush R, Gaston KJ, Lin BB, Dean J, Barber E, et al. Health benefits from nature experiences depend on dose. Sci Rep 2016;6. https://doi.org/10.1038/srep28551.
- Conolly AM. Improving People’s Health Through Spatial Planning. London: Public Health England; 2017.
- Braubach M, Fairburn J. Social inequities in environmental risks associated with housing and residential location: a review of evidence. Eur J Public Health 2010;20:36-42. https://doi.org/10.1093/eurpub/ckp221.
- Braubach M, Jacobs DE, Ormandy D. Summary Report: Environmental Burden of Disease Associated With Inadequate Housing 2011.
- Stafford M, Marmot M. Neighbourhood deprivation and health: does it affect us all equally?. Int J Epidemiol 2003;32:357-66. https://doi.org/10.1093/ije/dyg084.
- Ferrer-i-Carbonell A, Gowdy JM. Environmental degradation and happiness. Ecol Econ 2007;60:509-16. https://doi.org/10.1016/j.ecolecon.2005.12.005.
- Lelkes O. Knowing what is good for you: empirical analysis of personal preferences and the ‘objective good’. J Behav Exp Econ 2006;35:285-307. https://doi.org/10.1016/j.socec.2005.11.002.
- GL Assessment . Hospital and Depression Anxiety Scale 2015. www.gl-assessment.co.uk/products/hospital-anxiety-and-depression-scale/hospital-anxiety-and-depression-scale-faqs#FAQ4 (accessed July 2016).
- Office for National Statistics . First Annual ONS Experimental Subjective Well-Being Results 2012.
- Akhtar-Danesh N, Landeen J. Relation between depression and sociodemographic factors. Int J Ment Health Syst 2007;1. https://doi.org/10.1186/1752-4458-1-4.
- Dolan P, Peasgood T, White M. Do we really know what makes us happy? A review of the economic literature on the factors associated with subjective well-being. J Econ Psychol 2008;29:94-122. https://doi.org/10.1016/j.joep.2007.09.001.
- Birtchnell J, Masters N, Deahl M. Depression and the physical environment: a study of young married women on a London housing estate. Br J Psychiatry 1988;153:56-64. https://doi.org/10.1192/bjp.153.1.56.
- Sooman A, Macintyre S. Health and perceptions of the local environment in socially contrasting neighbourhoods in Glasgow. Health Place 1995;1:15-26. https://doi.org/10.1016/1353-8292(95)00003-5.
- Hunt S, McKenna SP. The impact of housing quality of mental and physical health. Housing Review 1992;41:47-9.
- Cummins S, Stafford M, Macintyre S, Marmot M, Ellaway A. Neighbourhood environment and its association with self rated health: evidence from Scotland and England. J Epidemiol Community Health 2005;59:207-13. https://doi.org/10.1136/jech.2003.016147.
- Leslie E, Cerin E. Are perceptions of the local environment related to neighbourhood satisfaction and mental health in adults?. Prev Med 2008;47:273-8. https://doi.org/10.1016/j.ypmed.2008.01.014.
- Ludwig J, Duncan GJ, Gennetian LA, Katz LF, Kessler RC, Kling JR, et al. Long-term neighborhood effects on low-income families: evidence from moving to opportunity. Am Econ Rev 2013;103:226-31. https://doi.org/10.1257/aer.103.3.226.
- Moore THM, Kesten JM, López-López JA, Ijaz S, McAleenan A, Richards A, et al. The effects of changes to the built environment on the mental health and well-being of adults: systematic review. Health Place 2018;53:237-57. https://doi.org/10.1016/j.healthplace.2018.07.012.
- Ludwig J, Duncan GJ, Gennetian LA, Katz LF, Kessler RC, Kling JR, et al. Neighborhood effects on the long-term well-being of low-income adults. Science 2012;337:1505-10. https://doi.org/10.1126/science.1224648.
- Ludwig J, Sanbonmatsu L, Gennetian L, Adam E, Duncan GJ, Katz LF, et al. Neighborhoods, obesity, and diabetes mellitus: a randomized social experiment. N Engl J Med 2011;365:1509-19. https://doi.org/10.1056/NEJMsa1103216.
- Kessler RC, Duncan GJ, Gennetian LA, Katz LF, Kling JR, Sampson NA, et al. Associations of housing mobility interventions for children in high-poverty neighborhoods with subsequent mental disorders during adolescence. JAMA 2014;311:937-48. https://doi.org/10.1001/jama.2014.607.
- Mehdipanah R, Rodríguez-Sanz M, Malmusi D, Muntaner C, Díez E, Bartoll X, et al. The effects of an urban renewal project on health and health inequalities: a quasi-experimental study in Barcelona. J Epidemiol Community Health 2014;68:811-7. https://doi.org/10.1136/jech-2013-203434.
- Jongeneel-Grimen B, Droomers M, Kramer D, Bruggink JW, van Oers H, Kunst AE, et al. Impact of a Dutch urban regeneration programme on mental health trends: a quasi-experimental study. J Epidemiol Community Health 2016;70:967-73. https://doi.org/10.1136/jech-2015-207016.
- Egan M, Katikireddi SV, Kearns A, Tannahill C, Kalacs M, Bond L. Health effects of neighborhood demolition and housing improvement: a prospective controlled study of 2 natural experiments in urban renewal. Am J Public Health 2013;103:e47-53. https://doi.org/10.2105/AJPH.2013.301275.
- Egan M, Kearns A, Katikireddi SV, Curl A, Lawson K, Tannahill C. Proportionate universalism in practice? A quasi-experimental study (GoWell) of a UK neighbourhood renewal programme’s impact on health inequalities. Soc Sci Med 2016;152:41-9. https://doi.org/10.1016/j.socscimed.2016.01.026.
- Lorenc T, Petticrew M, Whitehead M, Neary D, Clayton S, Wright K, et al. Fear of crime and the environment: systematic review of UK qualitative evidence. BMC Public Health 2013;13. https://doi.org/10.1186/1471-2458-13-496.
- Huxley P, Evans S, Leese M, Gately C, Rogers A, Thomas R, et al. Urban regeneration and mental health. Soc Psychiatry Psychiatr Epidemiol 2004;39:280-5. https://doi.org/10.1007/s00127-004-0739-3.
- Cummins S, Clark C, Lewis D, Smith N, Thompson C, Smuk M, et al. The effects of the London 2012 Olympics and related urban regeneration on physical and mental health: the ORiEL mixed-methods evaluation of a natural experiment. Public Health Res 2018;6. https://doi.org/10.3310/phr06120.
- London Legacy Development Corporation . Plot N08 East Village Ref: 14 00034 REM n.d. www.london.gov.uk/moderngovlldc/documents/b10635/Minutes%20-%20Appendix%204%20-%20Presentation%20-%20N08%20Zone%205%20Stratford%20City%20East%20Village%20Tuesday%2027-May-2014%20.pdf?T=9 (accessed 11 July 2019).
- Kearns A, Tannahill C, Bond L. Regeneration and health: conceptualising the connections. J Urb Regen Ren 2009;3:56-6.
- World Health Organization (WHO) . Closing the Gap in a Generation: Health and Equity Through Action on the Social Determinants of Health: Final Report of the Commission on Social Determinants of Health 2008.
- Thompson S, Kent J. Connecting and strengthening communities in places for health and well-being. Aust Planner 2014;51:260-71. https://doi.org/10.1080/07293682.2013.837832.
- Davis J, Thornley A. Urban regeneration for the London 2012 Olympics: issues of land acquisition and legacy. CCS 2010;1:89-98. https://doi.org/10.1016/j.ccs.2010.08.002.
- Minnaert L. An Olympic legacy for all? The non-infrastructural outcomes of the Olympic Games for socially excluded groups (Atlanta 1996–Beijing 2008). Tourism Manage 2012;33:361-70. https://doi.org/10.1016/j.tourman.2011.04.005.
- Wellings K, Datta J, Wilkinson P, Petticrew M. The 2012 Olympics: assessing the public health effect. Lancet 2011;378:1193-5. https://doi.org/10.1016/S0140-6736(11)60550-3.
- Thompson C, Lewis D, Greenhalgh T, Taylor S, Cummins S. A health and social legacy for East London: narratives of ‘problem’ and ‘solution’ around London 2012. Sociol Res Online 2013;18:1-6. https://doi.org/10.5153/sro.2966.
- McCartney G, Thomas S, Thomson H, Scott J, Hamilton V, Hanlon P, et al. The health and socioeconomic impacts of major multi-sport events: systematic review (1978–2008). BMJ 2010;340. https://doi.org/10.1136/bmj.c2369.
- Watt P, Bernstock P, Cohen P, Watt P. London 2012 and the Post-Olympics City: A Hollow Legacy?. London: Palgrave Macmillan; 2017.
- Bernstock P. Olympic Housing: A Critical Review of London 2012’s Legacy. London: Routledge; 2014.
- Watt P. ‘It’s not for us’: regeneration, the 2012 Olympics and the gentrification of East London. City 2013;17:99-118. https://doi.org/10.1080/13604813.2012.754190.
- Clark C, Smuk M, Cummins S, Eldridge S, Fahy A, Lewis D, et al. An Olympic legacy? Did the urban regeneration associated with the London 2012 Olympic Games influence adolescent mental health?. Am J Epidemiol 2018;187:474-83. https://doi.org/10.1093/aje/kwx205.
- Chatterjee R, Hemmings S. The Olympic healthcare legacy: a study to investigate the perceptions of relevant stakeholders to see how the 2012 Olympics have affected the health and well-being of children in East London by use of semi-structured interviews. Am J Sports Med 2018;6:60-6. https://doi.org/10.12691/ajssm-6-2-4.
- Crockett N, Cohen P, Humphry D. Speaking Out of Place: End of Project Report 2016. https://eprints.kingston.ac.uk/40415/6/Humphry-D-40415-VoR.pdf (accessed November 2019).
- Wilson S. Shared Spaces, Shared Lives? Striving for Mixed Communities in Stratford’s East Village 2016.
- Finch H, Lewis J, Ritchie J, Spencer J. Qualitative Research Practice. London: SAGE Publications; 2003.
- Braun V, Clarke V. Using thematic analysis in psychology. Qual Res Psychol 2006;3:77-101. https://doi.org/10.1191/1478088706qp063oa.
- Bond L, Sautkina E, Kearns A. Mixed messages about mixed tenure: do reviews tell the real story?. Housing Studies 2011;26:69-94. https://doi.org/10.1080/02673037.2010.512752.
- Cohen P, Cohen P, Watt P. London 2012 and the Post-Olympics City: A Hollow Legacy?. London: Macmillan Publishers; 2017.
- Bauman AE, Reis RS, Sallis JF, Wells JC, Loos RJ, Martin BW. Lancet Physical Activity Series Working Group . Correlates of physical activity: why are some people physically active and others not?. Lancet 2012;380:258-71. https://doi.org/10.1016/S0140-6736(12)60735-1.
- Sahlqvist S, Song Y, Ogilvie D. Is active travel associated with greater physical activity? The contribution of commuting and non-commuting active travel to total physical activity in adults. Prev Med 2012;55:206-11. https://doi.org/10.1016/j.ypmed.2012.06.028.
- Sahlqvist S, Goodman A, Cooper AR, Ogilvie D. iConnect consortium . Change in active travel and changes in recreational and total physical activity in adults: longitudinal findings from the iConnect study. Int J Behav Nutr Phys Act 2013;10. https://doi.org/10.1186/1479-5868-10-28.
- Audrey S, Procter S, Cooper AR. The contribution of walking to work to adult physical activity levels: a cross-sectional study. Int J Behav Nutr Phys Act 2014;11. https://doi.org/10.1186/1479-5868-11-37.
- Trost SG, Owen N, Bauman AE, Sallis JF, Brown W. Correlates of adults’ participation in physical activity: review and update. Med Sci Sports Exerc 2002;34:1996-2001. https://doi.org/10.1249/01.MSS.0000038974.76900.92.
- Lovasi GS, Grady S, Rundle A. Steps forward: review and recommendations for research on walkability, physical activity and cardiovascular health. Public Health Rev 2012;33:484-506. https://doi.org/10.1007/BF03391647.
- Pucher J, Dill J, Handy S. Infrastructure, programs, and policies to increase bicycling: an international review. Prev Med 2010;50:106-25. https://doi.org/10.1016/j.ypmed.2009.07.028.
- Fishman E, Schepers P, Kamphuis CB. Dutch cycling: quantifying the health and related economic benefits. Am J Public Health 2015;105:e13-5. https://doi.org/10.2105/AJPH.2015.302724.
- Gössling S. Urban transport transitions: Copenhagen, City of Cyclists. J Transp Geogr 2013;33:196-20. https://doi.org/10.1016/j.jtrangeo.2013.10.013.
- National Institute for Health and Care Excellence (NICE) . Physical Activity: Walking and Cycling 2012. www.nice.org.uk/guidance/ph41 (accessed January 2019).
- Kelly P, Kahlmeier S, Götschi T, Orsini N, Richards J, Roberts N, et al. Systematic review and meta-analysis of reduction in all-cause mortality from walking and cycling and shape of dose response relationship. Int J Behav Nutr Phys Act 2014;11. https://doi.org/10.1186/s12966-014-0132-x.
- Pikora T, Giles-Corti B, Bull F, Jamrozik K, Donovan R. Developing a framework for assessment of the environmental determinants of walking and cycling. Soc Sci Med 2003;56:1693-703. https://doi.org/10.1016/S0277-9536(02)00163-6.
- Salvo G, Lashewicz BM, Doyle-Baker PK, McCormack GR. Neighbourhood built environment influences on physical activity among adults: a systematized review of qualitative evidence. Int J Environ Res Public Health 2018;15. https://doi.org/10.3390/ijerph15050897.
- Lim SS, Vos T, Flaxman AD, Danaei G, Shibuya K, Adair-Rohani H, et al. A comparative risk assessment of burden of disease and injury attributable to 67 risk factors and risk factor clusters in 21 regions, 1990-2010: a systematic analysis for the Global Burden of Disease Study 2010. Lancet 2012;380:2224-60. https://doi.org/10.1016/S0140-6736(12)61766-8.
- Department of Health and Social Care (DHSC) . 2009 Annual Report of the Chief Medical Officer 2009. www.sthc.co.uk/Documents/CMO_Report_2009.pdf (accessed August 2013).
- Ekelund U, Ward HA, Norat T, Luan J, May AM, Weiderpass E, et al. Physical activity and all-cause mortality across levels of overall and abdominal adiposity in European men and women: the European Prospective Investigation into Cancer and Nutrition Study (EPIC). Am J Clin Nutr 2015;101:613-21. https://doi.org/10.3945/ajcn.114.100065.
- Adams J, Mytton O, White M, Monsivais P. Why are some population interventions for diet and obesity more equitable and effective than others? The role of individual agency. PLOS Med 2016;13. https://doi.org/10.1371/journal.pmed.1001990.
- National Obesity Observatory . Knowledge and Attitudes Towards Healthy Eating and Physical Activity: What the Data Tell Us 2011. http://publichealthwell.ie/node/90472 (accessed May 2019).
- Hollands GJ, Shemilt I, Marteau TM, Jebb SA, Kelly MP, Nakamura R, et al. Altering micro-environments to change population health behaviour: towards an evidence base for choice architecture interventions. BMC Public Health 2013;13. https://doi.org/10.1186/1471-2458-13-1218.
- Craig R, Mindell J, Hirani V. Health Survey for England 2008: Volume 1 – Physical Activity and Fitness. Leeds: NHS Digital; 2009.
- Townsend N, Wickramasinghe K, Williams J, Bhatnagar P, Rayner M. Physical Activity Statistics 2015. London: British Heart Foundation; 2015.
- Marmot M, Allen J, Goldblatt P, Boyce T, McNeish D, Grady M, et al. Fair Society, Healthy Lives: The Marmot Review – Strategic Review of Health Inequalities in England Post-2010 2010. www.parliament.uk/documents/fair-society-healthy-lives-full-report.pdf (accessed August 2013).
- Stokols D, Allen J, Bellingham RL. The social ecology of health promotion: implications for research and practice. Am J Health Promot 1996;10:247-51. https://doi.org/10.4278/0890-1171-10.4.247.
- Vickers AJ, Altman DG. Statistics notes: analysing controlled trials with baseline and follow up measurements. BMJ 2001;323:1123-4. https://doi.org/10.1136/bmj.323.7321.1123.
- Egbewale BE, Lewis M, Sim J. Bias, precision and statistical power of analysis of covariance in the analysis of randomized trials with baseline imbalance: a simulation study. BMC Med Res Methodol 2014;14. https://doi.org/10.1186/1471-2288-14-49.
- Limb ES, Ahmad S, Cook DG, Kerry SM, Ekelund U, Whincup PH, et al. Measuring change in trials of physical activity interventions: a comparison of self-report questionnaire and accelerometry within the PACE-UP trial. Int J Behav Nutr Phys Act 2019;16. https://doi.org/10.1186/s12966-018-0762-5.
- Deurenberg P, Yap M, van Staveren WA. Body mass index and percent body fat: a meta analysis among different ethnic groups. Int J Obes Relat Metab Disord 1998;22:1164-71. https://doi.org/10.1038/sj.ijo.0800741.
- Gallagher D, Visser M, Sepúlveda D, Pierson RN, Harris T, Heymsfield SB. How useful is body mass index for comparison of body fatness across age, sex, and ethnic groups?. Am J Epidemiol 1996;143:228-39. https://doi.org/10.1093/oxfordjournals.aje.a008733.
- Kawachi I, Kennedy BP. Health and social cohesion: why care about income inequality?. BMJ 1997;314:1037-40. https://doi.org/10.1136/bmj.314.7086.1037.
- Scheiner J, Holz-Rau C. Changes in travel mode use after residential relocation: a contribution to mobility biographies. Transportation 2013;40:431-58. https://doi.org/10.1007/s11116-012-9417-6.
- Klinger T, Lanzendorf M. Moving between mobility cultures: what affects the travel behaviour of new residents?. Transportation 2016;43:243-71. https://doi.org/10.1007/s11116-014-9574-x.
- Zhu X, Yu CY, Lee C, Lu Z, Mann G. A retrospective study on changes in residents’ physical activities, social interactions, and neighborhood cohesion after moving to a walkable community. Prev Med 2014;69:93-7. https://doi.org/10.1016/j.ypmed.2014.08.013.
- City of London Corporation . Green Spaces: The Benefits for London 2013. www.cityoflondon.gov.uk/business/economic-research-and-information/research-publications/Documents/research-2013/Green-Spaces-The-Benefits-for-London.pdf (accessed August 2018).
- Dwyer T, Pezic A, Sun C, Cochrane J, Venn A, Srikanth V, et al. Objectively measured daily steps and subsequent long term all-cause mortality: the tasped prospective cohort study. PLOS ONE 2015;10. https://doi.org/10.1371/journal.pone.0141274.
- Smith L, Panter J, Ogilvie D. Characteristics of the environment and physical activity in midlife: findings from UK Biobank. Prev Med 2019;118:150-8. https://doi.org/10.1016/j.ypmed.2018.10.024.
- Mayor of London . London Housing Strategy: Implementation Plan 2018. www.london.gov.uk/sites/default/files/2018_lhs_implementation_plan_rev1.pdf (accessed August 2018).
Appendix 1 Main questionnaire
Appendix 2 ENABLE London semistructured interview schedule
List of abbreviations
- BMI
- body mass index
- CI
- confidence interval
- CMD
- common mental disorder
- CPM
- counts per minute
- ENABLE London
- Examining Neighbourhood Activities in Built Living Environments in London
- GiGL
- Greenspace Information for Greater London
- GIS
- geographic information system
- GPS
- Global Positioning System
- IPAQ
- International Physical Activity Questionnaire
- IQR
- interquartile range
- LLI
- limiting longstanding illness
- LSHTM
- London School of Hygiene & Tropical Medicine
- MVPA
- moderate to vigorous physical activity
- NS-SEC
- National Statistics Socioeconomic Classification
- OR
- odds ratio
- OS
- Ordnance Survey
- PTAL
- public transport accessibility level
- RESIDE
- RESIDential Environment
- SD
- standard deviation
- STAMP-2
- Sedentary Time and Metabolic Health in People with type-2 diabetes mellitus
- TfL
- Transport for London
Notes
-
Questionnaire items included in the factor analysis on perceptions of the neighbourhood
-
Participant characteristics for 1240 adults with measurements of adiposity at baseline
-
Mean levels of adiposity and physical activity by participant characteristics
-
Associations between participant characteristics and physical activity variables among those with at least 4 days of physical activity data
-
Physical activity differences between weekday (Monday–Friday) and weekend (Saturday, Sunday) activity by housing sector
-
Baseline sociodemographic characteristics, physical activity and adiposity outcomes by housing sector for those followed up and not followed up
-
Summary data at baseline and follow-up for neighbourhood perception scores and built environment variables, overall and by housing sector
-
Sociodemographic characteristics of the ENABLE London participants included in ( n = 1064) and excluded from ( n = 214) the baseline analyses
-
Baseline sociodemographic characteristics of the ENABLE London participants included in ( n = 687) and excluded from ( n = 591) the longitudinal analyses
-
Definition, operationalisation and aggregation unit for the three residential built environment variables: street connectivity, residential density and land use mix
-
Description, number and size of metropolitan, district and local parks
-
Data sources and versions used for computing the residential built environmental variables
-
Daily steps and MVPA at weekends and on weekdays plotted against quintile of residential built environmental factors
-
Sensitivity analyses: baseline sociodemographic characteristics and daily steps of the ENABLE London participants by aspirational housing tenure ( n = 837)
-
Sensitivity analyses: regression estimates for the cross–sectional associations of daily steps and MVPA with residential built environment factors ( n = 837)
-
Sensitivity analyses: mean differences in daily steps and MVPA compared with intermediate housing seekers: effect of adjustment for residential built environment factors ( n = 837)
-
Within-person change (baseline to follow-up) in residential built environment characteristics by aspirational housing tenure and combined ( n = 687), for the East Village group and the control group
-
Associations of neighbourhood perceptions with mental health and well-being
-
Associations of change in scores of neighbourhood perceptions with change in mental health and well-being
Supplementary material can be found on the NIHR Journals Library report page (https://doi.org/10.3310/phr08120).
Supplementary material has been provided by the authors to support the report and any files provided at submission will have been seen by peer reviewers, but not extensively reviewed. Any supplementary material provided at a later stage in the process may not have been peer reviewed.